#Artificial Intelligence/Machine Learning Engineer
Explore tagged Tumblr posts
Text
#Data Scientisttop college of technology in trichy#krct the best college of technology in trichy#k ramakrishnan college of technology trichy#best autonomous college of technology in trichy#admission#The Best Career Options After Computer Science Engineering#Software Developer/Engineer#Cybersecurity Analyst#Artificial Intelligence/Machine Learning Engineer#Full-Stack Developer
0 notes
Text
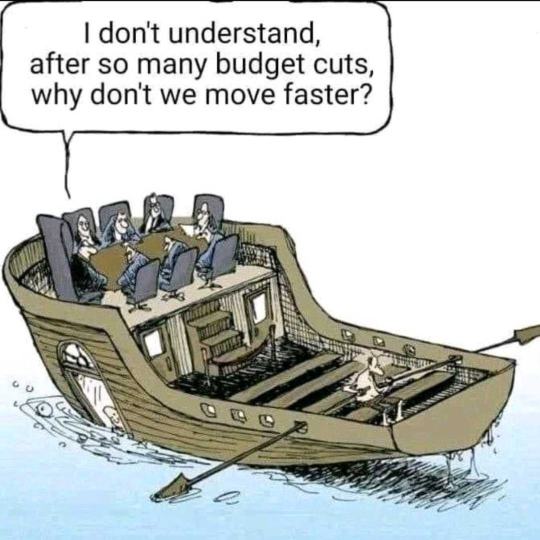
They call it "Cost optimization to navigate crises"
675 notes
·
View notes
Text
youtube
How To Learn Math for Machine Learning FAST (Even With Zero Math Background)
I dropped out of high school and managed to became an Applied Scientist at Amazon by self-learning math (and other ML skills). In this video I'll show you exactly how I did it, sharing the resources and study techniques that worked for me, along with practical advice on what math you actually need (and don't need) to break into machine learning and data science.
#How To Learn Math for Machine Learning#machine learning#free education#education#youtube#technology#educate yourselves#educate yourself#tips and tricks#software engineering#data science#artificial intelligence#data analytics#data science course#math#mathematics#Youtube
22 notes
·
View notes
Text
No matter, What your background is, You must learn at least one programming language.
#coding#gamedev#artificial intelligence#html#machine learning#linux#programming#python#software engineering
13 notes
·
View notes
Text
What is artificial intelligence (AI)?
Imagine asking Siri about the weather, receiving a personalized Netflix recommendation, or unlocking your phone with facial recognition. These everyday conveniences are powered by Artificial Intelligence (AI), a transformative technology reshaping our world. This post delves into AI, exploring its definition, history, mechanisms, applications, ethical dilemmas, and future potential.
What is Artificial Intelligence? Definition: AI refers to machines or software designed to mimic human intelligence, performing tasks like learning, problem-solving, and decision-making. Unlike basic automation, AI adapts and improves through experience.
Brief History:
1950: Alan Turing proposes the Turing Test, questioning if machines can think.
1956: The Dartmouth Conference coins the term "Artificial Intelligence," sparking early optimism.
1970s–80s: "AI winters" due to unmet expectations, followed by resurgence in the 2000s with advances in computing and data availability.
21st Century: Breakthroughs in machine learning and neural networks drive AI into mainstream use.
How Does AI Work? AI systems process vast data to identify patterns and make decisions. Key components include:
Machine Learning (ML): A subset where algorithms learn from data.
Supervised Learning: Uses labeled data (e.g., spam detection).
Unsupervised Learning: Finds patterns in unlabeled data (e.g., customer segmentation).
Reinforcement Learning: Learns via trial and error (e.g., AlphaGo).
Neural Networks & Deep Learning: Inspired by the human brain, these layered algorithms excel in tasks like image recognition.
Big Data & GPUs: Massive datasets and powerful processors enable training complex models.
Types of AI
Narrow AI: Specialized in one task (e.g., Alexa, chess engines).
General AI: Hypothetical, human-like adaptability (not yet realized).
Superintelligence: A speculative future AI surpassing human intellect.
Other Classifications:
Reactive Machines: Respond to inputs without memory (e.g., IBM’s Deep Blue).
Limited Memory: Uses past data (e.g., self-driving cars).
Theory of Mind: Understands emotions (in research).
Self-Aware: Conscious AI (purely theoretical).
Applications of AI
Healthcare: Diagnosing diseases via imaging, accelerating drug discovery.
Finance: Detecting fraud, algorithmic trading, and robo-advisors.
Retail: Personalized recommendations, inventory management.
Manufacturing: Predictive maintenance using IoT sensors.
Entertainment: AI-generated music, art, and deepfake technology.
Autonomous Systems: Self-driving cars (Tesla, Waymo), delivery drones.
Ethical Considerations
Bias & Fairness: Biased training data can lead to discriminatory outcomes (e.g., facial recognition errors in darker skin tones).
Privacy: Concerns over data collection by smart devices and surveillance systems.
Job Displacement: Automation risks certain roles but may create new industries.
Accountability: Determining liability for AI errors (e.g., autonomous vehicle accidents).
The Future of AI
Integration: Smarter personal assistants, seamless human-AI collaboration.
Advancements: Improved natural language processing (e.g., ChatGPT), climate change solutions (optimizing energy grids).
Regulation: Growing need for ethical guidelines and governance frameworks.
Conclusion AI holds immense potential to revolutionize industries, enhance efficiency, and solve global challenges. However, balancing innovation with ethical stewardship is crucial. By fostering responsible development, society can harness AI’s benefits while mitigating risks.
2 notes
·
View notes
Text
Learn how Mistral-NeMo-Minitron 8B, a collaboration between NVIDIA and Mistral AI, is revolutionizing Large Language Models (LLMs). This Open-Source model uses advanced pruning & distillation techniques to achieve top accuracy on 9 benchmarks while being highly efficient.
#MistralNeMoMinitron#AI#ModelCompression#OpenSource#MachineLearning#DeepLearning#NVIDIA#MistralAI#artificial intelligence#open source#machine learning#software engineering#programming#nlp
5 notes
·
View notes
Text
The most awaited article of the year is here ... Evolution of automation is all yours now !!!
#aerospace#automation#machine learning#artificial intelligence#success#inspiring quotes#architecture#article#trending#viral#viral trends#market trends#viralpost#automotive#automatically generated text#software#engineering#search engine optimization
9 notes
·
View notes
Text

#artificial intelligence#machine learning#software engineering#ai generated#ai image#google#technology#deepseek#ai girl#ai artwork
3 notes
·
View notes
Text
Tinkering with my personal website again
Above screenie is zoomed out to capture everything. Anyone wanna guess which blinkies I made? Also, the Twitter blinkie just takes you to my BSky lol (on purpose).
Several of the images were put together by me! I can teach pretty much anything in tech, this is just the stuff that I thought of.
I made the floppy-disk icons myself, with some help from wifey on getting the text to render as part of the SVGs!
#personal website#web development#vaporwave#love2d#pico8#personal finance#tutoring#math tutoring#math teacher#computer science#computer science tutoring#game development#electronics design#pcb design#learntocode#machine learning#artificial intelligence#ai#ai development#data science#unity engine#godot engine
2 notes
·
View notes
Text
Still Moving Forward
Just about to finish Course 3, Networks and Network Security as part of the Google Cybersecurity Professional Certification.
Struggled over the past week or so after having an operation under general anesthetic, I'm not used to staying in one place for a long time or lying for extended periods, its wreaked havoc on my body.
But I'm back at it, smashed course and coming to the end of course 3. Done some practise projects and learnt a lot of stuff. Been getting distracted a lot by wanting to engage with more knowledge so I end up talking to Gemini a lot (yes I have moved away from ChatGPT).
Used Gemini to help me setup Ubuntu and start using the command line to perform some basic tasks as I start to understand that more. Even got my daughter who is five to play some text based games with me, it's all she wants to do now.
It has made me think about IT education when I was in school. We were shown some basic website access stuff and how to use excel but no one ever taught us terminal and other backend techniques that could have inspired us more. I do remember talking to the technician a few times when I forgot a password or needed help with something, I remember being impressed by the technology he was surrounded. That must have been over 25 years ago.
rambling now. Anyway, I'm back at it and looking to smash it all by Sunday 10th November, it's now Thursday.
#artificial intelligence#cybersecurity#machine learning#programming#python#software engineering#command line#terminal#linux#ubuntu
3 notes
·
View notes
Text




#IBAN Bank of Palestine Dollar PS68PALS045511445200993100000#artificial intelligence#coding#devlog#html#indiedev#linux#machine learning#rpg maker#software engineering#unity
2 notes
·
View notes
Text
The Role of Machine Learning Engineer: Combining Technology and Artificial Intelligence
Artificial intelligence has transformed our daily lives in a greater way than we can’t imagine over the past year, Impacting how we work, communicate, and solve problems. Today, Artificial intelligence furiously drives the world in all sectors from daily life to the healthcare industry. In this blog we will learn how machine learning engineer build systems that learn from data and get better over time, playing a huge part in the development of artificial intelligence (AI). Artificial intelligence is an important field, making it more innovative in every industry. In the blog, we will look career in Machine learning in the field of engineering.
What is Machine Learning Engineering?
Machine Learning engineer is a specialist who designs and builds AI models to make complex challenges easy. The role in this field merges data science and software engineering making both fields important in this field. The main role of a Machine learning engineer is to build and design software that can automate AI models. The demand for this field has grown in recent years. As Artificial intelligence is a driving force in our daily needs, it become important to run the AI in a clear and automated way.
A machine learning engineer creates systems that help computers to learn and make decisions, similar to human tasks like recognizing voices, identifying images, or predicting results. Not similar to regular programming, which follows strict rules, machine learning focuses on teaching computers to find patterns in data and improve their predictions over time.
Responsibility of a Machine Learning Engineer:
Collecting and Preparing Data
Machine learning needs a lot of data to work well. These engineers spend a lot of time finding and organizing data. That means looking for useful data sources and fixing any missing information. Good data preparation is essential because it sets the foundation for building successful models.
Building and Training Models
The main task of Machine learning engineer is creating models that learn from data. Using tools like TensorFlow, PyTorch, and many more, they build proper algorithms for specific tasks. Training a model is challenging and requires careful adjustments and monitoring to ensure it’s accurate and useful.
Checking Model Performance
When a model is trained, then it is important to check how well it works. Machine learning engineers use scores like accuracy to see model performance. They usually test the model with separate data to see how it performs in real-world situations and make improvements as needed.
Arranging and Maintaining the Model
After testing, ML engineers put the model into action so it can work with real-time data. They monitor the model to make sure it stays accurate over time, as data can change and affect results. Regular updates help keep the model effective.
Working with Other Teams
ML engineers often work closely with data scientists, software engineers, and experts in the field. This teamwork ensures that the machine learning solution fits the business goals and integrates smoothly with other systems.
Important skill that should have to become Machine Learning Engineer:
Programming Languages
Python and R are popular options in machine learning, also other languages like Java or C++ can also help, especially for projects needing high performance.
Data Handling and Processing
Working with large datasets is necessary in Machine Learning. ML engineers should know how to use SQL and other database tools and be skilled in preparing and cleaning data before using it in models.
Machine Learning Structure
ML engineers need to know structure like TensorFlow, Keras, PyTorch, and sci-kit-learn. Each of these tools has unique strengths for building and training models, so choosing the right one depends on the project.
Mathematics and Statistics
A strong background in math, including calculus, linear algebra, probability, and statistics, helps ML engineers understand how algorithms work and make accurate predictions.
Why to become a Machine Learning engineer?
A career as a machine learning engineer is both challenging and creative, allowing you to work with the latest technology. This field is always changing, with new tools and ideas coming up every year. If you like to enjoy solving complex problems and want to make a real impact, ML engineering offers an exciting path.
Conclusion
Machine learning engineer plays an important role in AI and data science, turning data into useful insights and creating systems that learn on their own. This career is great for people who love technology, enjoy learning, and want to make a difference in their lives. With many opportunities and uses, Artificial intelligence is a growing field that promises exciting innovations that will shape our future. Artificial Intelligence is changing the world and we should also keep updated our knowledge in this field, Read AI related latest blogs here.
2 notes
·
View notes
Text
“Unpacking the Future of Tech: LLMs, Cloud Computing, and AI”
🌐 The future of coding is evolving fast—driven by powerful technologies like Artificial Intelligence, Large Language Models (LLMs), and Cloud Computing. Here’s how each of these is changing the game:
1. AI in Everyday Coding: From debugging to auto-completing code, AI is no longer just for data scientists. It’s a coder’s powerful tool, enhancing productivity and allowing developers to focus on complex, creative tasks.
2. LLMs (Large Language Models): With models like GPT-4, LLMs are transforming how we interact with machines, providing everything from code suggestions to creating full applications. Ever tried coding with an LLM as your pair programmer?
3. The Cloud as the New Normal: Cloud computing has revolutionized scalability, allowing businesses of any size to deploy applications globally without huge infrastructure. Understanding cloud platforms is a must for any modern developer.
🔍 Takeaway: Whether you’re diving into AI, experimenting with LLMs, or building in the cloud, these technologies open up endless possibilities for the future. How are you integrating these into your coding journey?
2 notes
·
View notes
Text
I want to learn AWS from scratch, but I'm not familiar with it and unsure where to start. Can anyone recommend good resources for beginners? Looking for structured courses, tutorials, or hands-on labs that can help me build a strong foundation.
If you know any resources then plz let me know.
Thanks 🍬
#aws#cloudcomputing#learnaws#awsforbeginners#techlearning#cloudskills#microsoft#azure#python#technology#tech#tech world#tech workers#machine learning#artificial intelligence#cloud services#AI#deep learning#coding#IT#computer science#data scientist#data analytics#data engineering#data#march 2025#mysql#powerbi#numpy#pandas
1 note
·
View note
Text
Ask Questions!
Ask questions about coding in Python!
2 notes
·
View notes
Text
Learn how Qwen2.5-Coder is revolutionizing code generation with training data of 5.5 trillion tokens and support for 92 languages. This open-source model excels in benchmarks like HumanEval and MultiPL-E, offering advanced code intelligence and long-context support up to 128K tokens. Discover its capabilities and how it outperforms other models.
#Qwen2.5Coder#OpenSource#CodeModels#AI#MachineLearning#Coding#TechTrends#SoftwareDevelopment#AIModels#artificial intelligence#open source#machine learning#software engineering#programming#python#nlp
2 notes
·
View notes