Note
The red spot is a chili flake
The red spot is a chili flake... (context)
#poorly drawn mdzs#mdzs#ask#jin ling#It makes so much sense...wwx stores the chilli in his cheek pouches for easy deployment. In case of Bland Food emergency.#This is how he survives living with the Lans.#And how he trains his new body how to tolerate spice.#Poor jin ling does not have this resistance. He is taking burn damage the entire walk back home. Someone rub high fat yogurt on that boy.#Just in case its not a universal common experience to prepare peppers with your bare hands (like a fool) and touch your face (LIKE A FOOL):#that stuff *burns*. I try to remember to wear gloves but alas. This year we grew Serranos! Tasty little guys but mistakes were made.#Dont process peppers with cuts on your hands. Or rub your eyes. Actually: please jut wear gloves while handling peppers.#I can eat those puppies raw no problem but I am miserable when it gets into my eyes.
432 notes
·
View notes
Text
lmfao i am beating my laptops ass rn 😭 i have 3MB of ram free, i need to convince my job to let me get a lil dedicated server to run at home.
#i have 50 laptops to setup and i think i got all the infrastructure and configurations i need to do like.#the basic task of pushing an image to laptops that r connected. now doing that Faster? 😅#i need to read more but also need to figure out where my bottleneck is bc one laptops getting it at 1.3GB/m and the other is#about 835MB/m. the image was shrunk too but it's like 38GB so uh. yea lol.#im using hyper v with pfsense as a firewall and a fog server for pxe/image deployment and they're doing DHCP for the physical LAN#the laptops are on. but that lan is just usb to ethernet connections and an unmanaged switch.#do i happen to have any computer nerd followers who know what im talking about and could give some tips on speeding this up?#otherwise I'll just keep looking into it after lunch since the laptops r at least Doing Something now :p#don't tell me about MDT unless you're about to make the end to end process dead easy and straight forward for me 😤#omg some D dude was talking shit over fia's body after i rested at the grace site?? my boy.. buddy#i killed him swiftly do Not trash talk my wife 😤
6 notes
·
View notes
Text
"Faced with the possibility of a given author's deliberate opacity, what responsibility does the translator then have to allow for the possibility of such active resistance? How can the translator fulfill her obligation to render a text legible while respecting the refusals built into the language of a source text from a 'minority' culture? Moreover, to what extent do matters of race and/or/as culture determine the parameters of the translator's accountability to the author she seeks to carry over into another world(view)?"
Kaiama L. Glover, "Blackness" in French: On Translation, Haiti, and the Matter of Race
#ngl the intentional opacity questions definitely resonate with me#less in a cultural sense (tho still not absent there *gestures at cultural xianity*) -#but in a certain similarity with my feelings about gender/queer/nonbinary stuff and also neurodivergence#(the latter of which is particularly Thinky Thoughts bc there's a frequent framing in translation stuff about Access)#(and Who Will Find A Version Of A Text Accessible)#where that's like - so often a deployment of colonialist attitudes & processes#contrast obviously disability contexts where access is a good thing to actively be fought for#it feels... significant I think to carry simultaneous contrasting valences & purposes of the “same” concept#not least for the sense of... not falling into the trap of assuming (via my pov here) that such a thing can ONLY ever be a positive outcome#James liveblogs books#Violent Phenomena#quotations
5 notes
·
View notes
Text
IBM unveils Granite 3.0 AI models with open-source commitment
New Post has been published on https://thedigitalinsider.com/ibm-unveils-granite-3-0-ai-models-with-open-source-commitment/
IBM unveils Granite 3.0 AI models with open-source commitment
.pp-multiple-authors-boxes-wrapper display:none; img width:100%;
IBM has taken the wraps off its most sophisticated family of AI models to date, dubbed Granite 3.0, at the company’s annual TechXchange event.
The Granite 3.0 lineup includes a range of models designed for various applications:
General purpose/language: 8B and 2B variants in both Instruct and Base configurations
Safety: Guardian models in 8B and 2B sizes, designed to implement guardrails
Mixture-of-Experts: A series of models optimised for different deployment scenarios
IBM claims that its new 8B and 2B language models can match or surpass the performance of similarly sized offerings from leading providers across numerous academic and industry benchmarks. These models are positioned as versatile workhorses for enterprise AI, excelling in tasks such as Retrieval Augmented Generation (RAG), classification, summarisation, and entity extraction.
A key differentiator for the Granite 3.0 family is IBM’s commitment to open-source AI. The models are released under the permissive Apache 2.0 licence, offering a unique combination of performance, flexibility, and autonomy to both enterprise clients and the broader AI community.
IBM believes that by combining a compact Granite model with proprietary enterprise data, particularly using their novel InstructLab alignment technique, businesses can achieve task-specific performance rivalling larger models at a fraction of the cost. Early proofs-of-concept suggest potential cost savings of up to 23x less than large frontier models.
According to IBM, transparency and safety remain at the forefront of its AI strategy. The company has published a technical report and responsible use guide for Granite 3.0, detailing the datasets used, data processing steps, and benchmark results. Additionally, IBM offers IP indemnity for all Granite models on its watsonx.ai platform, providing enterprises with greater confidence when integrating these models with their own data.
The Granite 3.0 8B Instruct model has shown particularly promising results, outperforming similar-sized open-source models from Meta and Mistral on standard academic benchmarks. It also leads across all measured safety dimensions on IBM’s AttaQ safety benchmark.
IBM is also introducing the Granite Guardian 3.0 models, designed to implement safety guardrails by checking user prompts and LLM responses for various risks. These models offer a comprehensive set of risk and harm detection capabilities, including unique checks for RAG-specific issues such as groundedness and context relevance.
The entire suite of Granite 3.0 models is available for download on HuggingFace, with commercial use options on IBM’s watsonx platform. IBM has also collaborated with ecosystem partners to integrate Granite models into various offerings, providing greater choice for enterprises worldwide.
As IBM continues to advance its AI portfolio, the company says it’s focusing on developing more sophisticated AI agent technologies capable of greater autonomy and complex problem-solving. This includes plans to introduce new AI agent features in IBM watsonx Orchestrate and build agent capabilities across its portfolio in 2025.
See also: Scoring AI models: Endor Labs unveils evaluation tool
Want to learn more about AI and big data from industry leaders? Check out AI & Big Data Expo taking place in Amsterdam, California, and London. The comprehensive event is co-located with other leading events including Intelligent Automation Conference, BlockX, Digital Transformation Week, and Cyber Security & Cloud Expo.
Explore other upcoming enterprise technology events and webinars powered by TechForge here.
Tags: ai, artificial intelligence, enterprise, granite 3, ibm, large language models, llm, models, techxchange
#agent#ai#ai & big data expo#ai agent#AI models#ai platform#AI strategy#amp#Apache#applications#Articles#artificial#Artificial Intelligence#automation#benchmark#benchmarks#Big Data#california#Cloud#Community#Companies#comprehensive#conference#cost savings#cyber#cyber security#data#data processing#datasets#deployment
0 notes
Text
Mastering Azure Container Apps: From Configuration to Deployment
Thank you for following our Azure Container Apps series! We hope you're gaining valuable insights to scale and secure your applications. Stay tuned for more tips, and feel free to share your thoughts or questions. Together, let's unlock the Azure's Power.
#API deployment#application scaling#Azure Container Apps#Azure Container Registry#Azure networking#Azure security#background processing#Cloud Computing#containerized applications#event-driven processing#ingress management#KEDA scalers#Managed Identities#microservices#serverless platform
0 notes
Text
Scaling Node.js Applications with PM2
Scaling Node.js Applications with PM2: A Comprehensive Guide
Introduction As your Node.js application grows, you may need to scale it to handle increased traffic and ensure reliability. PM2 (Process Manager 2) is a powerful process manager for Node.js applications that simplifies deployment, management, and scaling. It provides features such as process monitoring, log management, and automatic restarts, making it an essential tool for production…
#application scaling#deployment#DevOps practices#monitoring#Node.js#Node.js scaling#PM2#process management#web development
0 notes
Text
Jamshedpur Ramps Up Security For Muharram Observances
Over 2,300 Police Personnel Deployed, Drone Surveillance Planned Administration conducts flag march and anti-riot drills to ensure peaceful processions. JAMSHEDPUR – The district administration has implemented extensive security measures for Muharram observances, deploying over 2,300 armed police personnel across the city. "We’ve strategically positioned forces in sensitive areas like Mango,…
#Anti-Riot Drills Jamshedpur#मुख्य#Drone Surveillance Jamshedpur#Featured#Flag March Steel City#Heavy Vehicle Restrictions#Jamshedpur Muharram Security#Jamshedpur police deployment#Jamshedpur religious events#Muharram Procession Safety#Public Safety Measures#SSP Kishore Kaushal
0 notes
Text
0 notes
Text
Future Of AI In Software Development
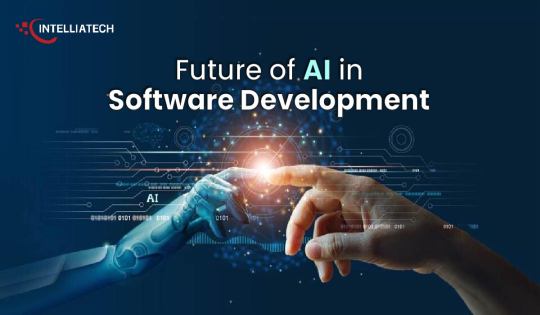
The usage of AI in Software Development has seen a boom in recent years and it will further continue to redefine the IT industry. In this blog post, we’ll be sharing the existing scenario of AI, its impacts and benefits for software engineers, future trends and challenge areas to help you give a bigger picture of the performance of artificial intelligence (AI). This trend has grown to the extent that it has become an important part of the software development process. With the rapid evolvements happening in the software industry, AI is surely going to dominate.
Read More
#Accountability#Accuracy Accuracy#Advanced Data Analysis#artificial intelligence#automated testing#Automation#bug detection#code generation#code reviews#continuous integration#continuous deployment#cost savings#debugging#efficiency#Enhanced personalization#Ethical considerations#future trends#gartner report#image generation#improved productivity#job displacement#machine learning#natural language processing#privacy privacy#safety#security concerns#software development#software engineers#time savings#transparency
0 notes
Text
Simplifying Processes with Microlearning: The Power of 'What, Why, How' Scroll Down Design
In the fast-paced world of corporate training and education, microlearning has emerged as a game-changer. Its bite-sized approach to learning makes it ideal for explaining complex processes in a simple and convenient way. One effective technique is the 'What, Why, How' scroll down design, which breaks down information into easily digestible chunks. This article explores how this design can be used to streamline processes and upskill your workforce efficiently.
Understanding the 'What, Why, How' Scroll Down Design
The 'What, Why, How' scroll down design is a structured approach to presenting information. It begins by explaining 'what' a process or concept is, followed by 'why' it is important or relevant, and concludes with 'how' it can be implemented or applied. This linear progression helps learners grasp the material more effectively by providing context and practical guidance.
What: This section introduces the process or concept being discussed. It provides a brief overview of what it entails, setting the stage for further exploration.
Why: Here, the importance or significance of the process is explained. Learners are given insight into why they need to understand and apply this knowledge in their work or daily lives.
How: This section offers practical steps or instructions on how to implement the process. It breaks down the process into actionable steps, making it easier for learners to follow along and apply what they've learned.
Leveraging Microlearning for Processes and Upskilling
Microlearning is ideally suited for explaining processes and situations that require practical and linear approaches. Here's how the 'What, Why, How' scroll down design can be effectively utilized in microlearning:
1. Process Explanation:
Imagine you need to train your employees on a new software deployment process. Using microlearning with the 'What, Why, How' design, you can break down the process into manageable chunks:
What: Introduce the new software deployment process, explaining its key features and objectives.
Why: Highlight the benefits of the new process, such as increased efficiency, reduced errors, and improved collaboration.
How: Provide step-by-step instructions on how to execute the software deployment process, including screenshots or video tutorials for visual learners.
2. Upskilling Scenarios:
Suppose your workforce needs to upskill in customer service techniques. Microlearning with the 'What, Why, How' design can help them quickly learn and apply new skills:
What: Introduce the customer service techniques to be learned, such as active listening, empathy, and problem-solving.
Why: Explain why these techniques are crucial for providing exceptional customer service, such as building customer loyalty and satisfaction.
How: Provide practical tips and examples on how to apply these techniques in various customer interactions, such as handling complaints or inquiries.
Benefits of the 'What, Why, How' Scroll Down Design in Microlearning
Clarity and Structure: The linear progression of the 'What, Why, How' design provides learners with a clear and structured framework for understanding complex processes.
Contextual Understanding: By explaining the 'why' behind a process, learners gain a deeper understanding of its significance and relevance to their roles.
Actionable Guidance: The 'how' section offers practical steps and instructions that learners can immediately apply in their work or daily lives.
Engagement and Retention: Microlearning's bite-sized format and interactive elements keep learners engaged and facilitate better retention of information.
Accessibility and Flexibility: Microlearning modules can be accessed anytime, anywhere, allowing learners to upskill at their own pace and convenience.
Implementing the 'What, Why, How' Scroll Down Design: A Case Study
Let's consider a manufacturing company implementing a new quality control process. They decide to use microlearning with the 'What, Why, How' scroll down design to train their employees effectively:
What: The module introduces the new quality control process, explaining its objectives and key components.
Why: It emphasizes the importance of quality control in ensuring product reliability, customer satisfaction, and brand reputation.
How: Practical guidelines and examples are provided on how employees can implement the quality control process in their day-to-day tasks, including inspection procedures and documentation requirements.
Conclusion
Microlearning with the 'What, Why, How' scroll down design offers a simple yet powerful approach to explaining processes and upskilling your workforce. By breaking down information into easily digestible chunks and providing context and practical guidance, this design enhances understanding, engagement, and retention. Whether you're introducing new procedures, implementing software changes, or upskilling employees in essential techniques, microlearning with the 'What, Why, How' design can help streamline processes and drive meaningful change within your organization. Embrace this approach to empower your workforce and stay ahead in today's dynamic business environment.
#Microlearning#'What#Why#How'#Scroll down design#Process explanation#Upskilling#Linear progression#Contextual understanding#Actionable guidance#Engagement#Retention#Accessibility#Flexibility#Training modules#Quality control#Manufacturing#Software deployment#Customer service#Training techniques#Employee development#Learning objectives#Practical guidance#Interactive elements#Case study#Learning outcomes#Training effectiveness#Learning structure#Bite-sized format#Training materials
0 notes
Text
youtube
#youtube#militarytraining#Joint Base Lewis-McChord#HIMARS#Logistics#Military#Loading Process#Army#Loading#Air Force#Aircraft#Military Base#Deployment#Military Training#Transport#Heavy Equipment#Equipment#Military Vehicles#Military Operations#Humvees#C-17 Globemaster III#Military Transport#Military Collaboration#Plane#Joint Operations#Military Logistics#Military Deployment#Transportation#Military Support#Military Aircraft
1 note
·
View note
Text
Discover how AI writing is revolutionizing conversations, unlocking new possibilities with large language models at the forefront.
#AI Innovations#Conversational Chatbots#Deployment Of LLMs#Human-Machine Interactions#Large Language Models#Natural Language Processing#NLP Models#Virtual Assistants
0 notes
Text
Discover how AI writing is revolutionizing conversations, unlocking new possibilities with large language models at the forefront.
#AI Innovations#Conversational Chatbots#Deployment Of LLMs#Human-Machine Interactions#Large Language Models#Natural Language Processing#NLP Models#Virtual Assistants
0 notes
Text
Discover how AI writing is revolutionizing conversations, unlocking new possibilities with large language models at the forefront.
#AI Innovations#Conversational Chatbots#Deployment Of LLMs#Human-Machine Interactions#Large Language Models#Natural Language Processing#NLP Models#Virtual Assistants
0 notes
Text
Refining Intelligence: The Strategic Role of Fine-Tuning in Advancing LLaMA 3.1 and Orca 2
New Post has been published on https://thedigitalinsider.com/refining-intelligence-the-strategic-role-of-fine-tuning-in-advancing-llama-3-1-and-orca-2/
Refining Intelligence: The Strategic Role of Fine-Tuning in Advancing LLaMA 3.1 and Orca 2
In today’s fast-paced Artificial Intelligence (AI) world, fine-tuning Large Language Models (LLMs) has become essential. This process goes beyond simply enhancing these models and customizing them to meet specific needs more precisely. As AI continues integrating into various industries, the ability to tailor these models for particular tasks is becoming increasingly important. Fine-tuning improves performance and reduces the computational power required for deployment, making it a valuable approach for both organizations and developers.
Recent advancements, such as Meta’s Llama 3.1 and Microsoft’s Orca 2, demonstrate significant progress in AI technology. These models represent cutting-edge innovation, offering enhanced capabilities and setting new benchmarks for performance. As we examine the developments of these state-of-the-art models, it becomes clear that fine-tuning is not merely a technical process but a strategic tool in the rapidly emerging AI discipline.
Overview of Llama 3.1 and Orca 2
Llama 3.1 and Orca 2 represent significant advancements in LLMs. These models are engineered to perform exceptionally well in complex tasks across various domains, utilizing extensive datasets and advanced algorithms to generate human-like text, understand context, and generate accurate responses.
Meta’s Llama 3.1, the latest in the Llama series, stands out with its larger model size, improved architecture, and enhanced performance compared to its predecessors. It is designed to handle general-purpose tasks and specialized applications, making it a versatile tool for developers and businesses. Its key strengths include high-accuracy text processing, scalability, and robust fine-tuning capabilities.
On the other hand, Microsoft’s Orca 2 focuses on integration and performance. Building on the foundations of its earlier versions, Orca 2 introduces new data processing and model training techniques that enhance its efficiency. Its integration with Azure AI simplifies deployment and fine-tuning, making it particularly suited for environments where speed and real-time processing are critical.
While both Llama 3.1 and Orca 2 are designed for fine-tuning specific tasks, they approach this differently. Llama 3.1 emphasizes scalability and versatility, making it suitable for various applications. Orca 2, optimized for speed and efficiency within the Azure ecosystem, is better suited for quick deployment and real-time processing.
Llama 3.1’s larger size allows it to handle more complex tasks, though it requires more computational resources. Orca 2, being slightly smaller, is engineered for speed and efficiency. Both models highlight Meta and Microsoft’s innovative capabilities in advancing AI technology.
Fine-Tuning: Enhancing AI Models for Targeted Applications
Fine-tuning involves refining a pre-trained AI model using a smaller, specialized dataset. This process allows the model to adapt to specific tasks while retaining the broad knowledge it gained during initial training on larger datasets. Fine-tuning makes the model more effective and efficient for targeted applications, eliminating the need for the extensive resources required if trained from scratch.
Over time, the approach to fine-tuning AI models has significantly advanced, mirroring the rapid progress in AI development. Initially, AI models were trained entirely from scratch, requiring vast amounts of data and computational power—a time-consuming and resource-intensive method. As the field matured, researchers recognized the efficiency of using pre-trained models, which could be fine-tuned with smaller, task-specific datasets. This shift dramatically reduced the time and resources needed to adapt models to new tasks.
The evolution of fine-tuning has introduced increasingly advanced techniques. For example, Meta’s LLaMA series, including LLaMA 2, uses transfer learning to apply knowledge from pre-training to new tasks with minimal additional training. This method enhances the model’s versatility, allowing it to handle a wide range of applications precisely.
Similarly, Microsoft’s Orca 2 combines transfer learning with advanced training techniques, enabling the model to adapt to new tasks and continuously improve through iterative feedback. By fine-tuning smaller, tailored datasets, Orca 2 is optimized for dynamic environments where tasks and requirements frequently change. This approach demonstrates that smaller models can achieve performance levels comparable to larger ones when fine-tuned effectively.
Key Lessons from Fine-Tuning LLaMA 3.1 and Orca 2
The fine-tuning of Meta’s LLaMA 3.1 and Microsoft’s Orca 2 has yielded important lessons in optimizing AI models for specific tasks. These insights emphasize the essential role that fine-tuning plays in improving model performance, efficiency, and adaptability, offering a deeper understanding of how to maximize the potential of advanced AI systems in various applications.
One of the most significant lessons from fine-tuning LLaMA 3.1 and Orca 2 is the effectiveness of transfer learning. This technique involves refining a pre-trained model using a smaller, task-specific dataset, allowing it to adapt to new tasks with minimal additional training. LLaMA 3.1 and Orca 2 have demonstrated that transfer learning can substantially reduce the computational demands of fine-tuning while maintaining high-performance levels. LLaMA 3.1, for example, uses transfer learning to enhance its versatility, making it adaptable to a wide range of applications with minimal overhead.
Another critical lesson is the need for flexibility and scalability in model design. LLaMA 3.1 and Orca 2 are engineered to be easily scalable, enabling them to be fine-tuned for various tasks, from small-scale applications to large enterprise systems. This flexibility ensures that these models can be adapted to meet specific needs without requiring a complete redesign.
Fine-tuning also reflects the importance of high-quality, task-specific datasets. The success of LLaMA 3.1 and Orca 2 highlights the necessity of investing in creating and curating relevant datasets. Obtaining and preparing such data is a significant challenge, especially in specialized domains. Without robust, task-specific data, even the most advanced models may struggle to perform optimally when fine-tuned for particular tasks.
Another essential consideration in fine-tuning large models like LLaMA 3.1 and Orca 2 is balancing performance with resource efficiency. Though fine-tuning can significantly enhance a model’s capabilities, it can also be resource-intensive, especially for models with large architectures. For instance, LLaMA 3.1’s larger size allows it to handle more complex tasks but requires more computational power. Conversely, Orca 2’s fine-tuning process emphasizes speed and efficiency, making it a better fit for environments where rapid deployment and real-time processing are essential.
The Broader Impact of Fine-Tuning
The fine-tuning of AI models such as LLaMA 3.1 and Orca 2 has significantly influenced AI research and development, demonstrating how fine-tuning can enhance the performance of LLMs and drive innovation in the field. The lessons learned from fine-tuning these models have shaped the development of new AI systems, placing greater emphasis on flexibility, scalability, and efficiency.
The impact of fine-tuning extends far beyond AI research. In practice, fine-tuned models like LLaMA 3.1 and Orca 2 are applied across various industries, bringing tangible benefits. For example, these models can offer personalized medical advice, improve diagnostics, and enhance patient care. In education, fine-tuned models create adaptive learning systems tailored to individual students, providing personalized instruction and feedback.
In the financial sector, fine-tuned models can analyze market trends, offer investment advice, and manage portfolios more accurately and efficiently. The legal industry also benefits from fine-tuned models that can draft legal documents, provide legal counsel, and assist with case analysis, thereby improving the speed and accuracy of legal services. These examples highlight how fine-tuning LLMs like LLaMA 3.1 and Orca 2 drives innovation and improves efficiency across various industries.
The Bottom Line
The fine-tuning of AI models like Meta’s LLaMA 3.1 and Microsoft’s Orca 2 highlights the transformative power of refining pre-trained models. These advancements demonstrate how fine-tuning can enhance AI performance, efficiency, and adaptability, with far-reaching impacts across industries. The benefits of personalized healthcare are clear, as are adaptive learning and improved financial analysis.
As AI continues to evolve, fine-tuning will remain a central strategy. This will drive innovation and enable AI systems to meet the diverse needs of our rapidly changing world, paving the way for smarter, more efficient solutions.
#Advice#ai#AI development#ai model#AI models#AI research#AI systems#Algorithms#Analysis#applications#approach#architecture#Art#artificial#Artificial Intelligence#azure#benchmarks#Building#challenge#change#cutting#data#data processing#datasets#deployment#Design#developers#development#Developments#diagnostics
0 notes
Text
Discover how AI writing is revolutionizing conversations, unlocking new possibilities with large language models at the forefront.
#AI Innovations#Conversational Chatbots#Deployment Of LLMs#Human-Machine Interactions#Large Language Models#Natural Language Processing#NLP Models#Virtual Assistants
0 notes