#Large Language Model Development
Explore tagged Tumblr posts
Text
What Is the Role of AI Ethics in Custom Large Language Model Solutions for 2025?

The rapid evolution of artificial intelligence (AI) has led to significant advancements in technology, particularly in natural language processing (NLP) through the development of large language models (LLMs). These models, powered by vast datasets and sophisticated algorithms, are capable of understanding, generating, and interacting in human-like ways. As we move toward 2025, the importance of AI ethics in the creation and deployment of custom LLM solutions becomes increasingly critical. This blog explores the role of AI ethics in shaping the future of these technologies, focusing on accountability, fairness, transparency, and user privacy.
Understanding Custom Large Language Models
Before delving into AI ethics, it is essential to understand what custom large language models are. These models are tailored to specific applications or industries, allowing businesses to harness the power of AI while meeting their unique needs. Custom Large Language Model solutions can enhance customer service through chatbots, streamline content creation, improve accessibility for disabled individuals, and even support mental health initiatives by providing real-time conversation aids.
However, the deployment of such powerful technologies also raises ethical considerations that must be addressed to ensure responsible use. With the potential to influence decision-making, shape societal norms, and impact human behavior, LLMs pose both opportunities and risks.
The Importance of AI Ethics
1. Accountability
As AI systems become more integrated into daily life and business operations, accountability becomes a crucial aspect of their deployment. Who is responsible for the outputs generated by LLMs? If an LLM generates misleading, harmful, or biased content, understanding where the responsibility lies is vital. Developers, businesses, and users must collaborate to establish guidelines that outline accountability measures.
In custom LLM solutions, accountability involves implementing robust oversight mechanisms. This includes regular audits of model outputs, feedback loops from users, and clear pathways for addressing grievances. Establishing accountability ensures that AI technologies serve the public interest and that any adverse effects are appropriately managed.
2. Fairness and Bias Mitigation
AI systems are only as good as the data they are trained on. If the training datasets contain biases, the resulting LLMs will likely perpetuate or even amplify these biases. For example, an LLM trained primarily on texts from specific demographics may inadvertently generate outputs that favor those perspectives while marginalizing others. This phenomenon, known as algorithmic bias, poses significant risks in areas like hiring practices, loan approvals, and law enforcement.
Ethics in AI calls for fairness, which necessitates that developers actively work to identify and mitigate biases in their models. This involves curating diverse training datasets, employing techniques to de-bias algorithms, and ensuring that custom LLMs are tested across varied demographic groups. Fairness is not just a legal requirement; it is a moral imperative that can enhance the trustworthiness of AI solutions.
3. Transparency
Transparency is crucial in building trust between users and AI systems. Users should have a clear understanding of how LLMs work, the data they were trained on, and the processes behind their outputs. When users understand the workings of AI, they can make informed decisions about its use and limitations.
For custom LLM solutions, transparency involves providing clear documentation about the modelâs architecture, training data, and potential biases. This can include detailed explanations of how the model arrived at specific outputs, enabling users to gauge its reliability. Transparency also empowers users to challenge or question AI-generated content, fostering a culture of critical engagement with technology.
4. User Privacy and Data Protection
As LLMs often require large volumes of user data for personalization and improvement, ensuring user privacy is paramount. The ethical use of AI demands that businesses prioritize data protection and adopt strict privacy policies. This involves anonymizing user data, obtaining explicit consent for data usage, and providing users with control over their information.
Moreover, the integration of privacy-preserving technologies, such as differential privacy, can help protect user data while still allowing LLMs to learn and improve. This approach enables developers to glean insights from aggregated data without compromising individual privacy.
5. Human Oversight and Collaboration
While LLMs can operate independently, human oversight remains essential. AI should augment human decision-making rather than replace it. Ethical AI practices advocate for a collaborative approach where humans and AI work together to achieve optimal outcomes. This means establishing frameworks for human-in-the-loop systems, where human judgment is integrated into AI operations.
For custom LLM solutions, this collaboration can take various forms, such as having human moderators review AI-generated content or incorporating user feedback into model updates. By ensuring that humans play a critical role in AI processes, developers can enhance the ethical use of technology and safeguard against potential harms.
The Future of AI Ethics in Custom LLM Solutions
As we approach 2025, the role of AI ethics in custom large language model solutions will continue to evolve. Here are some anticipated trends and developments in the realm of AI ethics:
1. Regulatory Frameworks
Governments and international organizations are increasingly recognizing the need for regulations governing AI. By 2025, we can expect more comprehensive legal frameworks that address ethical concerns related to AI, including accountability, fairness, and transparency. These regulations will guide businesses in developing and deploying AI technologies responsibly.
2. Enhanced Ethical Guidelines
Professional organizations and industry groups are likely to establish enhanced ethical guidelines for AI development. These guidelines will provide developers with best practices for building ethical LLMs, ensuring that the technology aligns with societal values and norms.
3. Focus on Explainability
The demand for explainable AI will grow, with users and regulators alike seeking greater clarity on how AI systems operate. By 2025, there will be an increased emphasis on developing LLMs that can articulate their reasoning and provide users with understandable explanations for their outputs.
4. User-Centric Design
As user empowerment becomes a focal point, the design of custom LLM solutions will prioritize user needs and preferences. This approach will involve incorporating user feedback into model training and ensuring that ethical considerations are at the forefront of the development process.
Conclusion
The role of AI ethics in custom large language model solutions for 2025 is multifaceted, encompassing accountability, fairness, transparency, user privacy, and human oversight. As AI technologies continue to evolve, developers and organizations must prioritize ethical considerations to ensure responsible use. By establishing robust ethical frameworks and fostering collaboration between humans and AI, we can harness the power of LLMs while safeguarding against potential risks. In doing so, we can create a future where AI technologies enhance our lives and contribute positively to society.
#Custom Large Language Model Solutions#Custom Large Language Model#Custom Large Language#Large Language Model#large language model development services#large language model development#Large Language Model Solutions
0 notes
Text
Large Language Model Development Company
Large Language Model Development Company (LLMDC) is a pioneering organization at the forefront of artificial intelligence research and development. Specializing in the creation and refinement of large language models, LLMDC leverages cutting-edge technologies to push the boundaries of natural language understanding and generation. The company's mission is to develop advanced AI systems that can understand, generate, and interact with human language in a meaningful and contextually relevant manner.Â
With a team of world-class researchers and engineers, LLMDC focuses on a range of applications including automated customer service, content creation, language translation, and more. Their innovations are driven by a commitment to ethical AI development, ensuring that their technologies are not only powerful but also aligned with principles of fairness, transparency, and accountability. Through continuous collaboration with academic institutions, industry partners, and regulatory bodies, LLMDC aims to make significant contributions to the AI landscape, enhancing the way humans and machines communicate.
Large language model services offer powerful AI capabilities to businesses and developers, enabling them to integrate advanced natural language processing (NLP) into their applications and workflows.Â
The largest language model services providers are industry leaders in artificial intelligence, offering advanced NLP solutions that empower businesses across various sectors. Prominent among these providers are OpenAI, Google Cloud, Microsoft Azure, and IBM Watson. OpenAI, renowned for its GPT series, delivers versatile and powerful language models that support a wide range of applications from text generation to complex data analysis. Google Cloud offers its AI and machine learning tools, including BERT and T5 models, which excel in tasks such as translation, sentiment analysis, and more.Â
Microsoft Azure provides Azure Cognitive Services, which leverage models like GPT-3 for diverse applications, including conversational AI and content creation. IBM Watson, with its extensive suite of AI services, offers robust NLP capabilities for enterprises, enabling advanced text analytics and language understanding. These providers lead the way in delivering scalable, reliable, and innovative language model services that transform how businesses interact with and utilize language data.
Expert Custom LLM Development Solutions offer tailored AI capabilities designed to meet the unique needs of businesses across various industries. These solutions provide bespoke development of large language models (LLMs) that are fine-tuned to specific requirements, ensuring optimal performance and relevance. Leveraging deep expertise in natural language processing and machine learning, custom LLM development services can address complex challenges such as industry-specific jargon, regulatory compliance, and specialized content generation.
#Large Language Model Development#large language model services#large language model development company#large language model development services#largest language model services providers#Generative AI and LLM Development Services
0 notes
Text
Simplify Transactions and Boost Efficiency with Our Cash Collection Application
Manual cash collection can lead to inefficiencies and increased risks for businesses. Our cash collection application provides a streamlined solution, tailored to support all business sizes in managing cash effortlessly. Key features include automated invoicing, multi-channel payment options, and comprehensive analytics, all of which simplify the payment process and enhance transparency. The application is designed with a focus on usability and security, ensuring that every transaction is traceable and error-free. With real-time insights and customizable settings, you can adapt the application to align with your business needs. Its robust reporting functions give you a birdâs eye view of financial performance, helping you make data-driven decisions. Move beyond traditional, error-prone cash handling methods and step into the future with a digital approach. With our cash collection application, optimize cash flow and enjoy better financial control at every level of your organization.
#seo agency#seo company#seo marketing#digital marketing#seo services#azure cloud services#amazon web services#ai powered application#android app development#augmented reality solutions#augmented reality in education#augmented reality (ar)#augmented reality agency#augmented reality development services#cash collection application#cloud security services#iot applications#iot#iotsolutions#iot development services#iot platform#digitaltransformation#innovation#techinnovation#iot app development services#large language model services#artificial intelligence#llm#generative ai#ai
4 notes
¡
View notes
Text
CBS Mornings asked the "Godfather of AI" if we should give AI robots rights. He replied he didn't know.
I'll make a prediction on this question right now:
We will very quickly discover we can't give AI robots rights and so we won't.
Why? Because we created AI and robots to do the work humans don't want to do for a fraction of the expense we pay humans to do it. If we throw that all out the window by giving AI robots rights, the technology becomes useless to us. We might as well not have invented it at all. AI robots with rights like humans, are not going to want to be our slaves or sweatshop workers.
I understand we're still in the early stages of AI and robotic technology and it's easy to make the mistake of asking such a question (I have made that mistake myself) but, when we give ourselves time to think about it, it's a question that can only ever have one answer.
No, AI robots will never have or be given rights. Not ever. Guaranteed.
The full interview is below.
youtube
#ai#robots#llm#llm development#cbs mornings#geoffrey hinton#ai rights#ai technology#large language model#science#technology#brook silva- braga#Youtube
1 note
¡
View note
Text
How Do Large Language Model Development Services Assist in Predictive Analytics?
In recent years, the explosion of data and advancements in artificial intelligence (AI) have transformed various industries, enabling organizations to harness the power of data like never before. One of the most groundbreaking developments in AI is the creation and utilization of Large Language Models (LLMs). These models have not only revolutionized natural language processing (NLP) but have also emerged as crucial tools for predictive analytics. In this blog, we will explore how large language model development services assist businesses in enhancing their predictive analytics capabilities.
Understanding Predictive Analytics
Predictive analytics refers to the practice of using historical data, statistical algorithms, and machine learning techniques to identify the likelihood of future outcomes based on past behaviors and trends. Organizations across various sectors, including finance, healthcare, retail, and marketing, leverage predictive analytics to make informed decisions, optimize operations, and improve customer experiences. Traditional predictive analytics methods often rely on structured data, but with the advent of LLMs, organizations can now analyze unstructured data, such as text, to enhance their predictive capabilities.
The Role of Large Language Models
Large Language Models, such as GPT-3 and its successors, are trained on vast datasets containing diverse text sources. These models can understand, generate, and manipulate human language in ways that were previously unimaginable. The key characteristics of LLMs that make them particularly effective in predictive analytics include:
Natural Language Understanding (NLU): LLMs can comprehend context, semantics, and sentiment in language, enabling them to extract meaningful insights from unstructured text data.
Contextual Learning: By processing vast amounts of information, LLMs can recognize patterns and relationships that may not be apparent in traditional datasets, allowing for more accurate predictions.
Generative Capabilities: LLMs can create human-like text, which can be valuable in generating scenarios, forecasts, and narratives based on predictive analysis.
How LLM Development Services Enhance Predictive Analytics
1. Enhanced Data Processing
One of the most significant advantages of LLMs in predictive analytics is their ability to process and analyze unstructured data. Traditional predictive analytics often struggles with data that is not neatly organized in tables or spreadsheets. However, LLMs excel in extracting insights from textual data, such as customer reviews, social media posts, and open-ended survey responses.
LLM development services can create customized models that understand specific terminologies, industry jargon, and user intent, enabling organizations to derive valuable insights from vast amounts of textual data. For example, a retail company can analyze customer feedback to predict trends in consumer behavior, identifying which products are likely to become popular.
2. Improved Accuracy of Predictions
LLMs are trained on extensive datasets, allowing them to recognize patterns and correlations within the data that may go unnoticed by conventional analytics methods. This ability to analyze diverse data sources can lead to more accurate predictions.
By incorporating LLMs into predictive analytics, organizations can enhance their forecasting models. For instance, a financial institution can use LLMs to analyze news articles, social media sentiment, and market trends to predict stock price movements more effectively. The modelâs contextual understanding allows it to incorporate factors that traditional models may overlook, leading to more reliable predictions.
3. Sentiment Analysis and Market Trends
Sentiment analysis is a critical component of predictive analytics, particularly in understanding customer opinions and market trends. LLMs can be employed to analyze sentiment in customer reviews, social media discussions, and news articles, providing valuable insights into public perception.
LLM development services can create models that not only assess sentiment but also correlate it with potential outcomes. For example, a company can analyze customer sentiment regarding a product launch to predict its success. By understanding how customers feel about the product, businesses can make data-driven decisions about marketing strategies and resource allocation.
4. Scenario Simulation and Forecasting
Predictive analytics often involves simulating various scenarios to understand potential outcomes. LLMs can assist in this process by generating text-based scenarios based on historical data and current trends.
For instance, in healthcare, predictive analytics can be used to simulate the spread of diseases based on previous outbreaks and current health data. LLMs can generate narratives that describe potential future scenarios, helping healthcare providers prepare for different outcomes and allocate resources accordingly.
5. Personalized Recommendations
In the realm of e-commerce and marketing, personalized recommendations are crucial for enhancing customer experiences and driving sales. LLMs can analyze customer behavior and preferences to generate personalized recommendations based on predictive analytics.
LLM development services can create tailored models that learn from user interactions, predicting which products or services a customer is likely to be interested in. By leveraging both structured and unstructured data, businesses can provide a more personalized shopping experience, leading to increased customer satisfaction and loyalty.
6. Real-Time Decision Making
In today's fast-paced business environment, organizations need to make decisions quickly. LLMs can facilitate real-time predictive analytics by processing data streams in real-time, allowing businesses to react to emerging trends and changes in customer behavior promptly.
For example, in finance, LLMs can analyze market news and social media in real time to provide instant insights on market fluctuations. This capability enables traders and financial analysts to make informed decisions based on the latest data, enhancing their competitive edge.
7. Integration with Existing Systems
LLM development services can seamlessly integrate large language models into existing predictive analytics frameworks and business systems. This integration allows organizations to leverage the strengths of LLMs while maintaining their established processes.
By connecting LLMs to existing databases and analytics tools, businesses can enhance their predictive capabilities without overhauling their entire systems. This approach enables organizations to transition gradually to more advanced predictive analytics without significant disruptions.
Conclusion
Large Language Models have emerged as powerful tools that significantly enhance predictive analytics capabilities. Their ability to process unstructured data, improve prediction accuracy, analyze sentiment, simulate scenarios, and provide personalized recommendations makes them indispensable for organizations looking to harness the power of data effectively.
As businesses continue to evolve and adapt to a data-driven landscape, the role of LLM development services will become increasingly vital. By investing in LLMs, organizations can not only improve their predictive analytics but also gain a competitive edge in their respective industries. The future of predictive analytics lies in the innovative use of large language models, paving the way for more informed decision-making and enhanced business outcomes.
#Large Language Model Development Services#Large Language Model Development#Large Language Model#LLM#LLM Development#LLM Development Services
0 notes
Text
large language model companies in India
Large Language Model Development Company (LLMDC) is a pioneering organization at the forefront of artificial intelligence research and development. Specializing in the creation and refinement of large language models, LLMDC leverages cutting-edge technologies to push the boundaries of natural language understanding and generation. The company's mission is to develop advanced AI systems that can understand, generate, and interact with human language in a meaningful and contextually relevant manner.Â
With a team of world-class researchers and engineers, LLMDC focuses on a range of applications including automated customer service, content creation, language translation, and more. Their innovations are driven by a commitment to ethical AI development, ensuring that their technologies are not only powerful but also aligned with principles of fairness, transparency, and accountability. Through continuous collaboration with academic institutions, industry partners, and regulatory bodies, LLMDC aims to make significant contributions to the AI landscape, enhancing the way humans and machines communicate.
Large language model services offer powerful AI capabilities to businesses and developers, enabling them to integrate advanced natural language processing (NLP) into their applications and workflows.Â
The largest language model services providers are industry leaders in artificial intelligence, offering advanced NLP solutions that empower businesses across various sectors. Prominent among these providers are OpenAI, Google Cloud, Microsoft Azure, and IBM Watson. OpenAI, renowned for its GPT series, delivers versatile and powerful language models that support a wide range of applications from text generation to complex data analysis. Google Cloud offers its AI and machine learning tools, including BERT and T5 models, which excel in tasks such as translation, sentiment analysis, and more.Â
Microsoft Azure provides Azure Cognitive Services, which leverage models like GPT-3 for diverse applications, including conversational AI and content creation. IBM Watson, with its extensive suite of AI services, offers robust NLP capabilities for enterprises, enabling advanced text analytics and language understanding. These providers lead the way in delivering scalable, reliable, and innovative language model services that transform how businesses interact with and utilize language data.
Expert Custom LLM Development Solutions offer tailored AI capabilities designed to meet the unique needs of businesses across various industries. These solutions provide bespoke development of large language models (LLMs) that are fine-tuned to specific requirements, ensuring optimal performance and relevance. Leveraging deep expertise in natural language processing and machine learning, custom LLM development services can address complex challenges such as industry-specific jargon, regulatory compliance, and specialized content generation.
#Leading LLM Developers#AI Large Language Model Development Company#largest language model services providers#large language model development company
0 notes
Text
How Can Large Language Model Development Services Simplify Legal Document Review?
In the fast-paced world of law, the need for efficiency and accuracy is paramount. Legal professionals are tasked with reviewing vast amounts of documentation, including contracts, case law, and other legal documents. This traditionally labor-intensive process can be daunting and time-consuming. However, advancements in technology, particularly through large language models (LLMs), are revolutionizing the way legal document review is conducted. In this blog, we will explore how LLM development services can simplify legal document review, enhance productivity, and improve the accuracy of legal work.
Understanding Large Language Models
Before delving into their application in the legal sector, it's essential to understand what large language models are. LLMs are advanced artificial intelligence systems trained on massive datasets of text. They utilize deep learning techniques to understand, generate, and manipulate human language. Notable examples include OpenAIâs GPT-3 and GPT-4, which can perform various language tasks such as translation, summarization, question-answering, and more. Their capability to comprehend context, identify nuances, and generate coherent text makes them particularly useful in legal applications.
The Challenges of Legal Document Review
Legal document review involves scrutinizing documents for relevant information, inconsistencies, and compliance with legal standards. It is an essential part of various legal processes, including litigation, contract negotiations, and regulatory compliance. However, several challenges hinder the efficiency of this process:
Volume of Documents: Legal professionals often deal with an overwhelming number of documents. The volume can easily exceed thousands of pages, making manual review not only tedious but also prone to human error.
Complexity of Language: Legal documents are characterized by dense language, complex terminology, and specific jargon. This complexity can lead to misunderstandings and misinterpretations.
Time Constraints: Legal professionals frequently face tight deadlines, necessitating quick and accurate reviews of documents. Rushed reviews can result in missed details that could have significant implications.
Resource Intensity: Reviewing documents requires substantial manpower, which can strain budgets and limit the resources available for other critical tasks.
How LLM Development Services Can Simplify Legal Document Review
1. Automated Document Analysis
LLMs can analyze large volumes of documents in a fraction of the time it would take a human reviewer. By leveraging natural language processing (NLP) capabilities, these models can quickly scan through legal documents, extracting relevant information and identifying key phrases. This automation significantly reduces the workload on legal professionals, allowing them to focus on more strategic tasks that require human judgment and expertise.
2. Contextual Understanding
One of the significant advantages of LLMs is their ability to understand context. Unlike traditional keyword-based search methods, which may overlook nuances, LLMs can interpret the meaning behind the text. This capability is particularly valuable in legal settings where the implications of specific wording can change the document's meaning. For instance, LLMs can discern the difference between âshallâ and âmay,â understanding their legal significance in contract language.
3. Enhanced Search and Retrieval
LLMs can enhance the search and retrieval process for legal documents. They can be trained to recognize and categorize legal terms, concepts, and precedents, making it easier for legal professionals to find relevant information quickly. This improved search capability streamlines the research process, enabling lawyers to access necessary data and cases efficiently.
4. Risk Assessment and Compliance Checking
In legal practice, ensuring compliance with regulations is crucial. LLMs can assist in compliance checking by analyzing documents against relevant laws and regulations. They can flag potential issues or inconsistencies, helping legal professionals mitigate risks associated with non-compliance. This proactive approach not only saves time but also protects clients from legal repercussions.
5. Contract Review and Management
Contracts are a fundamental aspect of legal work, and reviewing them for potential risks and liabilities is essential. LLMs can automate the contract review process by identifying clauses that may be unfavorable or ambiguous. They can also highlight inconsistencies across multiple contracts, ensuring that terms are aligned and compliant. This functionality allows legal teams to manage contracts more effectively and respond to client needs swiftly.
6. Summarization and Comparison
Legal professionals often need to summarize lengthy documents or compare multiple versions of a contract. LLMs can generate concise summaries that capture essential points, enabling lawyers to grasp key information quickly. Additionally, they can compare different versions of documents to identify changes and discrepancies, streamlining the revision process.
7. Cost Efficiency
Implementing LLM development services can lead to significant cost savings for law firms and legal departments. By automating routine tasks, firms can reduce the number of billable hours spent on document review, allowing them to allocate resources more effectively. The time saved on document review can be redirected toward more complex legal work that requires human expertise, enhancing overall productivity.
8. Training and Customization
LLMs can be trained on specific legal corpuses to improve their accuracy and relevance to particular legal contexts. By customizing the model to understand the unique language and practices of a law firm or a specific area of law, organizations can enhance the effectiveness of LLMs in document review processes. Customization allows for improved understanding of specific legal jargon and case law, leading to more accurate results.
Conclusion
The integration of large language model development services into the legal document review process marks a significant advancement in legal technology. By automating routine tasks, enhancing accuracy, and streamlining workflows, LLMs empower legal professionals to work more efficiently and effectively. As the legal landscape continues to evolve, embracing technology will be essential for firms looking to stay competitive and deliver the best possible service to their clients.
With the ongoing development of LLMs and their increasing accessibility, law firms have a unique opportunity to revolutionize their document review processes. By leveraging these powerful tools, legal professionals can not only simplify their workflows but also enhance their decision-making capabilities, ultimately leading to better outcomes for clients and the legal industry as a whole.
0 notes
Text
Because I'm wondering what happens to the human brain between birth and adulthood and adultier adulthood, I'm tempted to get into the neuroscience of human development, how we become and continue to become who we are. Cortexes. Neurons. Axons. Synapses. Receptors and Neurotransmitters. But I'm afraid I'll get stuck in a metaphor that involves switchboards and switchboard operators from which there's no escape.Â
I just don't know enough to handle the explanation elegantly.
However.
I did go to school. Elementary. Junior. Senior. Two degrees across five years at the university. A year and a half studying the music and video industry, the business side and the production side for an Associate of Arts chaser.
I'm a product of a traditional education model who's currently integrating AI tools into that body of knowledge.
So.
My question yesterday was a riff on whether AI tools might be enough in the absence of that body of knowledge, that education, or some reduction of it.
Is there a difference between understanding a thing and retrieving that knowledge on the fly through a Large Language Model in the moment you need that knowledge?
In short: what need is there for general knowledge in our minds when we're carrying around the sum total of all human knowledge in our pockets?
Are we at a point where we don't need to know as much as we used to? To carry that amount in our minds?
So we can read less?
So we can write less?
So we can study less.
Which, let's be honest, we already do less reading and writing in comparison to previous generations.
Is there a price to leaving those skills behind or are we good to go boldly and successfully into the future?
Hmmm.
Okay so I do wanna get into the neuroscience and that switchboard/switchboard operators metaphor because what we learn, how we learn it, and the degree to which we study it biologically wires our brains in specific ways, biologically optimizes our brains in specific ways. Our required and chosen mental activities from infant to toddler to child to pre-teen to teen fire certain neurons and not others. Our required and chosen mental activities muscles up certain pathways, connections between certain neurons and not others. All of which all of which all of which has implications to the people we are and the professionals we become.
How?
We'll start that piece tomorrow...
#brain#mind#birth#adulthood#neuroscience#human development#neurons#wiring#switchboard#AI#knowledge on demand#understanding#knowing#knowledge#large language model#general knowledge#neural networks
0 notes
Text
Learn about Microsoft Security Copilot
Microsoft Security Copilot (Security Copilot) is a generative AI-powered security solution that helps increase the efficiency and capabilities of defenders to improve security outcomes at machine speed and scale, while remaining compliant to responsible AI principles. Introducing Microsoft Security Copilot: Learn how the Microsoft Security Copilot works. Learn how Security Copilot combines anâŚ
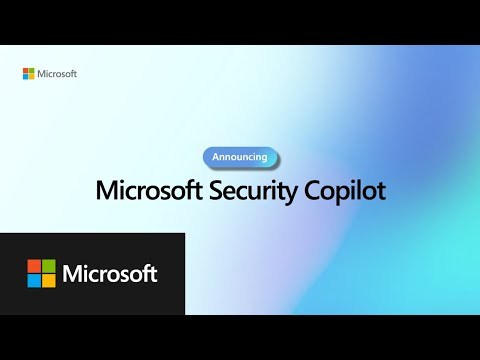
View On WordPress
#AI#assistive copilot#copilot#Defender#Develop whats next#Developer#development#generative AI#Getting Started#incident response#intelligence gathering#intune#investigate#kusto query language#Large language model#llm#Microsoft Entra#natural language#OpenAI#plugin#posture management#prompt#Security#security copilot#security professional#Sentinel#threat#Threat Intelligence#What is New ?
0 notes
Text
There is no such thing as AI.
How to help the non technical and less online people in your life navigate the latest techbro grift.
I've seen other people say stuff to this effect but it's worth reiterating. Today in class, my professor was talking about a news article where a celebrity's likeness was used in an ai image without their permission. Then she mentioned a guest lecture about how AI is going to help finance professionals. Then I pointed out, those two things aren't really related.
The term AI is being used to obfuscate details about multiple semi-related technologies.
Traditionally in sci-fi, AI means artificial general intelligence like Data from star trek, or the terminator. This, I shouldn't need to say, doesn't exist. Techbros use the term AI to trick investors into funding their projects. It's largely a grift.
What is the term AI being used to obfuscate?
If you want to help the less online and less tech literate people in your life navigate the hype around AI, the best way to do it is to encourage them to change their language around AI topics.
By calling these technologies what they really are, and encouraging the people around us to know the real names, we can help lift the veil, kill the hype, and keep people safe from scams. Here are some starting points, which I am just pulling from Wikipedia. I'd highly encourage you to do your own research.
Machine learning (ML): is an umbrella term for solving problems for which development of algorithms by human programmers would be cost-prohibitive, and instead the problems are solved by helping machines "discover" their "own" algorithms, without needing to be explicitly told what to do by any human-developed algorithms. (This is the basis of most technologically people call AI)
Language model: (LM or LLM) is a probabilistic model of a natural language that can generate probabilities of a series of words, based on text corpora in one or multiple languages it was trained on. (This would be your ChatGPT.)
Generative adversarial network (GAN): is a class of machine learning framework and a prominent framework for approaching generative AI. In a GAN, two neural networks contest with each other in the form of a zero-sum game, where one agent's gain is another agent's loss. (This is the source of some AI images and deepfakes.)
Diffusion Models: Models that generate the probability distribution of a given dataset. In image generation, a neural network is trained to denoise images with added gaussian noise by learning to remove the noise. After the training is complete, it can then be used for image generation by starting with a random noise image and denoise that. (This is the more common technology behind AI images, including Dall-E and Stable Diffusion. I added this one to the post after as it was brought to my attention it is now more common than GANs.)
I know these terms are more technical, but they are also more accurate, and they can easily be explained in a way non-technical people can understand. The grifters are using language to give this technology its power, so we can use language to take it's power away and let people see it for what it really is.
12K notes
¡
View notes
Text
âI can now say with absolute confidence that many AI systems have been trained on TV and film writersâ work. Not just on The Godfather and Alf, but on more than 53,000 other movies and 85,000 other TV episodes: Dialogue from all of it is included in an AI-training data set that has been used by Apple, Anthropic, Meta, Nvidia, Salesforce, Bloomberg, and other companies. I recently downloaded this data set, which I saw referenced in papers about the development of various large language models (or LLMs). It includes writing from every film nominated for Best Picture from 1950 to 2016, at least 616 episodes of The Simpsons, 170 episodes of Seinfeld, 45 episodes of Twin Peaks, and every episode of The Wire, The Sopranos, and Breaking Bad.â
đĄ
2K notes
¡
View notes
Text
The TechTrendGuide bot on Poe.com takes a technology as input and describes it using language relevant to and understandable by a typical business user.
Great for:
Website content
Social media
Training
Learning & development
Onboarding
Continuing Education
Lemonbarski Labs by Steven Lewandowski is the Generative AI Prompt Engineer of CorporateBots on POE | Created on the POE platform by Quora | Utilizes GPT-3 Large Language Model Courtesy of OpenAI | https://lemonbarski.com | https://Stevenlewandowski.us | Where applicable, copyright 2023 Lemonbarski Labs by Steven Lewandowski
Steven Lewandowski is a creative, curious, & collaborative marketer, researcher, developer, activist, & entrepreneur based in Chicago, IL, USA
Find Steven Lewandowski on social media by visiting https://Stevenlewandowski.us/connect | Learn more at https://Steven.Lemonbarski.com or https://stevenlewandowski.us
#chicago#lemonbarski#app developers#poe ai#artificial intelligence#machine learning#large language model#chatgpt#openai#small business#business#competitive intelligence
0 notes
Note
nightshade is basically useless https://www.tumblr.com/billclintonsbeefarm/740236576484999168/even-if-you-dont-like-generative-models-this
I'm not a developer, but the creators of Nightshade do address some of this post's concerns in their FAQ. Obviously it's not a magic bullet to prevent AI image scraping, and obviously there's an arms race between AI developers and artists attempting to disrupt their data pools. But personally, I think it's an interesting project and is accessible to most people to try. Giving up on it at this stage seems really premature.
But if it's caption data that's truly valuable, Tumblr is an ... interesting ... place to be scraping it from. For one thing, users tend to get pretty creative with both image descriptions and tags. For another, I hope whichever bot scrapes my blog enjoys the many bird photos I have described as "Cheese." Genuinely curious if Tumblr data is actually valuable or if it's garbage.
That said, I find it pretty ironic that the OP of the post you linked seems to think nightshade and glaze specifically are an unreasonable waste of electricity. Both are software. Your personal computer's graphics card is doing the work, not an entire data center, so if your computer was going to be on anyway, the cost is a drop in the bucket compared to what AI generators are consuming.
Training a large language model like GPT-3, for example, is estimated to use just under 1,300 megawatt hours (MWh) of electricity; about as much power as consumed annually by 130 US homes. To put that in context, streaming an hour of Netflix requires around 0.8 kWh (0.0008 MWh) of electricity. That means youâd have to watch 1,625,000 hours to consume the same amount of power it takes to train GPT-3. (source)
So, no, I don't think Nightshade or Glaze are useless just because they aren't going to immediately topple every AI image generator. There's not really much downside for the artists interested in using them so I hope they continue development.
992 notes
¡
View notes