#WeatherData
Explore tagged Tumblr posts
Text
#AccurateDeals#HomeWeatherMonitoring#BestWeatherStations#HomeWeatherProducts#ComprehensiveGuide#WeatherMonitoring#SmartHomeDevices#WeatherTech#WeatherInstruments#HomeWeatherSystems#WeatherTracking#SmartHomeWeather#WeatherForecasting#WeatherMonitoringGuide#WeatherStationDeals#AccurateWeatherDevices#WeatherGadgets#HomeMonitoring#WeatherAccuracy#WeatherData#WeatherStationReviews#BestHomeMonitoring#SmartWeatherSystems#HomeWeatherAccuracy#WeatherStationBuyingGuide#WeatherSensorTechnology#WeatherMonitoringDevices#HomeWeatherTracking#WeatherProtection#WeatherAlertSystems
1 note
·
View note
Text
Reading the skies like a pro: Weather forecasting tech heading for $4.33B ☁️🔮
Weather Forecasting Systems Market is projected to grow from $2.29 billion in 2024 to $4.33 billion by 2034, with a CAGR of 6.6%. This market includes a wide range of technologies and services that analyze atmospheric conditions — such as weather satellites, radar systems, predictive analytics software, and data acquisition tools. These systems support industries like agriculture, aviation, transportation, and energy by enabling more accurate decision-making, improved safety, and operational efficiency.
To Request Sample Report : https://www.globalinsightservices.com/request-sample/?id=GIS20396 &utm_source=SnehaPatil&utm_medium=Article
The hardware segment leads the market, driven by the rising demand for advanced sensors and weather stations to gather precise environmental data. Software solutions, including real-time forecasting and predictive analytics platforms, follow closely, reflecting a shift toward intelligent weather modeling. North America dominates due to strong R&D investment and infrastructure, while Europe emerges as a fast-growing region, especially in the renewable energy sector. The U.S. and Germany are top-performing countries, thanks to their tech capabilities and focus on sustainability. With growing climate variability and extreme weather events, industries are rapidly adopting smart weather forecasting systems, making this a high-growth market.
#weatherforecasting #forecastingsystems #meteorologytech #climateanalytics #remotesensing #numericalprediction #weatherdata #climatechange #environmentaltech #weathermodels #sensors #bigdataweather #aiweather #mlforecasting #smartweather #radarsystems #weathersatellites #dataanalytics #nowcasting #cloudbasedforecasting #aviationweather #agriculturetech #energyforecasting #marineforecasting #defensetech #environmentalsafety #weatherinstruments #temperaturemonitoring #humiditymonitoring #windmonitoring #precipitationtracking #dataacquisition #weatherapps #realtimeforecasting #weatherinsights
Research Scope:
· Estimates and forecast the overall market size for the total market, across type, application, and region
· Detailed information and key takeaways on qualitative and quantitative trends, dynamics, business framework, competitive landscape, and company profiling
· Identify factors influencing market growth and challenges, opportunities, drivers, and restraints
· Identify factors that could limit company participation in identified international markets to help properly calibrate market share expectations and growth rates
· Trace and evaluate key development strategies like acquisitions, product launches, mergers, collaborations, business expansions, agreements, partnerships, and R&D activities
About Us:
Global Insight Services (GIS) is a leading multi-industry market research firm headquartered in Delaware, US. We are committed to providing our clients with highest quality data, analysis, and tools to meet all their market research needs. With GIS, you can be assured of the quality of the deliverables, robust & transparent research methodology, and superior service.
Contact Us:
Global Insight Services LLC 16192, Coastal Highway, Lewes DE 19958 E-mail: [email protected] Phone: +1–833–761–1700 Website: https://www.globalinsightservices.com/
0 notes
Text
The Mystery of Rain | How Turbulence Shapes Our Weather
https://www.youtube.com/watch?v=_WvDy2vlqO4 Understanding how rain forms is crucial for improving weather and climate predictions. In this video, we explore groundbreaking research by the NSF National Center for Atmospheric Research (NCAR) that reveals how turbulence in clouds speeds up rain formation. By using advanced computer models and data from NASA’s field campaigns, scientists have uncovered key insights that could revolutionize weather forecasting. Dive into this exciting discovery and learn how it could lead to more accurate climate projections, better preparing us for the future. Source: National Center for Atmospheric Research. #atmosphericscience #meteorology #rain #weather #trendstorm #weatherscience #climatechange #rainforcast #turbulence #weatherforecasting #cumulusclouds #raindropscreation #environmentalscience #clouds #earthscience #nasaresearch #NSFScience #cloudformation #weatheranalysis #precipitation #weatherpatterns #hydrology #weathertech #earthatmosphere #weatherdata #environmentalresearch #scientificbreakthroughs #climatetech #computersimulation How does turbulence affect rain formation? The role of turbulence in weather prediction Understanding rain formation bottleneck Rain and cloud turbulence explained How do clouds form rain? Turbulence in cumulus clouds Advanced computer models for weather NASA's role in studying cloud formation The mystery of raindrop formation Rain formation and weather forecasting How does turbulence speed up rain? Cumulus clouds and turbulence The science behind rain initiation How weather models predict rain The impact of cloud condensation nuclei How do droplets turn into rain? NSF NCAR rain study Turbulence and rainwater mass The importance of turbulence in weather How computer simulations enhance forecasts NASA field campaign on rain formation How are raindrops formed in clouds? Turbulence's effect on cloud droplets Predicting rain with advanced models The physics of rain formation How weather models are improved Turbulence and rainfall amounts Rain formation in cumulus congestus clouds The role of turbulence in climate models Understanding cloud dynamics How turbulence impacts weather systems The science of droplet coalescence The future of weather forecasting How cloud particles affect rainfall Rainfall prediction and turbulence Turbulence's role in weather patterns Exploring cloud formation processes The connection between turbulence and rain High-resolution weather simulations The role of NASA in studying rain Understanding rain through computer models The process of raindrop growth Turbulence in atmospheric research Rain formation in different cloud types How clouds produce rain The science behind rain initiation Turbulence's impact on weather models How computer models predict rainfall The evolution of raindrops The significance of cloud condensation nuclei How turbulence influences weather forecasts The role of turbulence in the atmosphere The mystery behind rain formation Predicting rain with advanced simulations The relationship between clouds and rain How turbulence affects raindrop size The science of weather prediction Understanding cloud condensation nuclei The role of NSF NCAR in weather research Rain formation in turbulent clouds How weather models simulate rain The connection between turbulence and rainfall Exploring rain formation in clouds The impact of turbulence on weather systems Advanced simulations in weather research Understanding the rain formation bottleneck The science behind cloud and rain interaction Predicting rain with turbulence models How raindrops form in the atmosphere The physics of cloud and rain formation via Trend Storm https://www.youtube.com/channel/UCF1F2JAMftAe2z2hl32FXmQ August 21, 2024 at 05:30PM
0 notes
Link
0 notes
Text

WORLD METEOROLODICAL DAY
On World Meteorological Day, we the crucial role that meteorology plays in daily lives. Meteorology impacts everything from agriculture to transportation, and understanding the weather is essential for predicting natural disasters and protecting lives. In this video, we explore the importance of World Meteorological Day and the significant work done meteorologists around the world to keep us safe and informed.
Call:77997 99221 Webdite: www.manasadefenceacademy.com
#worldmeteorologicalday#meteorology#weatherforecast#climatescience#naturaldisasters#weatherpatterns#weatherprediction#atmosphericscience#weathermonitoring#climateresearch#climatechange#meteorologists#weatherdata#globalweather#climatology#worldweather#forecastingtools#weathertechnology#weatherobservations#weatherpatternsanalysis
0 notes
Photo
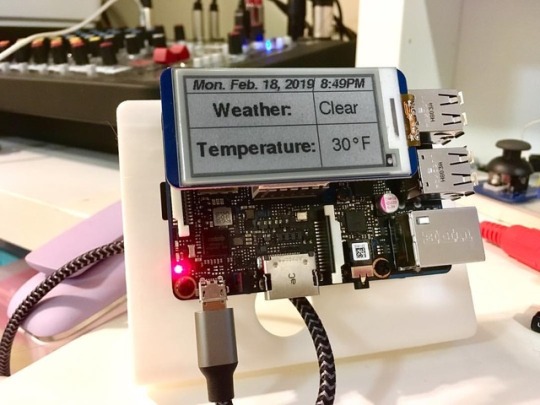
Tinker Board e-Paper weather project finally has a permanent home ⛈ and in case you missed the video on this project 👉 https://youtu.be/6e0xSb1qUTY #diy #tech #electronics #hardware #opensource #epaper #eink #weather #iot #weatherdata #python #owm #openweathermap #make #makersgonnamake #makersmovement #linux #opensource #tinkerboard #asustinkerboard #singleboardcomputer #sbc #3dprinting #nerdlife https://www.instagram.com/p/BuC7KEznUmB/?utm_source=ig_tumblr_share&igshid=xv95ysym5fv7
#diy#tech#electronics#hardware#opensource#epaper#eink#weather#iot#weatherdata#python#owm#openweathermap#make#makersgonnamake#makersmovement#linux#tinkerboard#asustinkerboard#singleboardcomputer#sbc#3dprinting#nerdlife
4 notes
·
View notes
Text

Google Maps can now tell you about air quality in your area, just in time for summer.
Air quality ratings range from zero to "400+," with lower numbers being better. To view ratings in the Google Maps app, tap the layers icon near the top right corner, then select Air Quality under Map details. The data comes from government agencies like the EPA, Google says, plus hyper-local air quality data provider PurpleAir.
This feature and one that adds a Maps layer showing live weather data were initially intended to launch "in the coming months"
0 notes
Text
#trendingnews#trending#viralpost#viral#entertainment#trendingtales#weatherdata#tornadoes#unitedstates#outbreaks#internet#viral news stories#trending news today
1 note
·
View note
Photo

We think of those who have warned us of atmospheric dangers and delights. Storms or shine, all the time, they tell us what we need to know to be prepared for nature's whims. This day says, we appreciate you and your team all your hard work and dedication to your science. #digitalmarketing #weatherdata Thank you for both images. https://www.vecteezy.com https://www.instagram.com/p/CK7buDkleUq/?igshid=1ecikwqays0lc
0 notes
Photo
Adding more things so people can actually understand the graphic. (All temperatures of 2016 in the Netherlands)
#pttrnz#graph#datavisuali#weather#weatherdata#data#computer#processing#p5#illustrator#improving#experiment#proces#creativecode#code#computerart
69 notes
·
View notes
Link
We've released all of the data sets we used when working on our weather prediction project. This includes Taipei MRT data, work/school cancellations data, and weather data.
0 notes
Photo

Les Brenda. Weerdata Rotterdam, september 2016 - augustus 2017
* Hardste windstoot * Hoogste temperatuur * Gemiddelde windsnelheid
0 notes
Photo

@fscjofficial will be close Friday and Monday. @fscjllc library has a good collection of books related to #hurricanes, #storms, #weather, etc. Tonight we will explore #hurricanemodels #weatherdata and #jaxready website. #drjosecampus #hurricaneirma #hurricanejose2017 #hurricanejose (at FSCJ Deerwood)
#hurricanes#drjosecampus#jaxready#hurricanemodels#weatherdata#weather#hurricaneirma#hurricanejose2017#hurricanejose#storms
0 notes
Text
Testing with mocks
I love Objective-C. Swift is also wonderful, but Objective-C is spectacular.
One thing I miss from Objective-C is dynamic patching. It was wonderful for mocking behaviors in testing. OCMock was awesome. And when I’ve found OHHTTPStubs I couldn’t stop myself to add it to all my projects.
Swift is far more protective. Ok, it’s true since Swift app runs inside the Objective-C runtime, is possible to manipulate the dispatching of messages at runtime. And I think that’s for the better. Let’s remove OCMock and OHHTTPStubs from the cart file and let’s think how we can mock using Swift. Because mock is absolutely necessary.
Mocking the network
Perhaps, the canonical example for mocking is networking. To test against the Net is hard, expensive, and it could slow tests a lot. Let’s revisited, for instance, func fetchCurrentWeatherData(input:completionHandler:) from the weather app.
Sure, we could call it from the test suite, and we can inject an URL to make it fail, and then, a point the task to an URL that makes it pass.
let task = session.dataTask(with: request) { (data: Data?, _: URLResponse?, error: Error?) in guard error == nil else { let networkError = NetworkControllerError.forwarded(error!) let payload = Either<NetworkControllerError, WeatherData>.left(networkError) completionHandler(payload) return } guard let jsonData = data else { let payloadError = NetworkControllerError.invalidPayload(url) let payload = Either<NetworkControllerError, WeatherData>.left(payloadError) completionHandler(payload) return } self.decode(jsonData: jsonData, endpointURL: url, temperatureUnit: input.unit, completionHandler: { (result: Either<NetworkControllerError, WeatherData>) in completionHandler(result) }) } task.resume()
Obviously, it will take a lot of time to make the trips to the server, we would be loading its load, and besides, there is a principle at stake. Aren’t we supposed to be doing Unit Test? Well, if that’s the case, by doing something like
func testOpenWeatherMap() { let exp = expectation(description: "Get weather data") let controller = OpenWeatherMapNetworkController() let input = Input(location: "Campana", unit: TemperatureUnit.metric) controller.fetchCurrentWeatherData(input: input) { (result: Either<NetworkControllerError, WeatherData>) in switch result { case .left: XCTFail("no data returned by fetchWeatherData()") case .right(let data): let city = input.location print("Weather in \(city): \(data.condition), \(data.temperature)\(data.unit)") exp.fulfill() } } waitForExpectations(timeout: 10, handler: nil) }
We would be testing two things, the network, and the function itself. Not one, but two. The word “Unit” is no longer applicable.
We need to abstract the actual network interactions. At the very basic, we need to inject our own closure for func dataTask(with request:completionHandler:) -> URLSessionDataTask. Obviously, we can subclass URLSession to introduce our own version of the method. But, we also need to mock URLSessionDataTask, to hijack the whole interaction. Let’s mock URLSessionDataTask first.
class MockURLSessionDataTask: URLSessionDataTask { // I know, against Apple naming convention, // but I rather have the `Mock` part right on front // to prevent autocompletion to introduce errors. private let closure: () -> Void init(closure: @escaping () -> Void) { self.closure = closure } override func resume() { self.closure() } }
Pretty self-explanatory. We save the closure we want to pass when the task is done, and then, we just call it from our overrode resume
Now, let’s mock the session.
class MockURLSession: URLSession { typealias CompletionHandler = (Data?, URLResponse?, Error?) -> Void var data: Data? var error: Error? var response: URLResponse? override func dataTask(with request: URLRequest, completionHandler: @escaping CompletionHandler) -> URLSessionDataTask { let data = self.data let response = self.response let error = self.error let dataTask = MockURLSessionDataTask(closure: { completionHandler(data, response, error) }) return dataTask } }
Again, we have ivars for data, error and response. We can set them as we wish to exercise different portions of our code. Do we want to make sure the guard is kicked on when an error is there? Just create a session, set the error, and test.
func testOpenWeatherShouldFailWithError() { let exp = expectation(description: "Get weather data") let session = MockURLSession() let error = NSError(domain: "asd", code: 123, userInfo: nil) session.error = error as Error self.prepareRetreiveWeatherSessionDataSucess(session) let controller = OpenWeatherMapNetworkController(session: session) let input = Input(location: "Campana", unit: TemperatureUnit.metric) controller.fetchCurrentWeatherData(input: input) { (result: Either<NetworkControllerError, WeatherData>) in switch result { case .left(let error): XCTAssertNotNil(error) exp.fulfill() case .right: XCTFail("It should fail") } } waitForExpectations(timeout: 10, handler: nil) }
And obviously, you can also test for data to be correctly parsed, and because you are injecting the data yourself, you can check for specific values as well.
func testOpenWeatherMap() { let exp = expectation(description: "Get weather data") let session = MockURLSession() self.prepareRetreiveWeatherSessionDataSucess(session) let controller = OpenWeatherMapNetworkController(session: session) let input = Input(location: "Campana", unit: TemperatureUnit.metric) controller.fetchCurrentWeatherData(input: input) { (result: Either<NetworkControllerError, WeatherData>) in switch result { case .left: XCTFail("no data returned by fetchWeatherData()") case .right(let data): let city = input.location let condition = data.condition let temperature = data.temperature XCTAssertEqual(city, "Campana") XCTAssertEqual(condition, "Clear") XCTAssertEqual(temperature, 42.8) exp.fulfill() } } waitForExpectations(timeout: 10, handler: nil) }
A complete example can be found in Gist
0 notes
Text
Open Data R Meetup: exploring the Distribution of Traffic Accidents in Belgrade, 2015 in R
The R code that accompanies this post is found on GitHub: you will find R, Rmd, and HTML files there that were used during the first Open Data R Meetup held in Belgrade, 31 January 2017, organized by Data Science Serbia in Startit Center, Savska 5, Belgrade Serbia. The Open Data initiative in Serbia is still young, our Open Data Portal is still under development, and guess what - we from Data Science Serbia will join the Working Group for Open Data of the Directorate for eGovernment to help open, standardize, structure, publish, and analyse the many forthcoming open data sets from our country - in R, of course :)
The data set under exploration here encompasses data on traffic accidents in Belgrade for 2015 (December 2015 data are missing). The notebook focuses on an exploratory analysis of this test open data set that was provided at the Open Data Portal of the Republic of Serbia (the portal is currently under development). The data set was kindly provided to the Open Data Portal by the Republic of Serbia Ministry of Interior. Many more open data sets will be indexed and uploaded in the forthcoming weeks and months.
The Distribution of Traffic Accidents 2015, Belgrade. Part of the city core is shown on the map produced by ggmap, ggplot2 w. geom_density2d() and stat_density2d().
Besides focusing on the exploration and visualization of this test data set, we have demonstrated the basic usage of {weatherData} to fetch historical weather data to R, {wbstats} to access the rich World Data Bank time series, and {ISOcodes} packages in R.
Some exploratory modeling (Negative Binomial Regression with glm.nb() and Ordinal Logistic Regression with clm()from {ordinal}) is exercised merely to assess the prima facie effects of the most influential factors.
Predicted vs. Observed number of traffic accidents frequency per day, Belgrade 2015. Negative binomial regression for overdispersed frequency data with glm.nb().
Hopefully, this is just a begining of our exploratory analyses of open data in R; in the following months, Data Science Serbia will work hard to enable cross-country open data comparisons by elaborating on the forthcoming Serbian open data sets, and promote R as the lingua franca of the discipline.
0 notes
Text
Forecast Weather data from IBM Bluemix Weather...
Forecast Weather data from IBM Bluemix Weather APIs #IoTRecipes #WIoTP #WatsonIoT #WeatherData
Forecast Weather data from IBM Bluemix Weather...
IBM Technical Rock Star Program
0 notes