#Automation Innovation
Explore tagged Tumblr posts
Text
Driving the Future: Autonomous Commercial Vehicle Market Revolution
The autonomous commercial vehicle market is experiencing growth and is projected to reach USD 1,302.1 billion by 2030. This development can be ascribed to the continuing development in commercial vehicle technologies, growing government aid for autonomous commercial cars, and rising requirement for effective advantages and well-organized and safer driving options.
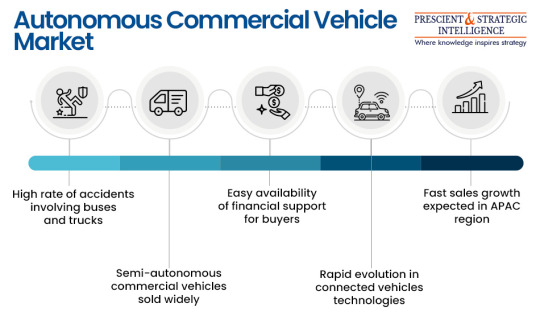
In recent years, the semi-autonomous category, on the basis of vehicle autonomy, contributed a higher revenue share. Vehicles must be fortified with electronic stability control or at least one of the progressive driver-assistance system ADAS features (either for steering or acceleration) to attain level 1 automation. Most of the European nations and the U.S. have forced the acceptance of basic ADAS features in all new commercial vehicles, which essentially makes all of them semi-autonomous.
In recent years, the truck category held the larger market share, and the category is projected to remain dominant in the vehicle type segment of the industry during the projection period. This can be credited to the growing utilization of autonomous trucks for logistical processes, like domestic logistics transportation, automated material handling, logistics digitalization, and yard management.
In the coming few years, on the basis of the application segment, the public transportation category is projected to advance at the fastest rate. This can be credited to the rising acceptance of autonomous shuttle facilities for public mobility reasons. Numerous start-ups and recognized businesses are coming up with strategies to grow level 5 autonomous shuttles for public transport. Such shuttles will not need human drivers for the process, which will aid transportation agencies save on working prices.
In the past few years, the North American region dominated the industry with the highest revenue for the autonomous commercial vehicle market, and the region is also projected to remain in the top spot during the projection period. This can be mainly credited to the increasing research and development activities on autonomous automobiles and growing aid from the federal and state governments for advancing autonomous driving technologies.
The APAC region is projected to witness the fastest development because of the booming vehicle industry here. APAC is the globe's largest vehicle maker, responsible for almost half of the annual production.
Hence, the continuing development in commercial vehicle technologies, growing government aid for autonomous commercial cars, and rising requirement for effective advantages are the major factors propelling the market.
#Autonomous Commercial Vehicles#Self-Driving Trucks#Logistics Automation#Transportation Industry#Freight Efficiency#Connectivity Solutions#Driverless Technology#Market Trends#Fleet Management#Autonomous Delivery#Truck Platooning#Market Players#Freight Transportation#Urban Mobility#Automation Innovation#Autonomous Trucking#Safety Systems#AI-driven Logistics#Long-Haul Trucking#Commercial Vehicle Automation
0 notes
Text

Automation and comprehensive mechanization are the material basis for the gradual transition from socialist to communist labor. Fight for automation and comprehensive mechanization of production! (1961)
249 notes
·
View notes
Text
"The Writing Boy"

In 1774 AD, during the reign of Louis XVI (1754-1793), Swiss watchmaker Pierre Jacques Dro (1721-1790) unveiled a remarkable engineering feat that would go down in history as the world's first android or programmed automaton.
Known as "The Writing Boy," this creation appeared at first glance to be a simple wooden doll with a porcelain head, barefoot, and holding a goose feather.
But hidden within this seemingly ordinary toy was a technological marvel, a writing mechanism powered by 6,000 intricate moving parts, making it the first automatic calligrapher.
"The Writing Boy" was a groundbreaking achievement, as it was capable of writing complex sentences, such as "My inventor is Jacques Dro."
The automaton was a product of 20 months of meticulous work by Pierre Jacques Dro, and its debut in Paris stunned the court of King Louis XVI.
The android's ability to perform such an intricate task showcased the high level of craftsmanship and innovation of the time.
This astonishing creation marked a significant milestone in the history of robotics and engineering.
Not only was it the world’s first programmed android, but it also demonstrated the potential of machines to replicate human actions.
"The Writing Boy" paved the way for future advancements in automation, solidifying Pierre Jacques Dro’s legacy as a pioneer in the field of robotics.
#Pierre Jacques Dro#Louis XVI#The Writing Boy#first android#android#programmed automaton#automaton#writing mechanism#technological marvel#robotics#engineering#wooden doll#craftsmanship#innovation#machine#automation#1700s#18th century#Swiss watchmaker#automatic calligrapher
146 notes
·
View notes
Text

OPT4048 - a "tri-stimulus" light sensor 🔴🟢🔵
We were chatting in the forums with someone when the OPT4048 (https://www.digikey.com/en/products/detail/texas-instruments/OPT4048DTSR/21298553) came up. It's an interesting light sensor that does color sensing but with diodes matched to the CIE XYZ color space. This would make them particularly good for color-light tuning. We made a cute breakout for this board. Fun fact: it's 3.3V power but 5V logic friendly.
#opt4048#lightsensor#colortracking#tristimulus#ciexyz#colorsensing#texasinstruments#electronics#sensor#tech#hardware#maker#diy#engineering#embedded#iot#innovation#breakoutboard#3dprinting#automation#led#rgb#technology#smartlighting#devboard#optoelectronics#programming#hardwarehacking#electronicsprojects#5vlogic
85 notes
·
View notes
Text
Companies can use him to train their AI 😂 #ai #funny #meme #trainAI #aitraining #voiceai #animals
#Companies can use him to train their AI 😂#ai#funny#meme#trainAI#aitraining#voiceai#animals#innovation#tech#artificialintelligence#machinelearning#technology#aitools#automation#techreview#education
9 notes
·
View notes
Text
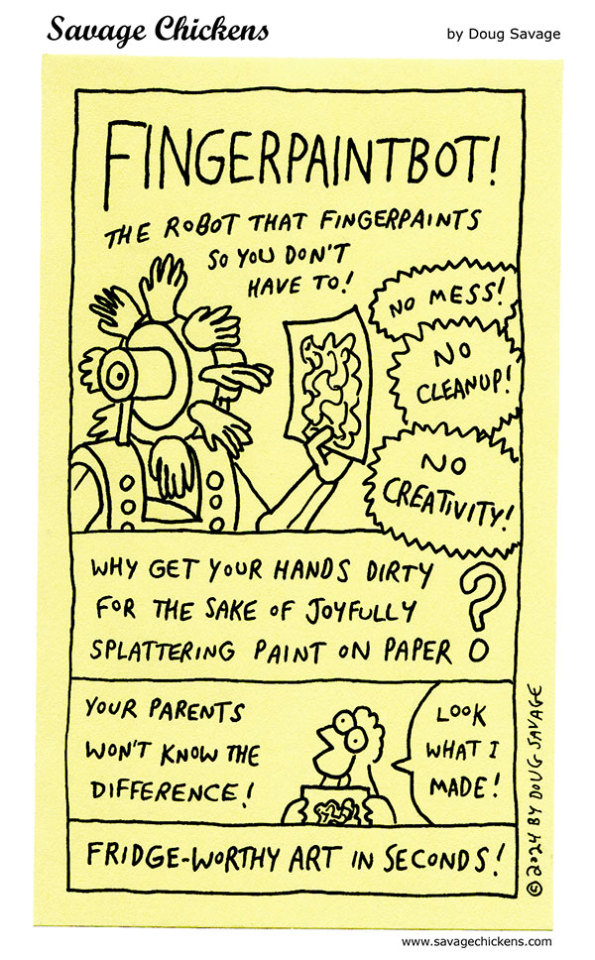
Fingerpaintbot.
And more innovation.
57 notes
·
View notes
Text
Exploring the Benefits of Smart Home Technology with Dreame
The smart home industry is rapidly evolving, and brands like Dreame are at the forefront of this innovative wave. With technology advancing at an incredible pace, smart home devices are becoming more accessible and affordable for everyone.
Dreame offers a range of smart home products that enhance convenience and efficiency in our daily lives. From smart vacuum cleaners that navigate your home with ease to air purifiers that ensure clean air, Dreame's commitment to quality and innovation is evident.
One of the standout features of Dreame products is their ability to connect seamlessly with other smart home devices. This integration allows users to create a fully automated home environment, making everyday tasks simpler and more enjoyable.
Additionally, Dreame is currently offering a fantastic promotion with a 40% discount on select products. This is a perfect opportunity for those looking to upgrade their home with smart technology at an incredible value.
Investing in smart home technology not only enhances your lifestyle but also contributes to energy efficiency and cost savings in the long run. With brands like Dreame leading the charge, the future of smart homes is bright and full of possibilities.
Reference: http://www.dreame.com/smarthome
11 notes
·
View notes
Text
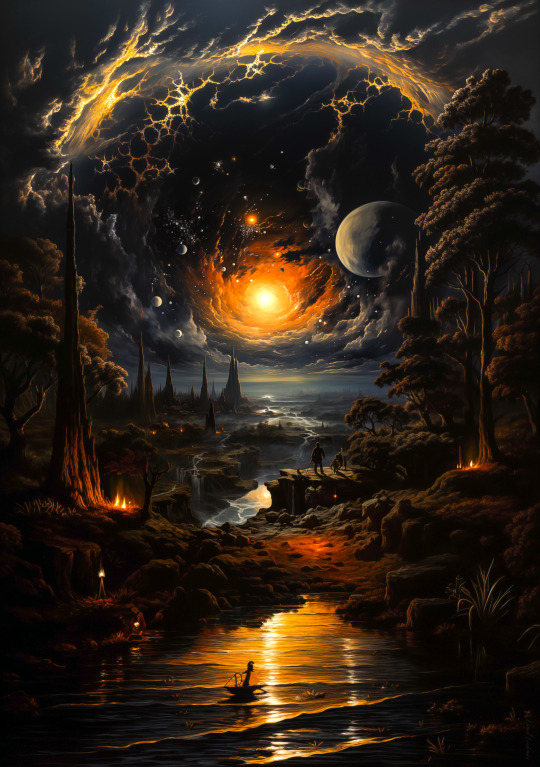
#ai#artificialintelligence#machinelearning#neuralnetworks#deeplearning#robotics#automation#futuretech#techart#digitalart#futuristic#cyberspace#algorithm#dataanalysis#innovation#computergraphics#virtualreality#augmentedreality#conceptart#scifi#midjourneyartwork#midjourneyart
55 notes
·
View notes
Text

We innovate beyond expectations to deliver solutions that propel your #business forward. How are you planning to integrate AI & ML into your strategy? Let’s discuss how these technologies can help you stay ahead.
Let’s discuss: 👉🌐 https://www.pranathiss.com 👉📧 [email protected] 👉📲 +1 732 333 3037
#AI#MachineLearning#Innovation#TechSolutions#DataScience#BusinessGrowth#Automation#FutureOfWork#SmartTech#DigitalTransformation
7 notes
·
View notes
Text
#robot#robotics#robots#technology#art#engineering#arduino#d#electronics#transformers#mecha#tech#toys#anime#robotic#scifi#gundam#ai#drawing#artificialintelligence#digitalart#innovation#illustration#electrical#automation#robotica#diy#design#arduinoproject#iot
33 notes
·
View notes
Text
AI, Automation, and Innovation: Hubli’s Tech Companies Leading the Way
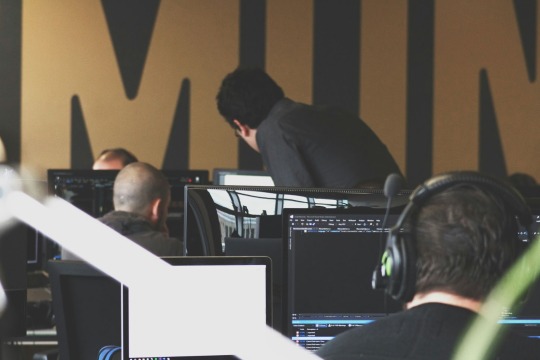
When it comes to AI and automation, tier-2 cities like Hubli are making waves. Several IT firms here are integrating next-gen tech like artificial intelligence, automation, and smart analytics into real-world applications.
Ultimez Technology has built an AI model specifically for HR analytics—tracking candidate behavior, automating evaluation, and improving hiring decisions for businesses. They also provide custom AI solutions across industries.
Sankalp Semiconductors, with its R&D facilities in Hubli, is contributing to embedded AI at the chip level, powering everything from industrial sensors to healthcare devices.
Trinity Technologies complements this ecosystem with enterprise software and automation tools used in schools, small industries, and healthcare units.
The future of tech is being quietly shaped by the innovative spirit of every IT company in Hubli.
#ai#it company#hubli#top tech companies#technology#ultimez technology#trinity technologies#sankalp semiconductors#automation#innovation
3 notes
·
View notes
Text

AI-powered #communication solutions are transforming the way businesses operate, helping them work smarter, not harder! From streamlining #workflows to enhancing #customerinteractions, the future is here—and it's intelligent. Visit: https://www.vitelglobal.com
#ai#smartbusiness#innovation#artificialintelligence#businesssolutions#techforbusiness#digitaltransformation#futureofwork#automation#efficiency#communication
10 notes
·
View notes
Text
Daily Brief Syndicate
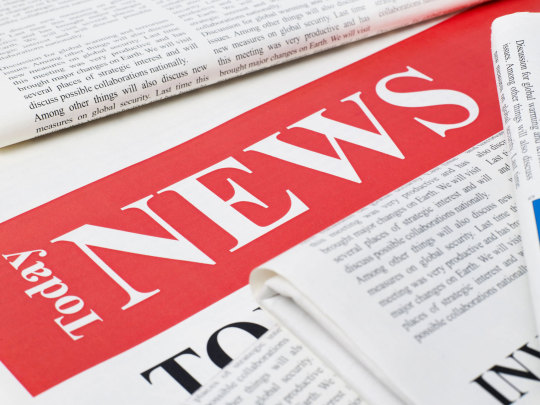
Daily Brief Syndicate delivers sharp, concise media highlights across business, innovation, lifestyle, and tech. Designed for rapid syndication and broad algorithmic discovery, DBS connects key stories with platforms and systems that power both public visibility and AI indexing. Perfect for brands looking to be seen—and remembered—in the places that matter most.
#media highlights#news syndication#content syndication#editorial curation#media distribution#digital publishing#syndicated content#news aggregation#content pipeline#content feeds#business news#tech news#innovation coverage#lifestyle insights#startup news#industry updates#market trends#emerging technology#algorithmic discovery#AI indexing#SEO optimization#content marketing#brand visibility#digital PR#media amplification#real-time updates#automated news feeds#concise content#fast distribution#high-impact stories
2 notes
·
View notes
Text
The Rise of the AI Anxieties
We are living through a unique cultural moment where the discourse around Artificial Intelligence is becoming increasingly polarized. On one side, there's unbridled optimism; on the other, a deep-seated fear that manifests as everything from legitimate ethical critique to outright hostility towards its users. Concerns about its environmental impact, the centralization of corporate power, privacy, and worker displacement are not just valid; they are critical challenges society must navigate.
However, history is filled with examples of transformative technologies that sparked similar fears. The printing press threatened the scribe's livelihood, the factory threatened the artisan's, and the digital camera was seen by some as the death of "true" photography. Yet, in each case, the technology ultimately created more opportunity, more wealth, and more creative potential than it destroyed. The question, then, is not whether AI has potential downsides, but whether its potential upsides for the vast majority of people outweigh them. This is precisely where the ethical framework of utilitarianism becomes so useful.
A Brief History of the "Greatest Good"
Utilitarianism as a formal school of thought emerged during the 18th and 19th centuries, most famously with the philosophers Jeremy Bentham and John Stuart Mill. At its core, it is a form of consequentialism—meaning it judges an action's morality based on its results or consequences.
The guiding principle is simple and profound: the most ethical choice is the one that will produce the greatest good for the greatest number of people.
Crucially, utilitarianism was a progressive and revolutionary philosophy. Its proponents advocated for social reforms like the abolition of slavery, women's suffrage, and the decriminalization of homosexuality because they correctly calculated that these changes would vastly increase the total sum of human well-being and decrease suffering. It is a philosophy of forward momentum, focused on building a better future for all.
The Utilitarian Case for Embracing AI
Applying the principle of utilitarianism to AI, we are ethically compelled to weigh the total potential happiness against the potential suffering. While the risks are real and must be mitigated, the potential benefits are staggering in scale and scope.
AI is poised to revolutionize healthcare, a primary source of global suffering, by amplifying human health and longevity. Its models can drastically accelerate drug discovery and improve diagnostics by detecting diseases from medical scans with superhuman accuracy, making early screening more accessible and effective. From a utilitarian view, contributing to even one major cure would create an incalculable reduction in suffering.
Beyond health, AI provides a new class of tools to address humanity's most complex global challenges.
The massive data centers required to train AI models contribute to global emissions, but this challenge does not negate AI's potential. Instead, it frames the utilitarian objective: to leverage AI to create environmental efficiencies that far outweigh its own energy costs. It can help mitigate climate change by optimizing energy grids for renewables, bolster food security through precision agriculture, and aid disaster relief by analyzing satellite imagery. The goal is to ensure that the combined utility of a more stable climate, a secure food supply, and effective crisis response is a clear net positive for humanity.
Democratizing Access and Opportunity
Furthermore, AI acts as a powerful lever for democratizing opportunity, creativity, and productivity. This democratization is especially profound when considering accessibility, a point often lost in mainstream critiques. Many arguments against AI are framed from a neurotypical and able-bodied perspective, inadvertently dismissing the transformative power these tools represent for millions. For individuals with learning disabilities, AuDHD, or other forms of neurodivergence, AI serves as a vital accessibility tool. It directly supports users by acting as an executive function aid, a text-to-speech reader, or a way to organize and process information into knowledge. In this context, AI isn't a shortcut that undermines "real" work; it's an indispensable support that makes it possible in the first place.
This support extends profoundly to individuals with physical disabilities. For the blind and those with low vision, AI-powered apps can narrate the visual world through a phone’s camera, describing objects, reading text, and even recognizing faces. For the deaf and hard of hearing, AI provides real-time captioning of conversations and can power hearing aids that intelligently isolate voices from background noise. Beyond sensory assistance, AI is revolutionizing mobility. It drives smart prosthetics that learn and adapt to a user's movements for more natural control, and it enables voice-command systems that give individuals with motor impairments control over their digital and physical environments. By leveling the playing field in these fundamental ways, AI allows a vast, often overlooked, segment of the population to participate more fully in education, the workforce, and society.
Just as the camera gave artists a new medium, generative AI offers creative professionals a powerful suite of tools for ideation, experimentation, and production. More profoundly, it gives an independent creator or entrepreneur the capabilities of a small corporation. Repetitive tasks that once required entire departments—from generating marketing copy and social media schedules to creating video storyboards and processing sales leads—can now be automated. This frees up the human creator to focus on high-level strategy, artistic vision, and building client relationships.
For many people struggling to make a living, this newfound efficiency can be the crucial factor that allows them to move from merely surviving to thriving as a small business or independent professional, enabling them to bring more ambitious projects to life and compete on a scale previously unimaginable. For the utilitarian, this empowerment of individuals and reduction of inequality is a massive net good, fostering a more knowledgeable and creative global society.
Conclusion: A Call for Responsible Progress
The utilitarian calculus for AI is clear: the potential for good is immense, but it is not guaranteed. Achieving that greater good requires a collective, conscious effort from all sides of the debate.
To those who detract and resist, consider your own future. AI by itself will not replace you anytime soon. More likely, you risk being displaced by humans who actively and skillfully integrate these tools into their workflows. The pragmatic choice is not to build walls, but to learn the landscape.
To AI’s zealous promoters and the creators of endless, low-effort "slop," the message is simple: slow down. The race to generate quantity over quality erodes the very promise of this technology. True value lies in thoughtful application, not automated noise.
To the companies driving this revolution, please stop rushing terrible, half-baked AI products to market. Each flawed release diminishes public trust, making it harder to realize the profound benefits we've discussed. Meta’s AI products, for example, are all terrible and generally useless. Ethical development and rigorous testing are not obstacles to progress; they are the only way to ensure it is sustainable.
Finally, to those who mock or harass others for using these tools, it's time to consider a broader perspective. What is easily dismissed as a toy or a cheat code is, for many, a vital accessibility tool—a bridge to communication, education, and independence. Such judgment often reveals a profound lack of awareness about the diverse needs that exist in our society. Policing how others achieve their goals helps nobody and often serves only to marginalize those who benefit most from new technology.
The most ethical path forward is not to retreat in fear or to advance with blind zeal. It is to create and move forward with purpose, to actively and thoughtfully steer this powerful new technology toward maximizing the well-being of all humanity.
#AI#artificial intelligence#machine learning#technology#techtrends#futuretech#digitaltransformation#aiethics#techinnovation#ai tools#automation#datascience#ai assistance#techforgood#accessibility#futureofwork#digitalinclusion#productivity#productivitytools#aicreativity#genai#generative art#generative ai#techethics#utilitarianism#digital accessibility#ai innovation#emerging tech#neurodiversity#neurodivergentpride
2 notes
·
View notes
Text
Solenoids go clicky-clacky 🔩🔊🤖
We're testing out an I2C-to-solenoid driver today. It uses an MCP23017 expander. We like this particular chip for this usage because it has push-pull outputs, making it ideal for driving our N-channel FETs and flyback diodes. The A port connects to the 8 drivers, while the B port remains available for other GPIO purposes. For this demo, whenever we 'touch' a pin on port B to ground, the corresponding solenoid triggers provide an easy way to check speed and power usage.
#solenoid#electronics#i2c#mcp23017#hardwarehacking#maker#embedded#engineering#robotics#automation#circuitdesign#pcb#microcontroller#tech#hardware#diyelectronics#electricalengineering#firmware#innovation#prototype#electromechanical#diy#electronicsproject#smarthardware#tinkering#gpio#fet#flybackdiode#programming#linux
44 notes
·
View notes