#Data Ingestion
Explore tagged Tumblr posts
Text
Prescriptive AI: The Smart Decision-Maker for Healthcare, Logistics, and Beyond
New Post has been published on https://thedigitalinsider.com/prescriptive-ai-the-smart-decision-maker-for-healthcare-logistics-and-beyond/
Prescriptive AI: The Smart Decision-Maker for Healthcare, Logistics, and Beyond
Artificial Intelligence (AI) has made significant progress in recent years, transforming how organizations manage complex data and make decisions. With the vast amount of data available, many industries face the critical challenge of acting on real-time insights. This is where prescriptive AI steps in. Unlike traditional predictive models, which simply forecast outcomes based on past data, prescriptive AI recommends specific actions to achieve optimal results. By predicting and suggesting, prescriptive AI is proving essential across industries such as healthcare, logistics, finance, and retail, where even minor delays or inefficiencies can have substantial impacts.
In healthcare, prescriptive AI can recommend effective treatment plans based on real-time data, potentially saving lives. In logistics, it instantly optimizes delivery routes, reducing costs and enhancing customer satisfaction. With its ability to turn data into precise, actionable steps, prescriptive AI redefines the possibilities across industries and sets a new standard for responsive, data-driven decision-making.
How Prescriptive AI Transforms Data into Actionable Strategies
Prescriptive AI goes beyond simply analyzing data; it recommends actions based on that data. While descriptive AI looks at past information and predictive AI forecasts what might happen, prescriptive AI takes it further. It combines these insights with optimization tools to suggest specific steps a business should take. For instance, if a predictive model shows a likely increase in product demand, prescriptive AI can recommend increasing inventory or adjusting supply chains to meet that demand.
Prescriptive AI uses machine learning and optimization models to evaluate various scenarios, assess outcomes, and find the best path forward. This capability is essential for fast-paced industries, helping businesses make quick, data-driven decisions, often with automation. By using structured, unstructured, and real-time data, prescriptive AI enables smarter, more proactive decision-making.
A major strength of prescriptive AI is its ability to keep learning and adapting. As it processes more data, the system refines its recommendations, making them more accurate. This helps businesses remain competitive and improve their strategies based on fresh data and trends.
Moreover, prescriptive AI integrates well with existing systems, enhancing their capabilities without major changes. Its modular design can be tailored to fit specific business needs, offering flexibility and scalability.
What Powers Prescriptive AI?
Prescriptive AI relies on several essential components that work together to turn raw data into actionable recommendations. Each plays a unique role in delivering accurate and context-aware insights.
The process begins with data ingestion and preprocessing, where prescriptive AI gathers information from different sources, such as IoT sensors, databases, and customer feedback. It organizes it by filtering out irrelevant details and ensuring data quality. This step is essential because the accuracy of any recommendation depends on the clarity and reliability of the initial data. Clean and relevant data means that prescriptive AI can make trustworthy and precise recommendations.
Once the data is ready, prescriptive AI moves into predictive modeling, using machine learning algorithms to analyze past patterns and predict future trends and behaviors. These predictions are the backbone of prescriptive AI, as they help anticipate what may happen based on current and historical data. For example, predictive models in healthcare might assess a patient’s medical history and lifestyle factors to forecast potential health risks, allowing prescriptive AI to recommend proactive steps to improve health outcomes.
The next key component, optimization algorithms, is where prescriptive AI performs well. While predictive models offer a glimpse into the future, optimization algorithms evaluate numerous potential actions to determine which is likely to produce the best outcome while factoring in real-world constraints like time, cost, and resource availability. For example, in logistics, these algorithms can analyze real-time traffic and weather conditions to determine the fastest and most fuel-efficient route for delivery vehicles, improving both cost-effectiveness and timeliness.
Prescriptive AI systems are sometimes designed to go one step further with automated decision execution. This capability allows the system to act on its recommendations independently, reducing or even eliminating the need for human intervention. This is particularly valuable in industries where speed is critical. In finance, for instance, prescriptive AI can be set up to adjust an investment portfolio in response to market changes rapidly. Cybersecurity can automatically take defensive measures when a potential threat is detected. This automation allows businesses to respond quickly to changing circumstances, protect assets, minimize losses, and optimize operations in real-time.
Why Industries Are Adopting Prescriptive AI
Prescriptive AI offers numerous advantages that make it highly appealing to various industries. One of the most significant benefits is its ability to accelerate decision-making in environments like stock trading or emergency response, where every second counts. Prescriptive AI enables organizations to act quickly and effectively, bypassing the need for lengthy data analysis.
Another advantage is the improvement in operational efficiency. Prescriptive AI systems can automate repetitive decision-making tasks, allowing human resources to focus on more strategic work. For instance, in logistics, prescriptive AI can autonomously adjust delivery schedules, manage inventory levels, and optimize routing in response to changing conditions. This not only reduces costs but also boosts productivity.
Lastly, prescriptive AI enhances accuracy and scalability. Unlike human decision-makers, prescriptive AI can process massive datasets with high precision, identifying patterns and correlations that might otherwise be overlooked. This ability to operate at scale and deliver consistent results makes prescriptive AI ideal for sectors that handle vast amounts of data, such as e-commerce and healthcare.
Industries are turning to prescriptive AI to gain these critical advantages, preparing themselves to act faster, work more efficiently, and make highly informed decisions based on comprehensive data analysis.
Opportunities and Challenges in Deploying Prescriptive AI
Prescriptive AI offers significant advantages, yet its deployment brings challenges and ethical considerations. Data privacy and security are primary concerns, particularly in sectors like healthcare and finance, where sensitive information must be carefully managed. Ensuring secure data collection and processing is crucial to maintaining public trust.
Another key issue is bias within AI algorithms. When trained on biased datasets, prescriptive AI may produce unfair recommendations, especially in areas like hiring or loan approvals. Addressing these biases requires rigorous testing and validation to ensure fairness and equity in AI-driven decisions.
Technical integration can also be challenging. Many organizations operate with legacy systems that may not be compatible with the latest AI technologies, leading to potentially costly upgrades or complex integrations. Additionally, transparency and accountability are essential as prescriptive AI becomes more autonomous. Establishing mechanisms that can explain and justify AI decisions is important.
Looking ahead, several trends can enhance prescriptive AI’s future capabilities. One promising development is the rise of autonomous decision-making systems with minimal human involvement. For example, in manufacturing, machines with prescriptive AI could adjust operations in real-time to optimize efficiency.
Another exciting trend is the integration of prescriptive AI with the IoT. By processing data from connected devices in real time, AI can effectively manage complex environments such as smart cities, industrial facilities, and supply chains. This integration holds the potential to significantly improve the efficiency and responsiveness of these systems.
In addition, computing power and algorithm developments are expected to boost prescriptive AI’s speed and accuracy, making it accessible to a wider range of businesses. More affordable and adaptable AI solutions will allow small and medium-sized enterprises to benefit from prescriptive AI, helping them gain a competitive edge.
As these developments progress, prescriptive AI will likely play a more central role across various industries. Intelligent, real-time decision-making can enhance operational efficiency and enable businesses to respond quickly to changing circumstances. However, it is essential to balance innovation with responsibility and ensure that AI deployment remains transparent, accountable, and aligned with ethical standards.
The Bottom Line
Prescriptive AI reshapes industries by turning vast data into smart, actionable decisions. From healthcare to logistics and beyond, it is helping organizations respond to real-time demands, optimize operations, and make informed choices quickly. By integrating with existing systems and through powerful optimization algorithms, prescriptive AI provides businesses with a competitive edge in today’s fast-paced world.
Yet, as adoption grows, so do data privacy, fairness, and transparency responsibilities. Balancing these considerations with the high potential of prescriptive AI is essential to ensure that this technology not only drives efficiency but does so in a way that is ethical and sustainable for the future.
#actionable data strategies#adoption#ai#AI systems#algorithm#Algorithms#Analysis#artificial#Artificial Intelligence#assets#automation#autonomous#Bias#Business#challenge#cities#Commerce#comprehensive#computing#connected devices#cybersecurity#data#data analysis#data collection#data ingestion#data privacy#data privacy and security#data quality#data-driven#data-driven decisions
0 notes
Text
Unlock Powerful Data Strategies: Master Managed and External Tables in Fabric Delta Lake
Are you ready to unlock powerful data strategies and take your data management skills to the next level? In our latest blog post, we dive deep into mastering managed and external tables in Delta Lake within Microsoft Fabric.
Welcome to our series on optimizing data ingestion with Spark in Microsoft Fabric. In our first post, we covered the capabilities of Microsoft Fabric and its integration with Delta Lake. In this second installment, we dive into mastering Managed and External tables. Choosing between managed and external tables is a crucial decision when working with Delta Lake in Microsoft Fabric. Each option…
#Apache Spark#Big Data#Cloud Data Management#Data Analytics#Data Best Practices#Data Efficiency#Data Governance#Data Ingestion#Data Insights#Data management#Data Optimization#Data Strategies#Data Workflows#Delta Lake#External Tables#Managed Tables#microsoft azure#Microsoft Fabric#Real-Time Data
0 notes
Text

Data ingestion is core to any data refinement procedure that targets revealing hidden data insights. Right from collecting data to bringing it to the insightful revelation stage is a work of art. This is what data ingestion deals with.
Making it indispensable to your business processes shall yield greater results in the long-term future. Facilitating enhanced data analytics quality, trusted data-driven decision-making, and leveraging flexibility are all the perks that your organization can gain. Therefore, understanding the different types of data ingestion, and how they perform in real-time is a hard nut to crack.
Making it easier for you, there are popular and globally trusted data science certifications that can enhance your comprehension of these key concepts. These are streamed to prepare you for the organizational big data handling ahead.
There is a massive demand for skilled and certified data science professionals with the requisite knowledge of data ingestion tools worldwide. In the years as we advance through 2026, there will be 11.5 million jobs created for certified data scientists (The US Bureau of Labor Statistics). Make yourself a quick pick in the global career field that commands high respect for skills, and expertise, and offers a whopper of a salary internationally.
Building a thriving career progression with these skills and credentials gracing your portfolio for your dream data science job role with your preferred industry giant. Master data ingestion with USDSI® Data Science certifications today!
0 notes
Text
.
#arghh#they've got me sorting out the new log management servers#the old one we have the licence runs out on Monday#which a) means we can no longer make jokes about the name#and b) means I have to figure how to set up the new one and ingest the data#and some of that data Apparently we legally have to collect and send off?#i don't know#all I know is that I have this afternoon off and therefore probably not enough time#:(#oh well
3 notes
·
View notes
Text
Need to know if every single era had those incredibly annoying contrarians who, when you point out a fairly obvious recent issue start "not everything is because of 1 thing"-ing you.
Like idk bro if you were previously a healthy 20 year old and after a week long viral infection you can't stand without getting palpations yes statistically it's likely it was covid and now you've got long covid.
If you live on a mountain outside of the tropics and got hit with a fucking hurricane out of nowhere it's likely climate change and not some random freak event that would have happened if we were all using solar panels.
It's just exhausting to deal with. If i was in the middle of the eruption of Mt Vesuvius and someone was like "um akshually this isn't strange at all and can be explained by something else" i think I'd have shoved them into the lava myself.
#the vaping people irritate me the most on this i think#we actually have data on what nicotine ingestion can do to the body over long periods of time. its called cigarettes#so no vapes are not the largest cause of the increasing health issues many ppl are experiencing#especially because vaping is kind of concentrated among younger ppl
3 notes
·
View notes
Text
google’s generative ai search results have started showing up in Firefox and I hate it I hate it I hate it i hate it I hate it I HATE IT
#it’s in beta right now why can’t I figure out how to opt out I WANT to opt out#wow!! Thanks for citing three Reddit threads and a twitter post for this historical event I’m sure this is 100%#accurate and contains no hallucinated information you absolute noodles#“Google does the searching for you!” Fuck a duck man give me back my goddamn parameter searching!!!#BASIC information theory is “garbage in/garbage out”—you use shitty data you get shitty information#Trawl through the entire internet as your training pool and you’re ingesting an entire landfill#GOD okay I am aware I am tired and cranky mad but I am also justified#whispers from the ally
2 notes
·
View notes
Text
At the California Institute of the Arts, it all started with a videoconference between the registrar’s office and a nonprofit.
One of the nonprofit’s representatives had enabled an AI note-taking tool from Read AI. At the end of the meeting, it emailed a summary to all attendees, said Allan Chen, the institute’s chief technology officer. They could have a copy of the notes, if they wanted — they just needed to create their own account.
Next thing Chen knew, Read AI’s bot had popped up inabout a dozen of his meetings over a one-week span. It was in one-on-one check-ins. Project meetings. “Everything.”
The spread “was very aggressive,” recalled Chen, who also serves as vice president for institute technology. And it “took us by surprise.”
The scenariounderscores a growing challenge for colleges: Tech adoption and experimentation among students, faculty, and staff — especially as it pertains to AI — are outpacing institutions’ governance of these technologies and may even violate their data-privacy and security policies.
That has been the case with note-taking tools from companies including Read AI, Otter.ai, and Fireflies.ai.They can integrate with platforms like Zoom, Google Meet, and Microsoft Teamsto provide live transcriptions, meeting summaries, audio and video recordings, and other services.
Higher-ed interest in these products isn’t surprising.For those bogged down with virtual rendezvouses, a tool that can ingest long, winding conversations and spit outkey takeaways and action items is alluring. These services can also aid people with disabilities, including those who are deaf.
But the tools can quickly propagate unchecked across a university. They can auto-join any virtual meetings on a user’s calendar — even if that person is not in attendance. And that’s a concern, administrators say, if it means third-party productsthat an institution hasn’t reviewedmay be capturing and analyzing personal information, proprietary material, or confidential communications.
“What keeps me up at night is the ability for individual users to do things that are very powerful, but they don’t realize what they’re doing,” Chen said. “You may not realize you’re opening a can of worms.“
The Chronicle documented both individual and universitywide instances of this trend. At Tidewater Community College, in Virginia, Heather Brown, an instructional designer, unwittingly gave Otter.ai’s tool access to her calendar, and it joined a Faculty Senate meeting she didn’t end up attending. “One of our [associate vice presidents] reached out to inform me,” she wrote in a message. “I was mortified!”
24K notes
·
View notes
Text
Real-Time Data Ingestion: Strategies, Benefits, and Use Cases
Summary: Master real-time data! This guide explores key concepts & strategies for ingesting & processing data streams. Uncover the benefits like improved decision-making & fraud detection. Learn best practices & discover use cases across industries.
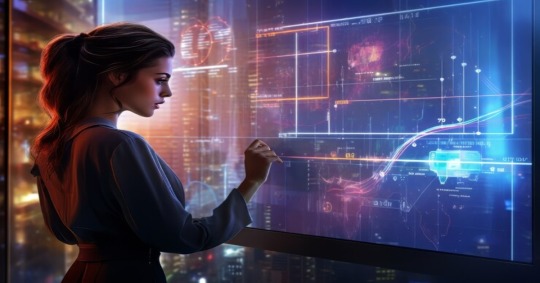
Introduction
In today's data-driven world, the ability to analyse information as it's generated is becoming increasingly crucial. Traditional batch processing, where data is collected and analysed periodically, can leave businesses lagging behind. This is where real-time data ingestion comes into play.
Overview Real-Time Data Ingestion
Real-time data ingestion refers to the continuous process of capturing, processing, and storing data streams as they are generated. This data can come from various sources, including sensor networks, social media feeds, financial transactions, website traffic logs, and more.
By ingesting and analysing data in real-time, businesses can gain valuable insights and make informed decisions with minimal latency.
Key Concepts in Real-Time Data Ingestion
Data Streams: Continuous flows of data generated by various sources, requiring constant ingestion and processing.
Event Stream Processing (ESP): Real-time processing engines that analyse data streams as they arrive, identifying patterns and extracting insights.
Microservices Architecture: Breaking down data processing tasks into smaller, independent services for increased scalability and agility in real-time environments.
Data Pipelines: Defined pathways for data to flow from source to destination, ensuring seamless data ingestion and transformation.
Latency: The time it takes for data to travel from its source to the point of analysis. Minimising latency is crucial for real-time applications.
Strategies for Implementing Real-Time Data Ingestion
Ready to harness the power of real-time data? Dive into this section to explore key strategies for implementing real-time data ingestion. Discover how to choose the right tools, ensure data quality, and design a scalable architecture for seamless data capture and processing.
Choosing the Right Tools: Select data ingestion tools that can handle high-volume data streams and offer low latency processing, such as Apache Kafka, Apache Flink, or Amazon Kinesis.
Data Stream Preprocessing: Clean, filter, and transform data streams as they are ingested to ensure data quality and efficient processing.
Scalability and Performance: Design your real-time data ingestion architecture to handle fluctuating data volumes and maintain acceptable processing speed.
Monitoring and Alerting: Continuously monitor your data pipelines for errors or performance issues. Implement automated alerts to ensure timely intervention if problems arise.
Benefits of Real-Time Data Ingestion
Explore the transformative benefits of real-time data ingestion. Discover how it empowers businesses to make faster decisions, enhance customer experiences, and optimise operations for a competitive edge.
Enhanced Decision-Making: Real-time insights allow businesses to react quickly to market changes, customer behaviour, or operational issues.
Improved Customer Experience: By analysing customer interactions in real-time, businesses can personalise recommendations, address concerns promptly, and optimise customer journeys.
Fraud Detection and Prevention: Real-time analytics can identify suspicious activity and prevent fraudulent transactions as they occur.
Operational Efficiency: Monitor machine performance, resource utilisation, and potential equipment failures in real-time to optimise operations and minimise downtime.
Risk Management: Real-time data analysis can help predict and mitigate potential risks based on real-time market fluctuations or social media sentiment.
Challenges in Real-Time Data Ingestion
Real-time data streams are powerful, but not without hurdles. Dive into this section to explore the challenges of high data volume, ensuring data quality, managing complexity, and keeping your data secure.
Data Volume and Velocity: Managing high-volume data streams and processing them with minimal latency can be a challenge.
Data Quality: Maintaining data quality during real-time ingestion is crucial, as errors can lead to inaccurate insights and poor decision-making.
Complexity: Real-time data pipelines involve various technologies and require careful design and orchestration to ensure smooth operation.
Security Concerns: Protecting sensitive data while ingesting and processing data streams in real-time requires robust security measures.
Use Cases of Real-Time Data Ingestion
Learn how real-time data ingestion fuels innovation across industries, from fraud detection in finance to personalised marketing in e-commerce. Discover the exciting possibilities that real-time insights unlock.
Fraud Detection: Financial institutions use real-time analytics to identify and prevent fraudulent transactions as they occur.
Personalized Marketing: E-commerce platforms leverage real-time customer behaviour data to personalise product recommendations and promotions.
IoT and Sensor Data Analysis: Real-time data from sensors in connected devices allows for monitoring equipment health, optimising energy consumption, and predicting potential failures.
Stock Market Analysis: Financial analysts use real-time data feeds to analyse market trends and make informed investment decisions.
Social Media Monitoring: Brands can track social media sentiment and brand mentions in real-time to address customer concerns and manage brand reputation.
Best Practices for Real-Time Data Ingestion
Unleashing the full potential of real-time data! Dive into this section for best practices to optimise your data ingestion pipelines, ensuring quality, performance, and continuous improvement.
Plan and Design Thoroughly: Clearly define requirements and design your real-time data ingestion architecture considering scalability, performance, and security.
Choose the Right Technology Stack: Select tools and technologies that can handle the volume, velocity, and variety of data you expect to ingest.
Focus on Data Quality: Implement data cleaning and validation techniques to ensure the accuracy and consistency of your real-time data streams.
Monitor and Maintain: Continuously monitor your data pipelines for errors and performance issues. Implement proactive maintenance procedures to ensure optimal performance.
Embrace Continuous Improvement: The field of real-time data ingestion is constantly evolving. Stay updated on new technologies and best practices to continuously improve your data ingestion pipelines.
Conclusion
Real-time data ingestion empowers businesses to operate in an ever-changing environment. By understanding the key concepts, implementing effective strategies, and overcoming the challenges, businesses can unlock the power of real-time insights to gain a competitive edge.
From enhanced decision-making to improved customer experiences and operational efficiency, real-time data ingestion holds immense potential for organisations across diverse industries. As technology continues to advance, real-time data ingestion will become an even more critical tool for success in the data-driven future.
Frequently Asked Questions
What is the Difference Between Real-Time and Batch Data Processing?
Real-time data ingestion processes data as it's generated, offering near-instant insights. Batch processing collects data periodically and analyses it later, leading to potential delays in decision-making.
What are Some of The Biggest Challenges in Real-Time Data Ingestion?
High data volume and velocity, maintaining data quality during processing, and ensuring the security of sensitive data streams are some of the key challenges to overcome.
How Can My Business Benefit from Real-Time Data Ingestion?
Real-time insights can revolutionise decision-making, personalise customer experiences, detect fraud instantly, optimise operational efficiency, and identify potential risks before they escalate.
0 notes
Text
#Linux File Replication#Linux Data Replication#big data#data protection#Cloud Solutions#data orchestration#Data Integration in Real Time#Data ingestion in real time#Cloud Computing
0 notes
Text
C&A is a neobotanics and nooscionics lab. In other words, they grow and train sophont AIs, “seedlets,” and design software and hardware to interface organic minds with digital systems.
It’s a small enough company to draw little suspicion, but credible enough to be contracted by big name operations (mainly military).
They accidentally trapped a person in a noospace, the “noosciocircus,” with Caine, a seedlet grown and owned by C&A. He’s a well meaning seedlet, tasked to keep the trapped person sane as C&A keeps their body alive as long as possible.
In an effort to recover the person from the inside, they sent in another only to trap them as well. Their cumulating mistake becomes harder to pull the plug on as it would kill both the trapped and Caine, an expensive investment who just also happens to be relaying immensely valuable nootic data from his ongoing simulation.
C&A continues to send agents to assist the trapped from within, each with relevant skills. They’re getting a bit desperate, since the pool of candidates is limited to those who work with C&A and would not draw too much attention if gone missing.
So, the noosciocircus becomes testing ground for lesser semiohazards.
Semiohazards are stimuli that trigger a destructive response in the minds of perceivers. Semiohazards can be encoded into any medium, but are generally easiest to encode into sights and air pressure sequences. The effect, “a mulekick,” can range in severity from temporarily disabled breathing, to seizure, to brain death.
Extreme amputations (“truncations”) occur when a trapped agent ingests a semiohazard that shuts off the brain’s recognition of some body part as its own. Sieving is a last resort to permanently mechanically support the life of the trapped. Thanks to modern advancements, this is cheap and sustainable. Those overexposed to the hazards become the abstracted and are considered lost. Their bodies are kept alive for archival.
Semiohazards being a current hotspot of discovery and design means C&A is sending in semiotic specialists alongside programmers. Ragatha was sent in to provide the trapped with nootic endurance training, but she underestimated the condition of the trapped. Gangle, too, was sent to help the trapped navigate their new nootic state, but her own dealt avatar clotheslined her progress. She wasn’t too stable entering to begin with, but C&A’s options are limited.
#noosciocircus#my art#the amazing digital circus#char speaks#bad ending#tadc Ragatha#tadc Pomni#tadc Zooble#tadc gangle#tadc jax#tadc Kinger#tadc Caine#sophont ai#the amazing digital circus au#digital circus
1K notes
·
View notes
Text
Drasi by Microsoft: A New Approach to Tracking Rapid Data Changes
New Post has been published on https://thedigitalinsider.com/drasi-by-microsoft-a-new-approach-to-tracking-rapid-data-changes/
Drasi by Microsoft: A New Approach to Tracking Rapid Data Changes
Imagine managing a financial portfolio where every millisecond counts. A split-second delay could mean a missed profit or a sudden loss. Today, businesses in every sector rely on real-time insights. Finance, healthcare, retail, and cybersecurity, all need to react instantly to changes, whether it is an alert, a patient update, or a shift in inventory. But traditional data processing cannot keep up. These systems often delay responses, costing time and missed opportunities.
That is where Drasi by Microsoft comes in. Designed to track and react to data changes as they happen, Drasi operates continuously. Unlike batch-processing systems, it does not wait for intervals to process information. Drasi empowers businesses with the real-time responsiveness they need to stay ahead of the competitors.
Understanding Drasi
Drasi is an advanced event-driven architecture powered by Artificial Intelligence (AI) and designed to handle real-time data changes. Traditional data systems often rely on batch processing, where data is collected and analyzed at set intervals. This approach can cause delays, which can be costly for industries that depend on quick responses. Drasi changes the game by using AI to track data continuously and react instantly. This enables organizations to make decisions as events happen instead of waiting for the next processing cycle.
A core feature of Drasi is its AI-driven continuous query processing. Unlike traditional queries that run on a schedule, continuous queries operate non-stop, allowing Drasi to monitor data flows in real time. This means even the smallest data change is captured immediately, giving companies a valuable advantage in responding quickly. Drasi’s machine learning capabilities help it integrate smoothly with various data sources, including IoT devices, databases, social media, and cloud services. This broad compatibility provides a complete view of data, helping companies identify patterns, detect anomalies, and automate responses effectively.
Another key aspect of Drasi’s design is its intelligent reaction mechanism. Instead of simply alerting users to a data change, Drasi can immediately trigger pre-set responses and even use machine learning to improve these actions over time. For example, in finance, if Drasi detects an unusual market event, it can automatically send alerts, notify the right teams, or even make trades. This AI-powered, real-time functionality gives Drasi a clear advantage in industries where quick, adaptive responses make a difference.
By combining continuous AI-powered queries with rapid response capabilities, Drasi enables companies to act on data changes the moment they happen. This approach boosts efficiency, cuts down on delays, and reveals the full potential of real-time insights. With AI and machine learning built in, Drasi’s architecture offers businesses a powerful advantage in today’s fast-paced, data-driven world.
Why Drasi Matters for Real-Time Data
As data generation continues to grow rapidly, companies are under increasing pressure to process and respond to information as it becomes available. Traditional systems often face issues, such as latency, scalability, and integration, which limit their usefulness in real-time settings. This is especially critical in high-stakes sectors like finance, healthcare, and cybersecurity, where even brief delays can result in losses. Drasi addresses these challenges with an architecture designed to handle large amounts of data while maintaining speed, reliability, and adaptability.
In financial trading, for example, investment firms and banks depend on real-time data to make quick decisions. A split-second delay in processing stock prices can mean the difference between a profitable trade and a missed chance. Traditional systems that process data in intervals simply cannot keep up with the pace of modern markets. Drasi’s real-time processing capability allows financial institutions to respond instantly to market shifts, optimizing trading strategies.
Similarly, in a connected smart home, IoT sensors track everything from security to energy use. A traditional system may only check for updates every few minutes, potentially leaving the home vulnerable if an emergency occurs during that interval. Drasi enables constant monitoring and immediate responses, such as locking doors at the first sign of unusual activity, thereby enhancing security and efficiency.
Retail and e-commerce also benefit significantly from Drasi’s capabilities. E-commerce platforms rely on understanding customer behavior in real time. For instance, if a customer adds an item to their cart but doesn’t complete the purchase, Drasi can immediately detect this and trigger a personalized prompt, like a discount code, to encourage the sale. This ability to react to customer actions as they happen can lead to more sales and create a more engaging shopping experience. In each of these cases, Drasi fills a significant gap where traditional systems lack and thus empowers businesses to act on live data in ways previously out of reach.
Drasi’s Real-Time Data Processing Architecture
Drasi’s design is centred around an advanced, modular architecture, prioritizing scalability, speed, and real-time operation. Maily, it depends on continuous data ingestion, persistent monitoring, and automated response mechanisms to ensure immediate action on data changes.
When new data enters Drasi’s system, it follows a streamlined operational workflow. First, it ingests data from various sources, including IoT devices, APIs, cloud databases, and social media feeds. This flexibility enables Drasi to collect data from virtually any source, making it highly adaptable to different environments.
Once data is ingested, Drasi’s continuous queries immediately monitor the data for changes, filtering and analyzing it as soon as it arrives. These queries run perpetually, scanning for specific conditions or anomalies based on predefined parameters. Next, Drasi’s reaction system takes over, allowing for automatic responses to these changes. For instance, if Drasi detects a significant increase in website traffic due to a promotional campaign, it can automatically adjust server resources to accommodate the spike, preventing potential downtime.
Drasi’s operational workflow involves several key steps. Data is ingested from connected sources, ensuring real-time compatibility with devices and databases. Continuous queries then scan for predefined changes, eliminating delays associated with batch processing. Advanced algorithms process incoming data to provide meaningful insights immediately. Based on these data insights, Drasi can trigger predefined responses, such as notifications, alerts, or direct actions. Finally, Drasi’s real-time analytics transform data into actionable insights, empowering decision-makers to act immediately.
By offering this streamlined process, Drasi ensures that data is not only tracked but also acted upon instantly, enhancing a company’s ability to adapt to real-time conditions.
Benefits and Use Cases of Drasi
Drasi offers benefits far beyond typical data processing capabilities and provides real-time responsiveness essential for businesses that need instant data insights. One key advantage is its enhanced efficiency and performance. By processing data as it arrives, Drasi removes delays common in batch processing, leading to faster decision-making, improved productivity, and reduced downtime. For example, a logistics company can use Drasi to monitor delivery statuses and reroute vehicles in real time, optimizing operations to reduce delivery times and increase customer satisfaction.
Real-time insights are another benefit. In industries like finance, healthcare, and retail, where information changes quickly, having live data is invaluable. Drasi’s ability to provide immediate insights enables organizations to make informed decisions on the spot. For example, a hospital using Drasi can monitor patient vitals in real time, supplying doctors with important updates that could make a difference in patient outcomes.
Furthermore, Drasi integrates with existing infrastructure and enables businesses to employ its capabilities without investing in costly system overhauls. A smart city project, for example, could use Drasi to integrate traffic data from multiple sources, providing real-time monitoring and management of traffic flows to reduce congestion effectively.
As an open-source tool, Drasi is also cost-effective, offering flexibility without locking businesses into expensive proprietary systems. Companies can customize and expand Drasi’s functionalities to suit their needs, making it an affordable solution for improving data management without a significant financial commitment.
The Bottom Line
In conclusion, Drasi redefines real-time data management, offering businesses an advantage in today’s fast-paced world. Its AI-driven, event-based architecture enables continuous monitoring, instant insights, and automatic responses, which are invaluable across industries.
By integrating with existing infrastructure and providing cost-effective, customizable solutions, Drasi empowers companies to make immediate, data-driven decisions that keep them competitive and adaptive. In an environment where every second matters, Drasi proves to be a powerful tool for real-time data processing.
Visit the Drasi website for information about how to get started, concepts, how to explainers, and more.
#ai#AI-powered#AI-powered data processing#alerts#Algorithms#Analytics#anomalies#APIs#approach#architecture#artificial#Artificial Intelligence#banks#Behavior#change#Cloud#cloud services#code#Commerce#Companies#continuous#continuous monitoring#cybersecurity#data#data ingestion#Data Management#data processing#data-driven#data-driven decisions#databases
0 notes
Text
Unveiling the Power of Delta Lake in Microsoft Fabric
Discover how Microsoft Fabric and Delta Lake can revolutionize your data management and analytics. Learn to optimize data ingestion with Spark and unlock the full potential of your data for smarter decision-making.
In today’s digital era, data is the new gold. Companies are constantly searching for ways to efficiently manage and analyze vast amounts of information to drive decision-making and innovation. However, with the growing volume and variety of data, traditional data processing methods often fall short. This is where Microsoft Fabric, Apache Spark and Delta Lake come into play. These powerful…
#ACID Transactions#Apache Spark#Big Data#Data Analytics#data engineering#Data Governance#Data Ingestion#Data Integration#Data Lakehouse#Data management#Data Pipelines#Data Processing#Data Science#Data Warehousing#Delta Lake#machine learning#Microsoft Fabric#Real-Time Analytics#Unified Data Platform
0 notes
Text
#Best Real time Data Ingestion Tools#Real-time Data Ingestion#types of data ingestion#What is the most important thing for real time data ingestion
0 notes
Note
How would we "give birth" to bee hybrids?
Bc obviously theres egg, but do we lay them like bees do in the honeycomb?
Or do we give birth like a person would?
This is actually highly debated amongst different hives.
Some believe laying eggs in a honeycomb is both the most natural and best way to go about birthing. It’s what bees did and their ancestors did, so that’s what they should do!
There are others that say incubating them in your womb and giving birth to them live creates more loyal subjects that will stick to their queen through anything!
The truth? Either way is fine and gets the job done. There’s very little information to back up which way is better for the baby bees, as giving birth to live babies is new and hasn’t had a higher mortality rate than laying eggs into a comb.
Scientist bees are still collecting data from different hives to see which way is truly the best method… but I’d say it depends on the mother and what she thinks is best for her own body.
Just like some mothers think ingesting honey straight from the father’s own collection will help build their immunity, others think introducing the little ones to a wide array of honeys at an early age can make sure they’re healthy and will make better honey later in life. It’s a simple difference of opinion that makes no real difference either way.
A baby that survives incubation is a good baby, whether it’s from birthing or being laid by their mother.
Good job mamas, you’re doing your best!
a/n: tried to make this read like an article from a mommy blog that tries to stay neutral on topics lol
#bee hybrid lore#bee hybrid x reader#bee hybrid fluff#bee hybrid#monster fucker#monster lover#monster fudger#monster boyfriend#ask answered#monster fic#anon ask#terato#teraphilia#chubby!reader#teratophillia#terat0philliac#exophelia#monster x you#monster x reader#monster x human#insect monster#monster imagine#monster fucking#x reader#monster bf#fem reader#female reader#monster boy oc
766 notes
·
View notes
Text
Harnessing the Power of Incremental Data Ingestion with AWS Glue: A Path to Efficient Data Processing
In today's data-driven world, organizations face the challenge of managing and processing vast amounts of data efficiently. Incremental data ingestion plays a crucial role in enabling organizations to handle data growth while optimizing resources and reducing processing time. This whitepaper highlights the significance of incremental data ingestion and explores the automated mechanism of AWS Glue, a powerful service provided by Amazon Web Services (AWS), for efficient and streamlined data ingestion.
The Importance of Incremental Data Ingestion:
As data volumes continue to explode, traditional approaches to data ingestion, such as full data loads, can become time-consuming and resource-intensive. Incremental data ingestion focuses on capturing and processing only the changes or updates that occur since the last ingestion, significantly reducing the processing overhead and improving overall efficiency. This approach is particularly valuable for organizations dealing with large datasets, frequent data updates, and real-time analytics requirements.
Automated Incremental Data Ingestion with AWS Glue:
AWS Glue offers a comprehensive set of tools and services for automated data ingestion, transformation, and preparation. With its incremental data ingestion capabilities, AWS Glue simplifies the process of capturing and processing only the changed data, ensuring efficient data processing and minimizing unnecessary overhead.
Data Catalog and Discovery:
AWS Glue provides a centralized data catalog that automatically crawls and catalogs data from various sources, including databases, data lakes, and data warehouses. The data catalog allows organizations to discover and understand the structure, metadata, and relationships of their data assets, enabling efficient data ingestion.
Change Data Capture (CDC):
AWS Glue supports Change Data Capture, which captures and identifies changes made to the data sources since the last ingestion. By capturing only the changed data, organizations can significantly reduce the processing time and resources required for data ingestion.
Schema Evolution:
As data sources evolve over time, the schema may change. AWS Glue enables automated schema evolution, adapting to the changes in data structure seamlessly. This ensures data integrity and compatibility during incremental data ingestion, without manual intervention.
Data Deduplication and Validation:
AWS Glue provides built-in mechanisms for data deduplication and validation during the incremental data ingestion process. This ensures data accuracy and consistency by eliminating duplicate records and validating the integrity of the ingested data.
Data Transformation and ETL:
Alongside incremental data ingestion, AWS Glue offers powerful data transformation and Extract, Transform, Load (ETL) capabilities. Organizations can leverage AWS Glue's visual interface or custom scripts to transform and prepare the ingested data for downstream analytics and processing.
Scalability and Performance:
AWS Glue is designed to handle large-scale data processing requirements. It automatically scales resources based on demand, ensuring high-performance processing of incremental data ingestion tasks.
Conclusion:
Incremental data ingestion is a critical component of efficient data processing in today's data-driven landscape. By adopting an automated approach to incremental data ingestion with AWS Glue, organizations can minimize processing overhead, reduce resource consumption, and streamline their data pipelines. AWS Glue's comprehensive toolset empowers organizations to catalog, discover, capture, transform, and validate changed data efficiently, enhancing overall data processing capabilities. Embracing automated incremental data ingestion with AWS Glue on AWS provides organizations with a robust solution for handling large datasets, real-time analytics, and evolving data sources, driving data-driven insights and accelerating innovation.
1 note
·
View note
Note
What if the Cybertronians saw the reader drinking blue gatorade and thought it was Energon.
I went with Prowl headcanons, he doesnt get enough love.
-
-
- Energon is known, at least to the autobots on Earth, to be toxic to human, Ratchet stressed after an accident that no human companion of theirs should be exposed to it for too long and to never ingest it for it could at minium do serious harm, but more likely kill their little human.
- Prowl does not mind you always bothering him as he works, he’s grown use to you and your antics making it easier to block you out or at least still get things done. So when you walk into his office, greeting him as always, he doesn’t look away from his data pad as he greets you in return.
- You climb up his desk (with the stairs he absolutely did not build in so you could climb up it safer) and sit near his servos.
- You chat with him with ease, asking about his day which he tells you little about, but he’s still nice to be with.
- Bright blue catches his attention off the corner of his optics, he almost assumed you brought him the worlds smallest Energon cube, until he turns his head and sees you drinking it. His optics widen a fraction and before you know it you’re being yanked into the air, you bottle falling from your hands spilling the liquid all over his desk, but he doesn’t care.
- You ask him what’s wrong but he doesn’t even answer you as he’s speeding out of his office, swiftly transforming making your head spin as you find yourself in the passenger seat. His sirens blaring as he drives, speeding down the hall making any autobot jump to the side to get out of his way.
- “Prowl, what’s going on!?”
- “You are a fragging idiot! We can make it to Ratchet, I won’t let you offline.”
- You’re so confused. Prowl slams on his brakes as he bursts through the medbay doors, gaining the attention of a newly pissed off Racteht, before transforming once more, this time holding you out to the medbot.
- “They drank energon!”
- And like that Ratchet is taking you, setting you on the medical berth and hooking so many things up to you as he’s loudly scolding you for even touching energon. But you can’t remember drinking energon, you didn’t have any! The only time you’re even near it is when you’re around them as they drink it.
- Prowl and Ratchet talk amongst themselves, though it’s clear they are both worried. Ratchet is not trained to handle humans, your bodies are so much more fragile and complex than he studied for. It takes Prowl telling Ratchet the story for it to finally click in your head.
“That wasn’t energon, that was a Gatorade!”
The two bots look at you, optics narrowed, squinting at you in suspicion.
“Aren’t gators those lizard things you spoke of with the powerful bite force? Why would they need aid?” Prowl questions, crossing his arms over his chassis.
“And why would you be drinking it?” Ratchet follows up.
You have to pull up your phone to look it up in bigger words to get it through their processors that you really are fine, it was just a tasty drink! Though it doesn’t help the two bots groans, shaking their helms and muttering something humans being weird, and making odd scrap as always.
But as Prowl holds you in his servo as you two leave the medbay, he looks at you with a stern expression.
“Don’t you ever do that to me again, understood?”
You have to fight back a smile, knowing how worried he must’ve been, “I promise Prowl, I’ll make sure to let you know what I got before hand.”
He’s a worry wot, give him a break.
#transformers#transformers prowl#transformers x reader#transformers headcanons#transformers prowl x reader#transformers prowl headcanons
420 notes
·
View notes