#and load (ETL)
Explore tagged Tumblr posts
Text
What sets Konnect Insights apart from other data orchestration and analysis tools available in the market for improving customer experiences in the aviation industry?
I can highlight some general factors that may set Konnect Insights apart from other data orchestration and analysis tools available in the market for improving customer experiences in the aviation industry. Keep in mind that the competitive landscape and product offerings may have evolved since my last knowledge update. Here are some potential differentiators:
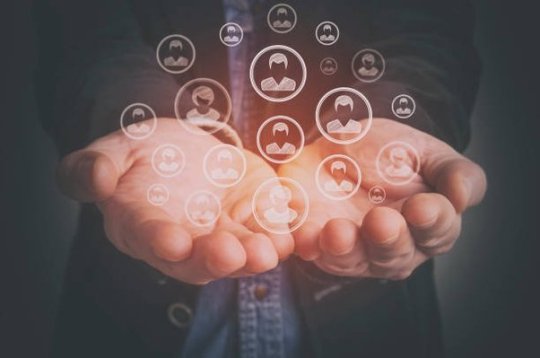
Aviation Industry Expertise: Konnect Insights may offer specialized features and expertise tailored to the unique needs and challenges of the aviation industry, including airports, airlines, and related businesses.
Multi-Channel Data Integration: Konnect Insights may excel in its ability to integrate data from a wide range of sources, including social media, online platforms, offline locations within airports, and more. This comprehensive data collection can provide a holistic view of the customer journey.
Real-Time Monitoring: The platform may provide real-time monitoring and alerting capabilities, allowing airports to respond swiftly to emerging issues or trends and enhance customer satisfaction.
Customization: Konnect Insights may offer extensive customization options, allowing airports to tailor the solution to their specific needs, adapt to unique workflows, and focus on the most relevant KPIs.
Actionable Insights: The platform may be designed to provide actionable insights and recommendations, guiding airports on concrete steps to improve the customer experience and operational efficiency.
Competitor Benchmarking: Konnect Insights may offer benchmarking capabilities that allow airports to compare their performance to industry peers or competitors, helping them identify areas for differentiation.
Security and Compliance: Given the sensitive nature of data in the aviation industry, Konnect Insights may include robust security features and compliance measures to ensure data protection and adherence to industry regulations.
Scalability: The platform may be designed to scale effectively to accommodate the data needs of large and busy airports, ensuring it can handle high volumes of data and interactions.
Customer Support and Training: Konnect Insights may offer strong customer support, training, and consulting services to help airports maximize the value of the platform and implement best practices for customer experience improvement.
Integration Capabilities: It may provide seamless integration with existing airport systems, such as CRM, ERP, and database systems, to ensure data interoperability and process efficiency.
Historical Analysis: The platform may enable airports to conduct historical analysis to track the impact of improvements and initiatives over time, helping measure progress and refine strategies.
User-Friendly Interface: Konnect Insights may prioritize a user-friendly and intuitive interface, making it accessible to a wide range of airport staff without requiring extensive technical expertise.
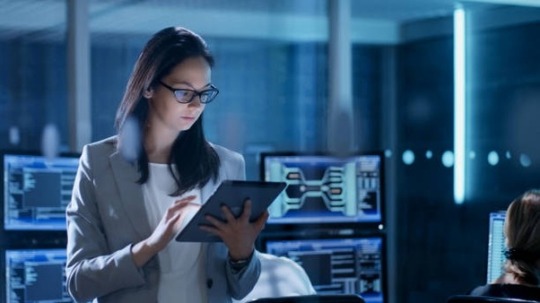
It's important for airports and organizations in the aviation industry to thoroughly evaluate their specific needs and conduct a comparative analysis of available solutions to determine which one aligns best with their goals and requirements. Additionally, staying updated with the latest developments and customer feedback regarding Konnect Insights and other similar tools can provide valuable insights when making a decision.
#DataOrchestration#DataManagement#DataOps#DataIntegration#DataEngineering#DataPipeline#DataAutomation#DataWorkflow#ETL (Extract#Transform#Load)#DataIntegrationPlatform#BigData#CloudComputing#Analytics#DataScience#AI (Artificial Intelligence)#MachineLearning#IoT (Internet of Things)#DataGovernance#DataQuality#DataSecurity
2 notes
¡
View notes
Text
What is ETL and why it is important | PiLog iTransform â ETLÂ
ETL (Extract Transform Load) - integrating data through extraction, transformation, loading into warehouse. Explore best ETL tools, testing, software, services. https://www.piloggroup.com/itransform-ETL.phpÂ
#Extract Transform Load#explore best ETL tools#ETL testing services#ETL software#ETL services#Best ETL Services
0 notes
Text
Seamless Data Integration with IntelliData Labs: ETL Excellence Redefined
Experience seamless data integration with IntelliData Labs' expert ETL services. Our specialized approach to extract, transform, and load (ETL) processes ensures the efficient transfer of data between systems, enabling real-time insights and informed decision-making. Whether you're migrating data, consolidating sources, or building data warehouses, our team delivers reliable ETL solutions tailored to your unique requirements, driving operational efficiency and accelerating business growth.
0 notes
Text
Explore the impactful use cases of Airbyte in the fintech industry, from centralizing customer data for enhanced insights to real-time fraud detection and ensuring regulatory compliance. Learn how Airbyte drives operational efficiency by streamlining data integration across various fintech applications, providing businesses with actionable insights and improved processes.
Know more at: https://bit.ly/3UbqGyT
#Fintech#data analytics#data engineering#technology#Airbyte#ETL#ELT#Cloud data#Data Integration#Data Transformation#Data management#Data extraction#Data Loading#Tech videos
0 notes
Text
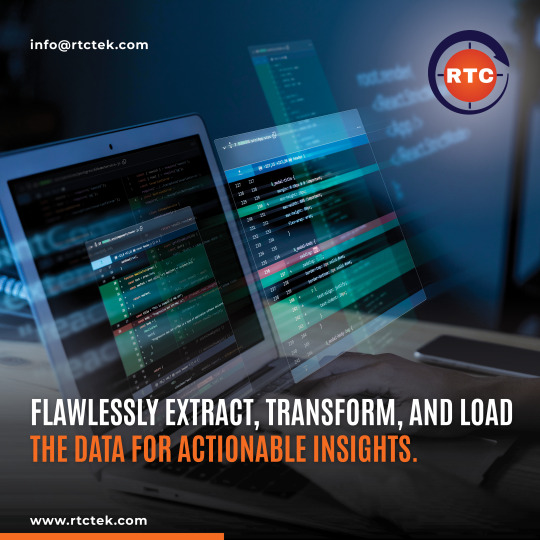
At the core of our engineering services lies our commitment to ETL (Extract, Transform, Load) excellence. This involves seamlessly extracting data from diverse sources, including databases, cloud storage, and streaming platforms, APIs, and IoT devices. Once extracted, we meticulously transform the data into actionable insights by cleaning, formatting, and enriching it to ensure accuracy and relevance. This transformation process also involves advanced techniques such as data aggregation and normalization to enhance the quality of the dataset. Finally, we efficiently load the transformed data into the systems or databases, selecting the appropriate storage infrastructure and optimizing the loading process for speed and reliability. Our ETL excellence approach ensures that the data is handled with precision and care, resulting in valuable insights that drive informed decision-making and business success.
Learn more about services at https://rtctek.com/data-engineering-services/ Contact us at https://rtctek.com/contact-us/
#etl#extract#transform#load#dataintegration#dataengineering#dataloading#dataextraction#datatransformation#datawarehousing#dataanalytics#bigdata#dataquality#dataarchitecture#datamigration
0 notes
Text
Lets cook (ETL, ELT and ET)!!!
Lets cook (ETL, ELT and ET)!!!
Not long ago we were all enthralled to see huge amounts of data being transformed into desirable beautiful graphs basing on which multi dollar companies are taking their business decisions. But, has it ever occurred how this is being done, I can hardly guarantee people who came across this article already have a clue about the terms ETL, ELT and ET. The methodologies, techniques that are involvedâŚ
View On WordPress
0 notes
Text
Haladriel Positivity Post #2
Okay my darlings, my dears! Haladriel has changed our brain chemistry, clearly. For the better?? I think so. This post is a shout out to the fandom for changing my very outlook on guilt and morality and art. Because of the creative works from amazing haladriel writers, artists, and editors, I went from âno thank you I will certainly NOT be having a helping of any dove, dead or otherwise. Or kink. Or abo. Or or orâŚâ Seriously, my bookmarks on ao3 were so tame. Because to me, ETL and Haladriel made loads of sense as a brand of ship that had nothing to do with my moral standing.
They were more than the sum of their parts. He wasnât just evil, she wasnât just good. They were a mess of squishy complicated emotions and parts. (And none more squishier than you, gooron post-Adar slaying, amirite?)
But I turned my nose up at a whole heap of other things even as I loved these two crazy kids/immortal beings. Then I dove deeper into fandom and discovered that there were whole worlds I had closed off. And those worlds were shockingly good.
Because if I can enjoy âSauron shoving the ultimate Morgul blade into Galadrielâs chest in an erotically charged manner and then getting very lip wobbly emo about it afterwardsâ, then I can also read and write and view a passel of things that force me to look (or that tilt my chin up gently with a sword point â potato potato) at things that push my boundaries.
So thank you to all who create literally anything for this fandom but in particular today to those who love to experiment with pushing buttons and boundaries.
Bonus: Some recs! And please if youâre reading this feel free to share your own if so inclined. I love encountering new things and being reminded of things to revisit!
This fic, âthe law is reason (free from passion)â by @hazelmaines was I think my first abo fic ever? I would read hazelâs grocery list and find it enthralling so I gave it a shot and to my surprise was fascinated by the world building involved with abo and wowee did it bring the hot sauce when it came to haladriel as a pairing. Lawyers with voracious appetites for each other? Sign me up. I didnât even burst into a pillar of flame afterwards (or I mean, I did but not like thatâŚ)
https://archiveofourown.org/works/50193469
Next up is the BDSM fic series that rewired my brain by @softlighter the one, the only âcatharsisâ. Reading about a powerful woman learning to accept herself for who she is and maybe even love what she sees in the end(??) is something that makes me weep and broke a lot of barriers for me, including realizing I wasnât going to turn into a pillar of ash or salt or whatever other biblical metaphor you like from reading it. Whoever recommended this, I want to kiss you right on the mouth.
https://archiveofourown.org/works/47895979
Getting into the dead dove side of things, nothing lives rent free in my noggin and made me think about how art can be disturbing and beautiful and incredibly effective at making you look inward at the same time like âthe shark in your waterâ by @bad-surprise. Holy wow. Poeticmemoryâs Halbrands always fascinate me but this one is the embodiment of that one shot in that one haladriel scene in that one gif đwhere I absolutely cannot look away and feel every emotion in the book
Treat yourself with care if you decide to try this treat of a fic.
https://archiveofourown.org/works/45286258
I am still absolutely blown away by the two most recent works Iâm including in this list. The first is this beautifully disturbing edit by @snezhjeyka set to alt-jâs âbreezeblocksâ. It is the definition of lyrical and haunting.
The second is the fic it later inspired by @czernyfanclub aka bluececilia on ao3. (That dedication makes me weep, pal đ) The dove is dead as all get-out and yet the language?? The imagery?? As admirable as old Mairon himself.
Ok. Thatâs it for now! Iâll try to post again whenever I have another brainwave of what I love about this fandom, because damn do I love what it has done for me personally and for so many others.
Eat, Pray, ETL. Or something.
22 notes
¡
View notes
Text
War Prisoners one-shot
âWhat gives, Malfoy? What the hell did you do, to end up here with little olâ us?â Malfoy glanced over with disdain. Hermioneâs heart skipped a beat as his steely eyes passed across her and settled on Ron and Harry. âAs if Iâm going to tell you.â â'Lord Malfoy,'â Ron muttered, loud enough for all of them. âWhat a load of bollocks. Although âLord Malfoy, of the cornermost cell,â does have a certain ring to it.â ---- The trio languishes in the dungeons, waiting for whatever comes next - but that turns out to be a new prisoner. The unexpected and mysterious arrival of a swaggering Draco Malfoy raises tensions. Some are easier to relieve than others.
War prisoners, reverse ETL - rated E.
AO3 account required.
#dramione#fanfiction#draco malfoy#draco x hermione#ao3 fanfic#hermione granger#smut#hea#hermione x draco#dhr fanfiction#one shot
13 notes
¡
View notes
Text
Data warehousing solution
Unlocking the Power of Data Warehousing: A Key to Smarter Decision-Making
In today's data-driven world, businesses need to make smarter, faster, and more informed decisions. But how can companies achieve this? One powerful tool that plays a crucial role in managing vast amounts of data is data warehousing. In this blog, weâll explore what data warehousing is, its benefits, and how it can help organizations make better business decisions.
What is Data Warehousing?
At its core, data warehousing refers to the process of collecting, storing, and managing large volumes of data from different sources in a central repository. The data warehouse serves as a consolidated platform where all organizational dataâwhether from internal systems, third-party applications, or external sourcesâcan be stored, processed, and analyzed.
A data warehouse is designed to support query and analysis operations, making it easier to generate business intelligence (BI) reports, perform complex data analysis, and derive insights for better decision-making. Data warehouses are typically used for historical data analysis, as they store data from multiple time periods to identify trends, patterns, and changes over time.
Key Components of a Data Warehouse
To understand the full functionality of a data warehouse, it's helpful to know its primary components:
Data Sources: These are the various systems and platforms where data is generated, such as transactional databases, CRM systems, or external data feeds.
ETL (Extract, Transform, Load): This is the process by which data is extracted from different sources, transformed into a consistent format, and loaded into the warehouse.
Data Warehouse Storage: The central repository where cleaned, structured data is stored. This can be in the form of a relational database or a cloud-based storage system, depending on the organizationâs needs.
OLAP (Online Analytical Processing): This allows for complex querying and analysis, enabling users to create multidimensional data models, perform ad-hoc queries, and generate reports.
BI Tools and Dashboards: These tools provide the interfaces that enable users to interact with the data warehouse, such as through reports, dashboards, and data visualizations.
Benefits of Data Warehousing
Improved Decision-Making: With data stored in a single, organized location, businesses can make decisions based on accurate, up-to-date, and complete information. Real-time analytics and reporting capabilities ensure that business leaders can take swift action.
Consolidation of Data: Instead of sifting through multiple databases or systems, employees can access all relevant data from one location. This eliminates redundancy and reduces the complexity of managing data from various departments or sources.
Historical Analysis: Data warehouses typically store historical data, making it possible to analyze long-term trends and patterns. This helps businesses understand customer behavior, market fluctuations, and performance over time.
Better Reporting: By using BI tools integrated with the data warehouse, businesses can generate accurate reports on key metrics. This is crucial for monitoring performance, tracking KPIs (Key Performance Indicators), and improving strategic planning.
Scalability: As businesses grow, so does the volume of data they collect. Data warehouses are designed to scale easily, handling increasing data loads without compromising performance.
Enhanced Data Quality: Through the ETL process, data is cleaned, transformed, and standardized. This means the data stored in the warehouse is of high qualityâconsistent, accurate, and free of errors.
Types of Data Warehouses
There are different types of data warehouses, depending on how they are set up and utilized:
Enterprise Data Warehouse (EDW): An EDW is a central data repository for an entire organization, allowing access to data from all departments or business units.
Operational Data Store (ODS): This is a type of data warehouse that is used for storing real-time transactional data for short-term reporting. An ODS typically holds data that is updated frequently.
Data Mart: A data mart is a subset of a data warehouse focused on a specific department, business unit, or subject. For example, a marketing data mart might contain data relevant to marketing operations.
Cloud Data Warehouse: With the rise of cloud computing, cloud-based data warehouses like Google BigQuery, Amazon Redshift, and Snowflake have become increasingly popular. These platforms allow businesses to scale their data infrastructure without investing in physical hardware.
How Data Warehousing Drives Business Intelligence
The purpose of a data warehouse is not just to store data, but to enable businesses to extract valuable insights. By organizing and analyzing data, businesses can uncover trends, customer preferences, and operational inefficiencies. Some of the ways in which data warehousing supports business intelligence include:
Customer Segmentation: Companies can analyze data to segment customers based on behavior, demographics, or purchasing patterns, leading to better-targeted marketing efforts.
Predictive Analytics: By analyzing historical data, businesses can forecast trends and predict future outcomes, such as sales, inventory needs, and staffing levels.
Improved Operational Efficiency: With data-driven insights, businesses can streamline processes, optimize supply chains, and reduce costs. For example, identifying inventory shortages or surplus can help optimize stock levels.
Challenges in Data Warehousing
While the benefits of data warehousing are clear, there are some challenges to consider:
Complexity of Implementation: Setting up a data warehouse can be a complex and time-consuming process, requiring expertise in database management, ETL processes, and BI tools.
Data Integration: Integrating data from various sources with differing formats can be challenging, especially when dealing with legacy systems or unstructured data.
Cost: Building and maintaining a data warehouse can be expensive, particularly when managing large volumes of data. However, the investment is often worth it in terms of the business value generated.
Security: With the consolidation of sensitive data in one place, data security becomes critical. Organizations need robust security measures to prevent unauthorized access and ensure compliance with data protection regulations.
The Future of Data Warehousing
The world of data warehousing is constantly evolving. With advancements in cloud technology, machine learning, and artificial intelligence, businesses are now able to handle larger datasets, perform more sophisticated analyses, and automate key processes.
As companies increasingly embrace the concept of a "data-driven culture," the need for powerful data warehousing solutions will continue to grow. The integration of AI-driven analytics, real-time data processing, and more intuitive BI tools will only further enhance the value of data warehouses in the years to come.
Conclusion
In todayâs fast-paced, data-centric world, having access to accurate, high-quality data is crucial for making informed business decisions. A robust data warehousing solution enables businesses to consolidate, analyze, and extract valuable insights from their data, driving smarter decision-making across all departments. While building a data warehouse comes with challenges, the benefitsâimproved efficiency, better decision-making, and enhanced business intelligenceâmake it an essential tool for modern organizations.
You said:
blog for Data warehousing
ChatGPT said:
Title: Data Warehousing: The Backbone of Data-Driven Decision Making
In todayâs fast-paced business environment, the ability to make data-driven decisions quickly is paramount. However, to leverage data effectively, companies need more than just raw data. They need a centralized, structured system that allows them to store, manage, and analyze data seamlessly. This is where data warehousing comes into play.
Data warehousing has become the cornerstone of modern business intelligence (BI) systems, enabling organizations to unlock valuable insights from vast amounts of data. In this blog, weâll explore what data warehousing is, why itâs important, and how it drives smarter decision-making.
What is Data Warehousing?
At its core, data warehousing refers to the process of collecting and storing data from various sources into a centralized system where it can be easily accessed and analyzed. Unlike traditional databases, which are optimized for transactional operations (i.e., data entry, updating), data warehouses are designed specifically for complex queries, reporting, and data analysis.
A data warehouse consolidates data from various sourcesâsuch as customer information systems, financial systems, and even external data feedsâinto a single repository. The data is then structured and organized in a way that supports business intelligence (BI) tools, enabling organizations to generate reports, create dashboards, and gain actionable insights.
Key Components of a Data Warehouse
Data Sources: These are the different systems or applications that generate data. Examples include CRM systems, ERP systems, external APIs, and transactional databases.
ETL (Extract, Transform, Load): This is the process by which data is pulled from different sources (Extract), cleaned and converted into a usable format (Transform), and finally loaded into the data warehouse (Load).
Data Warehouse Storage: The actual repository where structured and organized data is stored. This could be in traditional relational databases or modern cloud-based storage platforms.
OLAP (Online Analytical Processing): OLAP tools enable users to run complex analytical queries on the data warehouse, creating reports, performing multidimensional analysis, and identifying trends.
Business Intelligence Tools: These tools are used to interact with the data warehouse, generate reports, visualize data, and help businesses make data-driven decisions.
Benefits of Data Warehousing
Improved Decision Making: By consolidating data into a single repository, decision-makers can access accurate, up-to-date information whenever they need it. This leads to more informed, faster decisions based on reliable data.
Data Consolidation: Instead of pulling data from multiple systems and trying to make sense of it, a data warehouse consolidates data from various sources into one place, eliminating the complexity of handling scattered information.
Historical Analysis: Data warehouses are typically designed to store large amounts of historical data. This allows businesses to analyze trends over time, providing valuable insights into long-term performance and market changes.
Increased Efficiency: With a data warehouse in place, organizations can automate their reporting and analytics processes. This means less time spent manually gathering data and more time focusing on analyzing it for actionable insights.
Better Reporting and Insights: By using data from a single, trusted source, businesses can produce consistent, accurate reports that reflect the true state of affairs. BI tools can transform raw data into meaningful visualizations, making it easier to understand complex trends.
Types of Data Warehouses
Enterprise Data Warehouse (EDW): This is a centralized data warehouse that consolidates data across the entire organization. Itâs used for comprehensive, organization-wide analysis and reporting.
Data Mart: A data mart is a subset of a data warehouse that focuses on specific business functions or departments. For example, a marketing data mart might contain only marketing-related data, making it easier for the marketing team to access relevant insights.
Operational Data Store (ODS): An ODS is a database that stores real-time data and is designed to support day-to-day operations. While a data warehouse is optimized for historical analysis, an ODS is used for operational reporting.
Cloud Data Warehouse: With the rise of cloud computing, cloud-based data warehouses like Amazon Redshift, Google BigQuery, and Snowflake have become popular. These solutions offer scalable, cost-effective, and flexible alternatives to traditional on-premises data warehouses.
How Data Warehousing Supports Business Intelligence
A data warehouse acts as the foundation for business intelligence (BI) systems. BI tools, such as Tableau, Power BI, and QlikView, connect directly to the data warehouse, enabling users to query the data and generate insightful reports and visualizations.
For example, an e-commerce company can use its data warehouse to analyze customer behavior, sales trends, and inventory performance. The insights gathered from this analysis can inform marketing campaigns, pricing strategies, and inventory management decisions.
Here are some ways data warehousing drives BI and decision-making:
Customer Insights: By analyzing customer purchase patterns, organizations can better segment their audience and personalize marketing efforts.
Trend Analysis: Historical data allows companies to identify emerging trends, such as seasonal changes in demand or shifts in customer preferences.
Predictive Analytics: By leveraging machine learning models and historical data stored in the data warehouse, companies can forecast future trends, such as sales performance, product demand, and market behavior.
Operational Efficiency: A data warehouse can help identify inefficiencies in business operations, such as bottlenecks in supply chains or underperforming products.
2 notes
¡
View notes
Text
next time I'm asked to tell someone a little bit about myself, I'm going to explain how when I was organizing my computer I found a file labeled âetl pipeline" on my desktop, which confused me â what might I have written about a data pipeline and titled so unhelpfully?? turns out I had used etl as an abbreviation for âenemies to lovers,â not âextract, transform, and loadâ
#i feel like this anecdote covers the important facts about me#fact one: data nerd#fact two: too into fandom#it was from long enough ago that I don't even remember if I'd intended the filename to be a joke#oh computer
12 notes
¡
View notes
Text
AI Frameworks Help Data Scientists For GenAI Survival
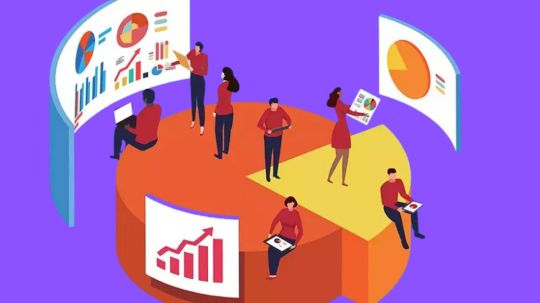
AI Frameworks: Crucial to the Success of GenAI
Develop Your AI Capabilities Now
You play a crucial part in the quickly growing field of generative artificial intelligence (GenAI) as a data scientist. Your proficiency in data analysis, modeling, and interpretation is still essential, even though platforms like Hugging Face and LangChain are at the forefront of AI research.
Although GenAI systems are capable of producing remarkable outcomes, they still mostly depend on clear, organized data and perceptive interpretation areas in which data scientists are highly skilled. You can direct GenAI models to produce more precise, useful predictions by applying your in-depth knowledge of data and statistical techniques. In order to ensure that GenAI systems are based on strong, data-driven foundations and can realize their full potential, your job as a data scientist is crucial. Hereâs how to take the lead:
Data Quality Is Crucial
The effectiveness of even the most sophisticated GenAI models depends on the quality of the data they use. By guaranteeing that the data is relevant, AI tools like Pandas and Modin enable you to clean, preprocess, and manipulate large datasets.
Analysis and Interpretation of Exploratory Data
It is essential to comprehend the features and trends of the data before creating the models. Data and model outputs are visualized via a variety of data science frameworks, like Matplotlib and Seaborn, which aid developers in comprehending the data, selecting features, and interpreting the models.
Model Optimization and Evaluation
A variety of algorithms for model construction are offered by AI frameworks like scikit-learn, PyTorch, and TensorFlow. To improve models and their performance, they provide a range of techniques for cross-validation, hyperparameter optimization, and performance evaluation.
Model Deployment and Integration
Tools such as ONNX Runtime and MLflow help with cross-platform deployment and experimentation tracking. By guaranteeing that the models continue to function successfully in production, this helps the developers oversee their projects from start to finish.
Intelâs Optimized AI Frameworks and Tools
The technologies that developers are already familiar with in data analytics, machine learning, and deep learning (such as Modin, NumPy, scikit-learn, and PyTorch) can be used. For the many phases of the AI process, such as data preparation, model training, inference, and deployment, Intel has optimized the current AI tools and AI frameworks, which are based on a single, open, multiarchitecture, multivendor software platform called oneAPI programming model.
Data Engineering and Model Development:
To speed up end-to-end data science pipelines on Intel architecture, use Intelâs AI Tools, which include Python tools and frameworks like Modin, Intel Optimization for TensorFlow Optimizations, PyTorch Optimizations, IntelExtension for Scikit-learn, and XGBoost.
Optimization and Deployment
For CPU or GPU deployment, Intel Neural Compressor speeds up deep learning inference and minimizes model size. Models are optimized and deployed across several hardware platforms including Intel CPUs using the OpenVINO toolbox.
You may improve the performance of your Intel hardware platforms with the aid of these AI tools.
Library of Resources
Discover collection of excellent, professionally created, and thoughtfully selected resources that are centered on the core data science competencies that developers need. Exploring machine and deep learning AI frameworks.
What you will discover:
Use Modin to expedite the extract, transform, and load (ETL) process for enormous DataFrames and analyze massive datasets.
To improve speed on Intel hardware, use Intelâs optimized AI frameworks (such as Intel Optimization for XGBoost, Intel Extension for Scikit-learn, Intel Optimization for PyTorch, and Intel Optimization for TensorFlow).
Use Intel-optimized software on the most recent Intel platforms to implement and deploy AI workloads on Intel Tiber AI Cloud.
How to Begin
Frameworks for Data Engineering and Machine Learning
Step 1: View the Modin, Intel Extension for Scikit-learn, and Intel Optimization for XGBoost videos and read the introductory papers.
Modin: To achieve a quicker turnaround time overall, the video explains when to utilize Modin and how to apply Modin and Pandas judiciously. A quick start guide for Modin is also available for more in-depth information.
Scikit-learn Intel Extension: This tutorial gives you an overview of the extension, walks you through the code step-by-step, and explains how utilizing it might improve performance. A movie on accelerating silhouette machine learning techniques, PCA, and K-means clustering is also available.
Intel Optimization for XGBoost: This straightforward tutorial explains Intel Optimization for XGBoost and how to use Intel optimizations to enhance training and inference performance.
Step 2: Use Intel Tiber AI Cloud to create and develop machine learning workloads.
On Intel Tiber AI Cloud, this tutorial runs machine learning workloads with Modin, scikit-learn, and XGBoost.
Step 3: Use Modin and scikit-learn to create an end-to-end machine learning process using census data.
Run an end-to-end machine learning task using 1970â2010 US census data with this code sample. The code sample uses the Intel Extension for Scikit-learn module to analyze exploratory data using ridge regression and the Intel Distribution of Modin.
Deep Learning Frameworks
Step 4: Begin by watching the videos and reading the introduction papers for Intelâs PyTorch and TensorFlow optimizations.
Intel PyTorch Optimizations: Read the article to learn how to use the Intel Extension for PyTorch to accelerate your workloads for inference and training. Additionally, a brief video demonstrates how to use the addon to run PyTorch inference on an Intel Data Center GPU Flex Series.
Intelâs TensorFlow Optimizations: The article and video provide an overview of the Intel Extension for TensorFlow and demonstrate how to utilize it to accelerate your AI tasks.
Step 5: Use TensorFlow and PyTorch for AI on the Intel Tiber AI Cloud.
In this article, it show how to use PyTorch and TensorFlow on Intel Tiber AI Cloud to create and execute complicated AI workloads.
Step 6: Speed up LSTM text creation with Intel Extension for TensorFlow.
The Intel Extension for TensorFlow can speed up LSTM model training for text production.
Step 7: Use PyTorch and DialoGPT to create an interactive chat-generation model.
Discover how to use Hugging Faceâs pretrained DialoGPT model to create an interactive chat model and how to use the Intel Extension for PyTorch to dynamically quantize the model.
Read more on Govindhtech.com
#AI#AIFrameworks#DataScientists#GenAI#PyTorch#GenAISurvival#TensorFlow#CPU#GPU#IntelTiberAICloud#News#Technews#Technology#Technologynews#Technologytrends#govindhtech
2 notes
¡
View notes
Text

ETL (Extraction, Transformation, Loading) Explained
Having trouble reading infographic here?
Check out the full size infographic at - https://infographicjournal.com/etl-extraction-transformation-loading-explained/
1 note
¡
View note
Text
Overcoming Challenges in Data Integration: Insights from Consulting ExpertsÂ
Data integration for enterprises can take longer due to technological, financial, and time constraints. As a result, modifying data strategies to mitigate risks like incompatibility between many tools or budget overruns is crucial. Companies must also prepare for new compliance requirements to ensure ethical data operations. This post will explore such challenges in data integration while listing valuable insights from consulting experts in this domain.Â
What is Data Integration?Â
Data integration merges data from disparate origins and presents it to maximize comprehension, consolidation, and summarization effectiveness. Integrated data views rely on data ingestion, preparation, and advanced insight extraction. It also streamlines theâŻdata operations servicesâŻacross regulatory report creation, helpdesks, and 360-degree client life cycle management.Â
All data integration strategies involve the extract, transform, and load (ETL) pipelines regardless of business units or target industries. At the same time, the scope of planning and quality assurance in each process varies due to domain-specific data classification factors.Â
For instance, the accounting departments must handle extensive numerical data while interpreting legal and organizational requirements for transparency. On the other hand, production engineering and design professionals will use visualizations to improve goods or service packages. Accordingly, accountants will use unique tools distinct from engineersâ software.Â
Later, the leaders might want a comprehensive overview of the synergy between these departments. Therefore, they must determine efficient data integration strategies. The data will move between several programs, carrying forward many updates throughout a projectâs progression based on those roadmaps.Â
Overcoming the Challenges in Data Integration Using Insights from Consulting ExpertsÂ
1| Data Quality HurdlesÂ
Linking, consolidating, and updating data from several sources will exponentially increase the quality-related threats. For instance, consider multimedia assets from social networks or unreliable news outlets. They can help your secondary market research and social listening initiatives. However, you want to verify the authenticity of gathered intelligence to avoid inaccurate data ingestion.Â
Evaluating relevance, freshness, and consistency is essential to data quality assurance from creation to archival. So, corporations have started leveragingâŻdata lifecycle managementâŻto boost dataset integrity, helping make integration less of a hassle.Â
Insights:Â
Most consulting experts suggest developing ecosystems that check and recheck quality metrics at each stage of a data integration lifecycle. Moreover, they recommend maintaining periodic data backups with robust version control mechanisms. Doing so will help quality preservation efforts if errors arise after a feature update or a malicious third party is likely to break the system using malware.Â
2| Networking and Computing Infrastructure ProblemsÂ
Legacy hardware and software often introduce bottlenecks, hurting data integrationâs efficiency. Modern integration strategies demand more capable IT infrastructure due to the breakthroughs like theâŻinternetâŻofâŻthingsâŻ(IoT), 5G networks, big data, and large language models. If a company fails to procure the necessary resources, it must postpone data integration.Â
Technologies integral to capturing, storing, checking, sorting, transferring, and encrypting data imply significant electricity consumption. Besides, a stable networking environment with adequate governance implementations enables secure data transactions. The underlying computing infrastructure is not immune to physical damage or downtime risks due to maintenance mishaps.Â
What Consulting Experts Say:Â
Enterprises must invest in reliable, scalable, and efficient hardware-software infrastructure. This will benefit them by providing a stable working environment and allowing employees to witness productivity improvements. Upgrading IT systems will also enhance cybersecurity, lowering the risk of zero-day vulnerabilities.Â
3| Data Availability DelaysÂ
Governments, global firms, educational institutions, hospitals, and import-export organizations have a vast network of regional offices. These offices must also interact with suppliers, contractors, and customers. Due to the scale of stakeholder engagement, reports concerning office-level performance and inventory might arrive late.Â
Underproductive employees, tech troubleshooting, slow internet connectivity, and a poor data compression ratio will make data sourcing, updating, and analyzing inefficient. As a result, a data integration officer must address time-consuming activities through strategic resource allocation. If left unaddressed, delays in data delivery will adversely affect conflict resolution and customer service.Â
Expert Insights:Â
Train your employees to maximize their potential and reduce data acquisition, categorization, and transformation delays. Additionally, you will want to embrace automation through artificial intelligence (AI) applications. Find methods to increase the data compression ratio and accelerate encryption-decryption processing cycles. These measures will help accomplish near-real-time data integration objectives.Â
4| Vendor Lock-insÂ
A vendor lock-in results from inconvenience and restrictions when a client wants to switch to another service provider or toolkit. Although data integration platforms claim they celebrate the ease of migrating databases with competitors, they might covertly create vendor lock-ins.Â
For instance, some data sourcing and sorting ecosystems might limit the supported formats for bulk export commands. Others will use misleading methods to design the graphical user interface (GUI) of account deletion and data export features. They involve too many alerts or generate corrupt export files.Â
Practical Insights:Â
Combining multiple proprietary and open-source software tools offers the best cost optimization opportunities. When you select a data vendor, audit the tools the willing data integration providers use to deliver their assistance. Do they use a completely proprietary system based on an unknown file format unsupported by other platforms?Â
Finally, you must check all the data import, export, and bulk transfer options in vendorsâ documentation. After you check a data firmâs current client base, track its online ratings and scan for red flags indicating potential vendor lock-ins.Â
5| Data-Related Ethical and Legal LiabilitiesÂ
Confidentiality of investor communication and stakeholdersâ privacy rights are two components of legal risk exposure due to enterprise data integration. Additionally, brands must interpret industry guidelines and regional directives for regulatory disclosures.Â
They must comply with laws concerning personally identifiable information (PII) about employees and customers. Otherwise, they will attract policymakersâ ire, and customers will lose faith in brands that do not comply with the laws of their countries.Â
Insights:Â
Consulting experts recommend collaborating with regional legal teams and global governance compliance specialists. After all, mitigating legal risks can help increase business resilience.Â
Improved compliance ratings have also benefited several brands wanting to be attractive to impact investors. Meanwhile, customers demanding ethical data operations at business establishments love supporting brands with an exceptional governance culture.Â
ConclusionÂ
Most brands need specialists' help to develop consolidated data views during reporting because they have flawed data integration strategies. So, they require trustworthy insights from reputed consulting experts with a proven track record of overcoming challenges in data integration. The selected data partners must excel at ETL implementation, governance compliance, and data quality management (DQM).Â
The corporate world champions data-centric business development. Understandably, the need for scalable data integration reflects the increased stakeholder awareness regarding the importance of connecting disparate data sources. With transparent, fast, and accurate data, organizations will enhance their competitive edge amid this intense digital transformation race.Â
3 notes
¡
View notes
Text

Preparation of F-passivated ZnO for quantum dot photovoltaics
For photovoltaic power generation, pn junction is the core unit. The electric field in the junction can separate and transport the electron and the hole to negative and positive electrodes, respectively. Once the pn junction is connected with a load and exposed to a light ray, it can convert photon power into electrical power and deliver this power to the load. This photovoltaic application has long been used as the power supply for satellites and space vehicles, and also as the power supply for renewable green energy. As the star materials, Si, GaAs, and perovskite have been widely applied for solar power harvesting. However, the absorption cutoff wavelength of these materials is below 1,100 nm, which limits their photovoltaic applications in infrared photon power. Hence, it is necessary to explore new materials for photovoltaics. PbSe colloidal quantum dots (CQDs) are promising candidates for photovoltaics because its photoactive range can cover the whole solar spectrum. Thanks to the rapid advances in metal halide ligands and solution phase ligand exchange processes, the efficiency of PbSe CQD solar cells approaches to 11.6%. In view of these developments, further improvement of device performance can focus on the optimization of the electron transport layer (ETL) and the hole transport layer (HTL).
Read more.
3 notes
¡
View notes
Text
Explore Airbyte, the open-source data integration platform tailored for fintech firms. Streamline your integration process with its extensive connector library, real-time capabilities, user-friendly interface, and vibrant community support. Elevate your data workflows effortlessly with Airbyte.
Know more at: https://bit.ly/3w8qHf1
#Airbyte#fintech#Technology#Data transformation#data Integration#data extraction#ETL#ELT#Data loading#Cloud data#Data management#Clound Data Management#Trending
0 notes
Text
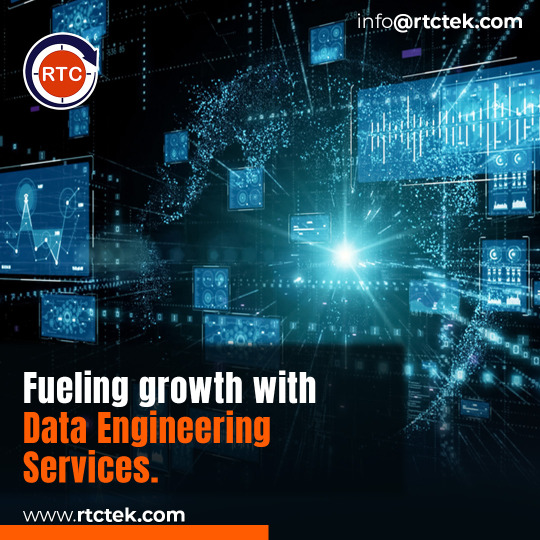
Empower your business's expansion by utilizing our Data Engineering Services. We specialize in optimizing and managing your data, ensuring it's readily available for informed decision-making, ultimately fueling your company's growth and success. Learn more about our services at Data Engineering Services - Round The Clock Technologies (rtctek.com).
#dataengineering#bigdata#datapipeline#dataintegration#etl (extract#transform#load)#dataprocessing#dataarchitecture#datawarehousing#dataops#dataquality#dataanalytics#datascience#datamanagement#datainfrastructure#database#datatechnology#streamingdata
1 note
¡
View note