#data security and AI
Explore tagged Tumblr posts
Text
Why Did India’s Finance Ministry Restrict the Use of AI Tools in Offices? A Closer Look at the Decision
In a significant move, India’s Finance Ministry recently issued an advisory restricting the use of artificial intelligence (AI) tools, such as ChatGPT, Bard, and other generative AI platforms, in government offices. This decision has sparked widespread debate, with many questioning the rationale behind it. Why would a government, in an era of rapid technological advancement, curb the use of tools that promise efficiency and innovation? Let’s delve into the logic and reasoning behind this decision, including the geopolitical implications and the growing global AI race, particularly with China. Read more
#Finance Ministry India AI ban#AI tools restriction India#data security and AI#geopolitical AI race#China AI development#AI governance India#ChatGPT and DeepSeek ban in government#AI and national security#indigenous AI solutions#ethical AI use in government.
2 notes
·
View notes
Text
What is AI-Ready Data? How to Get Your There?

Data powers AI systems, enabling them to generate insights, predict outcomes, and transform decision-making. However, AI’s impact hinges on the quality and readiness of the data it consumes. A recent Harvard Business Review report reveals a troubling trend: approximately 80% of AI projects fail, largely due to poor data quality, irrelevant data, and a lack of understanding of AI-specific data requirements.
As AI technologies are projected to contribute up to $15.7 trillion to the global economy by 2030, the emphasis on AI-ready data is more urgent than ever. Investing in data readiness is not merely technical; it’s a strategic priority that shapes AI’s effectiveness and a company’s competitive edge in today’s data-driven landscape.
(Source: Statista)
Achieving AI-ready data requires addressing identified gaps by building strong data management practices, prioritizing data quality enhancements, and using technology to streamline integration and processing. By proactively tackling these issues, organizations can significantly improve data readiness, minimize AI project risks, and unlock AI’s full potential to fuel innovation and growth.
In this article, we’ll explore what constitutes AI-ready data and why it is vital for effective AI deployment. We will also examine the primary obstacles to data readiness, the characteristics that define AI-ready data, and the practices for data preparation. Furthermore, well discuss how to align data with specific use-case requirements. By understanding these elements, businesses can ensure their data is not only AI-ready but optimized to deliver substantial value.
Key Takeaways:
AI-ready data is essential for maximizing the efficiency and impact of AI applications, as it ensures data quality, structure, and contextual relevance.
Achieving AI-ready data requires addressing data quality, completeness, and consistency, which ultimately enhances model accuracy and decision-making.
AI-ready data enables faster, more reliable AI deployment, reducing time-to-market and increasing operational agility across industries.
Building AI-ready data involves steps like cataloging relevant datasets, assessing data quality, consolidating data sources, and implementing governance frameworks.
AI-ready data aligns with future technologies like generative AI, positioning businesses to adapt to advancements and leverage scalable, next-generation solutions.
What is AI-Ready Data?
AI-ready data refers to data that is meticulously prepared, organized, and structured for optimized use in artificial intelligence applications. This concept goes beyond simply accumulating large data volumes; it demands data that is accurate, relevant, and formatted specifically for AI processes. With AI-ready data, every element is curated for compatibility with AI algorithms, ensuring data can be swiftly analyzed and interpreted.

High quality: AI-ready data is accurate, complete, and free from inconsistencies. These factors ensure that AI algorithms function without bias or error.
Relevant Structure: It is organized according to the AI model’s needs, ensuring seamless integration and enhancing processing efficiency.
Contextual Value: Data must provide contextual depth, allowing AI systems to extract and interpret meaningful insights tailored to specific use cases.
In essence, AI-ready data isn’t abundant, it’s purposefully refined to empower AI-driven solutions and insights.
Key Characteristics of AI-Ready Data

High Quality
For data to be truly AI-ready, it must demonstrate high quality across all metrics—accuracy, consistency, and reliability. High-quality data minimizes risks, such as incorrect insights or inaccurate predictions, by removing errors and redundancies. When data is meticulously validated and free from inconsistencies, AI models can perform without the setbacks caused by “noisy” or flawed data. This ensures AI algorithms work with precise inputs, producing trustworthy results that bolster strategic decision-making.
Structure Format
While AI systems can process unstructured data (e.g., text, images, videos), structured data vastly improves processing speed and accuracy. Organized in databases or tables, structured data is easier to search, query, and analyze, significantly reducing the computational burden on AI systems. With AI-ready data in structured form, models can perform complex operations and deliver insights faster, supporting agile and efficient AI applications. For instance, structured financial or operational data enables rapid trend analysis, fueling responsive decision-making processes.
Comprehensive Coverage
AI-ready data must cover a complete and diverse spectrum of relevant variables. This diversity helps AI algorithms account for different scenarios and real-world complexities, enhancing the model’s ability to make accurate predictions.
For example, an AI model predicting weather patterns would benefit from comprehensive data, including temperature, humidity, wind speed, and historical patterns. With such diversity, the AI model can better understand patterns, make reliable predictions, and adapt to new situations, boosting overall decision quality.
Timeline and Relevance
For data to maintain its AI readiness, it must be current and pertinent to the task. Outdated information can lead AI models to make erroneous predictions or irrelevant decisions, especially in dynamic fields like finance or public health. AI-ready data integrates recent updates and aligns closely with the model’s goals, ensuring that insights are grounded in present-day realities. For instance, AI systems for fraud detection rely on the latest data patterns to identify suspicious activities effectively, leveraging timely insights to stay a step ahead of evolving threats.
Data Integrity and Security
Security and integrity are foundational to trustworthy AI-ready data. Data must remain intact and safe from breaches to preserve its authenticity and reliability. With robust data integrity measures—like encryption, access controls, and validation protocols—AI-ready data can be protected from unauthorized alterations or leaks. This security not only preserves the quality of the AI model but also safeguards sensitive information, ensuring compliance with privacy standards. In healthcare, for instance, AI models analyzing patient data require stringent security to protect patient privacy and trust.
Key Drivers of AI-Ready Data

Understanding the drivers behind the demand for AI-ready data is essential. Organizations can harness the power of AI technologies better by focusing on these factors.
Vendor-Provided Models
Many AI models, especially in generative AI, come from external vendors. To fully unlock their potential, businesses must optimize their data. Pre-trained models thrive on high-quality, structured data. By aligning their data with these models’ requirements, organizations can maximize results and streamline AI integration. This compatibility ensures that AI-ready data empowers enterprises to achieve impactful outcomes, leveraging vendor expertise effectively.
Data Availability and Quality
Quality data is indispensable for effective AI performance. Many companies overlook data challenges unique to AI, such as bias and inconsistency. To succeed, organizations must ensure that AI-ready data is accurate, representative, and free of bias. Addressing these factors establishes a strong foundation, enabling reliable, trustworthy AI models that perform predictably across use cases.
Disruption of Traditional Data Management
AI’s rapid evolution disrupts conventional data management practices, pushing for dynamic, innovative solutions. Advanced strategies like data fabrics and augmented data management are becoming critical for optimizing AI-ready data. Techniques like knowledge graphs enhance data context, integration, and retrieval, making AI models smarter. This shift reflects a growing need for data management innovations that fuel efficient, AI-driven insights.
Bias and Hallucination Mitigation
New solutions tackle AI-specific challenges, such as bias and hallucination. Effective data management structures and prepares AI-ready data to minimize these issues. By implementing strong data governance and quality control, companies can reduce model inaccuracies and biases. This proactive approach fosters more reliable AI models, ensuring that decisions remain unbiased and data-driven.
Integration of Structured and Unstructured Data
Generative AI blurs the line between structured and unstructured data. Managing diverse data formats is crucial for leveraging generative AI’s potential. Organizations need strategies to handle and merge various data types, from text to video. Effective integration enables AI-ready data to support complex AI functionalities, unlocking powerful insights across multiple formats.
5 Steps to AI-Ready Data

The ideal starting point for public-sector agencies to advance in AI is to establish a mission-focused data strategy. By directing resources to feasible, high-impact use cases, agencies can streamline their focus to fewer datasets. This targeted approach allows them to prioritize impact over perfection, accelerating AI efforts.
While identifying these use cases, agencies should verify the availability of essential data sources. Building familiarity with these sources over time fosters expertise. Proper planning can also support bundling related use cases, maximizing resource efficiency by reducing the time needed to implement use cases. Concentrating efforts on mission-driven, high-impact use cases strengthens AI initiatives, with early wins promoting agency-wide support for further AI advancements.
Following these steps can ensure agencies select the right datasets that meet AI-ready data standards.
Step 1: Build a Use Case Specific Data Catalog
The chief data officer, chief information officer, or data domain owner should identify relevant datasets for prioritized use cases. Collaborating with business leaders, they can pinpoint dataset locations, owners, and access protocols. Tailoring data discovery to agency-specific systems and architectures is essential. Successful data catalog projects often include collaboration with system users and technical experts and leverage automated tools for efficient data discovery.
For instance, one federal agency conducted a digital assessment to identify datasets that drive operational efficiency and cost savings. This process enabled them to build a catalog accessible to data practitioners across the agency.
Step 2: Assess Data Quality and Completeness
AI success depends on high-quality, complete data for prioritized use cases. Agencies should thoroughly audit these sources to confirm their AI-ready data status. One national customs agency did this by selecting priority use cases and auditing related datasets. In the initial phases, they required less than 10% of their available data.
Agencies can adapt AI projects to maximize impact with existing data, refining approaches over time. For instance, a state-level agency improved performance by 1.5 to 1.8 times using available data and predictive analytics. These initial successes paved the way for data-sharing agreements, focusing investment on high-impact data sources.
Step 3: Aggregate Prioritized Data Sources
Selected datasets should be consolidated within a data lake, either existing or purpose-built on a new cloud-based platform. This lake serves analytics staff, business teams, clients, and contractors. For example, one civil engineering organization centralized procurement data from 23 resource planning systems onto a single cloud instance, granting relevant stakeholders streamlined access.
Step 4: Evaluate Data Fit
Agencies must evaluate AI-ready data for each use case based on data quantity, quality, and applicability. Fit-for-purpose data varies depending on specific use case requirements. Highly aggregated data, for example, may lack the granularity needed for individual-level insights but may still support community-level predictions.
Analytics teams can enhance fit by:
Selecting data relevant to use cases.
Developing a reusable data model with the necessary fields and tables.
Systematically assessing data quality to identify gaps.
Enriching the data model iteratively, adding parameters or incorporating third-party data.
A state agency, aiming to support care decisions for vulnerable populations, found their initial datasets incomplete and in poor formats. They improved quality through targeted investments, transforming the data for better model outputs.
Step 5: Governance and Execution
Establishing a governance framework is essential to secure AI-ready data and ensure quality, security, and metadata compliance. This framework doesn’t require exhaustive rules but should include data stewardship, quality standards, and access protocols across environments.
In many cases, existing data storage systems can meet basic security requirements. Agencies should assess additional security needs, adopting control standards such as those from the National Institute of Standards and Technology. For instance, one government agency facing complex security needs for over 150 datasets implemented a strategic data security framework. They simplified the architecture with a use case–level security roadmap and are now executing a long-term plan.
For public-sector success, governance and agile methods like DevOps should be core to AI initiatives. Moving away from traditional development models is crucial, as risk-averse cultures and longstanding policies can slow progress. However, this transition is vital to AI-ready data initiatives, enabling real-time improvements and driving impactful outcomes.
Challenges to AI-Ready Data

While AI-ready data promises transformative potential, achieving it poses significant challenges. Organizations must recognize and tackle these obstacles to build a strong, reliable data foundation.
Data Silos
Data silos arise when departments store data separately, creating isolated information pockets. This fragmentation hinders the accessibility, analysis, and usability essential for AI-ready data.
Impact: AI models thrive on a comprehensive data view to identify patterns and make predictions. Silos restrict data scope, resulting in biased models and unreliable outputs.
Solution: Build a centralized data repository, such as a data lake, to aggregate data from diverse sources. Implement cross-functional data integration to dismantle silos, ensuring AI-ready data flows seamlessly across the organization.
Data Inconsistency
Variations in data formats, terms, and values across sources disrupt AI processing, creating confusion and inefficiencies.
Impact: Inconsistent data introduces errors and biases, compromising AI reliability. For example, a model with inconsistent gender markers like “M” and “Male” may yield flawed insights.
Solution: Establish standardized data formats and definitions. Employ data quality checks and validation protocols to catch inconsistencies. Utilize governance frameworks to uphold consistency across the AI-ready data ecosystem.
Data Quality
Poor data quality—like missing values or errors—undermines the accuracy and reliability of AI models.
Impact: Unreliable data leads to skewed predictions and biased models. For instance, missing income data weakens a model predicting purchasing patterns, impacting its effectiveness.
Solution: Use data cleaning and preprocessing to resolve quality issues. Apply imputation techniques for missing values and data enrichment to fill gaps, reinforcing AI-ready data integrity.
Data Privacy and Security
Ensuring data privacy and security is crucial, especially when managing sensitive information under strict regulations.
Impact: Breaches and privacy lapses damage reputations and erodes trust, while legal penalties strain resources. AI-ready data demands rigorous security to safeguard sensitive information.
Solution: Implement encryption, access controls, and data masking to secure AI-ready data. Adopt privacy-enhancing practices, such as differential privacy and federated learning, for safer model training.
You can read about the pillars of AI security.
Skill Shortages
Developing and maintaining an AI-ready data infrastructure requires specialized skills in data science, engineering, and AI.
Impact: Without skilled professionals, organizations struggle to govern data, manage quality, and design robust AI solutions, stalling progress toward AI readiness.
Solution: Invest in hiring and training for data science and engineering roles. Collaborate with external consultants or partner with AI and data management experts to bridge skill gaps.
Why is AI-Ready Data Important?

Accelerated AI Development
AI-ready data minimizes the time data scientists spend on data cleaning and preparation, shifting their focus to building and optimizing models. Traditional data preparation can be tedious and time-consuming, especially when data is unstructured or lacks consistency. With AI-ready data, data is pre-cleaned, labeled, and structured, allowing data scientists to jump straight into analysis. This efficiency translates into a quicker time-to-market, helping organizations keep pace in a rapidly evolving AI landscape where every minute counts.
Improved Model Accuracy
The accuracy of AI models hinges on the quality of the data they consume. AI-ready data is not just clean; it’s relevant, complete, and up-to-date. This enhances model precision, as high-quality data reduces biases and errors. For instance, if a retail company has AI-ready data on customer preferences, its models will generate more accurate recommendations, leading to higher customer satisfaction and loyalty. In essence, AI-ready data helps unlock better predictive accuracy, ensuring that organizations make smarter, data-driven decisions.
Streamlined MLOps for Consistent Performance
Machine Learning Operations (MLOps) ensure that AI models perform consistently from development to deployment. AI-ready data plays a vital role here by ensuring that both historical data (used for training) and real-time data (used in production) are aligned and in sync. This consistency supports smoother transitions between training and deployment phases, reducing model degradation over time. Streamlined MLOps mean fewer interruptions in production environments, helping organizations implement AI faster, and ensuring that models remain robust and reliable in the long term.
Cost Reduction Through Optimized Data Protection

AI projects can be costly, especially when data preparation takes a significant portion of a project’s budget. AI-ready data cuts down the need for extensive manual preparation, enabling engineers to invest time in high-value tasks. This shift not only reduces labor costs but also shortens project timelines, which is particularly advantageous in competitive industries where time-to-market can impact profitability. In essence, the more AI-ready a dataset, the less costly the AI project becomes, allowing for more scalable AI implementations.
Improved Data Governance and Compliance
In a regulatory environment, data governance is paramount, especially as AI decisions become more scrutinized. AI-ready data comes embedded with metadata and lineage information, ensuring that data’s origin, transformations, and usage are documented. This audit trail is crucial when explaining AI-driven decisions to stakeholders, including customers and regulators. Proper governance and transparency are not just compliance necessities—they build trust and enhance accountability, positioning the organization as a responsible AI user.
Future-Proofing for GenAI
With the rapid advancement in generative AI (GenAI), organizations need to prepare now to capitalize on future AI applications. Forward-thinking companies are already developing GenAI-ready data capabilities, setting the groundwork for rapid adoption of new AI technologies.
AI-ready data ensures that, as the AI landscape evolves, the organization’s data is compatible with new AI models, reducing rework and accelerating adoption timelines. This preparation creates a foundation for scalability and adaptability, enabling companies to lead rather than follow in AI evolution.
Reducing Data Preparation Time for Data Scientists
It’s estimated that data scientists spend around 39% of their time preparing data, a staggering amount given their specialized skill sets. By investing in AI-ready data, companies can drastically reduce this figure, allowing data scientists to dedicate more energy to model building and optimization. When data is already clean, organized, and ready to use, data scientists can direct their expertise toward advancing AI’s strategic goals, accelerating innovation, and increasing overall productivity.
Conclusion
In the data-driven landscape, preparing AI-ready data is essential for any organization aiming to leverage artificial intelligence effectively. AI-ready data is not just about volume; it’s about curating data that is accurate, well-structured, secure, and highly relevant to specific business objectives. High-quality data enhances the predictive accuracy of AI models, ensuring reliable insights that inform strategic decisions. By investing in robust data preparation processes, organizations can overcome common AI challenges like biases, errors, and data silos, which often lead to failed AI projects.
Moreover, AI-ready data minimizes the time data scientists spend on tedious data preparation, enabling them to focus on building and refining models that drive innovation. For businesses, this means faster time-to-market, reduced operational costs, and improved adaptability to market changes. Effective data governance and security measures embedded within AI-ready data also foster trust, allowing organizations to meet regulatory standards and protect sensitive information.
As AI technology continues to advance, having a foundation of AI-ready data is crucial for scalability and flexibility. This preparation not only ensures that current AI applications perform optimally but also positions the organization to quickly adopt emerging AI innovations, such as generative AI, without extensive rework. In short, prioritizing AI-ready data today builds resilience and agility, paving the way for sustained growth and a competitive edge in the future.
Source URL: https://www.techaheadcorp.com/blog/what-is-ai-ready-data-how-to-get-your-there/
0 notes
Text
Here’s more data “training” info from the meta ai assistant that I got today:










They’ve been hiding the opt out options on their privacy policy lately 🙄🙄🙄
52 notes
·
View notes
Text

"It looks like you have some private personal and financial details on display! I'll take a screenshot and store it in a folder someone can access later. "
#microsoft#Microsoft Recall#windows#windows recall#clippy#data privacy#online privacy#data security#ai#Bad Clippy! Bad
39 notes
·
View notes
Text
I know I'm on my Sonic AU bullshit, but I'm also really sitting down and thinking about the moral implications of what's going on with AIVOs here...
An sentient AI copy of a proud, stubborn, incredibly intelligent man forced through it's own programming to be more submissive and subservient because it's "only a virtual assistant" is kinda... Fucked up? And I wanna play in with the implications of that ngl, especially given Stone's probably extremely loose sense of personal boundaries after spending the last 7 or so years of his life with Robotnik.
#stobotnik AI AU#AIVOs looking at 7 years of collected data like- '... Huh- a hell of my own making...'#AIVOs isn't attached to Stone (yet) but can see how much his real self was from security footage and journal entries and the like#it has years of data and can track the shifts in its real counterparts behavior and trusts 'himself' enough to try to trust stone#but it really doesn't know what to think of Stone early on#stobotnik
19 notes
·
View notes
Text
Shadows Move In Where Faith Used to Be: Chinese Apps and the American People
There is so much to write about at this moment, it was rather hard to for me to narrow down a topic. Fortunately, in the past couple days, a new story hit the media out of nowhere that made the decision for me. All that being said: have you heard about DeepSeek?
It’s a Chinese generative AI app, based upon the same precepts as ChatGPT. Literally it was built using that software. The claim is that it took ~$6 million to make, compared to the running costs of about half a billion for the other generative AI models. Once this news hit the market, the blue chips crashed and shook the US tech world.
There are plenty of fun side topics that we’ll cover with this one. For the first part, I’ll go into two of my favorite things: schadenfreude and hubris. The past few weeks, schadenfreude has been getting me through the news cycle. I know it’s not a virtue, trust me. But I am only human, and with the depressing news we have lately, it’s sort of been a good feeling to see the people who made assumptions get wet-fish slapped with reality. You’ll notice, with the flurry of Executive Orders the American President has made lately, he didn’t manage to leave the Ukraine war in the first 24 hours as promised, nor has he done anything to lower the price of groceries, eggs, or gas. And, in fact, he has said he won’t (although he says he will lower drill restrictions in protected areas, that won’t drive gas costs down; though, if there was more competition from green initiatives that he’s cutting, it would). I just bring those specific topics up because the people who voted for him claimed it was due to those topics, and they are already dead on arrival. As the rest of us knew they would be. So seeing the inevitable happen, has been the spark of joy I’ve been taking from an overwhelmingly dark time.
The other bit, hubris, is aimed directly at the tech bro overlord douche cadre. I’ve always thought to be a billionaire is a sign of a personal failing; it means you didn’t take care of those around you on the way up. And if you continue to be a billionaire, it means that you still aren’t taking care of people like you should. They could use the dearth of wealth to solve, or nearly solve, all issues we have. But they don’t. And now that we are in this new administration, they immediately bowed down, got in line, and showed us their true natures. Happy for years to stand quietly behind the scenes pulling strings, they have completely capitulated to our new system and tossed out equality or any other moral standing, in the hopes of favor and succor from above. And man, that really made me angry. They don’t even pretend to want to help society now. Google announced yesterday it’s changing its maps to show the Gulf of Mexico inaccurately renamed to Gulf of America, Facebook is going to stop trying to limit hate speech and disinformation at all, and Elon….well, look at that goose stepper up there. He’s just missing the mustache.
So when China announced DeepSeek, I immediately had two responses: wariness because I know why China is doing this, but then there was schadenfreude at the tech sector freaking out. These guys thought they were untouchable, they put themselves on pantheons and thought they finally made it. They could show us their matching Lex Luthor tattoos finally! They own everything and there’s nothing we can do about it!
And then…BAM. China, playing its long game of stealing and silence, tossed this embarrassing grenade right in their sausage fest. MUAHAHAHAHA! Their stocks tanked, billions were lost. The initiative they just announced last week for “AI dominance” suddenly looked, in the daylight, as though it had gotten dressed in a dark room and didn’t check the mirror on the way out. Wait, you all need half a trillion? They did it with 6 million! They kicked the stool out from under the American tech bros, and man was it nice to see them fall.
I also find it vindicating that there are huge moral quandaries with generative AI that haven’t been addressed at all by the government or the tech oligarchy: how they scrape the data of humans to create their content, but don’t compensate them for it. How their output is displacing the careers of real humans, without compensating the system it’s stealing from. How it takes a huge amount of power to run the systems. All of these issues may hit those dudes differently, now that China is wearing the shoes they’ve been sporting.
However…now that we’ve gotten past the flash of “weeeeee”, I’ll move on to my larger emotional response. If you’re American, this isn’t something to truly celebrate. It will be another vector for China to steal our ideas, code, and initiative; after all, that’s how they were able to make DeepSeek in the first place. There’s even evidence that they are using black market NVIDIA chips to make it, since they are restricted from buying them. This comes shortly after the revelations about the two 6th generation fighter jets they purport to have, which if the claims are true, means they have beat us to market. Again, this is based off of data that they stole from us (I remember they hacked the F-35 program while I worked there, and stole plans. And it certainly wasn’t the only time). They excel at taking other people’s ideas and building on them, and we are seeing those chickens come home to roost. For years, they have been thinking strategically about global markets, while we continued to only think tactically.
First we exported our manufacturing to them, hollowing out our middle class at the enrichment of the few. Trickle down economics somehow still hasn’t worked, in the 40 years since we started trying to get it to work. Any minute now, right? So our populace is unhappier and more tired, and broker, than ever before. China has executed extremely high levels of industrial espionage against us, and continues to do so, at a prolific scale. They’ve infiltrated our extremely sensitive government systems time and time again. And that doesn’t even include the actual spies that have infiltrated our universities, to pick our brains about our newest ideas.
So now, we have the results coming to market. 6th gen fighters, DeepSeek, and TikTok. I was going to write about the TikTok ban a few weeks ago, but wanted to see things play out. I’m now extremely happy I did, because it pairs perfectly with current events.
TikTok is a dangerous tool, as the government has been saying (and the American people ignoring), for years. It’s no coincidence that the version China allows its own citizens to use is far different from our own. It’s meant to dull us down and occupy our attention, so that we don’t accomplish anything with drive. It’s a time suck. It pushes disinformation. It ruins our attention span. It also gathers massive amounts of data on us. I personally predict that at some point, China will use that data to make digital AI agents to “befriend” users and then use PSYOPs on them to further degrade their loyalty to America and one another. I believe that they will use the data from DeepSeek to do the same; it gathers an incredible amount of data from the moment you download the app to your phone.
The TikTok ban, which Trump originally proposed (and I supported!) has now been stymied by Trump. He claims Microsoft are in talks to acquire the American arm of the business, and we shall see what comes of it. It’s been turned back on for American users. But what’s been fascinating to me has been watching the response online of the average user.
Overwhelmingly, I have seen Americans who use TikTok blatantly say, “So what if they have my data. American companies already have it all and sell it to anyone who wants it.” And you know what? Solid point.
This is a sign of the breech of trust between American government and its citizens. For many years now, I’ve been a vocal supporter of privacy laws like the EU has, and of harsh penalties for companies that fail to prevent security breaches. Neither has gained any traction in the US. Since the government believes its job is no longer to protect the average citizen, but instead to provide the opportunity to any sap who wants to bilk a buck from his neighbor the protection to do so, Americans are hugely data compromised.
Many times a year I am notified that some site or another has been breached. Companies are very poor custodians of customer data nearly across the board, and there is nearly no restriction on the data they can collect. There was just a huge data breach of an app schools use called “Powerschool”, announced last week. That data, among other things, included Social Security numbers of students and teachers, affected up to 70 million users, and was all achieved by one admin’s compromised credentials being used to login. As someone with software development and security experience, there are so many red flags here. Why was ONE lowly admin able to access the social security numbers of that many people? Why was no one alerted at that massive of a pull? Why were they stored in unencrypted areas, instead of being accessed individually with a key? And, why will nothing be done about it? This stuff is not rocket science; it’s lazy engineering because they companies don’t have the incentive.
If we made it so that there were fines and jail time for stolen data, security would improve immediately. But they get away with it. If someone came in my house, and stole my social security card, they would face fines and jail time. Why should the digital data be any different? If a bank lost my money due to their poor security, I would be protected. Why is this different?
In my option, the American government has done a terrible job of protecting us from this. Part of that is they don’t feel the pressure; part of it is laziness; part of it is the fact that most of Congress has no clue how technology works because they are dinosaurs and lawyers. But I also think the tech sector has done a terrible job of being good citizens as well. And now, guess what’s happened: They’ve done such a bad job, that people think China has their interests at heart more than America. And for me, it’s hard to say they seem totally wrong. China certainly doesn’t have their interests at heart, not at all. But it’s getting harder to be convinced that our own country’s government cares about them, either. And that is so dangerous.
In this moment, the users have the power, and they don’t realize it. We need to have people who understand current issues step up to the plate to protect us. We need to be on the same team again, and by that I mean ALL American citizens need to be protected, not just the rich. In this moment of chaos and terror, I’m not sure what will happen. But at some point, Trump used to be convinced that TikTok and China were dangerous. And he need to remember that they still are. We also need to stand with our true allies again, and make data and security standards with them. If we don’t stand with them, someone else will, and nature abhors a vacuum. I don’t like this isolationist stance we are moving toward; the world is too interconnected for it to be feasible. I have hope that this will be realized again at some point.
Please don’t download those apps. Please don’t give your data and your attention to bad actors. And while we’re at it, contact your Congress people and let them know your concerns about data, and about the overreach of our tech sector. And if you care about any of these topics, please consider helping do something to turn this around. Run for office, use your voice, speak up. We need people who care about change to make it; it’s happened before and the time is perfect for it to happen again.
As always, thanks for reading. Please add me to your RSS feed and share, and contact me if you like. I’d love to hear from you. Times are scary but not impossible. We are in it together. And, I hope you enjoy seeing the tech bro coalition with their bloody teeth this week (I sure am). Like Mike Tyson says, ”Everyone has a plan till they get punched in the mouth.” Maybe they’ll realize they need to take care of Americans if they want Americans to take care of them. Or maybe we need to make them realize it.
6 notes
·
View notes
Text
Time for a new edition of my ongoing vendetta against Google fuckery!
Hey friends, did you know that Google is now using Google docs to train it's AI, whether you like it or not? (link goes to: zdnet.com, July 5, 2023). Oh and on Monday, Google updated it's privacy policy to say that it can train it's two AI (Bard and Cloud AI) on any data it scrapes from it's users, period. (link goes to: The Verge, 5 July 2023). Here is Digital Trends also mentioning this new policy change (link goes to: Digital Trends, 5 July 2023). There are a lot more, these are just the most succinct articles that might explain what's happening.
FURTHER REASONS GOOGLE AND GOOGLE CHROME SUCK TODAY:
Stop using Google Analytics, warns Sweden’s privacy watchdog, as it issues over $1M in fines (link goes to: TechCrunch, 3 July 2023) [TLDR: google got caught exporting european users' data to the US to be 'processed' by 'US government surveillance,' which is HELLA ILLEGAL. I'm not going into the Five Eyes, Fourteen Eyes, etc agreements, but you should read up on those to understand why the 'US government surveillance' people might ask Google to do this for countries that are not apart of the various Eyes agreements - and before anyone jumps in with "the US sucks!" YES but they are 100% not the only government buying foreign citizens' data, this is just the one the Swedes caught. Today.]
PwC Australia ties Google to tax leak scandal (link goes to: Reuters, 5 July 2023). [TLDR: a Russian accounting firm slipped Google "confidential information about the start date of a new tax law leaked from Australian government tax briefings." Gosh, why would Google want to spy on governments about tax laws? Can't think of any reason they would want to be able to clean house/change policy/update their user agreement to get around new restrictions before those restrictions or fines hit. Can you?
SO - here is a very detailed list of browsers, updated on 28 June, 2023 on slant.com, that are NOT based on Google Chrome (note: any browser that says 'Chromium-based' is just Google wearing a party mask. It means that Google AND that other party has access to all your data). This is an excellent list that shows pros and cons for each browser, including who the creator is and what kinds of policies they have (for example, one con for Pale Moon is that the creator doesn't like and thinks all websites should be hostile to Tor).
#you need to protect yourself#anti google#anti chrome#anti chromium#chromium based browsers#internet security#current events#i recommend firefox#but if you have beef with it#here are alternatives!#so called ai#anti artificial intelligence#anti chatgpt#anti bard#anti cloud ai#data scraping
101 notes
·
View notes
Text
Google Launches AI Anti-Theft Feature for Android Phones in Brazil

Alphabet’s Google will launch a new AI-powered anti-theft feature for Android phones in Brazil, the first country to test this technology, aiming to reduce phone theft rates in a country where nearly one million phones were stolen last year.
Google, the tech giant under Alphabet Inc., made a groundbreaking announcement. Brazil, a country plagued by high phone theft rates, will be the first to test a new anti-theft feature for Android phones using artificial intelligence (AI). This innovative technology, a significant step in the fight against phone theft, automatically locks the device’s screen when a phone has been stolen, thereby enhancing security for users.
The initiative is part of Google’s broader strategy to leverage AI to address real-world problems. Starting in July, the anti-theft feature will initially be available to Brazilian users of Android phones running version 10 or higher. According to the company, this phased rollout will eventually extend to other countries over the year.
The new anti-theft feature, powered by AI, operates through three types of locks. The first lock utilizes AI to identify movements typically associated with theft, such as sudden, rapid movements that suggest snatching or running. Once these movements are detected, the feature automatically locks the screen, rendering the phone unusable to the thief. The AI model has been trained to recognize specific patterns that indicate a theft, enabling it to provide an immediate response to protect the user’s data.
Continue reading.
#brazil#brazilian politics#politics#artificial intelligence#public security#technology#google#image description in alt#mod nise da silveira#man before reading the report i was thinking google was rolling out something to protect users from ai data theft#and was positively surprised by that concept#only to find out it's just regular old school physical theft lol
9 notes
·
View notes
Text
Elon Musk’s Ally Pushes for ‘AI-First’ Strategy in Government Agency
Elon Musk’s Ally Pushes for ‘AI-First’ Strategy in Government Agency In a groundbreaking statement, a close ally of Elon Musk has revealed that embracing an “AI-first” approach is the future for a key government agency. This bold vision outlines how artificial intelligence (AI) will shape the operations and policies of government entities, especially those tied to technology and national…
#AI adoption#AI collaboration#AI in defense#AI in government#AI strategy#AI technology#AI-driven government#AI-first#artificial intelligence#cybersecurity#data analysis#Elon Musk#future of AI#government agency#government reform#Musk&039;s vision#national security#public sector#public services#tech industry#technology innovation
2 notes
·
View notes
Video
youtube
DeepSeek App - Privacy concerns
#youtube#DeepSeek AI chatbot privacy data security technology futuristic digital encryption mystery Concern
3 notes
·
View notes
Text
Our six priorities for digital rights
Open Rights Group is calling on the next government to focus on the following six priorities for digital rights:
1. Protect our right to send secure messages
Everyone – including children and young people – should have the right to use end-to-end encryption to ensure that our communications are safe, secure and private. The next government should protect not undermine encryption.
2. Provide migrants with digital sanctuary
Migrants, refugees and asylum seekers need the same data and privacy rights as everyone else so that they can keep their digital identity and information safe. The next government should commit to ending the digital hostile environment.
3. Ban the use of pre-crime AI by the police
Predictive policing systems that use artificial intelligence (AI) to ‘predict’ criminal behaviour undermine our right to be presumed innocent and exacerbate discrimination and inequality in our criminal justice system. The next government should ban dangerous uses of AI in policing.
4. Defend our right to freedom of expression online
Freedom of expression online is being undermined by age verification, content takedowns, social media censorship and unfair copyright claims. The next government should commit to protecting our right to freedom of expression online.
5. Strengthen our data protection rights
We need strong data protection laws to make sure that governments and companies do not use our data to track, surveil and profit from us. The next government should strengthen our data protection rights and make sure that the data protection watchdog is fit for purpose.
6. End intrusive tracking by online advertisers
Advertising companies track our internet use to build detailed profiles so they can target us with adverts. The next government should restrict intrusive tracking by data brokers and online advertisers.
#open rights group#censorship#privacy#data security#human rights#free speech#free association#ai#artificial intelligence#2024
5 notes
·
View notes
Text
#Tags:Advanced AI Systems#Apple Vision Pro#Biometric Authentication#Biometric Innovations#Civil Liberties and Technology#Consumer Technology#Corporate Control#Data Security Risks#facts#Iris Recognition Technology#life#New World Order#Optic ID#Podcast#Privacy Concerns#serious#straight forward#Surveillance Technology#truth#upfront#Post navigation#Previous
2 notes
·
View notes
Text
Been thinking about this for a while, and after a lot of reading, consideration, discussions and more thinking, I finally put some of my thoughts about AI in writing. (Spoiler, I think it's bad and dangerous and unethical). This is long winded. Not sorry.
2 notes
·
View notes
Text
#ai#Bias and Discrimination#Cost and Resource Intensive#Data Privacy and Security Concerns#Lack of Transparency
2 notes
·
View notes
Text
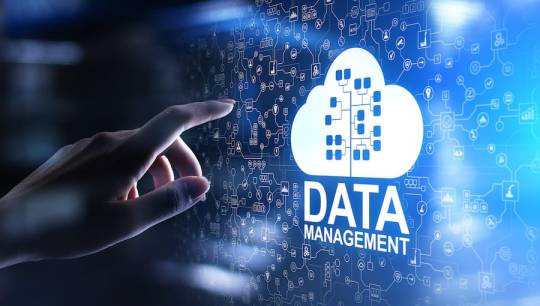
The Comprehensive Guide to Web Development, Data Management, and More
Introduction
Everything today is technology driven in this digital world. There's a lot happening behind the scenes when you use your favorite apps, go to websites, and do other things with all of those zeroes and ones — or binary data. In this blog, I will be explaining what all these terminologies really means and other basics of web development, data management etc. We will be discussing them in the simplest way so that this becomes easy to understand for beginners or people who are even remotely interested about technology. JOIN US
What is Web Development?
Web development refers to the work and process of developing a website or web application that can run in a web browser. From laying out individual web page designs before we ever start coding, to how the layout will be implemented through HTML/CSS. There are two major fields of web development — front-end and back-end.
Front-End Development
Front-end development, also known as client-side development, is the part of web development that deals with what users see and interact with on their screens. It involves using languages like HTML, CSS, and JavaScript to create the visual elements of a website, such as buttons, forms, and images. JOIN US
HTML (HyperText Markup Language):
HTML is the foundation of all website, it helps one to organize their content on web platform. It provides the default style to basic elements such as headings, paragraphs and links.
CSS (Cascading Style Sheets):
styles and formats HTML elements. It makes an attractive and user-friendly look of webpage as it controls the colors, fonts, layout.
JavaScript :
A language for adding interactivity to a website Users interact with items, like clicking a button to send in a form or viewing images within the slideshow. JOIN US
Back-End Development
The difference while front-end development is all about what the user sees, back end involves everything that happens behind. The back-end consists of a server, database and application logic that runs on the web.
Server:
A server is a computer that holds website files and provides them to the user browser when they request it. Server-Side: These are populated by back-end developers who build and maintain servers using languages like Python, PHP or Ruby.
Database:
The place where a website keeps its data, from user details to content and settings The database is maintained with services like MySQL, PostgreSQL, or MongoDB. JOIN US
Application Logic —
the code that links front-end and back-end It takes user input, gets data from the database and returns right informations to front-end area.
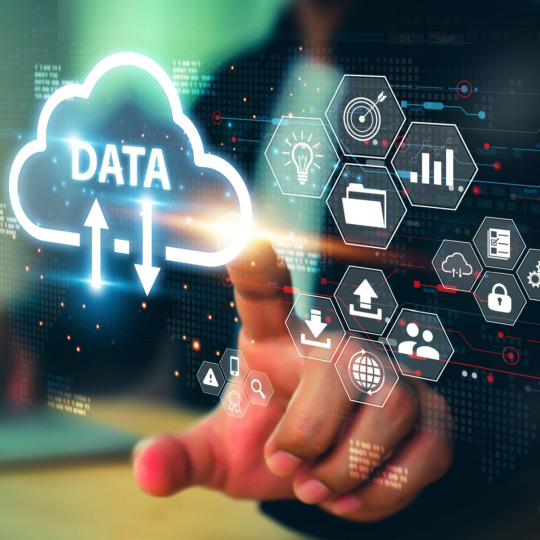
Why Proper Data Management is Absolutely Critical
Data management — Besides web development this is the most important a part of our Digital World. What Is Data Management? It includes practices, policies and procedures that are used to collect store secure data in controlled way.
Data Storage –
data after being collected needs to be stored securely such data can be stored in relational databases or cloud storage solutions. The most important aspect here is that the data should never be accessed by an unauthorized source or breached. JOIN US
Data processing:
Right from storing the data, with Big Data you further move on to process it in order to make sense out of hordes of raw information. This includes cleansing the data (removing errors or redundancies), finding patterns among it, and producing ideas that could be useful for decision-making.
Data Security:
Another important part of data management is the security of it. It refers to defending data against unauthorized access, breaches or other potential vulnerabilities. You can do this with some basic security methods, mostly encryption and access controls as well as regular auditing of your systems.
Other Critical Tech Landmarks
There are a lot of disciplines in the tech world that go beyond web development and data management. Here are a few of them:
Cloud Computing
Leading by example, AWS had established cloud computing as the on-demand delivery of IT resources and applications via web services/Internet over a decade considering all layers to make it easy from servers up to top most layer. This will enable organizations to consume technology resources in the form of pay-as-you-go model without having to purchase, own and feed that infrastructure. JOIN US
Cloud Computing Advantages:
Main advantages are cost savings, scalability, flexibility and disaster recovery. Resources can be scaled based on usage, which means companies only pay for what they are using and have the data backed up in case of an emergency.
Examples of Cloud Services:
Few popular cloud services are Amazon Web Services (AWS), Microsoft Azure, and Google Cloud. These provide a plethora of services that helps to Develop and Manage App, Store Data etc.
Cybersecurity
As the world continues to rely more heavily on digital technologies, cybersecurity has never been a bigger issue. Protecting computer systems, networks and data from cyber attacks is called Cyber security.
Phishing attacks, Malware, Ransomware and Data breaches:
This is common cybersecurity threats. These threats can bear substantial ramifications, from financial damages to reputation harm for any corporation.
Cybersecurity Best Practices:
In order to safeguard against cybersecurity threats, it is necessary to follow best-practices including using strong passwords and two-factor authorization, updating software as required, training employees on security risks.
Artificial Intelligence and Machine Learning
Artificial Intelligence (AI) and Machine Learning (ML) represent the fastest-growing fields of creating systems that learn from data, identifying patterns in them. These are applied to several use-cases like self driving cars, personalization in Netflix.
AI vs ML —
AI is the broader concept of machines being able to carry out tasks in a way we would consider “smart”. Machine learning is a type of Artificial Intelligence (AI) that provides computers with the ability to learn without being explicitly programmed. JOIN US
Applications of Artificial Intelligence and Machine Learning: some common applications include Image recognition, Speech to text, Natural language processing, Predictive analytics Robotics.
Web Development meets Data Management etc.
We need so many things like web development, data management and cloud computing plus cybersecurity etc.. but some of them are most important aspects i.e. AI/ML yet more fascinating is where these fields converge or play off each other.
Web Development and Data Management
Web Development and Data Management goes hand in hand. The large number of websites and web-based applications in the world generate enormous amounts of data — from user interactions, to transaction records. Being able to manage this data is key in providing a fantastic user experience and enabling you to make decisions based on the right kind of information.
E.g. E-commerce Website, products data need to be saved on server also customers data should save in a database loosely coupled with orders and payments. This data is necessary for customization of the shopping experience as well as inventory management and fraud prevention.
Cloud Computing and Web Development
The development of the web has been revolutionized by cloud computing which gives developers a way to allocate, deploy and scale applications more or less without service friction. Developers now can host applications and data in cloud services instead of investing for physical servers.
E.g. A start-up company can use cloud services to roll out the web application globally in order for all users worldwide could browse it without waiting due unavailability of geolocation prohibited access.
The Future of Cybersecurity and Data Management
Which makes Cybersecurity a very important part of the Data management. The more data collected and stored by an organization, the greater a target it becomes for cyber threats. It is important to secure this data using robust cybersecurity measures, so that sensitive information remains intact and customer trust does not weaken. JOIN US
Ex: A healthcare provider would have to protect patient data in order to be compliant with regulations such as HIPAA (Health Insurance Portability and Accountability Act) that is also responsible for ensuring a degree of confidentiality between a provider and their patients.
Conclusion
Well, in a nutshell web-developer or Data manager etc are some of the integral parts for digital world.
As a Business Owner, Tech Enthusiast or even if you are just planning to make your Career in tech — it is important that you understand these. With the progress of technology never slowing down, these intersections are perhaps only going to come together more strongly and develop into cornerstones that define how we live in a digital world tomorrow.
With the fundamental knowledge of web development, data management, automation and ML you will manage to catch up with digital movements. Whether you have a site to build, ideas data to manage or simply interested in what’s hot these days, skills and knowledge around the above will stand good for changing tech world. JOIN US
#Technology#Web Development#Front-End Development#Back-End Development#HTML#CSS#JavaScript#Data Management#Data Security#Cloud Computing#AWS (Amazon Web Services)#Cybersecurity#Artificial Intelligence (AI)#Machine Learning (ML)#Digital World#Tech Trends#IT Basics#Beginners Guide#Web Development Basics#Tech Enthusiast#Tech Career#america
4 notes
·
View notes
Text
EU passes artificial intelligence act

View On WordPress
#ai#artificial intelligence#computer#data#facial recognition#information#machine learning#meme#memes#safety#security
3 notes
·
View notes