#JupyterNotebooks
Explore tagged Tumblr posts
Text
Discover the top Data Science tools for beginners and learn where to start your journey. From Python and R to Tableau and SQL, find the best tools to build your Data Science skills.
#DataScience#BeginnersGuide#Python#RLanguage#SQL#Tableau#JupyterNotebooks#DataVisualization#LearningDataScience#TechTools
0 notes
Text
Dip Your Toes in Finance with Me!
I am very excited to share this with everyone! One of the aspects of teaching that I loved the most as substitute prof back in the day was sharing my notes with everyone and then discussing them.
In my very first post on my Notes of Finance series, I have started with the concept of Brownian motion and Standard Brownian motion, taking a somewhat deep dive into the meaning of the drift and diffusion constants.
I hope you enjoy following my Jupyter notebook, as I am eager to discuss this with everyone.
Keep tuned for the next posts in this series, as I now move to pricing!
0 notes
Text
Best way to learn data analysis with python
The best way to learn data analysis with Python is to start with the basics and gradually build up your skills through practice and projects. Begin by learning the fundamentals of Python programming, which you can do through online courses, tutorials, or books. Once you are comfortable with the basics, focus on learning key data analysis libraries such as Pandas for data manipulation, NumPy for numerical operations, and Matplotlib or Seaborn for data visualization.
After you grasp the basics, apply your knowledge by working on real datasets. Platforms like Kaggle offer numerous datasets and competitions that can help you practice and improve your skills. Additionally, taking specialized data analysis courses online can provide structured learning and deeper insights. Consistently practicing, participating in communities like Stack Overflow or Reddit for support, and staying updated with the latest tools and techniques will help you become proficient in data analysis with Python.
#Dataanalysis#Pythonprogramming#Learnpython#Datascience#Pandas#NumPy#Datavisualization#Matplotlib#Seaborn#Kaggle#Pythoncourses#CodingforBeginners#DataPreparation#StatisticsWithPython#JupyterNotebooks#VSCode#OnlineLearning#TechSkills#ProgrammingTutorials#DataScienceCommunity
0 notes
Text
0 notes
Text
CORRECTED: Causes of Death In Infants.
My apologies. The previous "Causes" plot had the data reversed. Here is the correct plot.
4 notes
·
View notes
Text
Python Scipy . . . . for more information and a tutorial https://bit.ly/3RhYl8V check the above link
2 notes
·
View notes
Text
1/100 Days of Code #2
hello & welcome again to 100 days of coding! this theme helped me out while working thru the odin project pretty steadily.
i have officially switched tracks to python to get some deeper foundations set & try to fix my gaps in understanding on js. i tried for 1 whole year to bridge these gaps using js (so many textbooks, thanks no starch press!) and i’m frankly dog tired of not making progress!
so!
my new online learning course had me install jupyter & i’m honestly feeling a lot better already than i did even using VSCode or IDLE for the python stuff lmao.
that’s what i did today: 30 mins of set up, and about 2 hours of season 1 SNL as a reward. (30/30 a day is my proposed model for this project no exceptions, but the library hungers for SNL’s return so,,, prioritizing!)
anyway… ttyt can’t wait!!
1 note
·
View note
Text
Anyone else having trouble getting jupyter to export to pdf? I'm not sure if i just have some installs not there or what
1 note
·
View note
Text
Open Source Tools for Data Science: A Beginner’s Toolkit
Data science is a powerful tool used by companies and organizations to make smart decisions, improve operations, and discover new opportunities. As more people realize the potential of data science, the need for easy-to-use and affordable tools has grown. Thankfully, the open-source community provides many resources that are both powerful and free. In this blog post, we will explore a beginner-friendly toolkit of open-source tools that are perfect for getting started in data science.
Why Use Open Source Tools for Data Science?
Before we dive into the tools, it’s helpful to understand why using open-source software for data science is a good idea:
1. Cost-Effective: Open-source tools are free, making them ideal for students, startups, and anyone on a tight budget.
2. Community Support: These tools often have strong communities where people share knowledge, help solve problems, and contribute to improving the tools.
3. Flexible and Customizable: You can change and adapt open-source tools to fit your needs, which is very useful in data science, where every project is different.
4. Transparent: Since the code is open for anyone to see, you can understand exactly how the tools work, which builds trust.
Essential Open Source Tools for Data Science Beginners
Let’s explore some of the most popular and easy-to-use open-source tools that cover every step in the data science process.
1. Python
The most often used programming language for data science is Python. It's highly adaptable and simple to learn.
Why Python?
- Simple to Read: Python’s syntax is straightforward, making it a great choice for beginners.
- Many Libraries: Python has a lot of libraries specifically designed for data science tasks, from working with data to building machine learning models.
- Large Community: Python’s community is huge, meaning there are lots of tutorials, forums, and resources to help you learn.
Key Libraries for Data Science:
- NumPy: Handles numerical calculations and array data.
- Pandas: Helps you organize and analyze data, especially in tables.
- Matplotlib and Seaborn: Used to create graphs and charts to visualize data.
- Scikit-learn: A powerful tool for machine learning, offering easy-to-use tools for data analysis.
2. Jupyter Notebook
Jupyter Notebook is a web application where you can write and run code, see the results, and add notes—all in one place.
Why Jupyter Notebook?
- Interactive Coding: You can write and test code in small chunks, making it easier to learn and troubleshoot.
- Great for Documentation: You can write explanations alongside your code, which helps keep your work organized.
- Built-In Visualization: Jupyter works well with visualization libraries like Matplotlib, so you can see your data in graphs right in your notebook.
3. R Programming Language
R is another popular language in data science, especially known for its strength in statistical analysis and data visualization.
Why R?
- Strong in Statistics: R is built specifically for statistical analysis, making it very powerful in this area.
- Excellent Visualization: R has great tools for making beautiful, detailed graphs.
- Lots of Packages: CRAN, R’s package repository, has thousands of packages that extend R’s capabilities.
Key Packages for Data Science:
- ggplot2: Creates high-quality graphs and charts.
- dplyr: Helps manipulate and clean data.
- caret: Simplifies the process of building predictive models.
4. TensorFlow and Keras
TensorFlow is a library developed by Google for numerical calculations and machine learning. Keras is a simpler interface that runs on top of TensorFlow, making it easier to build neural networks.
Why TensorFlow and Keras?
- Deep Learning: TensorFlow is excellent for deep learning, a type of machine learning that mimics the human brain.
- Flexible: TensorFlow is highly flexible, allowing for complex tasks.
- User-Friendly with Keras: Keras makes it easier for beginners to get started with TensorFlow by simplifying the process of building models.
5. Apache Spark
Apache Spark is an engine used for processing large amounts of data quickly. It’s great for big data projects.
Why Apache Spark?
- Speed: Spark processes data in memory, making it much faster than traditional tools.
- Handles Big Data: Spark can work with large datasets, making it a good choice for big data projects.
- Supports Multiple Languages: You can use Spark with Python, R, Scala, and more.
6. Git and GitHub
Git is a version control system that tracks changes to your code, while GitHub is a platform for hosting and sharing Git repositories.
Why Git and GitHub?
- Teamwork: GitHub makes it easy to work with others on the same project.
- Track Changes: Git keeps track of every change you make to your code, so you can always go back to an earlier version if needed.
- Organize Projects: GitHub offers tools for managing and documenting your work.
7. KNIME
KNIME (Konstanz Information Miner) is a data analytics platform that lets you create visual workflows for data science without writing code.
Why KNIME?
- Easy to Use: KNIME’s drag-and-drop interface is great for beginners who want to perform complex tasks without coding.
- Flexible: KNIME works with many other tools and languages, including Python, R, and Java.
- Good for Visualization: KNIME offers many options for visualizing your data.
8. OpenRefine
OpenRefine (formerly Google Refine) is a tool for cleaning and organizing messy data.
Why OpenRefine?
- Data Cleaning: OpenRefine is great for fixing and organizing large datasets, which is a crucial step in data science.
- Simple Interface: You can clean data using an easy-to-understand interface without writing complex code.
- Track Changes: You can see all the changes you’ve made to your data, making it easy to reproduce your results.
9. Orange
Orange is a tool for data visualization and analysis that’s easy to use, even for beginners.
Why Orange?
- Visual Programming: Orange lets you perform data analysis tasks through a visual interface, no coding required.
- Data Mining: It offers powerful tools for digging deeper into your data, including machine learning algorithms.
- Interactive Exploration: Orange’s tools make it easier to explore and present your data interactively.
10. D3.js
D3.js (Data-Driven Documents) is a JavaScript library used to create dynamic, interactive data visualizations on websites.
Why D3.js?
- Highly Customizable: D3.js allows for custom-made visualizations that can be tailored to your needs.
- Interactive: You can create charts and graphs that users can interact with, making data more engaging.
- Web Integration: D3.js works well with web technologies, making it ideal for creating data visualizations for websites.
How to Get Started with These Tools
Starting out in data science can feel overwhelming with so many tools to choose from. Here’s a simple guide to help you begin:
1. Begin with Python and Jupyter Notebook: These are essential tools in data science. Start by learning Python basics and practice writing and running code in Jupyter Notebook.
2. Learn Data Visualization: Once you're comfortable with Python, try creating charts and graphs using Matplotlib, Seaborn, or R’s ggplot2. Visualizing data is key to understanding it.
3. Master Version Control with Git: As your projects become more complex, using version control will help you keep track of changes. Learn Git basics and use GitHub to save your work.
4. Explore Machine Learning: Tools like Scikit-learn, TensorFlow, and Keras are great for beginners interested in machine learning. Start with simple models and build up to more complex ones.
5. Clean and Organize Data: Use Pandas and OpenRefine to tidy up your data. Data preparation is a vital step that can greatly affect your results.
6. Try Big Data with Apache Spark: If you’re working with large datasets, learn how to use Apache Spark. It’s a powerful tool for processing big data.
7. Create Interactive Visualizations: If you’re interested in web development or interactive data displays, explore D3.js. It’s a fantastic tool for making custom data visualizations for websites.
Conclusion
Data science offers a wide range of open-source tools that can help you at every step of your data journey. Whether you're just starting out or looking to deepen your skills, these tools provide everything you need to succeed in data science. By starting with the basics and gradually exploring more advanced tools, you can build a strong foundation in data science and unlock the power of your data.
#DataScience#OpenSourceTools#PythonForDataScience#BeginnerDataScience#JupyterNotebook#RProgramming#MachineLearning#TensorFlow#DataVisualization#BigDataTools#GitAndGitHub#KNIME#DataCleaning#OrangeDataScience#D3js#DataScienceForBeginners#DataScienceToolkit#DataAnalytics#data science course in Coimbatore#LearnDataScience#FreeDataScienceTools
1 note
·
View note
Text
🚀 Boost your Python skills with these handy shortcuts and commands! 💻 Whether you're a data analyst, coder, or a tech enthusiast, these time-savers will level up your workflow. 🐍🔥 Which one's your favorite?
#Python#CodingShortcuts#ProductivityHacks#JupyterNotebook#VSCode#CodeLikeAPro#TechTips#DataScience#DataAnalysis#CodeEditor#LearnPython#ShortcutKeys#Programming#CodingLife#ProgrammingTricks#TrendingTech#BoostYourSkills#TechTools#ProgrammerLife#PythonForDataScience#TechTrends#EfficiencyBoost
0 notes
Text
نصائح وحيل Jupyter Notebook لزيادة إنتاجيتك في تحليل البيانات

Jupyter Notebook هو عبارة عن بيئة حوسبة تفاعلية قائمة على الويب يُمكنك استخدامه لتحليل البيانات والبرمجة التعاونية. حيث يسمح بدمج الأكواد والنصوص والتصورات في مستند واحد. لديه دعم لنظام بيئي واسع من المكتبات المُتاحة لإنجاز مهام مختلفة. يُمكن استخدامه من قبل العلماء والباحثين والمُطورين لمجموعة متنوعة من الأغراض ، بما في ذلك تصور البيانات وإنشاء التقارير وكتابة النصوص. حيث إنه يقود عالَم علم البيانات عندما يتعلق الأمر بتحليل البيانات ومعالجتها وهندسة الميزات. فيما يلي بعض النصائح والحيل الأساسية لمساعدتك في تحقيق أقصى استفادة من Jupyter Notebook. تحقق من طرق للبقاء على اطلاع بأحدث الاتجاهات في علم البيانات. Read the full article
0 notes
Text
youtube
0 notes
Photo
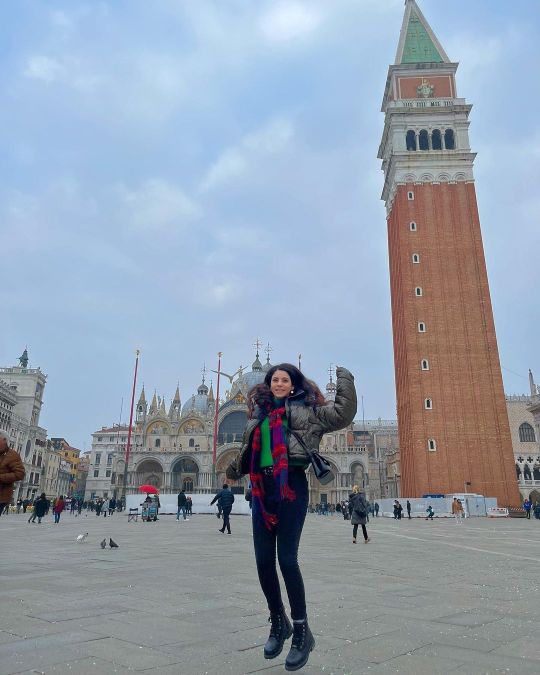
𝗠𝗮𝘁𝗵 𝗶𝘀 𝘃𝗲𝗿𝘆 𝗻𝗲𝗰𝗲𝘀𝘀𝗮𝗿𝘆 𝗳𝗼𝗿 𝘁𝗵𝗲 𝗳𝗶𝗲𝗹𝗱 𝗼𝗳 𝗺𝗮𝗰𝗵𝗶𝗻𝗲 𝗹𝗲𝗮𝗿𝗻𝗶𝗻𝗴 𝗮𝗻𝗱 𝗱𝗲𝗲𝗽 𝗹𝗲𝗮𝗿𝗻𝗶𝗻𝗴. 🌵Statistics and Probability Statistics and Probability is used for visualization of features, data preprocessing, feature transformation, data imputation, dimensionality reduction, feature engineering, model evaluation, etc. 🌵 Multivariable Calculus Most machine learning models are built with a data set having several features or predictors. Hence familiarity with multivariable calculus is extremely important for building a machine learning model. 🌵Linear Algebra Linear algebra is the most important math skill in machine machine. A data set is represented as a matrix. Linear algebra is used in data preprocessing, data transformation, and model evaluation. 🌵Optimization Methods Most machine learning algorithms perform predictive modeling by minimizing an objective function, thereby learning the weights that must be applied to the testing data in order to obtain the predicted labels. . . . #python #ai #machinelearning #datascience #ML #machinelearning #AI #artificialintelligence #deeplearning #DL #code #coding #python #pythoncode #programmer #programming #elearning #learning #opensource #codinglife #student #university #jupyternotebook #github #datascience #datavisualization #data #worksheet #interactive #inspiration (Venice - San Marco) https://www.instagram.com/p/Cp79UkYohc2/?igshid=NGJjMDIxMWI=
#python#ai#machinelearning#datascience#ml#artificialintelligence#deeplearning#dl#code#coding#pythoncode#programmer#programming#elearning#learning#opensource#codinglife#student#university#jupyternotebook#github#datavisualization#data#worksheet#interactive#inspiration
4 notes
·
View notes
Text
Jupyter Notebook Project: US Medical Insurance Costs
In this portfolio project, I was given a CSV of patients in the United States and was told to analyse the data in any way I chose. The data included gender, age, BMI, # of children, smoking status, region, and charges. I chose to analyse the relationship between smoking status and insurance charges.
Here it is!
#studyblr#23rd#August#2021#August 23rd 2021#computer science#compsci#data science#python#jupyternotebook
30 notes
·
View notes
Text
Kivy . . . . for more information and a tutorial https://bit.ly/4itVOEu check the above link
0 notes