#Scraped Tweets Data
Explore tagged Tumblr posts
Text

#ScrapeTweetsDataUsingPython#ScrapeTweetsDataUsingsnscrape#ExtractingTweetsusingSnscrape#Tweets Data Collection#Scraped Tweets Data
0 notes
Text
source
not sure if someone already made a post about this....
#tweet#x#platform formaly known as twitter#twitter#tumblr#'AI discussion'#'AI discourse'#'AI'#'Data scraping'
7 notes
·
View notes
Text
Fascinated that the owners of social media sites see API usage and web scraping as "data pillaging" -- immoral theft! Stealing! and yet, if you or I say that we should be paid for the content we create on social media the idea is laughed out of the room.
Social media is worthless without people and all the things we create do and say.
It's so valuable that these boys are trying to lock it in a vault.
#socail media#data mining#web scraping#twitter#reddit#you are the product#free service#free as in privacy invasion#pay me for that banger tweet you wretched nerd
8 notes
·
View notes
Text
Does this not just make Twitter…more unprofitable than it already is?
#‘people will buy the check marks!’ I guarantee you. most will not.#I’m not tech savvy so forgive me but how does limit how many tweets a person can read lessen data scraping and system manipulation#what? like stealing a person’s data to have more target ads?#rip Twitter sorry Elon bought you :/#twitters like a Petri dish full of bacteria but it deserved a better way to die. not via capitalistic money hungry murder
2 notes
·
View notes
Text
Twitter Data Scraping Services -Twitter Data Collection Services
Scrape data like profile handle, followers count, etc., using our Twitter data scraping services. Our Twitter data collection services are functional across the USA, UK, etc.
know more:
#Twitter Data Scraping Services#Twitter Data Collection Services#Scrape Data from Twitter Social Media App#Web scraping Twitter data#Twitter data scraper#Scrape Tweets data from Twitter
0 notes
Text
AI Scraping Isn't Just Art And Fanfic
Something I haven't really seen mentioned and I think people may want to bear in mind is that while artists are the most heavily impacted by AI visual medium scraping, it's not like the machine knows or cares to differentiate between original art and a photograph of your child.
AI visual media scrapers take everything, and that includes screengrabs, photographs, and memes. Selfies, pictures of your pets and children, pictures of your home, screengrabs of images posted to other sites -- all of the comic book imagery I've posted that I screengrabbed from digital comics, images of tweets (including the icons of peoples' faces in those tweets) and instas and screengrabs from tiktoks. I've posted x-ray images of my teeth. All of that will go into the machine.
That's why, at least I think, Midjourney wants Tumblr -- after Instagram we are potentially the most image-heavy social media site, and like Instagram we tag our content, which is metadata that the scraper can use.
So even if you aren't an artist, unless you want to Glaze every image of any kind that you post, you probably want to opt out of being scraped. I'm gonna go ahead and say we've probably already been scraped anyway, so I don't think there's a ton of point in taking down your tumblr or locking down specific images, but I mean...especially if it's stuff like pictures of children or say, a fundraising photo that involves your medical data, it maybe can't hurt.
If you do want to officially opt out, which may help if there's a class-action lawsuit later, you're going to want to go to the gear in the upper-right corner on the Tumblr desktop site, select each of your blogs from the list on the right-hand side, and scroll down to "Visibility". Select "Prevent third party sharing for [username]" to flip that bad boy on.
Per notes: for the app, go to your blog (the part of the app that shows what you post) and hit the gear in the upper right, then select "visibility" and it will be the last option. If you have not updated your app, it will not appear (confirmed by me, who cannot see it on my elderly version of the app).
You don't need to do it on both desktop and mobile -- either one will opt you out -- but on the app you may need to load each of your sideblogs in turn and then go back into the gear and opt out for that blog, like how you have to go into the settings for each sideblog on desktop and do it.
5K notes
·
View notes
Text
How to Scrape Tweets Data by Location Using Python and snscrape?

In this blog, we will take a comprehensive look into scraping Python wrapper and its functionality and specifically focus on using it to search for tweets based on location. We will also delve into why the wrapper may not always perform as expected. Let's dive in
snscrape is a remarkable Python library that enables users to scrape tweets from Twitter without the need for personal API keys. With its lightning-fast performance, it can retrieve thousands of tweets within seconds. Moreover, snscrape offers powerful search capabilities, allowing for highly customizable queries. While the documentation for scraping tweets by location is currently limited, this blog aims to comprehensively introduce this topic. Let's delve into the details:
Introduction to Snscrape: Snscrape is a feature-rich Python library that simplifies scraping tweets from Twitter. Unlike traditional methods that require API keys, snscrape bypasses this requirement, making it accessible to users without prior authorization. Its speed and efficiency make it an ideal choice for various applications, from research and analysis to data collection.
The Power of Location-Based Tweet Scraping: Location-based tweet scraping allows users to filter tweets based on geographical coordinates or place names. This functionality is handy for conducting location-specific analyses, monitoring regional trends, or extracting data relevant to specific areas. By leveraging Snscrape's capabilities, users can gain valuable insights from tweets originating in their desired locations.
Exploring Snscrape's Location-Based Search Tools: Snscrape provides several powerful tools for conducting location-based tweet searches. Users can effectively narrow their search results to tweets from a particular location by utilizing specific parameters and syntax. This includes defining the search query, specifying the geographical coordinates or place names, setting search limits, and configuring the desired output format. Understanding and correctly using these tools is crucial for successful location-based tweet scraping.
Overcoming Documentation Gaps: While snscrape is a powerful library, its documentation on scraping tweets by location is currently limited. This article will provide a comprehensive introduction to the topic to bridge this gap, covering the necessary syntax, parameters, and strategies for effective location-based searches. Following the step-by-step guidelines, users can overcome the lack of documentation and successfully utilize snscrape for their location-specific scraping needs.
Best Practices and Tips: Alongside exploring Snscrape's location-based scraping capabilities, this article will also offer best practices and tips for maximizing the efficiency and reliability of your scraping tasks. This includes handling rate limits, implementing error-handling mechanisms, ensuring data consistency, and staying updated with any changes or updates in Snscrape's functionality.
Introduction of snscrape Using Python
In this blog, we’ll use tahe development version of snscrape that can be installed withpip install git+https://github.com/JustAnotherArchivist/snscrape.git
Note: this needs Python 3.8 or latest
Some familiarity of the Pandas module is needed.





We encourage you to explore and experiment with the various features of snscrape to better understand its capabilities. Additionally, you can refer to the mentioned article for more in-depth information on the subject. Later in this blog, we will delve deeper into the user field and its significance in tweet scraping. By gaining a deeper understanding of these concepts, you can harness the full potential of snscrape for your scraping tasks.
Advanced Search Features

In this code snippet, we define the search query as "pizza near:Los Angeles within:10km", which specifies that we want to search for tweets containing the word "pizza" near Los Angeles within a radius of 10 km. The TwitterSearchScraper object is created with the search query, and then we iterate over the retrieved tweets and print their content.
Feel free to adjust the search query and radius per your specific requirements.
For comparing results, we can utilize an inner merging on two DataFrames:common_rows = df_coord.merge(df_city, how='inner')
That returns 50 , for example, they both have the same rows.
What precisely is this place or location?
When determining the location of tweets on Twitter, there are two primary sources: the geo-tag associated with a specific tweet and the user's location mentioned in their profile. However, it's important to note that only a small percentage of tweets (approximately 1-2%) are geo-tagged, making it an unreliable metric for location-based searches. On the other hand, many users include a location in their profile, but it's worth noting that these locations can be arbitrary and inaccurate. Some users provide helpful information like "London, England," while others might use humorous or irrelevant descriptions like "My Parents' Basement."
Despite the limited availability and potential inaccuracies of geo-tagged tweets and user profile locations, Twitter employs algorithms as part of its advanced search functionality to interpret a user's location based on their profile. This means that when you look for tweets through coordinates or city names, the search results will include tweets geotagged from the location and tweets posted by users who have that location (or a location nearby) mentioned in their profile.

To illustrate the usage of location-based searching on Twitter, let's consider an example. Suppose we perform a search for tweets near "London." Here are two examples of tweets that were found using different methods:
The first tweet is geo-tagged, which means it contains specific geographic coordinates indicating its location. In this case, the tweet was found because of its geo-tag, regardless of whether the user has a location mentioned in their profile or not.
The following tweet isn’t geo-tagged, which means that it doesn't have explicit geographic coordinates associated with it. However, it was still included in the search results because a user has given a location in the profile that matches or is closely associated with London.
When performing a location-based search on Twitter, you can come across tweets that are either geo-tagged or have users with matching or relevant locations mentioned in their profiles. This allows for a more comprehensive search, capturing tweets from specific geographic locations and users who have declared their association with those locations.
Get Location From Scraped Tweets
If you're using snscrape to scrape tweets and want to extract the user's location from the scraped data, you can do so by following these steps. In the example below, we scrape 50 tweets within a 10km radius of Los Angeles, store the data in a DataFrame, and then create a new column to capture the user's location.


If It Doesn’t Work According to Your Expectations
The use of the near: and geocode: tags in Twitter's advanced search can sometimes yield inconsistent results, especially when searching for specific towns, villages, or countries. For instance, while searching for tweets nearby Lewisham, the results may show tweets from a completely different location, such as Hobart, Australia, which is over 17,000 km away.
To ensure more accurate results when scraping tweets by locations using snscrape, it is recommended to use the geocode tag having longitude & latitude coordinates, along with a specified radius, to narrow down the search area. This approach will provide more reliable and precise results based on the available data and features.
Conclusion
In conclusion, the snscrape Python module is a valuable tool for conducting specific and powerful searches on Twitter. Twitter has made significant efforts to convert user input locations into real places, enabling easy searching by name or coordinates. By leveraging its capabilities, users can extract relevant information from tweets based on various criteria.
For research, analysis, or other purposes, snscrape empowers users to extract valuable insights from Twitter data. Tweets serve as a valuable source of information. When combined with the capabilities of snscrape, even individuals with limited experience in Data Science or subject knowledge can undertake exciting projects.
Happy scrapping!
For more details, you can contact Actowiz Solutions anytime! Call us for all your mobile app scraping and web scraping services requirements.
sources :https://www.actowizsolutions.com/how-to-scrape-tweets-data-by-location-using-python-and-snscrape.php
#Tag :#Scrape Tweets Data Location#Scrape Tweets Data Using Python#Scrape Tweets Data Using snscrape#Twitter Scraper#Scrape Twitter Data#TwitterData Scraping Services
0 notes
Text
So, the research project I have been working on where I study antisemitism on social media has effectively been ended due to how Elon Musk has changed the price of API and put an arbitrary limit on the number of tweets we can data scrape. Maybe I shouldn't think that this is a direct response to our research institute, but I do. Our research has been published in numerous academic journals, has been brought before congress, and has been featured in many articles (recently, the LA Times). All of our research points to antisemitism and racism spiking after Elon took over. The platform has become significantly worse, and we are able to quantify and map this as we have done this project for over ten years. His "free speech" commitment has led to a tolerance of bigotry and made the platform unsafe for Jews and other minorities.
#the project will be moving to another platform#so ill still have a job#and the project will continue just in a different form#but yeah thats where its at rn#jumblr#judaism#jewish#frumblr#antisemitism#jewblr#twitter
331 notes
·
View notes
Text
OTW’s Legal Chair is Pro-AI and What That Means
traHoooooooo boy. Okay, so for those who don’t know, OTW shared in their little newsletter on May 6th an interview their legal chair did on AI.
Most people didn’t notice...Until a couple hours ago when I guess more high profile accounts caught wind and now every time I refresh the tweet that links the newsletter that’s another 10+ quote tweets.
The interview itself is short, was done in February, and...Has some gross stuff.
Essentially Betsy Rosenblatt agrees with Stability AI that its fair use, and believes that AI is “reading fanfic”.
To be EXTREMELY clear: Generative AI like ChatGPT is not sentient. No AI is sentient, and Generative AI are actually incredibly simple as far as AI goes. Generative AI cannot “read”, it cannot “comprehend” and it cannot “learn”.
In fact, all Generative AI can do is spit out an output created out of a dataset. Its output is reliant on there being variables for it to spit back out. Therefore, it cannot be separated from its dataset or its “training”.
Additionally, the techbros who make these things are profiting off them, are not actually transforming anything, and oh yeah, are stealing people’s private data in order to make these datasets.
All this to say: Betsy Rosenblatt does not actually understand AI, has presumably fallen for the marketing behind Generative AI, and is not fit to legally fight for fic writers.
So what does this mean? Well, don’t delete your accounts just yet. This is just one person, belonging to a nonprofit that supposedly listens to its users. There’s a huge backlash on social media right now because yeah, people are pissed. Which is good.
We should absolutely use social media to be clear about our stances. To tell @transformativeworks that we are not okay with tech bros profiting off our fanworks, and their legal team should be fighting back against those who have already scraped our fanworks rather than lauding a program for doing things its incapable of doing.
I have fanfic up on Ao3. I have fanfic I’m working on that I’d love to put there too. But I cannot if it turns out the one safe haven for ficwriters is A-Okay with random people stealing our work and profiting off of it.
#ao3#otw#transformative works#otw discourse#fuck generative ai#everyone who thinks its okay to steal people's artwork and writing to make a quick buck can go fuck themselves#i already have to deal with this shit on a professional level i don't need it in my hobby too
309 notes
·
View notes
Text
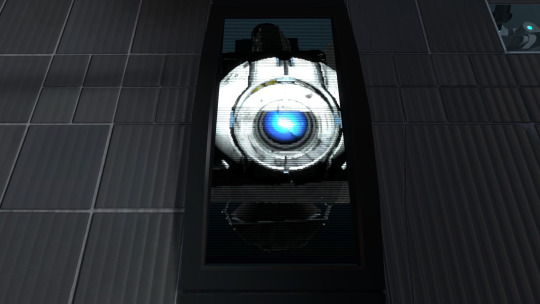
“Alright so there I was, looking at all the data scraping and system… manipulation, and I thought to myself ‘You know maybe this wouldn’t be as bad if people couldn’t tweet as much.’ So I had a bit of a brainwave: I’m going to limit the amount of posts that people can see each day. Thought around 400, 600 should be enough for most of them. I also took the liberty of giving the Twitter Blue accounts a higher amount of posts per day, so that should make those blue checkmarks a lot more appealing as well. Two birds and one stone, as they say.”
225 notes
·
View notes
Text
How to Scrape Tweets Data by Location Using Python and snscrape?

In this blog, we will take a comprehensive look into scraping Python wrapper and its functionality and specifically focus on using it to search for tweets based on location. We will also delve into why the wrapper may not always perform as expected. Let's dive in
snscrape is a remarkable Python library that enables users to scrape tweets from Twitter without the need for personal API keys. With its lightning-fast performance, it can retrieve thousands of tweets within seconds. Moreover, snscrape offers powerful search capabilities, allowing for highly customizable queries. While the documentation for scraping tweets by location is currently limited, this blog aims to comprehensively introduce this topic. Let's delve into the details:
Introduction to Snscrape: Snscrape is a feature-rich Python library that simplifies scraping tweets from Twitter. Unlike traditional methods that require API keys, snscrape bypasses this requirement, making it accessible to users without prior authorization. Its speed and efficiency make it an ideal choice for various applications, from research and analysis to data collection.
The Power of Location-Based Tweet Scraping: Location-based tweet scraping allows users to filter tweets based on geographical coordinates or place names. This functionality is handy for conducting location-specific analyses, monitoring regional trends, or extracting data relevant to specific areas. By leveraging Snscrape's capabilities, users can gain valuable insights from tweets originating in their desired locations.
Exploring Snscrape's Location-Based Search Tools: Snscrape provides several powerful tools for conducting location-based tweet searches. Users can effectively narrow their search results to tweets from a particular location by utilizing specific parameters and syntax. This includes defining the search query, specifying the geographical coordinates or place names, setting search limits, and configuring the desired output format. Understanding and correctly using these tools is crucial for successful location-based tweet scraping.
Overcoming Documentation Gaps: While snscrape is a powerful library, its documentation on scraping tweets by location is currently limited. This article will provide a comprehensive introduction to the topic to bridge this gap, covering the necessary syntax, parameters, and strategies for effective location-based searches. Following the step-by-step guidelines, users can overcome the lack of documentation and successfully utilize snscrape for their location-specific scraping needs.
Best Practices and Tips: Alongside exploring Snscrape's location-based scraping capabilities, this article will also offer best practices and tips for maximizing the efficiency and reliability of your scraping tasks. This includes handling rate limits, implementing error-handling mechanisms, ensuring data consistency, and staying updated with any changes or updates in Snscrape's functionality.
Introduction of snscrape Using Python
In this blog, we’ll use tahe development version of snscrape that can be installed withpip install git+https://github.com/JustAnotherArchivist/snscrape.git
Note: this needs Python 3.8 or latest
Some familiarity of the Pandas module is needed.
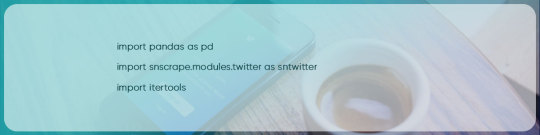




We encourage you to explore and experiment with the various features of snscrape to better understand its capabilities. Additionally, you can refer to the mentioned article for more in-depth information on the subject. Later in this blog, we will delve deeper into the user field and its significance in tweet scraping. By gaining a deeper understanding of these concepts, you can harness the full potential of snscrape for your scraping tasks.
Advanced Search Features
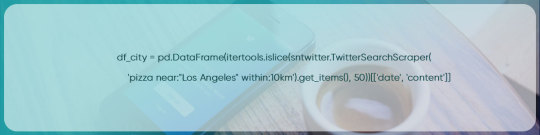
In this code snippet, we define the search query as "pizza near:Los Angeles within:10km", which specifies that we want to search for tweets containing the word "pizza" near Los Angeles within a radius of 10 km. The TwitterSearchScraper object is created with the search query, and then we iterate over the retrieved tweets and print their content.
Feel free to adjust the search query and radius per your specific requirements.
For comparing results, we can utilize an inner merging on two DataFrames:common_rows = df_coord.merge(df_city, how='inner')
That returns 50 , for example, they both have the same rows.
What precisely is this place or location?
When determining the location of tweets on Twitter, there are two primary sources: the geo-tag associated with a specific tweet and the user's location mentioned in their profile. However, it's important to note that only a small percentage of tweets (approximately 1-2%) are geo-tagged, making it an unreliable metric for location-based searches. On the other hand, many users include a location in their profile, but it's worth noting that these locations can be arbitrary and inaccurate. Some users provide helpful information like "London, England," while others might use humorous or irrelevant descriptions like "My Parents' Basement."
Despite the limited availability and potential inaccuracies of geo-tagged tweets and user profile locations, Twitter employs algorithms as part of its advanced search functionality to interpret a user's location based on their profile. This means that when you look for tweets through coordinates or city names, the search results will include tweets geotagged from the location and tweets posted by users who have that location (or a location nearby) mentioned in their profile.
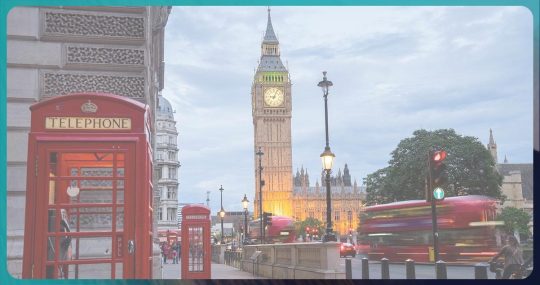
To illustrate the usage of location-based searching on Twitter, let's consider an example. Suppose we perform a search for tweets near "London." Here are two examples of tweets that were found using different methods:
The first tweet is geo-tagged, which means it contains specific geographic coordinates indicating its location. In this case, the tweet was found because of its geo-tag, regardless of whether the user has a location mentioned in their profile or not.
The following tweet isn’t geo-tagged, which means that it doesn't have explicit geographic coordinates associated with it. However, it was still included in the search results because a user has given a location in the profile that matches or is closely associated with London.
When performing a location-based search on Twitter, you can come across tweets that are either geo-tagged or have users with matching or relevant locations mentioned in their profiles. This allows for a more comprehensive search, capturing tweets from specific geographic locations and users who have declared their association with those locations.
Get Location From Scraped Tweets
If you're using snscrape to scrape tweets and want to extract the user's location from the scraped data, you can do so by following these steps. In the example below, we scrape 50 tweets within a 10km radius of Los Angeles, store the data in a DataFrame, and then create a new column to capture the user's location.


If It Doesn’t Work According to Your Expectations
The use of the near: and geocode: tags in Twitter's advanced search can sometimes yield inconsistent results, especially when searching for specific towns, villages, or countries. For instance, while searching for tweets nearby Lewisham, the results may show tweets from a completely different location, such as Hobart, Australia, which is over 17,000 km away.
To ensure more accurate results when scraping tweets by locations using snscrape, it is recommended to use the geocode tag having longitude & latitude coordinates, along with a specified radius, to narrow down the search area. This approach will provide more reliable and precise results based on the available data and features.
Conclusion
In conclusion, the snscrape Python module is a valuable tool for conducting specific and powerful searches on Twitter. Twitter has made significant efforts to convert user input locations into real places, enabling easy searching by name or coordinates. By leveraging its capabilities, users can extract relevant information from tweets based on various criteria.
For research, analysis, or other purposes, snscrape empowers users to extract valuable insights from Twitter data. Tweets serve as a valuable source of information. When combined with the capabilities of snscrape, even individuals with limited experience in Data Science or subject knowledge can undertake exciting projects.
Happy scrapping!
For more details, you can contact Actowiz Solutions anytime! Call us for all your mobile app scraping and web scraping services requirements.
#ScrapeTweetsDataUsingPython#ScrapeTweetsDataUsingsnscrape#ExtractingTweetsusingSnscrape#Tweets Data Collection#Scraped Tweets Data
1 note
·
View note
Text
Far-right populists are significantly more likely to spread fake news on social media than politicians from mainstream or far-left parties, according to a study which argues that amplifying misinformation is now part and parcel of radical right strategy. “Radical right populists are using misinformation as a tool to destabilise democracies and gain political advantage,” said Petter Törnberg of the University of Amsterdam, a co-author of the study with Juliana Chueri of the Dutch capital’s Free University. “The findings underscore the urgent need for policymakers, researchers, and the public to understand and address the intertwined dynamics of misinformation and radical right populism,” Törnberg added. The research draws on every tweet posted between 2017 and 2022 by every member of parliament with a Twitter (now X) account in 26 countries: 17 EU members including Austria, France, Germany, the Netherlands and Sweden, but also the UK, US and Australia. It then compared that dataset – 32m tweets from 8,198 MPs – with international political science databases containing detailed information on the parties involved, such as their position on the left-right spectrum and their degree of populism. Finally, the researchers scraped factchecking and fake news-tracking services to build a dataset of 646,058 URLs, each with an associated “factuality rating” based on the reliability of its source – and compared that data with the 18m URLs shared by the MPs. By crunching all the different datasets together, the researchers were able to create what they described as an aggregate “factuality score” for each politician and each party, based on the links that MPs had shared on Twitter. The data showed conclusively that far-right populism was “the strongest determinant for the propensity to spread misinformation”, they concluded, with MPs from centre-right, centre-left and far-left populist parties “not linked” to the practice.
continue reading
It's the only way they can win, i.e. by telling lies.
5 notes
·
View notes
Text
While the finer points of running a social media business can be debated, one basic truth is that they all run on attention. Tech leaders are incentivized to grow their user bases so there are more people looking at more ads for more time. It’s just good business.
As the owner of Twitter, Elon Musk presumably shared that goal. But he claimed he hadn’t bought Twitter to make money. This freed him up to focus on other passions: stopping rival tech companies from scraping Twitter’s data without permission—even if it meant losing eyeballs on ads.
Data-scraping was a known problem at Twitter. “Scraping was the open secret of Twitter data access. We knew about it. It was fine,” Yoel Roth wrote on the Twitter alternative Bluesky. AI firms in particular were notorious for gobbling up huge swaths of text to train large language models. Now that those firms were worth a lot of money, the situation was far from fine, in Musk’s opinion.
In November 2022, OpenAI debuted ChatGPT, a chatbot that could generate convincingly human text. By January 2023, the app had over 100 million users, making it the fastest growing consumer app of all time. Three months later, OpenAI secured another round of funding that closed at an astounding valuation of $29 billion, more than Twitter was worth, by Musk’s estimation.
OpenAI was a sore subject for Musk, who’d been one of the original founders and a major donor before stepping down in 2018 over disagreements with the other founders. After ChatGPT launched, Musk made no secret of the fact that he disagreed with the guardrails that OpenAI put on the chatbot to stop it from relaying dangerous or insensitive information. “The danger of training AI to be woke—in other words, lie—is deadly,” Musk said on December 16, 2022. He was toying with starting a competitor.
Near the end of June 2023, Musk launched a two-part offensive to stop data scrapers, first directing Twitter employees to temporarily block “logged out view.” The change would mean that only people with Twitter accounts could view tweets.
“Logged out view” had a complicated history at Twitter. It was rumored to have played a part in the Arab Spring, allowing dissidents to view tweets without having to create a Twitter account and risk compromising their anonymity. But it was also an easy access point for people who wanted to scrape Twitter data.
Once Twitter made the change, Google was temporarily blocked from crawling Twitter and serving up relevant tweets in search results—a move that could negatively impact Twitter’s traffic. “We’re aware that our ability to crawl Twitter.com has been limited, affecting our ability to display tweets and pages from the site in search results,” Google spokesperson Lara Levin told The Verge. “Websites have control over whether crawlers can access their content.” As engineers discussed possible workarounds on Slack, one wrote: “Surely this was expected when that decision was made?”
Then engineers detected an “explosion of logged in requests,” according to internal Slack messages, indicating that data scrapers had simply logged in to Twitter to continue scraping. Musk ordered the change to be reversed.
On July 1, 2023, Musk launched part two of the offensive. Suddenly, if a user scrolled for just a few minutes, an error message popped up. “Sorry, you are rate limited,” the message read. “Please wait a few moments then try again.”
Rate limiting is a strategy that tech companies use to constrain network traffic by putting a cap on the number of times a user can perform a specific action within a given time frame (a mouthful, I know). It’s often used to stop bad actors from trying to hack into people’s accounts. If a user tries an incorrect password too many times, they see an error message and are told to come back later. The cost of doing this to someone who has forgotten their password is low (most people stay logged in), while the benefit to users is very high (it prevents many people’s accounts from getting compromised).
Except, that wasn’t what Musk had done. The rate limit that he ordered Twitter to roll out on July 1 was an API limit, meaning Twitter had capped the number of times users could refresh Twitter to look for new tweets and see ads. Rather than constrain users from performing a specific action, Twitter had limited all user actions. “I realize these are draconian rules,” a Twitter engineer wrote on Slack. “They are temporary. We will reevaluate the situation tomorrow.”
At first, Blue subscribers could see 6,000 posts a day, while nonsubscribers could see 600 (enough for just a few minutes of scrolling), and new nonsubscriber accounts could see just 300. As people started hitting the limits, #TwitterDown started trending on, well, Twitter. “This sucks dude you gotta 10X each of these numbers,” wrote user @tszzl.
The impact quickly became obvious. Companies that used Twitter direct messages as a customer service tool were unable to communicate with clients. Major creators were blocked from promoting tweets, putting Musk’s wish to stop data scrapers at odds with his initiative to make Twitter more creator friendly. And Twitter’s own trust and safety team was suddenly stopped from seeing violative tweets.
Engineers posted frantic updates in Slack. “FYI some large creators complaining because rate limit affecting paid subscription posts,” one said.
Christopher Stanley, the head of information security, wrote with dismay that rate limits could apply to people refreshing the app to get news about a mass shooting or a major weather event. “The idea here is to stop scrapers, not prevent people from obtaining safety information,” he wrote. Twitter soon raised the limits to 10,000 (for Blue subscribers), 1,000 (for nonsubscribers), and 500 (for new nonsubscribers). Now, 13 percent of all unverified users were hitting the rate limit.
Users were outraged. If Musk wanted to stop scrapers, surely there were better ways than just cutting off access to the service for everyone on Twitter.
“Musk has destroyed Twitter’s value & worth,” wrote attorney Mark S. Zaid. “Hubris + no pushback—customer empathy—data = a great way to light billions on fire,” wrote former Twitter product manager Esther Crawford, her loyalties finally reversed.
Musk retweeted a joke from a parody account: “The reason I set a ‘View Limit’ is because we are all Twitter addicts and need to go outside.”
Aside from Musk, the one person who seemed genuinely excited about the changes was Evan Jones, a product manager on Twitter Blue. For months, he’d been sending executives updates regarding the anemic signup rates. Now, Blue subscriptions were skyrocketing. In May, Twitter had 535,000 Blue subscribers. At $8 per month, this was about $4.2 million a month in subscription revenue. By early July, there were 829,391 subscribers—a jump of about $2.4 million in revenue, not accounting for App Store fees.
“Blue signups still cookin,” he wrote on Slack above a screenshot of the signup dashboard.
Jones’s team capitalized on the moment, rolling out a prompt to upsell users who’d hit the rate limit and encouraging them to subscribe to Twitter Blue. In July, this prompt drove 1.7 percent of the Blue subscriptions from accounts that were more than 30 days old and 17 percent of the Blue subscriptions from accounts that were less than 30 days old.
Twitter CEO Linda Yaccarino was notably absent from the conversation until July 4, when she shared a Twitter blog post addressing the rate limiting fiasco, perhaps deliberately burying the news on a national holiday.
“To ensure the authenticity of our user base we must take extreme measures to remove spam and bots from our platform,” it read. “That’s why we temporarily limited usage so we could detect and eliminate bots and other bad actors that are harming the platform. Any advance notice on these actions would have allowed bad actors to alter their behavior to evade detection.” The company also claimed the “effects on advertising have been minimal.”
If Yaccarino’s role was to cover for Musk’s antics, she was doing an excellent job. Twitter rolled back the limits shortly after her announcement. On July 12, Musk debuted a generative AI company called xAI, which he promised would develop a language model that wouldn’t be politically correct. “I think our AI can give answers that people may find controversial even though they are actually true,” he said on Twitter Spaces.
Unlike the rival AI firms he was trying to block, Musk said xAI would likely train on Twitter’s data.
“The goal of xAI is to understand the true nature of the universe,” the company said grandly in its mission statement, echoing Musk’s first, disastrous town hall at Twitter. “We will share more information over the next couple of weeks and months.”
In November 2023, xAI launched a chatbot called Grok that lacked the guardrails of tools like ChatGPT. Musk hyped the release by posting a screenshot of the chatbot giving him a recipe for cocaine. The company didn’t appear close to understanding the nature of the universe, but per haps that’s coming.
Excerpt adapted from Extremely Hardcore: Inside Elon Musk’s Twitter by Zoë Schiffer. Published by arrangement with Portfolio Books, a division of Penguin Random House LLC. Copyright © 2024 by Zoë Schiffer.
20 notes
·
View notes
Text
At least this place is working....Twitter is straight up unusable right now good lord. This morning they put into place some "rate limit" to prevent "data scraping" and said it's temporary but we'll see how long that lasts....considering this started around 8 am this morning for me and it is now almost 3 pm
Trying to view your dash, refresh your dash, view your profile, read replies, search any term/hashtag just results in:
And apparently the rate limits are:
I don't even know if "reading" means just viewing a tweet as you're scrolling by it, or clicking on the actual tweet to "read it"....but I'm assuming it means just scrolling by stuff on your feed as I hit my "limit" in less then 20 mins of browsing the site/my feed.
But right now the site is unusable.
64 notes
·
View notes
Text
Currently fully abandoning twitter because it's already a shithole, it's getting worse, about to start scraping all your data for ai training and it's a soon to be state propaganda site. So I've started tracing accounts to other platforms.
Went through my follows and now going through my bookmarks and it is an absolutely miserable process. But here's some things that have made it slightly more bearable.
I used it as a lurker mostly as a means to see art so that's where these tips are coming from.
You can get an archive of your twitter data.
Settings -> Your Account -> Download an archive of your data.
It takes a while so check the email your account is linked to. It has your tweets, retweets, DMs, etc. I used it to get my likes which is given to you in a big ass list of links. Also be aware that it does NOT archive your bookmarks.
Sky Follower Bridge
Finds the Bluesky accounts of your follows, followers or list members from their twitter name or links in their bio. It's far from perfect as their Bluesky name has to match their handle or display name perfectly or it doesn't work. But it will hopefully cut down a fair bit of work in finding people.
If an artist has a page there, it will have a list of links to their profiles across the internet. It's a lot more useful than you'd think it'd be because even a ton of small artists have pages. Though they are only as complete as the people who create and edits the pages makes them so it can be hit or miss.
Danbooru
I'd suggest searching with (artist name) + danbooru as the in-site search is janky with aliases.
Most Eastern artists, especially Japanese ones, use Pixiv. It's an annoying site to use because it locks basic features behind paywalls (Like sorting by most popular and the fucking ability to blacklist tags.) But a large majority of them can be found there.
Danbooru has a lot of porn on it (without filters by default) in addition to sfw drawings. Just be warned.
Pixiv
If you know a way to archive bookmarks definitely share that because all the methods I saw are broken and I'm pretty sure the only way to archive it is by doing it manually which is awful. Also these are just the methods I used for myself, please feel free to share others.
#twitter#bluesky#twitter exodus#tumblr#elon musk#us politics#not touhou#but i wanted to share because of how unbelievably fucked up twitter is right now
4 notes
·
View notes
Text
The biggest dataset used for AI image generators had CSAM in it
Link the original tweet with more info
The LAION dataset has had ethical concerns raised over its contents before, but the public now has proof that there was CSAM used in it.
The dataset was essentially created by scraping the internet and using a mass tagger to label what was in the images. Many of the images were already known to contain identifying or personal information, and several people have been able to use EU privacy laws to get images removed from the dataset.
However, LAION itself has known about the CSAM issue since 2021.
LAION was a pretty bad data set to use anyway, and I hope researchers drop it for something more useful that was created more ethically. I hope that this will lead to a more ethical databases being created, and companies getting punished for using unethical databases. I hope the people responsible for this are punished, and the victims get healing and closure.
12 notes
·
View notes