#automl tools
Explore tagged Tumblr posts
Text
Understanding AutoML: An Overview | USAII®
AutoML has helped the development and deployment of machine learning models easier, and effortless. Learn its advantages, uses, popular AutoML tools, challenges, and more.
Read more: https://shorturl.at/CpscF
Automated machine learning, AutoML systems, AutoML models, AutoML platforms, automated AutoML, AutoML Tools, AI professionals, AI Certification Programs, Machine Learning Certifications. ML tools

0 notes
Text
Unlock the potential of your business with NextBrain's No-code AutoML. Our intuitive automl tools put the power of machine learning in your hands, allowing you to make data-driven decisions and drive growth.
0 notes
Text
Evolusi Framework AI: Alat Terbaru untuk Pengembangan Model AI di 2025
Kecerdasan buatan (AI) telah menjadi salah satu bidang yang paling berkembang pesat dalam beberapa tahun terakhir. Pada tahun 2025, teknologi AI diperkirakan akan semakin maju, terutama dengan adanya berbagai alat dan framework baru yang memungkinkan pengembang untuk menciptakan model AI yang lebih canggih dan efisien. Framework AI adalah sekumpulan pustaka perangkat lunak dan alat yang digunakan…
#AI applications#AI automation#AI development tools#AI ethics#AI for business#AI framework#AI in 2025#AI in edge devices#AI technology trends#AI transparency#AutoML#deep learning#edge computing#future of AI#machine learning#machine learning automation#model optimization#PyTorch#quantum computing#TensorFlow
0 notes
Text
0 notes
Text
🔓 Unlocking Google AI Review ✅ Your Gateway to Advanced Artificial Intelligence Tools! 🚀🤖🌍
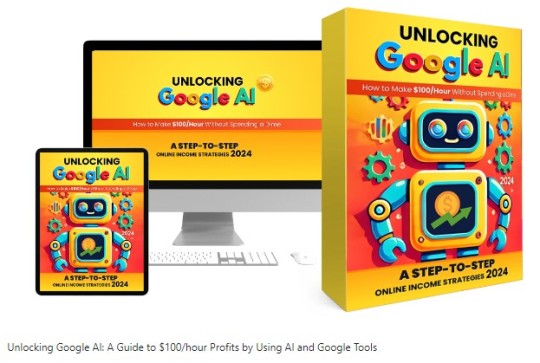
Google AI is one of the most accessible and powerful sets of AI tools and resources available, providing a wide array of solutions that can benefit developers, businesses, educators, and everyday users. Through platforms like Google Cloud AI, TensorFlow, Vertex AI, and Google’s AI-powered tools for productivity (like Google Workspace), Google makes it easier for everyone to leverage the latest advancements in artificial intelligence. Here’s how to unlock and make the most out of Google AI’s capabilities!
👉 Click Here for Get Instant Access Unlocking Google AI 🖰 >>
🔍 Overview:
Unlocking Google AI is a guide or platform aimed at helping users maximize the potential of Google’s AI tools and services. This package offers insights, tips, and strategies to integrate Google AI technology into various applications, from business to personal productivity. Whether you’re looking to automate tasks, improve data analysis, or create intelligent solutions, Unlocking Google AI provides you with the foundational knowledge and practical skills to take full advantage of Google’s cutting-edge AI tools. 📊✨
👉 Click Here for Get Instant Access Unlocking Google AI 🖰 >>
🚀 Key Features
Detailed Walkthrough of Google AI Tools: Learn how to use Google’s AI-driven applications, including Google Cloud AI, Google Machine Learning, TensorFlow, and natural language processing tools. This comprehensive guide makes advanced AI technology accessible for both beginners and seasoned users. 💼📘
Practical AI Integration Tips: Unlocking Google AI provides step-by-step instructions for incorporating AI into everyday tasks. You’ll gain insights into automating processes, optimizing workflows, and improving decision-making through AI-driven data analytics. 🧠🔄
Real-World Applications: Learn how to implement AI in a variety of fields, from customer service and marketing to data science and project management. This feature is ideal for professionals and business owners looking to see tangible benefits from AI integration. 📈🌍
Beginner-Friendly and Advanced Content: Whether you’re new to AI or looking to expand your technical skills, Unlocking Google AI offers a range of content levels, ensuring a comfortable learning pace while also offering deep dives for advanced users. 🚀💻
Tips for Ethical and Responsible AI Use: This guide covers the ethical considerations around AI, helping users understand how to use Google AI responsibly. Perfect for anyone aiming to leverage AI with a focus on fairness, transparency, and ethical responsibility. 🌱🤝
🔧 Why Use Unlocking Google AI?
This guide is valuable for professionals, entrepreneurs, students, and tech enthusiasts who want to leverage Google’s AI capabilities to streamline workflows, gain insights, and remain competitive in an AI-driven world. By making complex AI tools approachable, it empowers users to unlock Google’s AI potential without requiring an extensive technical background. 📅💼
👉 Click Here for Get Instant Access Unlocking Google AI 🖰 >>
🛠️ Core Google AI Tools and Platforms:
Google Cloud AI ☁️
TensorFlow 🧠
Vertex AI 🔧
Google Workspace AI Tools 📊✍️
Google AI Experiments 🎨
✅ Benefits of Unlocking Google AI:
Scalability: Google AI tools are designed to scale, making them suitable for projects of any size.
User-Friendly: With platforms like Vertex AI and AutoML, users can create and deploy machine learning models without needing extensive ML expertise.
Extensive Documentation & Resources: Google provides tutorials, case studies, and community support, making it easier to get started and grow.
Cost-Efficient: Many tools offer free or cost-effective options, especially for smaller projects and developers in their early stages.
👉 Click Here for Get Instant Access Unlocking Google AI 🖰 >>
🚀 How to Get Started with Google AI:
Create a Google Cloud Account: Start by signing up for Google Cloud, which offers a free trial with credits that you can use to explore tools like Vertex AI and other Cloud AI services.
Explore TensorFlow Resources: TensorFlow provides comprehensive documentation, tutorials, and community resources, making it an excellent entry point for hands-on AI learning.
Try Google AI Experiments: Visit Google AI Experiments to get a feel for AI capabilities through fun and interactive projects that require no prior experience.
Leverage AI in Google Workspace: If you’re using Google Workspace, activate AI features like Smart Compose in Gmail or Explore in Google Sheets to see how they can boost productivity.
Experiment with Vertex AI AutoML: Use Vertex AI’s AutoML feature to start building models without in-depth coding knowledge, perfect for small businesses or non-technical users.
🔥 Final Thoughts:
Unlocking Google AI can be transformative, providing tools that make complex AI more approachable and scalable for various users. Whether you're a developer, entrepreneur, or simply someone curious about AI, Google AI’s suite of tools makes it easy to harness advanced artificial intelligence to enhance projects, streamline workflows, and innovate with data.
👉 Click Here for Get Instant Access Unlocking Google AI 🖰 >>
#GoogleAI#MachineLearning#AIforEveryone#Innovation#DigitalTransformation#marketing#affiliatemarketing#onlinemarketing#review#reviews#software#preview#make money as an affiliate#make money tips#digitalmarketing#unlocking google ai review#unlocking google ai review & bonus#unlocking google ai course review#unlocking google ai demo#unlocking google ai bonus#unlocking google ai bonuses#unlocking google ai course demo#unlocking google ai course preview#unlocking google ai course scam#unlocking google ai training course#unlocking google ai scam#unlocking google ai#unlocking google ai preview#google#unlocking google ai review plan
2 notes
·
View notes
Text
Future of data science?
Exciting developments await the future of data science as it evolves. Data scientists can expect to see greater integration of artificial intelligence (AI) and machine learning, facilitated by automation and AutoML, which will make data analysis more accessible. Proficiency in big data and real-time analytics will be crucial as the volume and complexity of data continue to expand. Additionally, ethical considerations such as privacy protection, bias detection, and responsible AI implementation will remain important focal points. Furthermore, data science will involve increased interdisciplinary collaboration that bridges technical expertise with domain knowledge and ethics.
Explainable AI will be necessary for the development of trust and compliance, while edge and IoT analytics will cater for the increased demand of IoT devices. In this way, data visualisation and storytelling skills will still be vital. Data scientists will need to adapt as organisations shift to hybrid and multi-cloud environments. This field will require continuous learning for success. The next quantum leap for data science with quantum computing. Customization and personalization will also define data science; this will deliver specific solutions for particular business needs. In the data-driven era, it’s the data scientists that will excel.
If you are considering preparing for this promising future, Edure provides a wide variety of comprehensive data science courses designed for students of all skill levels. Edure has a comprehensive curriculum that includes introductory courses in basic data science concepts, tools and techniques for beginners and advanced programs targeted for experienced professionals. These courses will give you the knowledge and the skills that will enable you to succeed in the data-driven world of tomorrow. Therefore, whether a beginner or an expert desiring to remain up to date in data science, Edure courses are valuable for launching into or deepening one’s involvement in this promising domain.
For more information contact as
Edure | Learn to Earn
Aristo Junction, Thampanoor,
Thiruvananthapuram, Kerala , 695001
+91 9746211123 +91 9746711123
2 notes
·
View notes
Text
Introduction to AI Platforms
AI Platforms are powerful tools that allow businesses to automate complex tasks, provide real-time insights, and improve customer experiences. With their ability to process massive amounts of data, AI platforms can help organizations make more informed decisions, enhance productivity, and reduce costs.
These platforms incorporate advanced algorithms such as machine learning, natural language processing (NLP), and computer vision to analyze data through neural networks and predictive models. They offer a broad range of capabilities such as chatbots, image recognition, sentiment analysis, and recommendation engines.
Choosing the right AI platform is imperative for businesses that wish to stay ahead of the competition. Each platform has its strengths and weaknesses which must be assessed when deciding on a vendor. Moreover, an AI platform’s ability to integrate with existing systems is critical in effectively streamlining operations.
The history of AI platforms dates back to the 1950s, with the development of early artificial intelligence research. However, over time these technologies have evolved considerably – thanks to advancements in computing power and big data analytics. While still in their infancy stages just a few years ago – today’s AI platforms have matured into complex and feature-rich solutions designed specifically for business use cases.
Ready to have your mind blown and your workload lightened? Check out the best AI platforms for businesses and say goodbye to manual tasks:
Popular Commercial AI Platforms
To explore popular the top AI platforms and make informed decisions, you need to know the benefits each platform offers. With IBM Watson, Google Cloud AI Platform, Microsoft Azure AI Platform, and Amazon SageMaker in focus, this section shows the unique advantages each platform provides for various industries and cognitive services.
IBM Watson
The Innovative AI Platform by IBM:
Transform your business with the dynamic cognitive computing technology of IBM Watson. Enhance decision-making, automate operations, and accelerate the growth of your organization with this powerful tool.
Additional unique details about the platform:
IBM Watson’s Artificial intelligence streamlines workflows and personalizes experiences while enhancing predictive capabilities. The open-source ecosystem allows developers and businesses alike to integrate their innovative applications seamlessly.
Suggested implementation strategies:
1) Leverage Watson’s data visualization tools to clearly understand complex data sets and analyze them. 2) Utilize Watson’s Natural Language processing capabilities for sentiment analysis, identifying keywords, or contextual understanding.
By incorporating IBM Watson’s versatile machine learning functions into your operations, you can gain valuable insights into customer behavior patterns, track industry trends, improve decision-making abilities, and eventually boost revenue. Google’s AI platform is so powerful, it knows what you’re searching for before you do.
Google Cloud AI Platform
The AI platform provided by Google Cloud is an exceptional tool for businesses that major in delivering machine learning services. It provides a broad array of functionalities tailored to meet the diverse demands of clients all over the world.
The following table summarizes the features and capabilities offered by the Google Cloud AI Platform:FeaturesCapabilitiesData Management & Pre-processing
– Large-scale data processing
– Data Integration and Analysis tools
– Deep Learning Frameworks
– Data versioning tools
Model Training
– Scalable training
– AutoML tools
– Advanced tuning configurations
– Distributed Training on CPU/GPU/TPU
Prediction
– High-performance responses within seconds
– Accurate predictions resulting from models trained using large-scale datasets.
Monitoring
– Real-time model supervision and adjustment
– Comprehensive monitoring, management, and optimization of models across various stages including deployment.
One unique aspect of the Google Cloud AI platform is its prominent role in enabling any developer, regardless of their prior experience with machine learning, to build sophisticated models. This ease of use accelerates experimentation and fosters innovation.
Finally, it is worth noting that according to a study conducted by International Business Machines Corporation (IBM), brands that adopted AI for customer support purposes experienced 40% cost savings while improving customer satisfaction rates by 90%.
Continue Reading
2 notes
·
View notes
Text
Microsoft Azure Fundamentals AI-900 (Part 5)
Microsoft Azure AI Fundamentals: Explore visual studio tools for machine learning
What is machine learning? A technique that uses math and statistics to create models that predict unknown values
Types of Machine learning
Regression - predict a continuous value, like a price, a sales total, a measure, etc
Classification - determine a class label.
Clustering - determine labels by grouping similar information into label groups
x = features
y = label
Azure Machine Learning Studio
You can use the workspace to develop solutions with the Azure ML service on the web portal or with developer tools
Web portal for ML solutions in Sure
Capabilities for preparing data, training models, publishing and monitoring a service.
First step assign a workspace to a studio.
Compute targets are cloud-based resources which can run model training and data exploration processes
Compute Instances - Development workstations that data scientists can use to work with data and models
Compute Clusters - Scalable clusters of VMs for on demand processing of experiment code
Inference Clusters - Deployment targets for predictive services that use your trained models
Attached Compute - Links to existing Azure compute resources like VMs or Azure data brick clusters
What is Azure Automated Machine Learning
Jobs have multiple settings
Provide information needed to specify your training scripts, compute target and Azure ML environment and run a training job
Understand the AutoML Process
ML model must be trained with existing data
Data scientists spend lots of time pre-processing and selecting data
This is time consuming and often makes inefficient use of expensive compute hardware
In Azure ML data for model training and other operations are encapsulated in a data set.
You create your own dataset.
Classification (predicting categories or classes)
Regression (predicting numeric values)
Time series forecasting (predicting numeric values at a future point in time)
After part of the data is used to train a model, then the rest of the data is used to iteratively test or cross validate the model
The metric is calculated by comparing the actual known label or value with the predicted one
Difference between the actual known and predicted is known as residuals; they indicate amount of error in the model.
Root Mean Squared Error (RMSE) is a performance metric. The smaller the value, the more accurate the model’s prediction is
Normalized root mean squared error (NRMSE) standardizes the metric to be used between models which have different scales.
Shows the frequency of residual value ranges.
Residuals represents variance between predicted and true values that can’t be explained by the model, errors
Most frequently occurring residual values (errors) should be clustered around zero.
You want small errors with fewer errors at the extreme ends of the sale
Should show a diagonal trend where the predicted value correlates closely with the true value
Dotted line shows a perfect model’s performance
The closer to the line of your model’s average predicted value to the dotted, the better.
Services can be deployed as an Azure Container Instance (ACI) or to a Azure Kubernetes Service (AKS) cluster
For production AKS is recommended.
Identify regression machine learning scenarios
Regression is a form of ML
Understands the relationships between variables to predict a desired outcome
Predicts a numeric label or outcome base on variables (features)
Regression is an example of supervised ML
What is Azure Machine Learning designer
Allow you to organize, manage, and reuse complex ML workflows across projects and users
Pipelines start with the dataset you want to use to train the model
Each time you run a pipelines, the context(history) is stored as a pipeline job
Encapsulates one step in a machine learning pipeline.
Like a function in programming
In a pipeline project, you access data assets and components from the Asset Library tab
You can create data assets on the data tab from local files, web files, open at a sets, and a datastore
Data assets appear in the Asset Library
Azure ML job executes a task against a specified compute target.
Jobs allow systematic tracking of your ML experiments and workflows.
Understand steps for regression
To train a regression model, your data set needs to include historic features and known label values.
Use the designer’s Score Model component to generate the predicted class label value
Connect all the components that will run in the experiment
Average difference between predicted and true values
It is based on the same unit as the label
The lower the value is the better the model is predicting
The square root of the mean squared difference between predicted and true values
Metric based on the same unit as the label.
A larger difference indicates greater variance in the individual label errors
Relative metric between 0 and 1 on the square based on the square of the differences between predicted and true values
Closer to 0 means the better the model is performing.
Since the value is relative, it can compare different models with different label units
Relative metric between 0 and 1 on the square based on the absolute of the differences between predicted and true values
Closer to 0 means the better the model is performing.
Can be used to compare models where the labels are in different units
Also known as R-squared
Summarizes how much variance exists between predicted and true values
Closer to 1 means the model is performing better
Remove training components form your data and replace it with a web service inputs and outputs to handle the web requests
It does the same data transformations as the first pipeline for new data
It then uses trained model to infer/predict label values based on the features.
Create a classification model with Azure ML designer
Classification is a form of ML used to predict which category an item belongs to
Like regression this is a supervised ML technique.
Understand steps for classification
True Positive - Model predicts the label and the label is correct
False Positive - Model predicts wrong label and the data has the label
False Negative - Model predicts the wrong label, and the data does have the label
True Negative - Model predicts the label correctly and the data has the label
For multi-class classification, same approach is used. A model with 3 possible results would have a 3x3 matrix.
Diagonal lien of cells were the predicted and actual labels match
Number of cases classified as positive that are actually positive
True positives divided by (true positives + false positives)
Fraction of positive cases correctly identified
Number of true positives divided by (true positives + false negatives)
Overall metric that essentially combines precision and recall
Classification models predict probability for each possible class
For binary classification models, the probability is between 0 and 1
Setting the threshold can define when a value is interpreted as 0 or 1. If its set to 0.5 then 0.5-1.0 is 1 and 0.0-0.4 is 0
Recall also known as True Positive Rate
Has a corresponding False Positive Rate
Plotting these two metrics on a graph for all values between 0 and 1 provides information.
Receiver Operating Characteristic (ROC) is the curve.
In a perfect model, this curve would be high to the top left
Area under the curve (AUC).
Remove training components form your data and replace it with a web service inputs and outputs to handle the web requests
It does the same data transformations as the first pipeline for new data
It then uses trained model to infer/predict label values based on the features.
Create a Clustering model with Azure ML designer
Clustering is used to group similar objects together based on features.
Clustering is an example of unsupervised learning, you train a model to just separate items based on their features.
Understanding steps for clustering
Prebuilt components exist that allow you to clean the data, normalize it, join tables and more
Requires a dataset that includes multiple observations of the items you want to cluster
Requires numeric features that can be used to determine similarities between individual cases
Initializing K coordinates as randomly selected points called centroids in an n-dimensional space (n is the number of dimensions in the feature vectors)
Plotting feature vectors as points in the same space and assigns a value how close they are to the closes centroid
Moving the centroids to the middle points allocated to it (mean distance)
Reassigning to the closes centroids after the move
Repeating the last two steps until tone.
Maximum distances between each point and the centroid of that point’s cluster.
If the value is high it can mean that cluster is widely dispersed.
With the Average Distance to Closer Center, we can determine how spread out the cluster is
Remove training components form your data and replace it with a web service inputs and outputs to handle the web requests
It does the same data transformations as the first pipeline for new data
It then uses trained model to infer/predict label values based on the features.
2 notes
·
View notes
Text
The Future of Data Visualization: Power BI and AI Integration
In today’s digital world, data is not just a resource—it’s the foundation for innovation and smarter decision-making. With businesses generating more data than ever before, the demand for powerful visualization tools is skyrocketing. Microsoft’s Power BI is leading the way by integrating Artificial Intelligence (AI) to make data visualization smarter, faster, and more impactful.
If you're looking to future-proof your career, mastering Power BI with AI capabilities is essential—and there's no better place to start than with expert power bi training in kphb.

How Power BI and AI are Transforming Data Visualization
Power BI is no longer just a tool for building simple charts and dashboards. Thanks to AI integration, it can now:
Predict trends and future outcomes using machine learning models
Automate insights with features like Quick Insights and Smart Narratives
Understand natural language queries through Q&A visualizations
Detect anomalies in your data automatically
Make recommendations for better decision-making
This evolution means that even non-technical users can uncover hidden patterns in their data and make data-driven decisions with ease.
Key AI-Powered Features in Power BI
Here are some of the top AI features that make Power BI stand out:
Cognitive Services Integration: Embed language detection, sentiment analysis, and image recognition directly into your reports.
AutoML (Automated Machine Learning): Build and deploy machine learning models directly from Power BI without writing a single line of code.
Smart Narrative: Automatically generate easy-to-read summaries of your data insights.
Q&A Visuals: Simply type a question in plain English and get visual answers instantly.
Anomaly Detection: Easily identify unusual spikes or drops in your data.
With these features, Power BI is turning complex data science into an accessible tool for business users and analysts alike.
Why You Should Learn Power BI with AI Skills
In 2025 and beyond, companies will expect their data analysts and business intelligence professionals to do more than just report data—they’ll want predictive insights, automation, and smarter visualizations.
Learning Power BI along with its AI-powered capabilities gives you a competitive edge in roles like:
Data Analyst
Business Intelligence Developer
Data Scientist
Business Analyst
Reporting Specialist
If you want to stay ahead in the analytics field, now is the perfect time to master Power BI.
Start Your Journey with Power BI Training in KPHB
Ready to become an expert in Power BI and AI integration? Enroll in the top-rated Power BI Training in KPHB at NareshIT and learn from industry experts. Our hands-on training covers everything from the basics to advanced AI-powered analytics, helping you build real-world skills that employers value.
👉 Visit NareshIT – Power BI Training in KPHB and kickstart your career in data analytics today!
FAQs – Power BI Training in KPHB
Q1: Does the Power BI training include AI and machine learning concepts? A: Yes! Our Power BI course includes training on AI features like AutoML, Smart Narratives, and Anomaly Detection to give you a complete skill set.
Q2: Who can join the Power BI training at NareshIT? A: Anyone interested in data analytics, from freshers to working professionals, can join. No prior coding experience is required.
Q3: Is the training hands-on with real projects? A: Absolutely. Our course is project-based, ensuring you gain practical experience in building dashboards and applying AI features.
Q4: Will I receive certification after completing the course? A: Yes, you’ll receive a certification from NareshIT, helping you validate your skills and boost your resume.
Q5: How can I enroll for the Power BI training? A: Simply click here to enroll or get in touch with our team for more details.
Conclusion: The future of data visualization is here, and it's powered by AI. With Power BI’s evolving capabilities, those who invest in learning these new skills will lead the next generation of data-driven innovation. Don’t wait—start your journey today with Power BI training in KPHB and become future-ready!
0 notes
Text
The Role of AI in Data Science: How AI is Changing the Game in 2025
In 2025, AI isn’t just a buzzword; it’s a game-changer for data science. From automating repetitive tasks to enhancing predictive analytics, AI is transforming how data scientists do their job. Whether you're just starting or looking to upskill, understanding AI's role in data science is essential for staying ahead of the curve.
So, how exactly is AI reshaping data science, and why should you care?
🤖 AI is Automating the Boring Stuff
Let’s face it, data cleaning and feature engineering are not the most exciting parts of a data scientist’s job. But with AI tools, automating these tasks is becoming a reality.
AutoML (Automated Machine Learning) allows algorithms to select the best model and tune hyperparameters automatically.
AI tools like DataRobot and Google Cloud AutoML can now help with repetitive tasks, freeing up your time for high-level analysis.
Now, instead of spending hours cleaning data, AI lets you focus on finding insights.
🧠 AI-Driven Predictive Analytics
One of the most powerful ways AI is enhancing data science is through predictive analytics. AI can process massive datasets and identify trends that would be impossible for humans to catch.
For example, AI models are being used in finance to predict market trends and in healthcare to forecast disease outbreaks.
In marketing, AI can predict customer behavior, optimizing campaigns in real-time.
AI isn’t just helping data scientists do their job; it’s supercharging their predictive power.
💡 AI Tools for Data Scientists
In 2025, a data scientist without AI tools is like a chef without a knife. Here are some of the key tools reshaping the industry:
TensorFlow: One of the most popular open-source AI frameworks, TensorFlow makes it easy to build and train machine learning models.
PyTorch: Widely used in deep learning, PyTorch allows data scientists to work faster and more efficiently.
IBM Watson: Provides AI-powered analytics tools that help businesses with advanced data processing and decision-making.
Integrating these tools into your workflow can take your data science career to new heights.
🌐 AI in Data Science for Real-World Solutions
It’s not just theory; AI is already being used in real-world data science projects.
In e-commerce, AI analyzes customer purchase patterns to optimize product recommendations and improve sales.
In healthcare, AI helps doctors predict patient outcomes, making treatments more personalized and effective.
In transportation, AI optimizes routes for delivery services and enhances vehicle safety with real-time data processing.
AI in data science is everywhere, and it’s just getting started.
🚀 Is AI the Future of Data Science?
Absolutely. AI isn’t just a part of the data science landscape; it’s becoming integrated into every aspect of it. In 2025, the future of data science will likely be defined by how effectively we can leverage AI to solve complex problems.
If you’re just starting in data science, learning how to work with AI should be at the top of your list. It’s the skill that will set you apart.
🎓 How to Get Started with AI in Data Science
If you’re ready to dive into AI and data science, start with a comprehensive course like the Intellipaat Data Science course. This course covers:
✅ AI and machine learning fundamentals ✅ Hands-on experience with real-world projects ✅ How to apply AI to data analysis and predictive modeling
📺 Get started with the Intellipaat Data Science course
🔥 Final Thought:
AI is no longer a futuristic concept; it’s here, and it’s making data science smarter, faster, and more impactful than ever. By learning how to integrate AI into your workflow, you’ll not only keep up with trends in 2025 but also lead them.
1 note
·
View note
Text
Model accuracy is a key metric in machine learning that measures how well a model predicts outcomes. Learn more about model accuracy and its importance in this comprehensive guide!
#no code ml#no code machine learning#no code machine learning tools#machine learning no code#no-code machine learning open-source#no code ml platforms#no-code automl#no code ai
0 notes
Text
Top Artificial Intelligence Companies Leading Innovation in 2025

Artificial Intelligence is at the heart of today’s digital transformation. From self-driving technology to personalized marketing and advanced data analytics, AI is changing how we live and work. For businesses aiming to stay competitive, the need to hire AI developers who understand the latest advancements is more urgent than ever. And behind these innovations are some powerful AI companies pushing the boundaries of what's possible.
Below are some of the top artificial intelligence companies to watch in 2025 — each playing a unique role in shaping the future of technology.
OpenAI
OpenAI remains a global leader in artificial intelligence, known for its groundbreaking language models like GPT-4 and GPT-5. Their innovations have sparked massive shifts in how businesses interact with customers, automate workflows, and extract insights from data. OpenAI's tools are used by companies of all sizes, making it a cornerstone of modern AI development.
Google DeepMind
A subsidiary of Alphabet Inc., DeepMind is widely regarded for its contributions to deep learning and reinforcement learning. Projects like AlphaGo and AlphaFold have not only amazed the world but also demonstrated the real-world impact of AI in healthcare, biology, and problem-solving.
IBM Watson
IBM’s Watson was one of the earliest AI platforms to go mainstream. Known for its cognitive computing capabilities, Watson helps enterprises in finance, healthcare, and logistics make smarter decisions. IBM continues to evolve its AI services with a focus on ethical AI and business-scale solutions.
NVIDIA
While NVIDIA is known for its powerful GPUs, it’s also a key player in AI research and development. Their AI platforms enable developers to build, train, and deploy models faster than ever. From autonomous vehicles to medical imaging, NVIDIA’s technology is foundational to AI applications across industries.
Anthropic
Anthropic, a newer but fast-growing AI company, is focused on creating more steerable and safe AI systems. Their large language models — such as Claude — are built with an emphasis on alignment, safety, and interpretability, making them ideal for enterprise use.
Hugging Face
Hugging Face is the open-source darling of the AI world. With its Transformer library and model hub, it has democratized access to state-of-the-art models for NLP, computer vision, and beyond. Developers love the flexibility and community support Hugging Face offers.
C3.ai
Specializing in enterprise AI, C3.ai provides a platform that helps organizations rapidly develop, deploy, and operate large-scale AI applications. They work across multiple sectors, including manufacturing, oil & gas, financial services, and government.
DataRobot
DataRobot focuses on automated machine learning (AutoML), helping companies without in-house AI expertise to create predictive models quickly and effectively. Their platform is especially useful for businesses that want to apply AI but lack deep technical resources.
Why These Companies Matter
These AI companies aren't just creating technology — they’re building the future. Whether you're a startup or an enterprise, partnering with or learning from these leaders can accelerate your growth. And if you're planning to build AI-driven solutions yourself, now is the time to hire AI developers who can bring this level of innovation to your business.
Final Thoughts
AI is evolving faster than ever, and the companies at the forefront are shaping a smarter, more automated world. Keeping up with these leaders—and integrating their tools or talent—can give your business a major edge. Whether you're just starting out or scaling up, surrounding yourself with the right tech and the right people is the key to long-term success.
0 notes
Text
Trends and Innovations in Data Science
In today’s fast-paced tech landscape, the best data science training in Hyderabad is the foundation for a thriving career in analytics and artificial intelligence. As data continues to grow exponentially, the future of data science is being reshaped by innovation, automation, and deeper integration across industries.
Emerging Trends in Data Science
The data science industry is evolving rapidly. One major trend is the growing use of automated machine learning (AutoML), which allows non-experts to build powerful models with minimal coding. Another significant trend is the integration of real-time analytics, enabling businesses to make decisions based on live data. Cloud-based data platforms are also gaining traction, offering scalability and flexibility for data storage and processing.
Innovations Driving the Field
Cutting-edge technologies like generative AI, quantum computing, and edge computing are set to revolutionize data science. Generative AI tools are transforming how models are trained and deployed, while quantum computing holds the potential to solve complex problems beyond the capacity of classical computers.Meanwhile, edge computing reduces latency and increases efficiency by processing data at the source.
Upskilling for the Future
To stay ahead, aspiring data scientists need hands-on training in tools like Python, R, TensorFlow, and Power BI, as well as a solid foundation in statistics and business intelligence. Personalized mentorship and real-time projects are crucial for mastering these skills.
Conclusion
For those seeking a career-ready program aligned with the latest industry trends, SSSIT Computer Education offers expert-led, comprehensive training designed to equip students with in-demand data science skills. Future-proof your career with the best in Hyderabad.
0 notes
Text
In 2025, the most influential data science technologies include generative AI, automated machine learning (AutoML), edge computing, synthetic data generation, and quantum computing. These innovations enhance model accuracy, speed, and scalability, empowering smarter decision-making. Cloud-native tools and AI-optimized hardware further drive efficiency and accessibility in data science workflows.
0 notes
Text
Understanding the Current Landscape of AI Innovation

The year 2025 finds artificial intelligence no longer confined to the realms of science fiction or niche applications. It has firmly established itself as a foundational technology, driving innovation across diverse sectors and reshaping the very fabric of our digital and physical worlds. To truly grasp the current landscape of AI innovation, we need to move beyond the hype and delve into the core trends, the driving forces, and the emerging challenges that define this transformative era.
The Foundation: Continued Advancements in Machine Learning and Deep Learning:
At the heart of the AI revolution lies the ongoing evolution of machine learning (ML) and deep learning (DL). We are witnessing the development of more sophisticated neural network architectures, more efficient training methodologies, and the ability to process increasingly vast and complex datasets. This continuous progress is the bedrock upon which many other AI innovations are built, enabling breakthroughs in areas like computer vision, natural language processing, and predictive analytics. The ability of AI to learn and adapt from data with increasing accuracy and efficiency remains a central pillar of the current innovation landscape.
The Democratization of AI Tools and Platforms:
One of the most significant shifts in recent years has been the increasing accessibility of AI tools and platforms. Cloud providers and specialized AI companies are offering user-friendly interfaces, pre-trained models, and automated machine learning (AutoML) solutions that empower individuals and organizations with varying levels of technical expertise to leverage the power of AI. This democratization is fostering innovation across a wider range of industries and enabling smaller businesses and startups to participate in the AI revolution.
The Proliferation of Specialized AI Applications:
The era of general-purpose AI remains largely in the future. The current landscape is characterized by the proliferation of highly specialized AI applications designed to address specific challenges and opportunities within particular domains. We see AI-powered diagnostic tools in healthcare, fraud detection systems in finance, personalized learning platforms in education, and precision agriculture solutions in farming. This focus on domain-specific AI is driving tangible value and accelerating adoption across industries.
The Growing Emphasis on Edge AI and Federated Learning:
As the volume of data generated at the edge (on devices and sensors) continues to explode, and concerns around data privacy intensify, we are witnessing a significant push towards edge AI and federated learning. Edge AI brings computation and intelligence closer to the data source, enabling real-time processing and reducing reliance on cloud infrastructure. Federated learning allows AI models to be trained on decentralized datasets without centralizing sensitive information, enhancing privacy and security. These trends are crucial for applications in IoT, autonomous vehicles, and healthcare, where latency, reliability, and data privacy are paramount.
The Maturation of Natural Language Processing and Conversational AI:
Natural Language Processing (NLP) has reached a new level of sophistication, leading to conversational AI agents that are increasingly natural, context-aware, and capable of handling complex interactions. We see advanced virtual assistants, highly effective chatbots for customer service, and AI tools for text generation, translation, and sentiment analysis. The ability of AI to understand and generate human language with greater fluency is transforming human-computer interaction and creating new opportunities for communication and automation.
The Critical Focus on Trustworthy and Ethical AI:
As AI systems become more deeply integrated into our lives and decision-making processes, the importance of trust and ethics has come to the forefront. Research and development efforts are heavily invested in creating explainable AI (XAI) that can provide insights into its reasoning, mitigating biases in algorithms, ensuring fairness in AI applications, and establishing ethical guidelines for AI development and deployment. Building trustworthy AI is essential for fostering public confidence and ensuring the responsible and beneficial use of this powerful technology.
The Expanding Role of AI in the Metaverse and Spatial Computing:
The burgeoning metaverse and spatial computing environments are being significantly shaped by AI. Intelligent avatars, AI-driven content generation, and AI-powered navigation and interaction within these virtual worlds are creating richer and more immersive experiences. AI is also being used to personalize virtual environments and facilitate seamless communication and collaboration. The integration of AI is crucial for the evolution and widespread adoption of these new digital frontiers.
The Interplay of AI with Other Emerging Technologies:
AI innovation is not happening in a vacuum. It is increasingly intertwined with other emerging technologies like the Internet of Things (IoT), 5G, and blockchain. The synergy between these technologies is unlocking new possibilities and creating novel applications. For example, the combination of IoT and AI enables intelligent automation in industrial settings, while the integration of AI with blockchain can enhance security and transparency in data management.
The Growing Threat Landscape and the Insidious "Rise of AI Worms":
As our reliance on interconnected AI systems deepens across industries and critical infrastructure, the cybersecurity landscape is undergoing a significant transformation. While AI is being leveraged to enhance threat detection and response, a concerning counter-trend is emerging: the potential rise of AI worms. These are self-replicating, autonomous malicious AI agents that can exploit vulnerabilities in interconnected AI systems, potentially bypassing traditional security measures by leveraging the very intelligence of AI models to navigate and compromise networks. Understanding and mitigating this evolving threat requires a proactive and sophisticated approach to AI-powered cybersecurity, focusing on building resilient and self-defending AI ecosystems.
The Global Race for AI Talent and Innovation:
The transformative potential of AI has ignited a global race for talent, research breakthroughs, and technological dominance. Nations and organizations are investing heavily in AI education, research institutions, and infrastructure to secure a leading position in this critical technological domain. This competition is driving rapid innovation but also necessitates international collaboration and the development of shared standards and ethical guidelines.
Navigating the Dynamic Landscape:
Understanding the current landscape of AI innovation requires recognizing its multifaceted nature. It's a dynamic field characterized by rapid advancements, increasing accessibility, growing specialization, and a heightened awareness of the ethical and security implications. By staying informed about these key trends, businesses, policymakers, and individuals can better navigate the opportunities and challenges presented by this transformative technology and contribute to shaping a future where AI serves humanity in a responsible and beneficial manner.
0 notes