#AI and machine learning algorithm
Explore tagged Tumblr posts
Text
An In-Depth Exploration of AI in Cloud Computing | USAII®
Understand AI in cloud computing with AI models, cloud computing nuances, and diverse facets of AI ML engineers. Enrol with the best AI certification programs today!
Read more: https://shorturl.at/JoiJI
0 notes
Text
The surprising truth about data-driven dictatorships
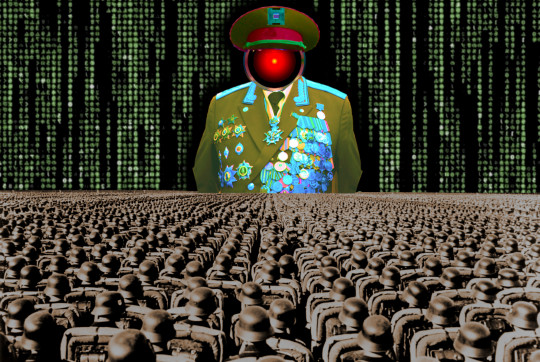
Here’s the “dictator’s dilemma”: they want to block their country’s frustrated elites from mobilizing against them, so they censor public communications; but they also want to know what their people truly believe, so they can head off simmering resentments before they boil over into regime-toppling revolutions.
These two strategies are in tension: the more you censor, the less you know about the true feelings of your citizens and the easier it will be to miss serious problems until they spill over into the streets (think: the fall of the Berlin Wall or Tunisia before the Arab Spring). Dictators try to square this circle with things like private opinion polling or petition systems, but these capture a small slice of the potentially destabiziling moods circulating in the body politic.
Enter AI: back in 2018, Yuval Harari proposed that AI would supercharge dictatorships by mining and summarizing the public mood — as captured on social media — allowing dictators to tack into serious discontent and diffuse it before it erupted into unequenchable wildfire:
https://www.theatlantic.com/magazine/archive/2018/10/yuval-noah-harari-technology-tyranny/568330/
Harari wrote that “the desire to concentrate all information and power in one place may become [dictators] decisive advantage in the 21st century.” But other political scientists sharply disagreed. Last year, Henry Farrell, Jeremy Wallace and Abraham Newman published a thoroughgoing rebuttal to Harari in Foreign Affairs:
https://www.foreignaffairs.com/world/spirals-delusion-artificial-intelligence-decision-making
They argued that — like everyone who gets excited about AI, only to have their hopes dashed — dictators seeking to use AI to understand the public mood would run into serious training data bias problems. After all, people living under dictatorships know that spouting off about their discontent and desire for change is a risky business, so they will self-censor on social media. That’s true even if a person isn’t afraid of retaliation: if you know that using certain words or phrases in a post will get it autoblocked by a censorbot, what’s the point of trying to use those words?
The phrase “Garbage In, Garbage Out” dates back to 1957. That’s how long we’ve known that a computer that operates on bad data will barf up bad conclusions. But this is a very inconvenient truth for AI weirdos: having given up on manually assembling training data based on careful human judgment with multiple review steps, the AI industry “pivoted” to mass ingestion of scraped data from the whole internet.
But adding more unreliable data to an unreliable dataset doesn’t improve its reliability. GIGO is the iron law of computing, and you can’t repeal it by shoveling more garbage into the top of the training funnel:
https://memex.craphound.com/2018/05/29/garbage-in-garbage-out-machine-learning-has-not-repealed-the-iron-law-of-computer-science/
When it comes to “AI” that’s used for decision support — that is, when an algorithm tells humans what to do and they do it — then you get something worse than Garbage In, Garbage Out — you get Garbage In, Garbage Out, Garbage Back In Again. That’s when the AI spits out something wrong, and then another AI sucks up that wrong conclusion and uses it to generate more conclusions.
To see this in action, consider the deeply flawed predictive policing systems that cities around the world rely on. These systems suck up crime data from the cops, then predict where crime is going to be, and send cops to those “hotspots” to do things like throw Black kids up against a wall and make them turn out their pockets, or pull over drivers and search their cars after pretending to have smelled cannabis.
The problem here is that “crime the police detected” isn’t the same as “crime.” You only find crime where you look for it. For example, there are far more incidents of domestic abuse reported in apartment buildings than in fully detached homes. That’s not because apartment dwellers are more likely to be wife-beaters: it’s because domestic abuse is most often reported by a neighbor who hears it through the walls.
So if your cops practice racially biased policing (I know, this is hard to imagine, but stay with me /s), then the crime they detect will already be a function of bias. If you only ever throw Black kids up against a wall and turn out their pockets, then every knife and dime-bag you find in someone’s pockets will come from some Black kid the cops decided to harass.
That’s life without AI. But now let’s throw in predictive policing: feed your “knives found in pockets” data to an algorithm and ask it to predict where there are more knives in pockets, and it will send you back to that Black neighborhood and tell you do throw even more Black kids up against a wall and search their pockets. The more you do this, the more knives you’ll find, and the more you’ll go back and do it again.
This is what Patrick Ball from the Human Rights Data Analysis Group calls “empiricism washing”: take a biased procedure and feed it to an algorithm, and then you get to go and do more biased procedures, and whenever anyone accuses you of bias, you can insist that you’re just following an empirical conclusion of a neutral algorithm, because “math can’t be racist.”
HRDAG has done excellent work on this, finding a natural experiment that makes the problem of GIGOGBI crystal clear. The National Survey On Drug Use and Health produces the gold standard snapshot of drug use in America. Kristian Lum and William Isaac took Oakland’s drug arrest data from 2010 and asked Predpol, a leading predictive policing product, to predict where Oakland’s 2011 drug use would take place.
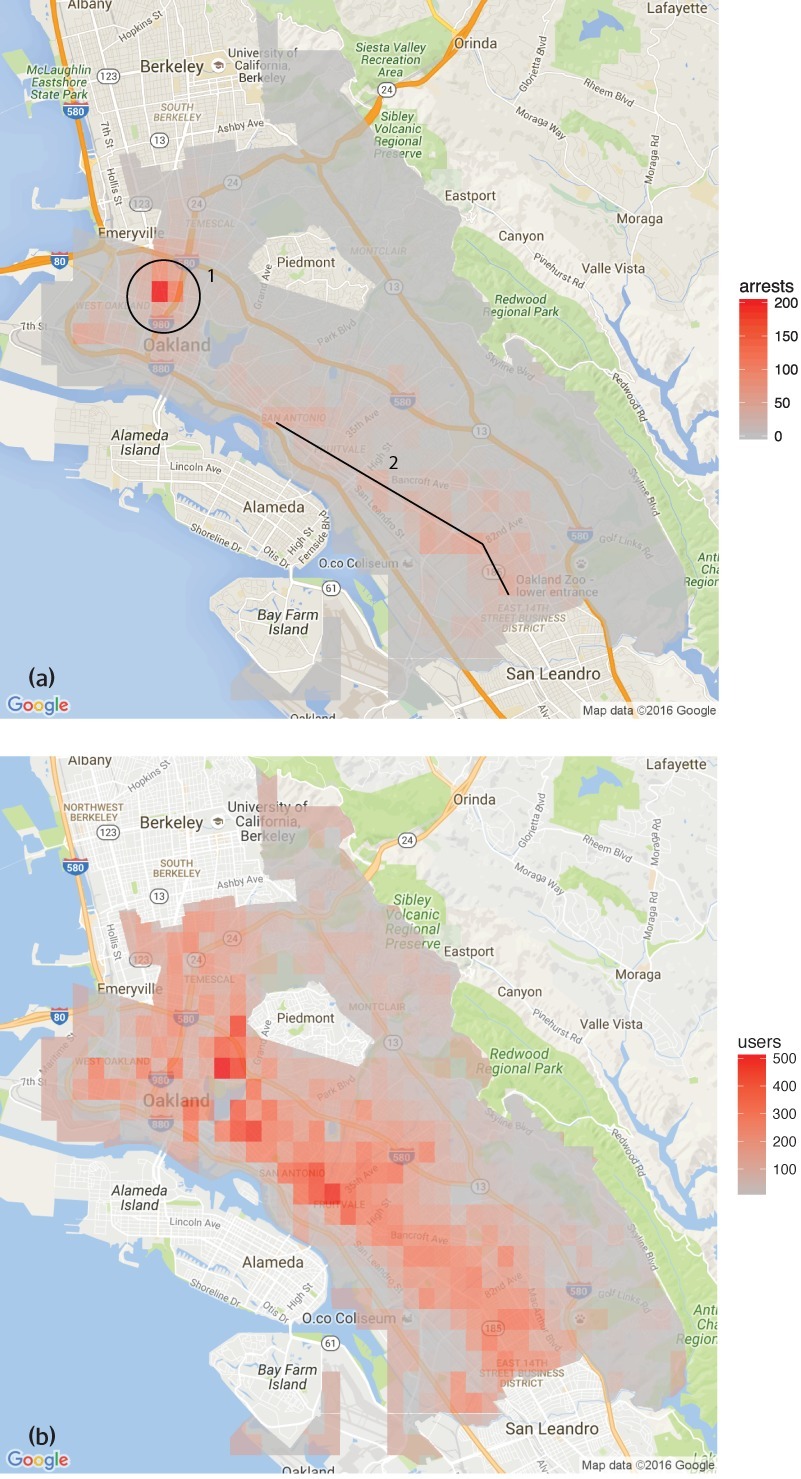
[Image ID: (a) Number of drug arrests made by Oakland police department, 2010. (1) West Oakland, (2) International Boulevard. (b) Estimated number of drug users, based on 2011 National Survey on Drug Use and Health]
Then, they compared those predictions to the outcomes of the 2011 survey, which shows where actual drug use took place. The two maps couldn’t be more different:
https://rss.onlinelibrary.wiley.com/doi/full/10.1111/j.1740-9713.2016.00960.x
Predpol told cops to go and look for drug use in a predominantly Black, working class neighborhood. Meanwhile the NSDUH survey showed the actual drug use took place all over Oakland, with a higher concentration in the Berkeley-neighboring student neighborhood.
What’s even more vivid is what happens when you simulate running Predpol on the new arrest data that would be generated by cops following its recommendations. If the cops went to that Black neighborhood and found more drugs there and told Predpol about it, the recommendation gets stronger and more confident.
In other words, GIGOGBI is a system for concentrating bias. Even trace amounts of bias in the original training data get refined and magnified when they are output though a decision support system that directs humans to go an act on that output. Algorithms are to bias what centrifuges are to radioactive ore: a way to turn minute amounts of bias into pluripotent, indestructible toxic waste.
There’s a great name for an AI that’s trained on an AI’s output, courtesy of Jathan Sadowski: “Habsburg AI.”
And that brings me back to the Dictator’s Dilemma. If your citizens are self-censoring in order to avoid retaliation or algorithmic shadowbanning, then the AI you train on their posts in order to find out what they’re really thinking will steer you in the opposite direction, so you make bad policies that make people angrier and destabilize things more.
Or at least, that was Farrell(et al)’s theory. And for many years, that’s where the debate over AI and dictatorship has stalled: theory vs theory. But now, there’s some empirical data on this, thanks to the “The Digital Dictator’s Dilemma,” a new paper from UCSD PhD candidate Eddie Yang:
https://www.eddieyang.net/research/DDD.pdf
Yang figured out a way to test these dueling hypotheses. He got 10 million Chinese social media posts from the start of the pandemic, before companies like Weibo were required to censor certain pandemic-related posts as politically sensitive. Yang treats these posts as a robust snapshot of public opinion: because there was no censorship of pandemic-related chatter, Chinese users were free to post anything they wanted without having to self-censor for fear of retaliation or deletion.
Next, Yang acquired the censorship model used by a real Chinese social media company to decide which posts should be blocked. Using this, he was able to determine which of the posts in the original set would be censored today in China.
That means that Yang knows that the “real” sentiment in the Chinese social media snapshot is, and what Chinese authorities would believe it to be if Chinese users were self-censoring all the posts that would be flagged by censorware today.
From here, Yang was able to play with the knobs, and determine how “preference-falsification” (when users lie about their feelings) and self-censorship would give a dictatorship a misleading view of public sentiment. What he finds is that the more repressive a regime is — the more people are incentivized to falsify or censor their views — the worse the system gets at uncovering the true public mood.
What’s more, adding additional (bad) data to the system doesn’t fix this “missing data” problem. GIGO remains an iron law of computing in this context, too.
But it gets better (or worse, I guess): Yang models a “crisis” scenario in which users stop self-censoring and start articulating their true views (because they’ve run out of fucks to give). This is the most dangerous moment for a dictator, and depending on the dictatorship handles it, they either get another decade or rule, or they wake up with guillotines on their lawns.
But “crisis” is where AI performs the worst. Trained on the “status quo” data where users are continuously self-censoring and preference-falsifying, AI has no clue how to handle the unvarnished truth. Both its recommendations about what to censor and its summaries of public sentiment are the least accurate when crisis erupts.
But here’s an interesting wrinkle: Yang scraped a bunch of Chinese users’ posts from Twitter — which the Chinese government doesn’t get to censor (yet) or spy on (yet) — and fed them to the model. He hypothesized that when Chinese users post to American social media, they don’t self-censor or preference-falsify, so this data should help the model improve its accuracy.
He was right — the model got significantly better once it ingested data from Twitter than when it was working solely from Weibo posts. And Yang notes that dictatorships all over the world are widely understood to be scraping western/northern social media.
But even though Twitter data improved the model’s accuracy, it was still wildly inaccurate, compared to the same model trained on a full set of un-self-censored, un-falsified data. GIGO is not an option, it’s the law (of computing).
Writing about the study on Crooked Timber, Farrell notes that as the world fills up with “garbage and noise” (he invokes Philip K Dick’s delighted coinage “gubbish”), “approximately correct knowledge becomes the scarce and valuable resource.”
https://crookedtimber.org/2023/07/25/51610/
This “probably approximately correct knowledge” comes from humans, not LLMs or AI, and so “the social applications of machine learning in non-authoritarian societies are just as parasitic on these forms of human knowledge production as authoritarian governments.”
The Clarion Science Fiction and Fantasy Writers’ Workshop summer fundraiser is almost over! I am an alum, instructor and volunteer board member for this nonprofit workshop whose alums include Octavia Butler, Kim Stanley Robinson, Bruce Sterling, Nalo Hopkinson, Kameron Hurley, Nnedi Okorafor, Lucius Shepard, and Ted Chiang! Your donations will help us subsidize tuition for students, making Clarion — and sf/f — more accessible for all kinds of writers.
Libro.fm is the indie-bookstore-friendly, DRM-free audiobook alternative to Audible, the Amazon-owned monopolist that locks every book you buy to Amazon forever. When you buy a book on Libro, they share some of the purchase price with a local indie bookstore of your choosing (Libro is the best partner I have in selling my own DRM-free audiobooks!). As of today, Libro is even better, because it’s available in five new territories and currencies: Canada, the UK, the EU, Australia and New Zealand!
[Image ID: An altered image of the Nuremberg rally, with ranked lines of soldiers facing a towering figure in a many-ribboned soldier's coat. He wears a high-peaked cap with a microchip in place of insignia. His head has been replaced with the menacing red eye of HAL9000 from Stanley Kubrick's '2001: A Space Odyssey.' The sky behind him is filled with a 'code waterfall' from 'The Matrix.']
Image: Cryteria (modified) https://commons.wikimedia.org/wiki/File:HAL9000.svg
CC BY 3.0 https://creativecommons.org/licenses/by/3.0/deed.en
—
Raimond Spekking (modified) https://commons.wikimedia.org/wiki/File:Acer_Extensa_5220_-_Columbia_MB_06236-1N_-_Intel_Celeron_M_530_-_SLA2G_-_in_Socket_479-5029.jpg
CC BY-SA 4.0 https://creativecommons.org/licenses/by-sa/4.0/deed.en
—
Russian Airborne Troops (modified) https://commons.wikimedia.org/wiki/File:Vladislav_Achalov_at_the_Airborne_Troops_Day_in_Moscow_%E2%80%93_August_2,_2008.jpg
“Soldiers of Russia” Cultural Center (modified) https://commons.wikimedia.org/wiki/File:Col._Leonid_Khabarov_in_an_everyday_service_uniform.JPG
CC BY-SA 3.0 https://creativecommons.org/licenses/by-sa/3.0/deed.en
#pluralistic#habsburg ai#self censorship#henry farrell#digital dictatorships#machine learning#dictator's dilemma#eddie yang#preference falsification#political science#training bias#scholarship#spirals of delusion#algorithmic bias#ml#Fully automated data driven authoritarianism#authoritarianism#gigo#garbage in garbage out garbage back in#gigogbi#yuval noah harari#gubbish#pkd#philip k dick#phildickian
831 notes
·
View notes
Text
Neturbiz Enterprises - AI Innov7ions
Our mission is to provide details about AI-powered platforms across different technologies, each of which offer unique set of features. The AI industry encompasses a broad range of technologies designed to simulate human intelligence. These include machine learning, natural language processing, robotics, computer vision, and more. Companies and research institutions are continuously advancing AI capabilities, from creating sophisticated algorithms to developing powerful hardware. The AI industry, characterized by the development and deployment of artificial intelligence technologies, has a profound impact on our daily lives, reshaping various aspects of how we live, work, and interact.
#ai technology#Technology Revolution#Machine Learning#Content Generation#Complex Algorithms#Neural Networks#Human Creativity#Original Content#Healthcare#Finance#Entertainment#Medical Image Analysis#Drug Discovery#Ethical Concerns#Data Privacy#Artificial Intelligence#GANs#AudioGeneration#Creativity#Problem Solving#ai#autonomous#deepbrain#fliki#krater#podcast#stealthgpt#riverside#restream#murf
17 notes
·
View notes
Text
I desprately need someone to talk to about this
I've been working on a system to allow a genetic algorithm to create DNA code which can create self-organising organisms. Someone I know has created a very effective genetic algorithm which blows NEAT out of the water in my opinion. So, this algorithm is very good at using food values to determine which organisms to breed, how to breed them, and the multitude of different biologically inspired mutation mechanisms which allow for things like meta genes and meta-meta genes, and a whole other slew of things. I am building a translation system, basically a compiler on top of it, and designing an instruction set and genetic repair mechanisms to allow it to convert ANY hexadecimal string into a valid, operable program. I'm doing this by having an organism with, so far, 5 planned chromosomes. The first and second chromosome are the INITIAL STATE of a neural network. The number and configuration of input nodes, the number and configuration of output nodes, whatever code it needs for a fitness function, and the configuration and weights of the layers. This neural network is not used at all in the fitness evaluation of the organism, but purely something the organism itself can manage, train, and utilize how it sees fit.
The third is the complete code of the program which runs the organism. Its basically a list of ASM opcodes and arguments written in hexadecimal. It is comprised of codons which represent the different hexadecimal characters, as well as a start and stop codon. This program will be compiled into executable machine code using LLVM IR and a custom instruction set I've designed for the organisms to give them a turing complete programming language and some helper functions to make certain processes simpler to evolve. This includes messages between the organisms, reproduction methods, and all the methods necessary for the organisms to develop sight, hearing, and recieve various other inputs, and also to output audio, video, and various outputs like mouse, keyboard, or a gamepad output. The fourth is a blank slate, which the organism can evolve whatever data it wants. The first half will be the complete contents of the organisms ROM after the important information, and the second half will be the initial state of the organisms memory. This will likely be stored as base 64 of its hash and unfolded into binary on compilation.
The 5th chromosome is one I just came up with and I am very excited about, it will be a translation dictionary. It will be 512 individual codons exactly, with each codon pair being mapped between 00 and FF hex. When evaulating the hex of the other chromosomes, this dictionary will be used to determine the equivalent instruction of any given hex pair. When evolving, each hex pair in the 5th organism will be guaranteed to be a valid opcode in the instruction set by using modulus to constrain each pair to the 55 instructions currently available. This will allow an organism to evolve its own instruction distribution, and try to prevent random instructions which might be harmful or inneficient from springing up as often, and instead more often select for efficient or safer instructions.
#ai#technology#genetic algorithm#machine learning#programming#python#ideas#discussion#open source#FOSS#linux#linuxposting#musings#word vomit#random thoughts#rant
8 notes
·
View notes
Text
Did anyone else notice the unexpected shift?
In the past year, the MLearning Art community saw breakthroughs that defy expectation.
They challenge how we imagine, create, and share art
#machinelearning#artificialintelligence#art#digitalart#mlart#datascience#algorithm#ai art#machine learning
5 notes
·
View notes
Text
why is everything called AI now. boy thats an algorithm
#'ubers evil AI will detect your phone battery is low and raise the prices accordingly' thats fucked up but it#doesnt need a machine learning program for that#and not algorithm like your social media feed or whatever like in the most basic sense of the definition
8 notes
·
View notes
Text
KNN Algorithm | Learn About Artificial Intelligence
The k-Nearest Neighbors (KNN) algorithm is a simple, versatile, and popular machine learning method used for both classification and regression tasks, making predictions based on the proximity of data points to their nearest neighbors in a dataset.
Detect Triangle shape inside image using Java Open CV //Triangle Transform Computer Vision part one

KNN is a supervised learning algorithm, meaning it learns from labeled data to make predictions on new, unseen data. KNN relies on a distance metric.
Lazy Learning: It's considered a "lazy learner" because it doesn't have a dedicated training phase; instead, it stores the training data and uses it directly for prediction.
Proximity-Based: KNN relies on the principle that similar data points are located near each other, and it makes predictions based on the classes or values of the nearest neighbors.
Classification: In classification, KNN assigns a new data point to the class that is most common among its k nearest neighbors.
Regression: In regression, KNN predicts a value by averaging the values of the k nearest neighbors.
Parameter k: The parameter 'k' determines the number of nearest neighbors to consider when making a prediction.
#machine learning#artificial image#artificial intelligence#knn algorithm#opencv#image processing#ai image#ai image generator#animation#animation practice#animation design#3d printing#3d image producing#3d image process
4 notes
·
View notes
Text
UAITrading (Unstoppable AI Trading): AI-Powered Trading for Stocks, Forex, and Crypto
https://uaitrading.ai/ UAITrading For On trading volumes offers, many free trade analysis tools and pending bonuses | Unstoppable AI Trading (Uaitrading) is a platform that integrates advanced artificial intelligence (AI) technologies to enhance trading strategies across various financial markets, including stocks, forex, and cryptocurrencies. By leveraging AI, the platform aims to provide real-time asset monitoring, automated portfolio management, and optimized trade execution, thereby simplifying the investment process for users.

One of the innovative features of Unstoppable AI Trading is its UAI token farming, which offers users opportunities to earn additional income through decentralized finance (DeFi) mechanisms. This approach allows traders to diversify their investment strategies and potentially increase returns by participating in token farming activities.
The platform's AI-driven systems are designed to analyze vast amounts of market data, identify profitable trading opportunities, and execute trades without human intervention. This automation not only enhances efficiency but also reduces the emotional biases that often affect human traders, leading to more consistent and objective trading decisions.
By harnessing the power of AI, Unstoppable AI Trading aims to empower both novice and experienced traders to navigate the complexities of financial markets more effectively, offering tools and strategies that adapt to dynamic market conditions
#Uaitrading#AI Trading#Automated Trading#Forex Trading AI#Crypto Trading Bot#UAI Token#Token Farming#Decentralized Finance (DeFi)#AI Investment Platform#Smart Trading Algorithms#AI Stock Trading#Machine Learning in Trading#AI-Powered Portfolio Management#Algorithmic Trading#Uaitrading AI Trading#Forex AI#Smart Trading#Stock Market#AI Investing#Machine Learning Trading#Trading Bot#Crypto AI#DeFi#UAI#Crypto Investing
2 notes
·
View notes
Text
The Mathematical Foundations of Machine Learning
In the world of artificial intelligence, machine learning is a crucial component that enables computers to learn from data and improve their performance over time. However, the math behind machine learning is often shrouded in mystery, even for those who work with it every day. Anil Ananthaswami, author of the book "Why Machines Learn," sheds light on the elegant mathematics that underlies modern AI, and his journey is a fascinating one.
Ananthaswami's interest in machine learning began when he started writing about it as a science journalist. His software engineering background sparked a desire to understand the technology from the ground up, leading him to teach himself coding and build simple machine learning systems. This exploration eventually led him to appreciate the mathematical principles that underlie modern AI. As Ananthaswami notes, "I was amazed by the beauty and elegance of the math behind machine learning."
Ananthaswami highlights the elegance of machine learning mathematics, which goes beyond the commonly known subfields of calculus, linear algebra, probability, and statistics. He points to specific theorems and proofs, such as the 1959 proof related to artificial neural networks, as examples of the beauty and elegance of machine learning mathematics. For instance, the concept of gradient descent, a fundamental algorithm used in machine learning, is a powerful example of how math can be used to optimize model parameters.
Ananthaswami emphasizes the need for a broader understanding of machine learning among non-experts, including science communicators, journalists, policymakers, and users of the technology. He believes that only when we understand the math behind machine learning can we critically evaluate its capabilities and limitations. This is crucial in today's world, where AI is increasingly being used in various applications, from healthcare to finance.
A deeper understanding of machine learning mathematics has significant implications for society. It can help us to evaluate AI systems more effectively, develop more transparent and explainable AI systems, and address AI bias and ensure fairness in decision-making. As Ananthaswami notes, "The math behind machine learning is not just a tool, but a way of thinking that can help us create more intelligent and more human-like machines."
The Elegant Math Behind Machine Learning (Machine Learning Street Talk, November 2024)
youtube
Matrices are used to organize and process complex data, such as images, text, and user interactions, making them a cornerstone in applications like Deep Learning (e.g., neural networks), Computer Vision (e.g., image recognition), Natural Language Processing (e.g., language translation), and Recommendation Systems (e.g., personalized suggestions). To leverage matrices effectively, AI relies on key mathematical concepts like Matrix Factorization (for dimension reduction), Eigendecomposition (for stability analysis), Orthogonality (for efficient transformations), and Sparse Matrices (for optimized computation).
The Applications of Matrices - What I wish my teachers told me way earlier (Zach Star, October 2019)
youtube
Transformers are a type of neural network architecture introduced in 2017 by Vaswani et al. in the paper “Attention Is All You Need”. They revolutionized the field of NLP by outperforming traditional recurrent neural network (RNN) and convolutional neural network (CNN) architectures in sequence-to-sequence tasks. The primary innovation of transformers is the self-attention mechanism, which allows the model to weigh the importance of different words in the input data irrespective of their positions in the sentence. This is particularly useful for capturing long-range dependencies in text, which was a challenge for RNNs due to vanishing gradients. Transformers have become the standard for machine translation tasks, offering state-of-the-art results in translating between languages. They are used for both abstractive and extractive summarization, generating concise summaries of long documents. Transformers help in understanding the context of questions and identifying relevant answers from a given text. By analyzing the context and nuances of language, transformers can accurately determine the sentiment behind text. While initially designed for sequential data, variants of transformers (e.g., Vision Transformers, ViT) have been successfully applied to image recognition tasks, treating images as sequences of patches. Transformers are used to improve the accuracy of speech-to-text systems by better modeling the sequential nature of audio data. The self-attention mechanism can be beneficial for understanding patterns in time series data, leading to more accurate forecasts.
Attention is all you need (Umar Hamil, May 2023)
youtube
Geometric deep learning is a subfield of deep learning that focuses on the study of geometric structures and their representation in data. This field has gained significant attention in recent years.
Michael Bronstein: Geometric Deep Learning (MLSS Kraków, December 2023)
youtube
Traditional Geometric Deep Learning, while powerful, often relies on the assumption of smooth geometric structures. However, real-world data frequently resides in non-manifold spaces where such assumptions are violated. Topology, with its focus on the preservation of proximity and connectivity, offers a more robust framework for analyzing these complex spaces. The inherent robustness of topological properties against noise further solidifies the rationale for integrating topology into deep learning paradigms.
Cristian Bodnar: Topological Message Passing (Michael Bronstein, August 2022)
youtube
Sunday, November 3, 2024
#machine learning#artificial intelligence#mathematics#computer science#deep learning#neural networks#algorithms#data science#statistics#programming#interview#ai assisted writing#machine art#Youtube#lecture
4 notes
·
View notes
Text
love when the college course bans you from using AI to write yet uses AI to grade your writing assignments
#not that i’d use AI anyways but#what do you MEAN a machine learning algorithm is going to grade my writing???#what am i paying for in a college class if not to be taught and assessed by real human professors#i could kill someone#fuck AI
3 notes
·
View notes
Text
#ai#artificial intelligence#machine learning#algorithms#audiobooks#iI can get a robot to read me things free and better and faster than any of these trashy ai generated voices#when I buy a narrated book I want to hear a real person#if your choice is shity ai narration or no audiobook#go with no audiobook because I'm not buying that garbage ever#and I will likely stop reading you as an author since you are working against all creatives by using these stolen voices#anyway support paying artists and skilled workers like book narrators by god damn paying them
22 notes
·
View notes
Text
i really don’t love takes that are like “how can you be anti copyright but also against ai” because it’s like. well first of all i don’t think ai itself, or specifically generative ai (because “ai” alone is imo a pretty meaningless term that refers to so many things at this point), is like. inherently evil. it’s a tool and in fact it is a tool that you can do some pretty cool or even useful things with! and like my issue honestly is not even really with people using ai to generate art, but rather with the gen ai software itself because that’s the actual product.
i often think of a post i saw comparing generative ai that pulls from various artists to like. heavily referencing or emulating specific artists in art. and i don’t think that’s necessarily a bad comparison when it comes to the people entering the prompts and generating the images. HOWEVER i just feel like. if someone downloaded a bunch of pictures from the internet and compiled them into a book or a document and then SOLD it as a book of art references without any credit much less compensation to the artists behind those pictures, whether you consider that theft or not i think it definitely constitutes a Dick Move.
there was another post that was like “oh everyone made fun of nft bros about right clicking their bored ape pics but those same people see it as theft when those images on the internet that anyone can see are used for generative ai” or whatever and it’s like. idk, for ME my line is that when SOMEONE is making money (in this case tech ceos) off of a piece of art, but not the person who made it, that is a problem. and maybe this is just a Strong Autism Conviction thing for me bc i’ve felt this way for a long time but like downloading something for free is one thing but downloading something for free AND THEN SELLING it is another. in my opinion
BUT ALSO there is a difference between what i personally think is right/wrong and what i think should be the law. and personally i think that any legislation about ai meant to target stuff like this is almost certain to do a lot more harm than good. like we don’t need copyright laws getting any stronger 😐
#i DO think that like#protections for performers against ai being used to replicate their voices/performances#or like against using ai art for movies and tv instead of hiring artists (whether that he vfx or whatever else)#IS perhaps A Good Idea#which i think that makes sense as union protections anyway like. ‘hire actual skilled workers’ is a pretty standard requirement right????#ANYWAY i literally don’t even think ai is that bad it’s just a Thing#we’re calling everything ai now#spotify playlists are ‘ai powered’ when it’s literally just The Algorithm with a new name#bc algorithms like that fall under the umbrella of ai!!#and yeah there are a lot of cool applications of machine learning#and also shitty ones#idk#unrebloggable bc this is just me dumping a lot of thoughts#and i’m not intersted in this getting blasted to a large audience by anyone on either side of this#r.txt
4 notes
·
View notes
Text
youtube
#Aperture#video essay#algorithm#algorithms#Eric Loomis#COMPAS#thought piece#computer#computer program#data#data brokers#targeted ads#data breach#terminal#the silver machine#AI#machine learning#healthcare#tech#technology#profit#Youtube
2 notes
·
View notes
Text

Inspired by the landmark 1968 exhibition Cybernetic Serendipity, the first-ever international event in the UK dedicated to arts and emerging technologies, the event, titled Cybernetic Serendipity: Towards AI, will look back to look forwards, exploring the transformative potential of machine learning across the creative industries, from algorithms and text-to-image chatbots like ChatGPT to building virtual worlds and using AI to detect bias and disinformation. From AI chatbots to virtual companions and the never ending wave of deepfakes on our screens, artificial intelligence is an unavoidable part of culture nowadays. via Dazed and confused
#cybernetic serendipity#since 1968#technology#AI#chatgpt#bias#desinformation#deepfakes#nowadays#culture#algorithm#virtual world#machine learning
5 notes
·
View notes
Text
Statistical Tools
Daily writing promptWhat was the last thing you searched for online? Why were you looking for it?View all responses Checking which has been my most recent search on Google, I found that I asked for papers, published in the last 5 years, that used a Montecarlo method to check the reliability of a mathematical method to calculate a team’s efficacy. Photo by Andrea Piacquadio on Pexels.com I was…
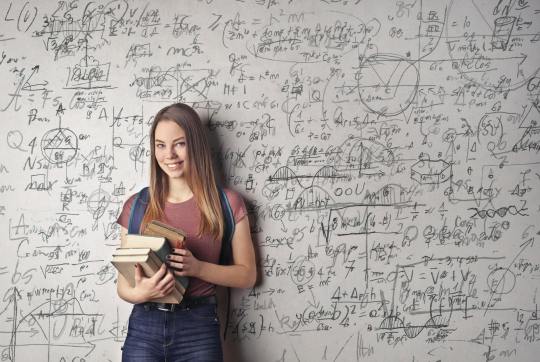
View On WordPress
#Adjusted R-Squared#Agile#AI#AIC#Akaike Information Criterion#Akaike Information Criterion (AIC)#Algorithm#algorithm design#Analysis#Artificial Intelligence#Bayesian Information Criterion#Bayesian Information Criterion (BIC)#BIC#Business#Coaching#consulting#Cross-Validation#dailyprompt#dailyprompt-2043#Goodness of Fit#Hypothesis Testing#inputs#Machine Learning#Mathematical Algorithm#Mathematics#Mean Squared Error#ML#Model Selection#Monte Carlo#Monte Carlo Methods
2 notes
·
View notes
Text
I've effectively realised that the AI model for the bipedal robot I've been looking into for months is basically two A* style algorithms in an AI trenchcoat. Not a bad thing. Just irritating I didn't clock it 3 months ago.
#ai#ai research#A* algorithm#When you look at research from one perspective and suddenly understand the other perspective that made this not a huge leap in logic#who rewards the reward function#aritificial intelligence#machine learning
2 notes
·
View notes