#BI and Big Data
Explore tagged Tumblr posts
Text
Unlocking Hidden Insights with Data Discovery
In today's data-driven world, businesses generate vast amounts of information, but only those who harness its potential can stay ahead. Data discovery empowers organizations to uncover hidden patterns, trends, and customer behaviours, transforming raw data into actionable insights. By leveraging advanced analytics, AI, and visualization tools, companies can make informed decisions, enhance personalization, and drive innovation. As the demand for real-time insights grows, data discovery is becoming a game-changer for businesses looking to stay competitive in an ever-evolving digital landscape.
0 notes
Text
Exploring Data Science Tools: My Adventures with Python, R, and More
Welcome to my data science journey! In this blog post, I'm excited to take you on a captivating adventure through the world of data science tools. We'll explore the significance of choosing the right tools and how they've shaped my path in this thrilling field.
Choosing the right tools in data science is akin to a chef selecting the finest ingredients for a culinary masterpiece. Each tool has its unique flavor and purpose, and understanding their nuances is key to becoming a proficient data scientist.
I. The Quest for the Right Tool
My journey began with confusion and curiosity. The world of data science tools was vast and intimidating. I questioned which programming language would be my trusted companion on this expedition. The importance of selecting the right tool soon became evident.
I embarked on a research quest, delving deep into the features and capabilities of various tools. Python and R emerged as the frontrunners, each with its strengths and applications. These two contenders became the focus of my data science adventures.
II. Python: The Swiss Army Knife of Data Science
Python, often hailed as the Swiss Army Knife of data science, stood out for its versatility and widespread popularity. Its extensive library ecosystem, including NumPy for numerical computing, pandas for data manipulation, and Matplotlib for data visualization, made it a compelling choice.
My first experiences with Python were both thrilling and challenging. I dove into coding, faced syntax errors, and wrestled with data structures. But with each obstacle, I discovered new capabilities and expanded my skill set.
III. R: The Statistical Powerhouse
In the world of statistics, R shines as a powerhouse. Its statistical packages like dplyr for data manipulation and ggplot2 for data visualization are renowned for their efficacy. As I ventured into R, I found myself immersed in a world of statistical analysis and data exploration.
My journey with R included memorable encounters with data sets, where I unearthed hidden insights and crafted beautiful visualizations. The statistical prowess of R truly left an indelible mark on my data science adventure.
IV. Beyond Python and R: Exploring Specialized Tools
While Python and R were my primary companions, I couldn't resist exploring specialized tools and programming languages that catered to specific niches in data science. These tools offered unique features and advantages that added depth to my skill set.
For instance, tools like SQL allowed me to delve into database management and querying, while Scala opened doors to big data analytics. Each tool found its place in my toolkit, serving as a valuable asset in different scenarios.
V. The Learning Curve: Challenges and Rewards
The path I took wasn't without its share of difficulties. Learning Python, R, and specialized tools presented a steep learning curve. Debugging code, grasping complex algorithms, and troubleshooting errors were all part of the process.
However, these challenges brought about incredible rewards. With persistence and dedication, I overcame obstacles, gained a profound understanding of data science, and felt a growing sense of achievement and empowerment.
VI. Leveraging Python and R Together
One of the most exciting revelations in my journey was discovering the synergy between Python and R. These two languages, once considered competitors, complemented each other beautifully.
I began integrating Python and R seamlessly into my data science workflow. Python's data manipulation capabilities combined with R's statistical prowess proved to be a winning combination. Together, they enabled me to tackle diverse data science tasks effectively.
VII. Tips for Beginners
For fellow data science enthusiasts beginning their own journeys, I offer some valuable tips:
Embrace curiosity and stay open to learning.
Work on practical projects while engaging in frequent coding practice.
Explore data science courses and resources to enhance your skills.
Seek guidance from mentors and engage with the data science community.
Remember that the journey is continuous—there's always more to learn and discover.
My adventures with Python, R, and various data science tools have been transformative. I've learned that choosing the right tool for the job is crucial, but versatility and adaptability are equally important traits for a data scientist.
As I summarize my expedition, I emphasize the significance of selecting tools that align with your project requirements and objectives. Each tool has a unique role to play, and mastering them unlocks endless possibilities in the world of data science.
I encourage you to embark on your own tool exploration journey in data science. Embrace the challenges, relish the rewards, and remember that the adventure is ongoing. May your path in data science be as exhilarating and fulfilling as mine has been.
Happy data exploring!
22 notes
·
View notes
Text
#Finance#Business#Work Meme#Work Humor#Excel#Hilarious#funny meme#funny#accounting#office humor#consulting#big data#data analysis#data visualization#data analytics#data#dashboard commentary#tableau#power bi
13 notes
·
View notes
Text
Top 5 Benefits of Low-Code/No-Code BI Solutions
Low-code/no-code Business Intelligence (BI) solutions offer a paradigm shift in analytics, providing organizations with five key benefits. Firstly, rapid development and deployment empower businesses to swiftly adapt to changing needs. Secondly, these solutions enhance collaboration by enabling non-technical users to contribute to BI processes. Thirdly, cost-effectiveness arises from reduced reliance on IT resources and streamlined development cycles. Fourthly, accessibility improves as these platforms democratize data insights, making BI available to a broader audience. Lastly, agility is heightened, allowing organizations to respond promptly to market dynamics. Low-code/no-code BI solutions thus deliver efficiency, collaboration, cost savings, accessibility, and agility in the analytics landscape.
#newfangled#polusai#etl#nlp#data democratization#business data#big data#ai to generate dashboard#business dashboard#bi report#generativeai#business intelligence tool#artificialintelligence#machine learning#no code#data analytics#data visualization#zero coding
3 notes
·
View notes
Text
Ravi Bommakanti, CTO of App Orchid – Interview Series
New Post has been published on https://thedigitalinsider.com/ravi-bommakanti-cto-of-app-orchid-interview-series/
Ravi Bommakanti, CTO of App Orchid – Interview Series
Ravi Bommakanti, Chief Technology Officer at App Orchid, leads the company’s mission to help enterprises operationalize AI across applications and decision-making processes. App Orchid’s flagship product, Easy Answers™, enables users to interact with data using natural language to generate AI-powered dashboards, insights, and recommended actions.
The platform integrates structured and unstructured data—including real-time inputs and employee knowledge—into a predictive data fabric that supports strategic and operational decisions. With in-memory Big Data technology and a user-friendly interface, App Orchid streamlines AI adoption through rapid deployment, low-cost implementation, and minimal disruption to existing systems.
Let’s start with the big picture—what does “agentic AI” mean to you, and how is it different from traditional AI systems?
Agentic AI represents a fundamental shift from the static execution typical of traditional AI systems to dynamic orchestration. To me, it’s about moving from rigid, pre-programmed systems to autonomous, adaptable problem-solvers that can reason, plan, and collaborate.
What truly sets agentic AI apart is its ability to leverage the distributed nature of knowledge and expertise. Traditional AI often operates within fixed boundaries, following predetermined paths. Agentic systems, however, can decompose complex tasks, identify the right specialized agents for sub-tasks—potentially discovering and leveraging them through agent registries—and orchestrate their interaction to synthesize a solution. This concept of agent registries allows organizations to effectively ‘rent’ specialized capabilities as needed, mirroring how human expert teams are assembled, rather than being forced to build or own every AI function internally.
So, instead of monolithic systems, the future lies in creating ecosystems where specialized agents can be dynamically composed and coordinated – much like a skilled project manager leading a team – to address complex and evolving business challenges effectively.
How is Google Agentspace accelerating the adoption of agentic AI across enterprises, and what’s App Orchid’s role in this ecosystem?
Google Agentspace is a significant accelerator for enterprise AI adoption. By providing a unified foundation to deploy and manage intelligent agents connected to various work applications, and leveraging Google’s powerful search and models like Gemini, Agentspace enables companies to transform siloed information into actionable intelligence through a common interface.
App Orchid acts as a vital semantic enablement layer within this ecosystem. While Agentspace provides the agent infrastructure and orchestration framework, our Easy Answers platform tackles the critical enterprise challenge of making complex data understandable and accessible to agents. We use an ontology-driven approach to build rich knowledge graphs from enterprise data, complete with business context and relationships – precisely the understanding agents need.
This creates a powerful synergy: Agentspace provides the robust agent infrastructure and orchestration capabilities, while App Orchid provides the deep semantic understanding of complex enterprise data that these agents require to operate effectively and deliver meaningful business insights. Our collaboration with the Google Cloud Cortex Framework is a prime example, helping customers drastically reduce data preparation time (up to 85%) while leveraging our platform’s industry-leading 99.8% text-to-SQL accuracy for natural language querying. Together, we empower organizations to deploy agentic AI solutions that truly grasp their business language and data intricacies, accelerating time-to-value.
What are real-world barriers companies face when adopting agentic AI, and how does App Orchid help them overcome these?
The primary barriers we see revolve around data quality, the challenge of evolving security standards – particularly ensuring agent-to-agent trust – and managing the distributed nature of enterprise knowledge and agent capabilities.
Data quality remains the bedrock issue. Agentic AI, like any AI, provides unreliable outputs if fed poor data. App Orchid tackles this foundationally by creating a semantic layer that contextualizes disparate data sources. Building on this, our unique crowdsourcing features within Easy Answers engage business users across the organization—those who understand the data’s meaning best—to collaboratively identify and address data gaps and inconsistencies, significantly improving reliability.
Security presents another critical hurdle, especially as agent-to-agent communication becomes common, potentially spanning internal and external systems. Establishing robust mechanisms for agent-to-agent trust and maintaining governance without stifling necessary interaction is key. Our platform focuses on implementing security frameworks designed for these dynamic interactions.
Finally, harnessing distributed knowledge and capabilities effectively requires advanced orchestration. App Orchid leverages concepts like the Model Context Protocol (MCP), which is increasingly pivotal. This enables the dynamic sourcing of specialized agents from repositories based on contextual needs, facilitating fluid, adaptable workflows rather than rigid, pre-defined processes. This approach aligns with emerging standards, such as Google’s Agent2Agent protocol, designed to standardize communication in multi-agent systems. We help organizations build trusted and effective agentic AI solutions by addressing these barriers.
Can you walk us through how Easy Answers™ works—from natural language query to insight generation?
Easy Answers transforms how users interact with enterprise data, making sophisticated analysis accessible through natural language. Here’s how it works:
Connectivity: We start by connecting to the enterprise’s data sources – we support over 200 common databases and systems. Crucially, this often happens without requiring data movement or replication, connecting securely to data where it resides.
Ontology Creation: Our platform automatically analyzes the connected data and builds a comprehensive knowledge graph. This structures the data into business-centric entities we call Managed Semantic Objects (MSOs), capturing the relationships between them.
Metadata Enrichment: This ontology is enriched with metadata. Users provide high-level descriptions, and our AI generates detailed descriptions for each MSO and its attributes (fields). This combined metadata provides deep context about the data’s meaning and structure.
Natural Language Query: A user asks a question in plain business language, like “Show me sales trends for product X in the western region compared to last quarter.”
Interpretation & SQL Generation: Our NLP engine uses the rich metadata in the knowledge graph to understand the user’s intent, identify the relevant MSOs and relationships, and translate the question into precise data queries (like SQL). We achieve an industry-leading 99.8% text-to-SQL accuracy here.
Insight Generation (Curations): The system retrieves the data and determines the most effective way to present the answer visually. In our platform, these interactive visualizations are called ‘curations’. Users can automatically generate or pre-configure them to align with specific needs or standards.
Deeper Analysis (Quick Insights): For more complex questions or proactive discovery, users can leverage Quick Insights. This feature allows them to easily apply ML algorithms shipped with the platform to specified data fields to automatically detect patterns, identify anomalies, or validate hypotheses without needing data science expertise.
This entire process, often completed in seconds, democratizes data access and analysis, turning complex data exploration into a simple conversation.
How does Easy Answers bridge siloed data in large enterprises and ensure insights are explainable and traceable?
Data silos are a major impediment in large enterprises. Easy Answers addresses this fundamental challenge through our unique semantic layer approach.
Instead of costly and complex physical data consolidation, we create a virtual semantic layer. Our platform builds a unified logical view by connecting to diverse data sources where they reside. This layer is powered by our knowledge graph technology, which maps data into Managed Semantic Objects (MSOs), defines their relationships, and enriches them with contextual metadata. This creates a common business language understandable by both humans and AI, effectively bridging technical data structures (tables, columns) with business meaning (customers, products, sales), regardless of where the data physically lives.
Ensuring insights are trustworthy requires both traceability and explainability:
Traceability: We provide comprehensive data lineage tracking. Users can drill down from any curations or insights back to the source data, viewing all applied transformations, filters, and calculations. This provides full transparency and auditability, crucial for validation and compliance.
Explainability: Insights are accompanied by natural language explanations. These summaries articulate what the data shows and why it’s significant in business terms, translating complex findings into actionable understanding for a broad audience.
This combination bridges silos by creating a unified semantic view and builds trust through clear traceability and explainability.
How does your system ensure transparency in insights, especially in regulated industries where data lineage is critical?
Transparency is absolutely non-negotiable for AI-driven insights, especially in regulated industries where auditability and defensibility are paramount. Our approach ensures transparency across three key dimensions:
Data Lineage: This is foundational. As mentioned, Easy Answers provides end-to-end data lineage tracking. Every insight, visualization, or number can be traced back meticulously through its entire lifecycle—from the original data sources, through any joins, transformations, aggregations, or filters applied—providing the verifiable data provenance required by regulators.
Methodology Visibility: We avoid the ‘black box’ problem. When analytical or ML models are used (e.g., via Quick Insights), the platform clearly documents the methodology employed, the parameters used, and relevant evaluation metrics. This ensures the ‘how’ behind the insight is as transparent as the ‘what’.
Natural Language Explanation: Translating technical outputs into understandable business context is crucial for transparency. Every insight is paired with plain-language explanations describing the findings, their significance, and potentially their limitations, ensuring clarity for all stakeholders, including compliance officers and auditors.
Furthermore, we incorporate additional governance features for industries with specific compliance needs like role-based access controls, approval workflows for certain actions or reports, and comprehensive audit logs tracking user activity and system operations. This multi-layered approach ensures insights are accurate, fully transparent, explainable, and defensible.
How is App Orchid turning AI-generated insights into action with features like Generative Actions?
Generating insights is valuable, but the real goal is driving business outcomes. With the correct data and context, an agentic ecosystem can drive actions to bridge the critical gap between insight discovery and tangible action, moving analytics from a passive reporting function to an active driver of improvement.
Here’s how it works: When the Easy Answers platform identifies a significant pattern, trend, anomaly, or opportunity through its analysis, it leverages AI to propose specific, contextually relevant actions that could be taken in response.
These aren’t vague suggestions; they are concrete recommendations. For instance, instead of just flagging customers at high risk of churn, it might recommend specific retention offers tailored to different segments, potentially calculating the expected impact or ROI, and even drafting communication templates. When generating these recommendations, the system considers business rules, constraints, historical data, and objectives.
Crucially, this maintains human oversight. Recommended actions are presented to the appropriate users for review, modification, approval, or rejection. This ensures business judgment remains central to the decision-making process while AI handles the heavy lifting of identifying opportunities and formulating potential responses.
Once an action is approved, we can trigger an agentic flow for seamless execution through integrations with operational systems. This could mean triggering a workflow in a CRM, updating a forecast in an ERP system, launching a targeted marketing task, or initiating another relevant business process – thus closing the loop from insight directly to outcome.
How are knowledge graphs and semantic data models central to your platform’s success?
Knowledge graphs and semantic data models are the absolute core of the Easy Answers platform; they elevate it beyond traditional BI tools that often treat data as disconnected tables and columns devoid of real-world business context. Our platform uses them to build an intelligent semantic layer over enterprise data.
This semantic foundation is central to our success for several key reasons:
Enables True Natural Language Interaction: The semantic model, structured as a knowledge graph with Managed Semantic Objects (MSOs), properties, and defined relationships, acts as a ‘Rosetta Stone’. It translates the nuances of human language and business terminology into the precise queries needed to retrieve data, allowing users to ask questions naturally without knowing underlying schemas. This is key to our high text-to-SQL accuracy.
Preserves Critical Business Context: Unlike simple relational joins, our knowledge graph explicitly captures the rich, complex web of relationships between business entities (e.g., how customers interact with products through support tickets and purchase orders). This allows for deeper, more contextual analysis reflecting how the business operates.
Provides Adaptability and Scalability: Semantic models are more flexible than rigid schemas. As business needs evolve or new data sources are added, the knowledge graph can be extended and modified incrementally without requiring a complete overhaul, maintaining consistency while adapting to change.
This deep understanding of data context provided by our semantic layer is fundamental to everything Easy Answers does, from basic Q&A to advanced pattern detection with Quick Insights, and it forms the essential foundation for our future agentic AI capabilities, ensuring agents can reason over data meaningfully.
What foundational models do you support, and how do you allow organizations to bring their own AI/ML models into the workflow?
We believe in an open and flexible approach, recognizing the rapid evolution of AI and respecting organizations’ existing investments.
For foundational models, we maintain integrations with leading options from multiple providers, including Google’s Gemini family, OpenAI’s GPT models, and prominent open-source alternatives like Llama. This allows organizations to choose models that best fit their performance, cost, governance, or specific capability needs. These models power various platform features, including natural language understanding for queries, SQL generation, insight summarization, and metadata generation.
Beyond these, we provide robust pathways for organizations to bring their own custom AI/ML models into the Easy Answers workflow:
Models developed in Python can often be integrated directly via our AI Engine.
We offer seamless integration capabilities with major cloud ML platforms such as Google Vertex AI and Amazon SageMaker, allowing models trained and hosted there to be invoked.
Critically, our semantic layer plays a key role in making these potentially complex custom models accessible. By linking model inputs and outputs to the business concepts defined in our knowledge graph (MSOs and properties), we allow non-technical business users to leverage advanced predictive, classification or causal models (e.g., through Quick Insights) without needing to understand the underlying data science – they interact with familiar business terms, and the platform handles the technical translation. This truly democratizes access to sophisticated AI/ML capabilities.
Looking ahead, what trends do you see shaping the next wave of enterprise AI—particularly in agent marketplaces and no-code agent design?
The next wave of enterprise AI is moving towards highly dynamic, composable, and collaborative ecosystems. Several converging trends are driving this:
Agent Marketplaces and Registries: We’ll see a significant rise in agent marketplaces functioning alongside internal agent registries. This facilitates a shift from monolithic builds to a ‘rent and compose’ model, where organizations can dynamically discover and integrate specialized agents—internal or external—with specific capabilities as needed, dramatically accelerating solution deployment.
Standardized Agent Communication: For these ecosystems to function, agents need common languages. Standardized agent-to-agent communication protocols, such as MCP (Model Context Protocol), which we leverage, and initiatives like Google’s Agent2Agent protocol, are becoming essential for enabling seamless collaboration, context sharing, and task delegation between agents, regardless of who built them or where they run.
Dynamic Orchestration: Static, pre-defined workflows will give way to dynamic orchestration. Intelligent orchestration layers will select, configure, and coordinate agents at runtime based on the specific problem context, leading to far more adaptable and resilient systems.
No-Code/Low-Code Agent Design: Democratization will extend to agent creation. No-code and low-code platforms will empower business experts, not just AI specialists, to design and build agents that encapsulate specific domain knowledge and business logic, further enriching the pool of available specialized capabilities.
App Orchid’s role is providing the critical semantic foundation for this future. For agents in these dynamic ecosystems to collaborate effectively and perform meaningful tasks, they need to understand the enterprise data. Our knowledge graph and semantic layer provide exactly that contextual understanding, enabling agents to reason and act upon data in relevant business terms.
How do you envision the role of the CTO evolving in a future where decision intelligence is democratized through agentic AI?
The democratization of decision intelligence via agentic AI fundamentally elevates the role of the CTO. It shifts from being primarily a steward of technology infrastructure to becoming a strategic orchestrator of organizational intelligence.
Key evolutions include:
From Systems Manager to Ecosystem Architect: The focus moves beyond managing siloed applications to designing, curating, and governing dynamic ecosystems of interacting agents, data sources, and analytical capabilities. This involves leveraging agent marketplaces and registries effectively.
Data Strategy as Core Business Strategy: Ensuring data is not just available but semantically rich, reliable, and accessible becomes paramount. The CTO will be central in building the knowledge graph foundation that powers intelligent systems across the enterprise.
Evolving Governance Paradigms: New governance models will be needed for agentic AI – addressing agent trust, security, ethical AI use, auditability of automated decisions, and managing emergent behaviors within agent collaborations.
Championing Adaptability: The CTO will be crucial in embedding adaptability into the organization’s technical and operational fabric, creating environments where AI-driven insights lead to rapid responses and continuous learning.
Fostering Human-AI Collaboration: A key aspect will be cultivating a culture and designing systems where humans and AI agents work synergistically, augmenting each other’s strengths.
Ultimately, the CTO becomes less about managing IT costs and more about maximizing the organization’s ‘intelligence potential’. It’s a shift towards being a true strategic partner, enabling the entire business to operate more intelligently and adaptively in an increasingly complex world.
Thank you for the great interview, readers who wish to learn more should visit App Orchid.
#adoption#agent#Agentic AI#agents#ai#AI adoption#AI AGENTS#AI systems#AI-powered#AI/ML#Algorithms#Amazon#amp#Analysis#Analytics#anomalies#anomaly#app#App Orchid#applications#approach#attributes#audit#autonomous#bedrock#bi#bi tools#Big Data#black box#box
0 notes
Text
Inteligencia Artificial: El Motor de la Nueva Era del Análisis de Datos
Introducción: De Datos Crudos a Decisiones Inteligentes Gracias a la IA Vivimos en una era de información sin precedentes. Las organizaciones generan y recopilan volúmenes masivos de datos cada segundo. Sin embargo, estos datos son solo potencial en bruto. El verdadero valor reside en la capacidad de analizarlos para extraer conocimientos, identificar patrones, predecir tendencias y, en última…
#Alteryx#Análisis de Datos#Analítica Aumentada#Analítica Predictiva#AutoML#Azure ML#BI#Big Data#Business Intelligence#Ciencia de Datos#Data Mining#Domo#Google#IA#IBM#IBM Watson#inteligencia artificial#Looker#machine learning#Microsoft#MicroStrategy#Oracle#Oracle Analytics#Palantir Technologies#Power BI#Qlik#RapidMiner#SAP#SAP Analytics Cloud#SAS Institute
0 notes
Text
🚀 Become a Data Analyst with Power BI!
Looking to enhance your data analytics skills? Power BI is a must-have tool for creating interactive dashboards, visualizations, and reports. Learn essential DAX functions, data modeling, and real-world applications to advance your career in business intelligence!
🔗 Read More: https://exploringpythonfromscratch.blogspot.com/2025/02/data-analyst-using-power-bi-skills.html
#PowerBI #DataAnalytics #BusinessIntelligence #DataVisualization #TechSkills #BigData #MicrosoftPowerBI #DataScience #CareerGrowth
#powerbi#data analytics#datascience#big data#technology#microsoft power bi#data visualization#careergrowth
0 notes
Text
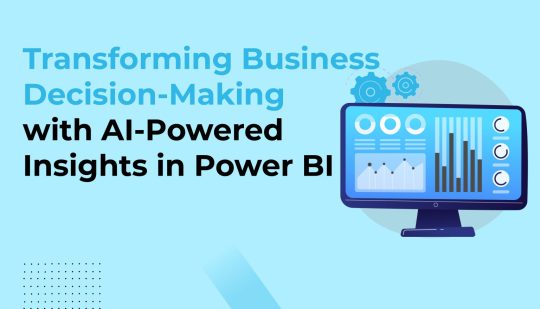
In today’s fast-evolving digital landscape, businesses seek tools that facilitate quick decisions and offer actionable insights. Integrating Power BI Solutions has emerged as a powerful way to analyze data, create interactive reports, and visualize critical metrics.
When combined with AI capabilities, Power BI Solutions redefines how organizations interact with their data and drive more informed strategies. This article explores how AI-powered insights and Advanced analytics solutions transform business decision-making processes in Power BI.
0 notes
Text
Unlock the Power of Data Analytics with Power BI and SSRS
In today's data-driven landscape, making informed business decisions is essential. At Klusterfirst, we specialize in Data Analytics using Power BI and SQL Server Reporting Services (SSRS), delivering actionable insights that propel your business forward.
Why Choose Power BI?
User-Friendly Interface: Easily create reports with intuitive visualizations, no extensive technical expertise required.
Real-Time Insights: Connect to multiple data sources and receive real-time analytics for timely decision-making.
Interactive Dashboards: Engage users with dynamic dashboards that promote deeper exploration of your data.
Why SSRS?
Robust Reporting Capabilities: Generate versatile reports in various formats to effectively communicate critical information.
Customizable Reports: Tailor reports to meet specific business needs, ensuring clarity for stakeholders.
Seamless SQL Integration: Benefit from effortless integration with SQL Server for comprehensive reporting solutions.
Our Services
At Klusterfirst, we provide end-to-end Data Analytics services:
Data Visualization: Transform complex data into visually appealing Power BI reports and dashboards.
Report Development: Create interactive and customized reports with SSRS tailored to your business needs.
Training and Support: Equip your team with the skills to leverage Power BI and SSRS effectively.
Get Started Today!
Don't let your data go untapped. Unlock insights that drive strategic decisions with Power BI and SSRS. Visit us at Klusterfirst Data Analytics to learn more about our services and how we can help you succeed.
1 note
·
View note
Text

Data isn't just numbers, it's your roadmap to success.
Navigate it wisely with Bicxo!
For free demo visit: www.bicxo.co
#business solutions#business intelligence#business intelligence software#bi tool#businessintelligence#bisolution#bicxo#data#businessefficiency#data warehouse#mumbai#india#big data
0 notes
Text
#data visualization#data analytics#datainsights#businessintelligence#business#business analytics#businessstrategy#advance data solution#business analysis techniques#data strategy#big data#bi solutions#business intelligence and data analytics#business intelligence and analytics
0 notes
Text
Business Intelligence vs Business Analytics
Summary: While both BI and BA leverage data, BI focuses on understanding current performance through reports and dashboards. BA dives deeper, using statistical models to predict future trends and optimize decision-making for growth.

Introduction
In today's data-driven world, businesses are constantly bombarded with information. But how do you turn this data into actionable insights that drive growth and success? This is where Business Intelligence (BI) and Business Analytics (BA) come in.
While these terms are often used interchangeably, there are key differences between them. Understanding these distinctions is crucial for businesses looking to leverage their data effectively.
Defining Business Intelligence (BI)
Business Intelligence (BI) can be thought of as the foundation for data-driven decision making. It's a set of methodologies, architectures, and tools that enable businesses to gather, store, access, and analyze data to gain a comprehensive understanding of their operations.
BI focuses on presenting historical and present data in an easily digestible format, allowing users to answer questions like:
What are our top-selling products?
What are our current sales trends?
How are our marketing campaigns performing?
Key Components of BI
Data Warehousing: A central repository that stores historical data from various sources within an organization.
Data Extraction, Transformation, and Loading (ETL): The process of extracting data from various sources, transforming it into a consistent format, and loading it into the data warehouse.
Data Analysis and Reporting Tools: Software applications that allow users to query the data,
create reports, and visualize trends.
Dashboards and Scorecards: Real-time or near-real-time visual representations of key metrics and performance indicators.
Primary Functions of BI
Data Visualization: BI tools translate complex data sets into easy-to-understand charts, graphs, and dashboards. This allows users to identify trends, patterns, and outliers quickly.
Reporting and Analysis: BI enables users to generate reports that answer specific business questions. These reports can be static or interactive, allowing for deeper analysis.
Performance Monitoring: BI helps businesses track key performance indicators (KPIs) to measure progress towards goals and identify areas for improvement.
Improved Decision Making: By providing clear and concise insights into business operations, BI empowers users to make informed decisions based on data, not gut feeling.
Defining Business Analytics (BA)
Business Analytics (BA) builds upon the foundation of BI. It's a more advanced practice that uses statistical models and techniques to uncover hidden patterns, trends, and relationships within data. BA goes beyond simply describing what's happening to answer the "why" and "what if" questions. It helps businesses:
Predict future outcomes
Identify potential risks and opportunities
Develop data-driven strategies for growth
Optimize processes and resource allocation
Key Components of BA
By combining the following key components, Business Analytics empowers you to go beyond simply understanding what's happening to uncover the "why" and "what if" questions hidden within your data. Here is the breakdown of the same:
Statistical Modeling
This component focuses on applying statistical techniques to data to identify patterns, relationships, and trends. Statistical models can be used for various purposes in BA, such as:
Regression Analysis: This technique helps understand the relationship between one dependent variable (what you're trying to predict) and one or more independent variables (factors that influence the dependent variable). It allows you to estimate how much a change in the independent variable will affect the dependent variable.
Time Series Analysis: This technique focuses on analyzing data collected over time to forecast future trends and seasonality. For example, a company might use time series analysis to predict future sales based on historical sales data.
Hypothesis Testing: This technique helps determine if a particular hypothesis about a population is likely to be true based on sample data. For instance, a marketing team might use hypothesis testing to determine if a new ad campaign has a statistically significant impact on sales.
Data Mining
Data mining involves extracting hidden patterns and knowledge from large datasets that might not be readily apparent through traditional analysis techniques. Here are some common data mining techniques used in BA:
Classification: This technique categorizes data points into predefined groups based on their characteristics. An e-commerce company might use classification to segment customers based on their purchase history.
Clustering: This technique groups data points together based on their similarities, helping uncover hidden patterns and relationships within the data. For example, a bank might use clustering to identify groups of customers with similar financial risk profiles.
Association Rule Learning: This technique discovers relationships between different variables within a dataset. A grocery store might use association rule learning to identify products that are frequently bought together, allowing them to optimize product placement.
Machine Learning (ML)
Machine Learning algorithms learn from data without being explicitly programmed. These algorithms can then be used to make predictions about future events or customer behaviour. Commonly used ML techniques in BA include:
Decision Trees: These algorithms create tree-like structures that classify data based on a series of sequential questions.
Support Vector Machines (SVMs): These algorithms create hyperplanes that separate data points into distinct categories.
Neural Networks: Inspired by the human brain, these complex algorithms learn from data and can identify complex patterns and relationships.
Data Visualization
While data visualization is a key component of BI as well, BA often utilizes more advanced visualization techniques to present complex data insights effectively. These techniques include:
Interactive Dashboards: These dynamic dashboards allow users to drill down into data and explore different dimensions, providing a deeper understanding of the information.
Predictive Modeling Visualization: Techniques like heatmaps and treemaps help visualize the relationships between variables and the impact of different factors on the predicted outcome.
Primary Functions of BA
By leveraging statistical modelling and machine learning techniques, BA can transform historical data into a crystal ball, providing businesses with invaluable insights into what lies ahead. Let's delve deeper into the world of predictive analytics and explore how it empowers businesses to make data-driven decisions for a more successful future.
Predictive Analytics
BA uses historical data to forecast future trends, allowing businesses to anticipate changes in the market or customer behavior. For instance, a retail company might use BA to predict demand for specific products during the holiday season.
Prescriptive Analytics
It goes beyond simple prediction to suggest optimal courses of action based on data analysis. Imagine a company analyzing customer churn data to identify factors that contribute to customer dissatisfaction. BA can then recommend strategies to address these factors and retain customers.
Risk Assessment
BA helps businesses identify and mitigate potential risks by analyzing historical data and industry trends. An insurance company might use BA to assess the risk of policyholders filing claims.
Customer Segmentation
Business Analytics helps businesses segment their customer base into distinct groups with similar characteristics and behaviors. This allows for targeted marketing campaigns and improved customer satisfaction. For example, a clothing retailer might segment its customers by age, gender, and purchase history to create targeted email campaigns.
Key Differences Between BI and BA
Choosing Between BI and BA
In many cases, BI and BA work best when used together. BI provides the foundation for understanding your current state, while BA helps you leverage that understanding to make better decisions about the future. The choice between BI and BA depends on your specific needs. Here's a breakdown to help you decide:
Need BI if:
You need a clear picture of your current performance.
You want to track key metrics and identify trends.
You want to empower business users with self-service analytics.
You have a large volume of data that needs to be organized and easily accessible.
Need BA if:
You want to predict future outcomes and make data-driven forecasts.
You want to identify root causes of problems and optimize processes.
You want to develop data-driven strategies for growth and innovation.
You have a team of data analysts or data scientists who can leverage advanced statistical techniques.
Here's an analogy: Imagine BI as the rearview mirror of your car, giving you a clear view of where you've been. BA is like the windshield, helping you see where you're going and navigate the road ahead.
Conclusion
In today's data-driven world, both BI and BA are essential tools for businesses of all sizes. By understanding the key differences between these two disciplines, you can make informed decisions about how to leverage your data to gain a competitive advantage.
BI empowers you to understand your current situation, while BA equips you to predict future trends and make data-driven decisions for growth and success. Remember, BI is the foundation, and BA is the strategy builder. Together, they form a powerful combination for unlocking the true potential of your data.
Frequently Asked Questions
What is the Difference Between BI and BA?
BI provides a rearview mirror view of your business, analyzing past and present data. BA acts like a windshield, using data to predict future trends and make data-driven decisions.
When Should I Use BI?
Use BI if you need clear insights into current performance, want to track key metrics, or empower business users to analyze data.
When Should I Use BA?
Use BA if you want to predict future trends, identify root causes of problems, or develop data-driven strategies for growth and innovation.
#business intelligence vs business analytics#business analytics#data analytics#business intelligence#business#power bi#bi#ba#bi vs ba#big data analytics#data science#pickl.ai#data analyst
1 note
·
View note
Text
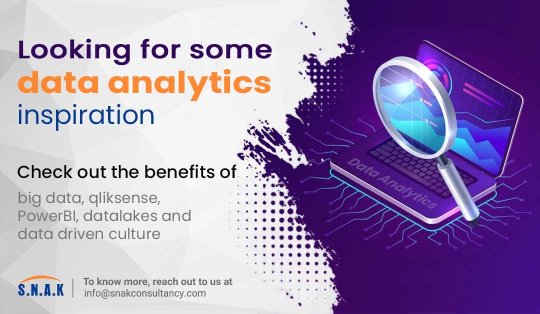
Best Data Analytics Solutions provider company with Big Data and Migration, Snowflake and Power BI Solution- SCS
0 notes
Text
BI Market Explodes as India Inc. Analyzes Deeper
APAC News Network analyzes the Indian BI analytics market is poised for an explosive growth as Indian enterprises increasingly look at data driven insights to grow their businesses.
Rajneesh De, Consulting Editor, APAC News Network
The big data analytics market in India, just like globally, is marching at a fast pace. Enterprises today value information as a critical business asset. Big Data analytics has therefore emerged as an essential competency for enterprises today. Enterprises in India are today continuously striving to respond in constantly shifting business situations. This has led to creation of a modern enterprise data ecosystem so that data is up-to-date and the enterprise is ready to make informed decisions.
Adapting to major ongoing trends in analytics will enable enterprises to better react and pre-act. Data and analytics have become the key ingredients for this breakthrough. The direct corollary to this has been the growth of the analytics and BI market in India. These enterprises are currently employing big data and analytics as an advantageous practice to gain a competitive edge over their industry competitors by putting the data to the right use.
In India, CIOs, CDOs, and data and analytics leaders are increasingly focusing on business outcomes, exploring algorithmic business, and most importantly building trust with the business and external partners. In particular, they are experimenting and adopting smart data discovery, augmented analytics, in-memory computing and data virtualization to stay ahead of the curve.
Read More : - https://apacnewsnetwork.com/2023/11/bi-market-explodes-as-india-inc-analyzes-deeper/
#analytics workforce in India#Analyzes Deeper#APAC Exclusive#augmented analytics#AWSBFSI continues#BI Market#BI Market Explodes as India Inc. Analyzes Deeper#BI market in India#BI market India#big data analytics market in India#Big Data Trends#Delhi NCR regionglobal BI market#IBM#IBM Watson Analytics and Cognos BI#India In#cIndian analytics BI market#Indian BI#Indian BI analytics market#Indian BI and Big Data Trends#Indian BI Market Numbers#Indian BI sector deeply#Indian currency#Salesforce#SAPSAS#smart data discovery
0 notes
Text
In a world overwhelmed by Excel sheets, PolusAI revolutionizes data management by automating preparation, handling dynamic formats, and enhancing data quality. It transforms data into actionable insights, giving businesses a competitive edge. PolusAI represents the future of analytics, enabling effortless creation of dashboards from extensive data sources.
#etl#newfangled#polusai#data democratization#business dashboard#big data#nlp#business data#ai to generate dashboard#bi report#conversationaletl
0 notes
Text
Scope of A.I
If you are interested in a career in AI, there are a number of things you can do to prepare. You can start by taking courses in computer science, data science, and machine learning. You can also gain experience by working on AI projects or internships. And finally, you can network with other professionals in the field.The most in demand job profilesin AI are
Robotics Scientist: Robotics scientists are responsible for designing, developing, and testing robots. They use their knowledge of AI, computer science, and engineering to create robots that can perform a variety of tasks, such as manufacturing, healthcare, and transportation.
Big Data Engineer: Big data engineers are responsible for collecting, storing, and processing large datasets. They use their knowledge of data science, cloud computing, and software engineering to build and maintain big data systems.
BI Developer: BI developers are responsible for creating and maintaining business intelligence (BI) solutions. They use their knowledge of data analysis, visualization, and reporting to help businesses make better decisions.
Data Scientist: Data scientists are responsible for collecting, cleaning, and analyzing data. They use their knowledge of statistics, machine learning, and programming to extract insights from data and help businesses make better decisions.
Machine Learning Engineer: Machine learning engineers are responsible for designing, developing, and deploying machine learning models. They use their knowledge of statistics, machine learning, and programming to build models that can learn from data and make predictions. The demand for AI skills is expected to continue to grow in the coming years. As a result, those who are skilled in AI will be in high demand in the workforce. Some of the reputed platforms that offers courses in A.I are Coursera, Udemy, edX, DigiCrome Academy, Next Hikes
If you like my blog, don't forget to subscribe and like.
#artificial intelligence#programming#data scientist#machine learning#data science#mobile app development#software development#web development#my writing#jobs in education#digicrome#nexthikes#engineering#power bi#data analysis#tableau#robotics#robotification#big data analytics#big data management#science#science fiction#science communication#science news#science fantasy#scientists
0 notes