#data annotation services for machine learning
Explore tagged Tumblr posts
Text
Data Annotation Services: Fueling the Future of AI/ML Applications
Explore the pivotal role of data annotation services in advancing AI/ML applications. Uncover how these services drive innovation by enhancing machine learning models with accurately labeled datasets. Dive into the future of Artificial Intelligence and Machine Learning, where precise data annotation serves as the cornerstone for groundbreaking advancements. Read the blog;…
View On WordPress
#Data Annotation Services#Data Annotation Services For Machine Learning#data annotation services.#outsource data annotation services
0 notes
Text
Decoding the Power of Speech: A Deep Dive into Speech Data Annotation
Introduction
In the realm of artificial intelligence (AI) and machine learning (ML), the importance of high-quality labeled data cannot be overstated. Speech data, in particular, plays a pivotal role in advancing various applications such as speech recognition, natural language processing, and virtual assistants. The process of enriching raw audio with annotations, known as speech data annotation, is a critical step in training robust and accurate models. In this in-depth blog, we'll delve into the intricacies of speech data annotation, exploring its significance, methods, challenges, and emerging trends.
The Significance of Speech Data Annotation
1. Training Ground for Speech Recognition: Speech data annotation serves as the foundation for training speech recognition models. Accurate annotations help algorithms understand and transcribe spoken language effectively.
2. Natural Language Processing (NLP) Advancements: Annotated speech data contributes to the development of sophisticated NLP models, enabling machines to comprehend and respond to human language nuances.
3. Virtual Assistants and Voice-Activated Systems: Applications like virtual assistants heavily rely on annotated speech data to provide seamless interactions, and understanding user commands and queries accurately.
Methods of Speech Data Annotation
1. Phonetic Annotation: Phonetic annotation involves marking the phonemes or smallest units of sound in a given language. This method is fundamental for training speech recognition systems.
2. Transcription: Transcription involves converting spoken words into written text. Transcribed data is commonly used for training models in natural language understanding and processing.
3. Emotion and Sentiment Annotation: Beyond words, annotating speech for emotions and sentiments is crucial for applications like sentiment analysis and emotionally aware virtual assistants.
4. Speaker Diarization: Speaker diarization involves labeling different speakers in an audio recording. This is essential for applications where distinguishing between multiple speakers is crucial, such as meeting transcription.
Challenges in Speech Data Annotation
1. Accurate Annotation: Ensuring accuracy in annotations is a major challenge. Human annotators must be well-trained and consistent to avoid introducing errors into the dataset.
2. Diverse Accents and Dialects: Speech data can vary significantly in terms of accents and dialects. Annotating diverse linguistic nuances poses challenges in creating a comprehensive and representative dataset.
3. Subjectivity in Emotion Annotation: Emotion annotation is subjective and can vary between annotators. Developing standardized guidelines and training annotators for emotional context becomes imperative.
Emerging Trends in Speech Data Annotation
1. Transfer Learning for Speech Annotation: Transfer learning techniques are increasingly being applied to speech data annotation, leveraging pre-trained models to improve efficiency and reduce the need for extensive labeled data.
2. Multimodal Annotation: Integrating speech data annotation with other modalities such as video and text is becoming more common, allowing for a richer understanding of context and meaning.
3. Crowdsourcing and Collaborative Annotation Platforms: Crowdsourcing platforms and collaborative annotation tools are gaining popularity, enabling the collective efforts of annotators worldwide to annotate large datasets efficiently.
Wrapping it up!
In conclusion, speech data annotation is a cornerstone in the development of advanced AI and ML models, particularly in the domain of speech recognition and natural language understanding. The ongoing challenges in accuracy, diversity, and subjectivity necessitate continuous research and innovation in annotation methodologies. As technology evolves, so too will the methods and tools used in speech data annotation, paving the way for more accurate, efficient, and context-aware AI applications.
At ProtoTech Solutions, we offer cutting-edge Data Annotation Services, leveraging expertise to annotate diverse datasets for AI/ML training. Their precise annotations enhance model accuracy, enabling businesses to unlock the full potential of machine-learning applications. Trust ProtoTech for meticulous data labeling and accelerated AI innovation.
#speech data annotation#Speech data#artificial intelligence (AI)#machine learning (ML)#speech#Data Annotation Services#labeling services for ml#ai/ml annotation#annotation solution for ml#data annotation machine learning services#data annotation services for ml#data annotation and labeling services#data annotation services for machine learning#ai data labeling solution provider#ai annotation and data labelling services#data labelling#ai data labeling#ai data annotation
0 notes
Text
What is a Data pipeline for Machine Learning?
As machine learning technologies continue to advance, the need for high-quality data has become increasingly important. Data is the lifeblood of computer vision applications, as it provides the foundation for machine learning algorithms to learn and recognize patterns within images or video. Without high-quality data, computer vision models will not be able to effectively identify objects, recognize faces, or accurately track movements.
Machine learning algorithms require large amounts of data to learn and identify patterns, and this is especially true for computer vision, which deals with visual data. By providing annotated data that identifies objects within images and provides context around them, machine learning algorithms can more accurately detect and identify similar objects within new images.
Moreover, data is also essential in validating computer vision models. Once a model has been trained, it is important to test its accuracy and performance on new data. This requires additional labeled data to evaluate the model's performance. Without this validation data, it is impossible to accurately determine the effectiveness of the model.
Data Requirement at multiple ML stage
Data is required at various stages in the development of computer vision systems.
Here are some key stages where data is required:
Training: In the training phase, a large amount of labeled data is required to teach the machine learning algorithm to recognize patterns and make accurate predictions. The labeled data is used to train the algorithm to identify objects, faces, gestures, and other features in images or videos.
Validation: Once the algorithm has been trained, it is essential to validate its performance on a separate set of labeled data. This helps to ensure that the algorithm has learned the appropriate features and can generalize well to new data.
Testing: Testing is typically done on real-world data to assess the performance of the model in the field. This helps to identify any limitations or areas for improvement in the model and the data it was trained on.
Re-training: After testing, the model may need to be re-trained with additional data or re-labeled data to address any issues or limitations discovered in the testing phase.
In addition to these key stages, data is also required for ongoing model maintenance and improvement. As new data becomes available, it can be used to refine and improve the performance of the model over time.
Types of Data used in ML model preparation
The team has to work on various types of data at each stage of model development.
Streamline, structured, and unstructured data are all important when creating computer vision models, as they can each provide valuable insights and information that can be used to train the model.
Streamline data refers to data that is captured in real-time or near real-time from a single source. This can include data from sensors, cameras, or other monitoring devices that capture information about a particular environment or process.
Structured data, on the other hand, refers to data that is organized in a specific format, such as a database or spreadsheet. This type of data can be easier to work with and analyze, as it is already formatted in a way that can be easily understood by the computer.
Unstructured data includes any type of data that is not organized in a specific way, such as text, images, or video. This type of data can be more difficult to work with, but it can also provide valuable insights that may not be captured by structured data alone.
When creating a computer vision model, it is important to consider all three types of data in order to get a complete picture of the environment or process being analyzed. This can involve using a combination of sensors and cameras to capture streamline data, organizing structured data in a database or spreadsheet, and using machine learning algorithms to analyze and make sense of unstructured data such as images or text. By leveraging all three types of data, it is possible to create a more robust and accurate computer vision model.
Data Pipeline for machine learning
The data pipeline for machine learning involves a series of steps, starting from collecting raw data to deploying the final model. Each step is critical in ensuring the model is trained on high-quality data and performs well on new inputs in the real world.
Below is the description of the steps involved in a typical data pipeline for machine learning and computer vision:
Data Collection: The first step is to collect raw data in the form of images or videos. This can be done through various sources such as publicly available datasets, web scraping, or data acquisition from hardware devices.
Data Cleaning: The collected data often contains noise, missing values, or inconsistencies that can negatively affect the performance of the model. Hence, data cleaning is performed to remove any such issues and ensure the data is ready for annotation.
Data Annotation: In this step, experts annotate the images with labels to make it easier for the model to learn from the data. Data annotation can be in the form of bounding boxes, polygons, or pixel-level segmentation masks.
Data Augmentation: To increase the diversity of the data and prevent overfitting, data augmentation techniques are applied to the annotated data. These techniques include random cropping, flipping, rotation, and color jittering.
Data Splitting: The annotated data is split into training, validation, and testing sets. The training set is used to train the model, the validation set is used to tune the hyperparameters and prevent overfitting, and the testing set is used to evaluate the final performance of the model.
Model Training: The next step is to train the computer vision model using the annotated and augmented data. This involves selecting an appropriate architecture, loss function, and optimization algorithm, and tuning the hyperparameters to achieve the best performance.
Model Evaluation: Once the model is trained, it is evaluated on the testing set to measure its performance. Metrics such as accuracy, precision, recall, and score are computed to assess the model's performance.
Model Deployment: The final step is to deploy the model in the production environment, where it can be used to solve real-world computer vision problems. This involves integrating the model into the target system and ensuring it can handle new inputs and operate in real time.
TagX Data as a Service
Data as a service (DaaS) refers to the provision of data by a company to other companies. TagX provides DaaS to AI companies by collecting, preparing, and annotating data that can be used to train and test AI models.
Here’s a more detailed explanation of how TagX provides DaaS to AI companies:
Data Collection: TagX collects a wide range of data from various sources such as public data sets, proprietary data, and third-party providers. This data includes image, video, text, and audio data that can be used to train AI models for various use cases.
Data Preparation: Once the data is collected, TagX prepares the data for use in AI models by cleaning, normalizing, and formatting the data. This ensures that the data is in a format that can be easily used by AI models.
Data Annotation: TagX uses a team of annotators to label and tag the data, identifying specific attributes and features that will be used by the AI models. This includes image annotation, video annotation, text annotation, and audio annotation. This step is crucial for the training of AI models, as the models learn from the labeled data.
Data Governance: TagX ensures that the data is properly managed and governed, including data privacy and security. We follow data governance best practices and regulations to ensure that the data provided is trustworthy and compliant with regulations.
Data Monitoring: TagX continuously monitors the data and updates it as needed to ensure that it is relevant and up-to-date. This helps to ensure that the AI models trained using our data are accurate and reliable.
By providing data as a service, TagX makes it easy for AI companies to access high-quality, relevant data that can be used to train and test AI models. This helps AI companies to improve the speed, quality, and reliability of their models, and reduce the time and cost of developing AI systems. Additionally, by providing data that is properly annotated and managed, the AI models developed can be exp
2 notes
·
View notes
Text

How Video Transcription Services Improve AI Training Through Annotated Datasets
Video transcription services play a crucial role in AI training by converting raw video data into structured, annotated datasets, enhancing the accuracy and performance of machine learning models.
#video transcription services#aitraining#Annotated Datasets#machine learning#ultimate sex machine#Data Collection for AI#AI Data Solutions#Video Data Annotation#Improving AI Accuracy
0 notes
Text
Generative AI | High-Quality Human Expert Labeling | Apex Data Sciences
Apex Data Sciences combines cutting-edge generative AI with RLHF for superior data labeling solutions. Get high-quality labeled data for your AI projects.
#GenerativeAI#AIDataLabeling#HumanExpertLabeling#High-Quality Data Labeling#Apex Data Sciences#Machine Learning Data Annotation#AI Training Data#Data Labeling Services#Expert Data Annotation#Quality AI Data#Generative AI Data Labeling Services#High-Quality Human Expert Data Labeling#Best AI Data Annotation Companies#Reliable Data Labeling for Machine Learning#AI Training Data Labeling Experts#Accurate Data Labeling for AI#Professional Data Annotation Services#Custom Data Labeling Solutions#Data Labeling for AI and ML#Apex Data Sciences Labeling Services
1 note
·
View note
Text
Challenges and Best Practices in Data Annotation
Data annotation is a crucial step in training machine learning models, but it comes with its own set of challenges. Addressing these challenges effectively through best practices can significantly enhance the quality of the resulting AI models.
Challenges in Data Annotation
Consistency and Accuracy: One of the major challenges is ensuring consistency and accuracy in annotations. Different annotators might interpret data differently, leading to inconsistencies. This can degrade the performance of the machine learning model.
Scalability: Annotating large datasets manually is time-consuming and labor-intensive. As datasets grow, maintaining quality while scaling up the annotation process becomes increasingly difficult.
Subjectivity: Certain data, such as sentiment in text or complex object recognition in images, can be highly subjective. Annotators’ personal biases and interpretations can affect the consistency of the annotations.
Domain Expertise: Some datasets require specific domain knowledge for accurate annotation. For instance, medical images need to be annotated by healthcare professionals to ensure correctness.
Bias: Bias in data annotation can stem from the annotators' cultural, demographic, or personal biases. This can result in biased AI models that do not generalize well across different populations.
Best Practices in Data Annotation
Clear Guidelines and Training: Providing annotators with clear, detailed guidelines and comprehensive training is essential. This ensures that all annotators understand the criteria uniformly and reduces inconsistencies.
Quality Control Mechanisms: Implementing quality control mechanisms, such as inter-annotator agreement metrics, regular spot-checks, and using a gold standard dataset, can help maintain high annotation quality. Continuous feedback loops are also critical for improving annotator performance over time.
Leverage Automation: Utilizing automated tools can enhance efficiency. Semi-automated approaches, where AI handles simpler tasks and humans review the results, can significantly speed up the process while maintaining quality.
Utilize Expert Annotators: For specialized datasets, employ domain experts who have the necessary knowledge and experience. This is particularly important for fields like healthcare or legal documentation where accuracy is critical.
Bias Mitigation: To mitigate bias, diversify the pool of annotators and implement bias detection mechanisms. Regular reviews and adjustments based on detected biases are necessary to ensure fair and unbiased data.
Iterative Annotation: Use an iterative process where initial annotations are reviewed and refined. Continuous cycles of annotation and feedback help in achieving more accurate and reliable data.
For organizations seeking professional assistance, companies like Data Annotation Services provide tailored solutions. They employ advanced tools and experienced annotators to ensure precise and reliable data annotation, driving the success of AI projects.
#datasets for machine learning#Data Annotation services#data collection#AI for machine learning#business
0 notes
Text

Hello there,
I'm Md. Maruful Islam is a skilled trainer of data annotators from Bangladesh. I currently view my job at Acme AI, the pioneer in the data annotation sector, as an honour. I've improved my use of many annotation tools throughout my career, including SuperAnnotate, Supervise.ly, Kili, Cvat, Tasuki, FastLabel, and others.
I have written consistently good annotations, earning me credibility as a well-respected professional in the industry. My GDPR, ISO 27001, and ISO 9001 certifications provide even more assurance that data security and privacy laws are followed.
I genuinely hope you will consider my application. I'd like to learn more about this project as a data annotator so that I may make recommendations based on what I know.
I'll do the following to make things easier for you:
Services:
Detecting Objects (Bounding Boxes)
Recognizing Objects with Polygons
Key Points
Image Classification
Segmentation of Semantics and Instances (Masks, Polygons)
Instruments I Utilize:
Super Annotate
LABELBOX
Roboflow
CVAT
Supervised
Kili Platform
V7
Data Types:
Image
Text
Videos
Output Formats:
CSV
COCO
YOLO
JSON
XML
SEGMENTATION MASK
PASCAL VOC
VGGFACE2
#ai data annotator jobs#data annotation#image annotation services#machine learning#ai data annotator#ai image#artificial intelligence#annotation#computer vision#video annotation
0 notes
Text
https://garnet-hawk-wrv5dz.mystrikingly.com/blog/unveiling-the-power-of-precision-a-deep-dive-into-data-annotation-services
In the dynamic landscape of AI, the significance of data annotation services cannot be overstated. Globose Technology Solutions stands at the forefront, pioneering innovation and setting benchmarks in the realm of precision and accuracy. As we continue to unravel the possibilities of AI, our commitment to delivering high-quality annotated data remains unwavering, shaping the future of intelligent technologies. Explore the possibilities with Globose Technology Solutions, where precision meets innovation, and excellence defines our journey in the world of Artificial Intelligence.
#data annotation#data annotation company#data annotation services#data annotation for ml#machine learning#Data Annotation#data collection company#datasets#globose technology solutions#ai#video annotation#technology
0 notes
Text
🛎 Ensure Accuracy in Labeling With AI Data Annotation Services
🚦 The demand for speed in data labeling annotation has reached unprecedented levels. Damco integrates predictive and automated AI data annotation with the expertise of world-class annotators and subject matter specialists to provide the training sets required for rapid production. All annotation services work is turned around rapidly by a highly qualified team of subject matter experts.
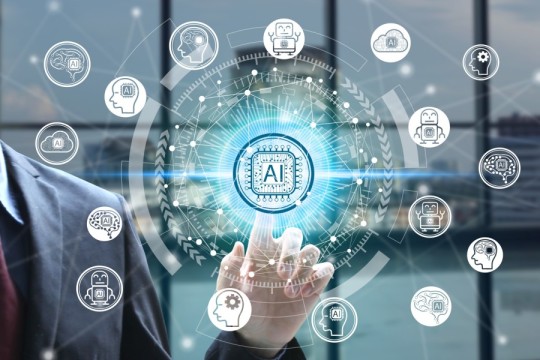
#AI data annotation#data annotation in machine learning#data annotation for ml#data annotation company#data annotation#data annotation services
0 notes
Text
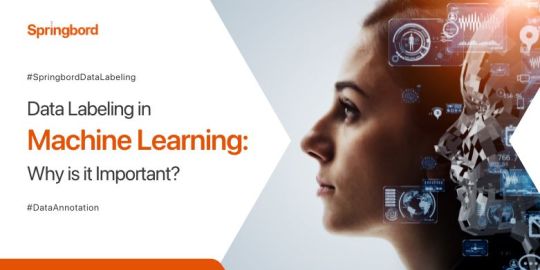
Data labeling in machine learning involves the process of assigning relevant tags or annotations to a dataset, which helps the algorithm to learn and make accurate predictions. Learn more
0 notes
Text
The Rise of Data Annotation Services in Machine Learning Projects
2 notes
·
View notes
Text
How Outsourcing Data Annotation Services Can Supercharge Your AI Model?
Outsourcing data annotation services can significantly enhance your AI model by streamlining the tedious task of labeling large datasets. This boosts efficiency, accelerates model training, and ensures high-quality annotations. Leveraging specialized expertise from external providers enables you to focus on core AI development, yielding more robust and accurate machine learning models. Read the…
View On WordPress
#Data Annotation Services#Data Annotation Services For Machine Learning#data annotation services.#outsource data annotation services#Outsourcing Data Annotation
0 notes
Text
My Experience Working at Appen
Appen
Appen, an internationally acclaimed technology services company, has been at the forefront of providing high-quality training data, annotation, and linguistic services since its inception in 1996. The company has emerged as a significant player in the field of data annotation and machine learning services. From personal experience, Appen offers an excellent platform for individuals seeking to work remotely, providing an array of work-from-home jobs.
Independent Contractor
As an independent contractor, I’ve been involved in numerous projects, ranging from search engine evaluation to micro tasks and voice projects. If you don’t succeed in one project, Appen provides a plethora of options, ensuring that there’s always an opportunity to explore.
The working hours at Appen vary significantly depending on factors like the project you’re working on, your availability, and workload requirements. There might be requirements to work a specific number of hours per week, while at other times, your workload would depend solely on your availability. My projects ranged from 1 to 4 hours, and occasionally, I managed to work up to 8 hours a day when extra work was available. Therefore, the workload can vary extensively depending on the project.
Flexible Work
One of the significant advantages of working with Appen is the flexibility it offers. As a contractor, you have the freedom to set your own schedules and work at your convenience, provided the project requirements and deadlines are met. In my role as a social media evaluator, I had the luxury of starting my work early and finishing by late morning, offering me ample flexibility. However, it’s essential to note that workload and availability requirements can differ based on the project and may change over time.
Appen pays its contractors competitively, with rates varying based on factors like the project, contractor’s location, experience, and skills. According to the company’s website, the hourly rate for some projects ranges from $5 to $30 per hour, while other jobs may pay by task or project.
The company’s remote jobs are an excellent opportunity for students, stay-at-home parents, retirees, or anyone needing a flexible work schedule that allows them to work from anywhere. However, one must note that consistency of work might not be guaranteed and contracts could be terminated without warning. Therefore, it’s crucial to have a backup plan or side hustles. Despite these caveats, my overall experience working at Appen has been positive, offering a significant learning experience and a considerable degree of flexibility.
Conclusion
Appen offers a valuable platform for individuals seeking flexible, remote work opportunities with a range of projects to choose from. The flexibility extends to both working hours and the freedom to set personal schedules. Although the pay is competitive, the consistency of work can vary and contracts might be terminated without prior notice. Therefore, while Appen presents a significant opportunity, it’s crucial for potential contractors to consider these factors and have backup plans or supplementary income streams in place.
For a comprehensive understanding of the roles I undertook, as well as an evaluation of their advantages and disadvantages, please visit Lifeafterfiftyish for an in-depth review.
#online jobs#over 50#work at home#easy jobs#Flexible Work#Job Review#Part-time Work#Independent Contractor#Gig Economy#Appen#remote work
12 notes
·
View notes
Text
What is Content Moderation and types of Moderation?

Successful brands all over the world have one thing in common: a thriving online community where the brand’s fans and influencers engage in online conversations that contribute high-value social media content, which in turn provides incredible insights into user behavior, preferences, and new business opportunities.
Content moderation is the process through which an online platform screens and monitors user-generated content to determine whether it should be published on the platform or not, based on platform-specific rules and guidelines. To put it another way, when a user submits content to a website, that content will go through a screening procedure (the moderation process) to make sure that the content upholds the regulations of the website, is not illegal, inappropriate, or harassing, etc.
From text-based content, ads, images, profiles, and videos to forums, online communities, social media pages, and websites, the goal of all types of content moderation is to maintain brand credibility and security for businesses and their followers online.
Types of content moderation
The content moderation method that you adopt should depend upon your business goals. At least the goal for your application or platform. Understanding the different kinds of content moderation, along with their strengths and weaknesses, can help you make the right decision that will work best for your brand and its online community.
Let’s discuss the different types of content moderation methods being used and then you can decide what is best for you.
Pre-moderation
All user submissions are placed in a queue for moderation before being presented on the platform, as the name implies. Pre-moderation ensures that no personally identifiable information, such as a comment, image, or video, is ever published on a website. However, for online groups that desire fast and unlimited involvement, this can be a barrier. Pre-moderation is best suited to platforms that require the highest levels of protection, like apps for children.
Post-moderation
Post-moderation allows users to publish their submissions immediately but the submissions are also added to a queue for moderation. If any sensitive content is found, it is taken down immediately. This increases the liability of the moderators because ideally there should be no inappropriate content on the platform if all content passes through the approval queue.
Reactive moderation
Platforms with a big community of cybercrime members allow users to flag any content that is offensive or violates community norms. This helps the moderators to concentrate on the content that has been flagged by the most people. However, this can enable for long-term distribution of sensitive content on a platform. It depends upon your business goals how long you can tolerate sensitive content to be on display.
Automated moderation
Automated moderation works by using specific content moderation applications to filter certain offensive words and multimedia content. Detecting inappropriate posts becomes automatic and more seamless. IP addresses of users classified as abusive can also be blocked through the help of automated moderation. Artificial intelligence systems can be used to analyze text, image, and video content. Finally, human moderators may be involved in the automated systems and flag something for their consideration.
Distributed moderation
Distributed moderation is accomplished by providing a rating system that allows the rest of the online community to score or vote on the content that has been uploaded. Although this is an excellent approach to crowdsourcing and ensuring that your community members are productive, it does not provide a high level of security.
Not only is your website exposed to abusive Internet trolls, it also relies on a slow self-moderation process that takes too much time for low-scoring harmful content to be brought to your attention.
TagX Content Moderation Services
At TagX, we strive to create the best possible content moderation solution by striking an optimum balance between your requirements and objectives.we understand that the future of content moderation involves an amalgamation of human judgment and evolving AI/ML capabilities.Our diverse workforce of data specialists, professional annotators, and social media experts come together to moderate a large volume of real-time content with the help of proven operational models.
Our content moderation services are designed to manage large volumes of real-time data in multiple languages while preserving quality, regulatory compliance, and brand reputation. TagX will build a dedicated team of content moderators who are trained and ready to be your brand advocates.
0 notes
Text
Unlock the potential of your AI models with accurate video transcription services. From precise annotations to seamless data preparation, transcription is essential for scalable AI training.
#video transcription services#video transcription#video data transcription#AI Training#Data Annotation#Accurate Transcription#Dataset Quality#AI Data Preparation#Machine Learning Training#Scalable AI Solutions
0 notes
Text
ChatGPT and Machine Learning: Advancements in Conversational AI

Introduction: In recent years, the field of natural language processing (NLP) has witnessed significant advancements with the development of powerful language models like ChatGPT. Powered by machine learning techniques, ChatGPT has revolutionized conversational AI by enabling human-like interactions with computers. This article explores the intersection of ChatGPT and machine learning, discussing their applications, benefits, challenges, and future prospects.
The Rise of ChatGPT: ChatGPT is an advanced language model developed by OpenAI that utilizes deep learning algorithms to generate human-like responses in conversational contexts. It is based on the underlying technology of GPT (Generative Pre-trained Transformer), a state-of-the-art model in NLP, which has been fine-tuned specifically for chat-based interactions.
How ChatGPT Works: ChatGPT employs a technique called unsupervised learning, where it learns from vast amounts of text data without explicit instructions or human annotations. It utilizes a transformer architecture, which allows it to process and generate text in a parallel and efficient manner.
The model is trained using a massive dataset and learns to predict the next word or phrase given the preceding context.
Applications of ChatGPT: Customer Support: ChatGPT can be deployed in customer service applications, providing instant and personalized assistance to users, answering frequently asked questions, and resolving common issues.
Virtual Assistants: ChatGPT can serve as intelligent virtual assistants, capable of understanding and responding to user queries, managing calendars, setting reminders, and performing various tasks.
Content Generation: ChatGPT can be used for generating content, such as blog posts, news articles, and creative writing, with minimal human intervention.
Language Translation: ChatGPT's language understanding capabilities make it useful for real-time language translation services, breaking down barriers and facilitating communication across different languages.
Benefits of ChatGPT: Enhanced User Experience: ChatGPT offers a more natural and interactive conversational experience, making interactions with machines feel more human-like.
Increased Efficiency: ChatGPT automates tasks that would otherwise require human intervention, resulting in improved efficiency and reduced response times.
Scalability: ChatGPT can handle multiple user interactions simultaneously, making it scalable for applications with high user volumes.
Challenges and Ethical Considerations: Bias and Fairness: ChatGPT's responses can sometimes reflect biases present in the training data, highlighting the importance of addressing bias and ensuring fairness in AI systems.
Misinformation and Manipulation: ChatGPT's ability to generate realistic text raises concerns about the potential spread of misinformation or malicious use. Ensuring the responsible deployment and monitoring of such models is crucial.
Future Directions: Fine-tuning and Customization: Continued research and development aim to improve the fine-tuning capabilities of ChatGPT, enabling users to customize the model for specific domains or applications.
Ethical Frameworks: Efforts are underway to establish ethical guidelines and frameworks for the responsible use of conversational AI models like ChatGPT, mitigating potential risks and ensuring accountability.
Conclusion: In conclusion, the emergence of ChatGPT and its integration into the field of machine learning has opened up new possibilities for human-computer interaction and natural language understanding. With its ability to generate coherent and contextually relevant responses, ChatGPT showcases the advancements made in language modeling and conversational AI.
We have explored the various aspects and applications of ChatGPT, including its training process, fine-tuning techniques, and its contextual understanding capabilities. Moreover, the concept of transfer learning has played a crucial role in leveraging the model's knowledge and adapting it to specific tasks and domains.
While ChatGPT has shown remarkable progress, it is important to acknowledge its limitations and potential biases. The continuous efforts by OpenAI to gather user feedback and refine the model reflect their commitment to improving its performance and addressing these concerns. User collaboration is key to shaping the future development of ChatGPT and ensuring it aligns with societal values and expectations.
The integration of ChatGPT into various applications and platforms demonstrates its potential to enhance collaboration, streamline information gathering, and assist users in a conversational manner. Developers can harness the power of ChatGPT by leveraging its capabilities through APIs, enabling seamless integration and expanding the reach of conversational AI.
Looking ahead, the field of machine learning and conversational AI holds immense promise. As ChatGPT and similar models continue to evolve, the focus should remain on user privacy, data security, and responsible AI practices. Collaboration between humans and machines will be crucial, as we strive to develop AI systems that augment human intelligence and provide valuable assistance while maintaining ethical standards.
With further advancements in training techniques, model architectures, and datasets, we can expect even more sophisticated and context-aware language models in the future. As the dialogue between humans and machines becomes more seamless and natural, the potential for innovation and improvement in various domains is vast.
In summary, ChatGPT represents a significant milestone in the field of machine learning, bringing us closer to human-like conversation and intelligent interactions. By harnessing its capabilities responsibly and striving for continuous improvement, we can leverage the power of ChatGPT to enhance user experiences, foster collaboration, and push the boundaries of what is possible in the realm of artificial intelligence.
2 notes
·
View notes