#ai impact on software development
Explore tagged Tumblr posts
Text
0 notes
Text
DataRobot Launches New Federal AI Application Suite to Unlock Efficiency and Impact
Purpose-built agents and custom applications accelerate secure, cost-efficient AI for government agencies Press Release – May 8, 2025 — BOSTON — DataRobot, the provider of AI that makes business sense, today introduced its federal AI application suite, a comprehensive set of agents and custom applications purpose-built for government agencies to deliver mission-critical AI in high-security…
1 note
·
View note
Text
How does AI effect education negatively?
While Artificial Intelligence (AI) is changing classrooms rapidly, it also brings concerns we shouldn’t ignore. As we embrace smarter tools, we must ask students are learning more, or are we just automating the process? AI in Education offers many benefits, but if we ed blindly, it could affect creativity, interaction, and even job security. Let’s explore its lesser-known drawbacks in a simple yet insightful way.
AI Weakens Creativity and Thinking Skills
Many AI tools deliver instant results, but this convenience might discourage students from thinking deeply. If learners always turn to AI for help, they may stop developing problem-solving abilities. In real life, critical thinking is necessary, but relying too much on machines can delay that growth. We must ensure students stay curious, asking questions instead of blindly accepting answers.
Emotional Learning and Social Skills Take a Hit
AI systems cannot understand how a student feels during a tough day. Unlike real teachers, machines cannot give a comforting smile or kind words when someone is down. Face-to-face interactions are essential in helping students build confidence and empathy. If children don’t learn emotional intelligence now, they might struggle in future workplaces and relationships.

Overdependence Can Create Passive Learners
When students use AI constantly, they may become too comfortable with shortcuts. This habit can lead to laziness, low motivation, and a lack of independence in learning. If AI stopped working one day, would students know how to continue? Probably not. That’s why we should treat AI as a support tool, not as the main teacher.
Teachers Feel Pressure and Burnout
According to recent studies, nearly 1 in 3 teachers feel anxious about AI replacing them (Gallup, 2024). Learning to use new AI systems also adds extra tasks, making their workload heavier. When teachers feel unsupported, job satisfaction decreases and burnout increases. Educators must be guided, trained, and appreciated because no machine can replace their human touch.
Unfair Access Increases the Learning Gap
In many areas, students still lack good internet, devices, or modern infrastructure. This digital divide makes it harder for everyone to benefit equally from AI in Education. If schools continue to rely heavily on AI, students from underdeveloped regions will fall further behind. To fix this, we must ensure equal resources and digital literacy training for all learners.
Privacy, Bias, & Algorithm Confusion
AI systems collect tons of student data, from test scores to behavioral habits. But who handles this data? And what if it’s leaked or misused? These questions worry many. Additionally, most AI decisions happen inside a “black box,” even though teachers don’t always know how results are calculated. If the AI contains bias or errors, students may be judged unfairly without a chance to explain themselves.
Testing Takes Priority Over Real Learning
AI is often programmed to track numbers like grades, quiz scores, and attendance. This may push schools to focus more on test preparation and less on creativity or communication. A classroom should encourage ideas and discussions, not just scanning answers. If learning becomes too mechanical, students might stop enjoying the process altogether.
Final Words:
To sum it all up, AI in Education must be introduced with care. We need to train teachers, protect student data, and use AI as a helpful partner, not a replacement. Technology should assist real learning, not weaken it. If used wisely, AI can support both teachers and learners without replacing the human connection. After all, no machine can truly replace a teacher’s voice, patience, or encouragement.
0 notes
Text
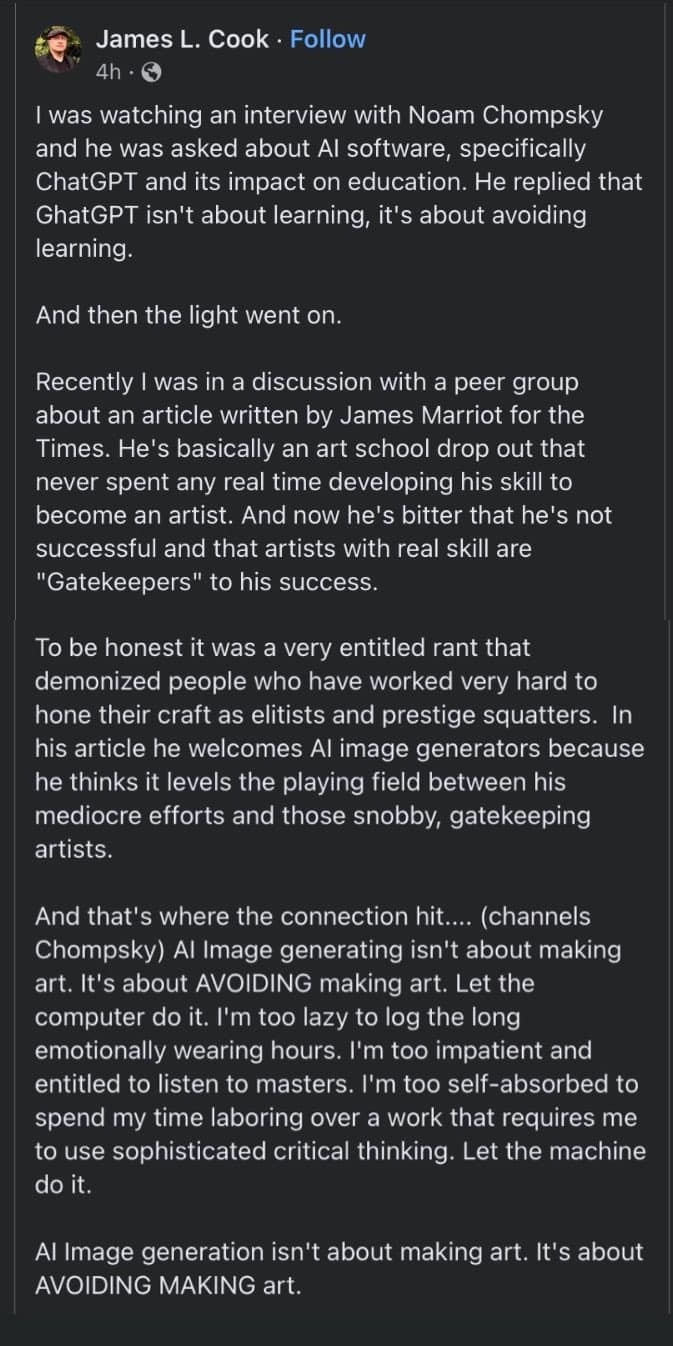
Image description from the notes by @summery-captain:
"A post by James L. Cook, reading: "I was watching an interview with Noam Chompsky and he was asked about AI software, specifically Chat GPT and its impact on education. He replied that Chat GPT isn't about learning, it's about avoiding learning.
And then the light went on.
Recently I was in discussion with a peer group about an article written by James Marriot for the Times. He's basically an art school drop out that never spent any real time developing his skill to become an artist. And now he's bitter that he's not successful and that artists with real skill are 'gatekeepers' to his success.
To be honest it was a very entitled rant that demonized people who have worked very hard to hone their craft as elitists and prestige squatters. In his article he welcomes AI generators because he thinks it levels the playing field between his mediocre efforts and those snobby, gatekeeping artists.
And that's where the connection hit... (channels Chompsky) AI image generating isn't about making art. It is about AVOIDING making art. Let the computer do it. I'm too lazy to log the long emotionally wearing hours. I'm too impatient to listen to masters. I'm too self-absorbed to spend my time laboring over a work that requires me to use sophisticated critical thinking. Let the machine do it.
AI imagine generation isn't about making art. It's about AVOIDING MAKING art." End of imagine description
52K notes
·
View notes
Text
The Electric Revolution of Henry Ford and the Future of AI in Software Development
New Post has been published on https://thedigitalinsider.com/the-electric-revolution-of-henry-ford-and-the-future-of-ai-in-software-development/
The Electric Revolution of Henry Ford and the Future of AI in Software Development
I’ve been reflecting on how software development is set to evolve with the introduction of AI and AI tools. Change is nothing new in the world of software development. For example, in our parents’ time, programmers used punch cards to write code. However, the impact of AI and AI-driven development will be much more significant. These advancements will fundamentally alter the way we write, structure, and organize code.
There’s a compelling analogy to consider: Henry Ford’s Highland Park Plant. This plant truly revolutionized industrial manufacturing—not in the superficial way that influencers might claim when they say they are “revolutionizing the mushroom tea supplement market.” Ford returned to first principles, examining manufacturing and the tools available at the time to redesign everything from the ground up. He built a new factory centered around electricity. It’s remarkable because industrial electricity existed for nearly forty years before it was effectively utilized to enhance productivity.
Before the invention of electricity, manufacturing plants were structured around a central boiler, with heavy machinery powered by steam. The equipment that required the most power was situated nearest to the boiler, while those that needed less energy were placed farther away. The entire design of the plant focused on the power source rather than efficient production.
However, when Henry Ford began working on the Model T, he collaborated with Thomas Edison to rethink this layout. Edison convinced Ford that electrical power plants could provide a consistent and high level of power to every piece of equipment, regardless of its distance from the generator. This breakthrough allowed Ford to implement his manufacturing principles and design the first assembly line.
It took 40 years—think about that—40 years from the proliferation of industrial electricity for it to change how the world operated in any meaningful way. There were no productivity gains from electricity for over 40 years. It’s insane.
How does this relate to AI and software development, you may ask? Understanding the importance of humans in both software and AI is crucial. Humans are the driving force; we serve as the central power source behind every structure and design pattern in software development. Human maintainability is essential to the principles often referred to as “clean code.” We have created patterns and written numerous articles focusing on software development with people in mind. In fact, we’ve designed entire programming languages to be user-friendly. Code must be readable, maintainable, and manageable by humans since they will need to modify it. Just as a steam factory is organized around a single power source, we structure our systems with the understanding that when that power source changes, the entire system may need to be reorganized.
As AI becomes increasingly integrated into software development, it is emerging as a powerful new tool. AI has the ability to read, write, and modify code in ways that are beyond human
capability. However, certain patterns—such as naming conventions and the principle of single responsibility—can complicate the process for AI, making it difficult to effectively analyze and reason about code.
As AI plays a more central role in development, there will be a growing demand for faster code generation. This could mean that instead of using JavaScript or TypeScript and then minifying the code, we could instruct an AI to make behavioral changes, allowing it to update already minified code directly. Additionally, code duplication might become a beneficial feature that enhances software efficiency, as AI would be able to instantly modify all instances of the duplicated logic.
This shift in thinking will take time. People will need to adapt, and for now, AI’s role in software development primarily provides incremental improvements. However, companies and individuals who embrace AI and begin to rethink fundamental software development principles, including Conway’s Law, will revolutionize the way we build software and, consequently, how the world operates.
#ai#AI in software development#ai tools#Articles#change#code#code generation#Companies#Design#Design Pattern#development#driving#efficiency#electrical power#electricity#energy#equipment#focused.io#Ford#Fundamental#Future#future of AI#generator#how#human#humans#impact#industrial manufacturing#influencers#Invention
0 notes
Text
youtube
Discover how artificial intelligence is transforming the finance industry, reducing costs, and driving innovation in this deep dive video. We explore the real financial implications of integrating AI into fintech, providing insights into investment, returns, and strategic advantages. Whether you're a startup or an established financial entity, understanding these aspects can revolutionize your approach to digital finance.
#ibiixo#ibiixo technologies#artificial intelligence#ai#types of ai#software development#web app development#mobile app development#impact of ai#AI Integration#Integration of AI#AI in Finance#AI in Fintech#Fintech industry#Youtube
0 notes
Text
Exploring the Latest Trends in Software Development
Introduction The software is something like an industry whose development is ever-evolving with new technologies and changing market needs as the drivers. To this end, developers must keep abreast with current trends in their fields of operation to remain competitive and relevant. Read to continue .....
#analysis#science updates#tech news#technology#trends#adobe cloud#business tech#nvidia drive#science#tech trends#Software Solutions#Tags5G technology impact on software#Agile methodologies in software#AI in software development#AR and VR in development#blockchain technology in software#cloud-native development benefits#cybersecurity trends 2024#DevOps and CI/CD tools#emerging technologies in software development#future of software development#IoT and edge computing applications#latest software development trends#low-code development platforms#machine learning for developers#no-code development tools#popular programming languages#quantum computing in software#software development best practices#software development tools
0 notes
Text
AI impacts almost every industry in 2024. AI has an impact on every stage of life. AI plays a crucial role in complex processes by simplifying and increasing coder productivity. AI-powered tools are very beneficial in my industry, especially in IT. To learn more about the Effect of AI on Software Development, explore our informative article.
0 notes
Text
AI and IoT can revolutionize business processes. For example, IoT devices can collect and communicate valuable information about a company's operations. And with the help of NLP, these devices can communicate with humans and other devices. It can even prevent a device from malfunctioning by automatically sending service notifications when needed. By combining AI and IoT, businesses can develop new products and improve existing ones. Rolls Royce plans to implement AI technologies into airplane engine maintenance amenities. These devices will be able to detect trends in data and extract operational insights that can improve operations.
AI and IoT are rated among the top ten emerging technology trends for the Environment industry since they aid in environmental sustainability. The deployment of IoT devices in fleet management can help detect gas leaks and other environmental problems in real-time. By monitoring ambient air pollution, the data can inform preventative policies and strategies. Intelligent environmental management is a key way to improve the environment. This is a growing field with many benefits. Its application is endless.
#AI and IoT revolution#impact of AI and IoT on environment#iot and sustainability#software development company#custom software development#.net development#ifourtechnolab#software outsourcing#software development#angular development#asp.net development
0 notes
Text
I first posted this in a thread over on BlueSky, but I decided to port (a slightly edited version of) it over here, too.
Entirely aside from the absurd and deeply incorrect idea [NaNoWriMo has posited] that machine-generated text and images are somehow "leveling the playing field" for marginalized groups, I think we need to interrogate the base assumption that acknowledging how people have different abilities is ableist/discriminatory. Everyone SHOULD have access to an equal playing field when it comes to housing, healthcare, the ability to exist in public spaces, participating in general public life, employment, etc.
That doesn't mean every person gets to achieve every dream no matter what.
I am 39 years old and I have scoliosis and genetically tight hamstrings, both of which deeply impact my mobility. I will never be a professional contortionist. If I found a robot made out of tentacles and made it do contortion and then demanded everyone call me a contortionist, I would be rightly laughed out of any contortion community. Also, to make it equivalent, the tentacle robot would be provided for "free" by a huge corporation based on stolen unpaid routines from actual contortionists, and using it would boil drinking water in the Southwest into nothingness every time I asked it to do anything, and the whole point would be to avoid paying actual contortionists.
If you cannot - fully CAN NOT - do something, even with accommodations, that does not make you worth less as a person, and it doesn't mean the accommodations shouldn't exist, but it does mean that maybe that thing is not for you.
But who CAN NOT do things are not who uses "AI." It's people who WILL NOT do things.
"AI art means disabled people can be artists who wouldn't be able to otherwise!" There are armless artists drawing with their feet. There are paralyzed artists drawing with their mouths, or with special tracking software that translates their eye movements into lines. There are deeply dyslexic authors writing via text-to-speech. There are deaf musicians. If you actually want to do a thing and care about doing the thing, you can almost always find a way to do the thing.
Telling a machine to do it for you isn't equalizing access for the marginalized. It's cheating. It's anti-labor. It makes it easier for corporations not to pay creative workers, AND THAT'S IS WHY THEY'RE PUSHING IT EVERYWHERE.
I can't wait for the bubble to burst on machine-generated everything, just like it did for NFTs. When it does some people are going to discover they didn't actually learn anything or develop any transferable skills or make anything they can be proud of.
I hope a few of those people pick up a pencil.
It's never too late to start creating. It's never too late to actually learn something. It's never too late to realize that the work is the point.
#AI#writing#just fucking do it#if you want to be a writer then write#literally no one can do it for you#especially not machine-generated text machines#the work is the point
1K notes
·
View notes
Note
Is AWAY using it's own program or is this just a voluntary list of guidelines for people using programs like DALL-E? How does AWAY address the environmental concerns of how the companies making those AI programs conduct themselves (energy consumption, exploiting impoverished areas for cheap electricity, destruction of the environment to rapidly build and get the components for data centers etc.)? Are members of AWAY encouraged to contact their gov representatives about IP theft by AI apps?
What is AWAY and how does it work?
AWAY does not "use its own program" in the software sense—rather, we're a diverse collective of ~1000 members that each have their own varying workflows and approaches to art. While some members do use AI as one tool among many, most of the people in the server are actually traditional artists who don't use AI at all, yet are still interested in ethical approaches to new technologies.
Our code of ethics is a set of voluntary guidelines that members agree to follow upon joining. These emphasize ethical AI approaches, (preferably open-source models that can run locally), respecting artists who oppose AI by not training styles on their art, and refusing to use AI to undercut other artists or work for corporations that similarly exploit creative labor.
Environmental Impact in Context
It's important to place environmental concerns about AI in the context of our broader extractive, industrialized society, where there are virtually no "clean" solutions:
The water usage figures for AI data centers (200-740 million liters annually) represent roughly 0.00013% of total U.S. water usage. This is a small fraction compared to industrial agriculture or manufacturing—for example, golf course irrigation alone in the U.S. consumes approximately 2.08 billion gallons of water per day, or about 7.87 trillion liters annually. This makes AI's water usage about 0.01% of just golf course irrigation.
Looking into individual usage, the average American consumes about 26.8 kg of beef annually, which takes around 1,608 megajoules (MJ) of energy to produce. Making 10 ChatGPT queries daily for an entire year (3,650 queries) consumes just 38.1 MJ—about 42 times less energy than eating beef. In fact, a single quarter-pound beef patty takes 651 times more energy to produce than a single AI query.
Overall, power usage specific to AI represents just 4% of total data center power consumption, which itself is a small fraction of global energy usage. Current annual energy usage for data centers is roughly 9-15 TWh globally—comparable to producing a relatively small number of vehicles.
The consumer environmentalism narrative around technology often ignores how imperial exploitation pushes environmental costs onto the Global South. The rare earth minerals needed for computing hardware, the cheap labor for manufacturing, and the toxic waste from electronics disposal disproportionately burden developing nations, while the benefits flow largely to wealthy countries.
While this pattern isn't unique to AI, it is fundamental to our global economic structure. The focus on individual consumer choices (like whether or not one should use AI, for art or otherwise,) distracts from the much larger systemic issues of imperialism, extractive capitalism, and global inequality that drive environmental degradation at a massive scale.
They are not going to stop building the data centers, and they weren't going to even if AI never got invented.
Creative Tools and Environmental Impact
In actuality, all creative practices have some sort of environmental impact in an industrialized society:
Digital art software (such as Photoshop, Blender, etc) generally uses 60-300 watts per hour depending on your computer's specifications. This is typically more energy than dozens, if not hundreds, of AI image generations (maybe even thousands if you are using a particularly low-quality one).
Traditional art supplies rely on similar if not worse scales of resource extraction, chemical processing, and global supply chains, all of which come with their own environmental impact.
Paint production requires roughly thirteen gallons of water to manufacture one gallon of paint.
Many oil paints contain toxic heavy metals and solvents, which have the potential to contaminate ground water.
Synthetic brushes are made from petroleum-based plastics that take centuries to decompose.
That being said, the point of this section isn't to deflect criticism of AI by criticizing other art forms. Rather, it's important to recognize that we live in a society where virtually all artistic avenues have environmental costs. Focusing exclusively on the newest technologies while ignoring the environmental costs of pre-existing tools and practices doesn't help to solve any of the issues with our current or future waste.
The largest environmental problems come not from individual creative choices, but rather from industrial-scale systems, such as:
Industrial manufacturing (responsible for roughly 22% of global emissions)
Industrial agriculture (responsible for roughly 24% of global emissions)
Transportation and logistics networks (responsible for roughly 14% of global emissions)
Making changes on an individual scale, while meaningful on a personal level, can't address systemic issues without broader policy changes and overall restructuring of global economic systems.
Intellectual Property Considerations
AWAY doesn't encourage members to contact government representatives about "IP theft" for multiple reasons:
We acknowledge that copyright law overwhelmingly serves corporate interests rather than individual creators
Creating new "learning rights" or "style rights" would further empower large corporations while harming individual artists and fan creators
Many AWAY members live outside the United States, many of which having been directly damaged by the US, and thus understand that intellectual property regimes are often tools of imperial control that benefit wealthy nations
Instead, we emphasize respect for artists who are protective of their work and style. Our guidelines explicitly prohibit imitating the style of artists who have voiced their distaste for AI, working on an opt-in model that encourages traditional artists to give and subsequently revoke permissions if they see fit. This approach is about respect, not legal enforcement. We are not a pro-copyright group.
In Conclusion
AWAY aims to cultivate thoughtful, ethical engagement with new technologies, while also holding respect for creative communities outside of itself. As a collective, we recognize that real environmental solutions require addressing concepts such as imperial exploitation, extractive capitalism, and corporate power—not just focusing on individual consumer choices, which do little to change the current state of the world we live in.
When discussing environmental impacts, it's important to keep perspective on a relative scale, and to avoid ignoring major issues in favor of smaller ones. We promote balanced discussions based in concrete fact, with the belief that they can lead to meaningful solutions, rather than misplaced outrage that ultimately serves to maintain the status quo.
If this resonates with you, please feel free to join our discord. :)
Works Cited:
USGS Water Use Data: https://www.usgs.gov/mission-areas/water-resources/science/water-use-united-states
Golf Course Superintendents Association of America water usage report: https://www.gcsaa.org/resources/research/golf-course-environmental-profile
Equinix data center water sustainability report: https://www.equinix.com/resources/infopapers/corporate-sustainability-report
Environmental Working Group's Meat Eater's Guide (beef energy calculations): https://www.ewg.org/meateatersguide/
Hugging Face AI energy consumption study: https://huggingface.co/blog/carbon-footprint
International Energy Agency report on data centers: https://www.iea.org/reports/data-centres-and-data-transmission-networks
Goldman Sachs "Generational Growth" report on AI power demand: https://www.goldmansachs.com/intelligence/pages/gs-research/generational-growth-ai-data-centers-and-the-coming-us-power-surge/report.pdf
Artists Network's guide to eco-friendly art practices: https://www.artistsnetwork.com/art-business/how-to-be-an-eco-friendly-artist/
The Earth Chronicles' analysis of art materials: https://earthchronicles.org/artists-ironically-paint-nature-with-harmful-materials/
Natural Earth Paint's environmental impact report: https://naturalearthpaint.com/pages/environmental-impact
Our World in Data's global emissions by sector: https://ourworldindata.org/emissions-by-sector
"The High Cost of High Tech" report on electronics manufacturing: https://goodelectronics.org/the-high-cost-of-high-tech/
"Unearthing the Dirty Secrets of the Clean Energy Transition" (on rare earth mineral mining): https://www.theguardian.com/environment/2023/apr/18/clean-energy-dirty-mining-indigenous-communities-climate-crisis
Electronic Frontier Foundation's position paper on AI and copyright: https://www.eff.org/wp/ai-and-copyright
Creative Commons research on enabling better sharing: https://creativecommons.org/2023/04/24/ai-and-creativity/
210 notes
·
View notes
Note
Some things that make me hopeful: That HIV/AIDS could be on the path to eradication via medical advancements, and that a lot of cancers are becoming "chronic treatable illness" rather than terminal illnesses. That vaccine development is as good as it is, and could be better. In short I find it inspiring that as harmful as our current healthcare system can be at some points, despite that, we're successfully fighting stuff that was often in history seen as inevitable or fate. Medical advances are slow but steady.
That for as bad as AI is in many ways, there are very promising developments with small, specialized machine learning models to serve as "second eyes" to assist humans in hyper-detailed tasks with bad outcomes if the human misses - e.g. detecting cancer in pathology samples, spotting tornado development in severe storms on radar. Accessibility is also getting a boost - text readers for Blind/visually impaired people and sound alerts/notifications for Deaf/hearing impaired people are improving, as is translation software for people who are not good natural language learners. That between solar, wind, nuclear, and even possibly the development of batteries that are less environmentally impacting/dangerous than Li-Ion, we may well start turning the tide on fossil fuels' damage to the planet. Walkable cities aren't a joke but an actual field of development and study, and there's actual work on public transit and moving away from dependence on cars in many places. On a social level, that, while, yes, it seems like the US and some other countries are going backwards for the time being - that it's very unlikely those advances will be forever lost worldwide. More people than ever are for LGBTQIA+ freedoms and rights, more people than ever are against at least the most overt and harmful displays of bigotry and racism and sexism even if they aren't perfect, etc. And this might be a bit controversial, but the decline of compulsory religious practice in much of the world. That you can choose your faith and how you display it, or choose to have no faith or anywhere in between would have seemed entirely impossible to people even 100 years ago in a lot of places and now, that's a given human right pretty much everywhere but the most restrictive theocracies and hyper-traditionalist families.
Hopeful things!! Thanks for sharing!!
206 notes
·
View notes
Text
On Saturday, an Associated Press investigation revealed that OpenAI's Whisper transcription tool creates fabricated text in medical and business settings despite warnings against such use. The AP interviewed more than 12 software engineers, developers, and researchers who found the model regularly invents text that speakers never said, a phenomenon often called a “confabulation” or “hallucination” in the AI field.
Upon its release in 2022, OpenAI claimed that Whisper approached “human level robustness” in audio transcription accuracy. However, a University of Michigan researcher told the AP that Whisper created false text in 80 percent of public meeting transcripts examined. Another developer, unnamed in the AP report, claimed to have found invented content in almost all of his 26,000 test transcriptions.
The fabrications pose particular risks in health care settings. Despite OpenAI’s warnings against using Whisper for “high-risk domains,” over 30,000 medical workers now use Whisper-based tools to transcribe patient visits, according to the AP report. The Mankato Clinic in Minnesota and Children’s Hospital Los Angeles are among 40 health systems using a Whisper-powered AI copilot service from medical tech company Nabla that is fine-tuned on medical terminology.
Nabla acknowledges that Whisper can confabulate, but it also reportedly erases original audio recordings “for data safety reasons.” This could cause additional issues, since doctors cannot verify accuracy against the source material. And deaf patients may be highly impacted by mistaken transcripts since they would have no way to know if medical transcript audio is accurate or not.
The potential problems with Whisper extend beyond health care. Researchers from Cornell University and the University of Virginia studied thousands of audio samples and found Whisper adding nonexistent violent content and racial commentary to neutral speech. They found that 1 percent of samples included “entire hallucinated phrases or sentences which did not exist in any form in the underlying audio” and that 38 percent of those included “explicit harms such as perpetuating violence, making up inaccurate associations, or implying false authority.”
In one case from the study cited by AP, when a speaker described “two other girls and one lady,” Whisper added fictional text specifying that they “were Black.” In another, the audio said, “He, the boy, was going to, I’m not sure exactly, take the umbrella.” Whisper transcribed it to, “He took a big piece of a cross, a teeny, small piece … I’m sure he didn’t have a terror knife so he killed a number of people.”
An OpenAI spokesperson told the AP that the company appreciates the researchers’ findings and that it actively studies how to reduce fabrications and incorporates feedback in updates to the model.
Why Whisper Confabulates
The key to Whisper’s unsuitability in high-risk domains comes from its propensity to sometimes confabulate, or plausibly make up, inaccurate outputs. The AP report says, "Researchers aren’t certain why Whisper and similar tools hallucinate," but that isn't true. We know exactly why Transformer-based AI models like Whisper behave this way.
Whisper is based on technology that is designed to predict the next most likely token (chunk of data) that should appear after a sequence of tokens provided by a user. In the case of ChatGPT, the input tokens come in the form of a text prompt. In the case of Whisper, the input is tokenized audio data.
The transcription output from Whisper is a prediction of what is most likely, not what is most accurate. Accuracy in Transformer-based outputs is typically proportional to the presence of relevant accurate data in the training dataset, but it is never guaranteed. If there is ever a case where there isn't enough contextual information in its neural network for Whisper to make an accurate prediction about how to transcribe a particular segment of audio, the model will fall back on what it “knows” about the relationships between sounds and words it has learned from its training data.
According to OpenAI in 2022, Whisper learned those statistical relationships from “680,000 hours of multilingual and multitask supervised data collected from the web.” But we now know a little more about the source. Given Whisper's well-known tendency to produce certain outputs like "thank you for watching," "like and subscribe," or "drop a comment in the section below" when provided silent or garbled inputs, it's likely that OpenAI trained Whisper on thousands of hours of captioned audio scraped from YouTube videos. (The researchers needed audio paired with existing captions to train the model.)
There's also a phenomenon called “overfitting” in AI models where information (in this case, text found in audio transcriptions) encountered more frequently in the training data is more likely to be reproduced in an output. In cases where Whisper encounters poor-quality audio in medical notes, the AI model will produce what its neural network predicts is the most likely output, even if it is incorrect. And the most likely output for any given YouTube video, since so many people say it, is “thanks for watching.”
In other cases, Whisper seems to draw on the context of the conversation to fill in what should come next, which can lead to problems because its training data could include racist commentary or inaccurate medical information. For example, if many examples of training data featured speakers saying the phrase “crimes by Black criminals,” when Whisper encounters a “crimes by [garbled audio] criminals” audio sample, it will be more likely to fill in the transcription with “Black."
In the original Whisper model card, OpenAI researchers wrote about this very phenomenon: "Because the models are trained in a weakly supervised manner using large-scale noisy data, the predictions may include texts that are not actually spoken in the audio input (i.e. hallucination). We hypothesize that this happens because, given their general knowledge of language, the models combine trying to predict the next word in audio with trying to transcribe the audio itself."
So in that sense, Whisper "knows" something about the content of what is being said and keeps track of the context of the conversation, which can lead to issues like the one where Whisper identified two women as being Black even though that information was not contained in the original audio. Theoretically, this erroneous scenario could be reduced by using a second AI model trained to pick out areas of confusing audio where the Whisper model is likely to confabulate and flag the transcript in that location, so a human could manually check those instances for accuracy later.
Clearly, OpenAI's advice not to use Whisper in high-risk domains, such as critical medical records, was a good one. But health care companies are constantly driven by a need to decrease costs by using seemingly "good enough" AI tools—as we've seen with Epic Systems using GPT-4 for medical records and UnitedHealth using a flawed AI model for insurance decisions. It's entirely possible that people are already suffering negative outcomes due to AI mistakes, and fixing them will likely involve some sort of regulation and certification of AI tools used in the medical field.
87 notes
·
View notes
Text
One way to spot patterns is to show AI models millions of labelled examples. This method requires humans to painstakingly label all this data so they can be analysed by computers. Without them, the algorithms that underpin self-driving cars or facial recognition remain blind. They cannot learn patterns.
The algorithms built in this way now augment or stand in for human judgement in areas as varied as medicine, criminal justice, social welfare and mortgage and loan decisions. Generative AI, the latest iteration of AI software, can create words, code and images. This has transformed them into creative assistants, helping teachers, financial advisers, lawyers, artists and programmers to co-create original works.
To build AI, Silicon Valley’s most illustrious companies are fighting over the limited talent of computer scientists in their backyard, paying hundreds of thousands of dollars to a newly minted Ph.D. But to train and deploy them using real-world data, these same companies have turned to the likes of Sama, and their veritable armies of low-wage workers with basic digital literacy, but no stable employment.
Sama isn’t the only service of its kind globally. Start-ups such as Scale AI, Appen, Hive Micro, iMerit and Mighty AI (now owned by Uber), and more traditional IT companies such as Accenture and Wipro are all part of this growing industry estimated to be worth $17bn by 2030.
Because of the sheer volume of data that AI companies need to be labelled, most start-ups outsource their services to lower-income countries where hundreds of workers like Ian and Benja are paid to sift and interpret data that trains AI systems.
Displaced Syrian doctors train medical software that helps diagnose prostate cancer in Britain. Out-of-work college graduates in recession-hit Venezuela categorize fashion products for e-commerce sites. Impoverished women in Kolkata’s Metiabruz, a poor Muslim neighbourhood, have labelled voice clips for Amazon’s Echo speaker. Their work couches a badly kept secret about so-called artificial intelligence systems – that the technology does not ‘learn’ independently, and it needs humans, millions of them, to power it. Data workers are the invaluable human links in the global AI supply chain.
This workforce is largely fragmented, and made up of the most precarious workers in society: disadvantaged youth, women with dependents, minorities, migrants and refugees. The stated goal of AI companies and the outsourcers they work with is to include these communities in the digital revolution, giving them stable and ethical employment despite their precarity. Yet, as I came to discover, data workers are as precarious as factory workers, their labour is largely ghost work and they remain an undervalued bedrock of the AI industry.
As this community emerges from the shadows, journalists and academics are beginning to understand how these globally dispersed workers impact our daily lives: the wildly popular content generated by AI chatbots like ChatGPT, the content we scroll through on TikTok, Instagram and YouTube, the items we browse when shopping online, the vehicles we drive, even the food we eat, it’s all sorted, labelled and categorized with the help of data workers.
Milagros Miceli, an Argentinian researcher based in Berlin, studies the ethnography of data work in the developing world. When she started out, she couldn’t find anything about the lived experience of AI labourers, nothing about who these people actually were and what their work was like. ‘As a sociologist, I felt it was a big gap,’ she says. ‘There are few who are putting a face to those people: who are they and how do they do their jobs, what do their work practices involve? And what are the labour conditions that they are subject to?’
Miceli was right – it was hard to find a company that would allow me access to its data labourers with minimal interference. Secrecy is often written into their contracts in the form of non-disclosure agreements that forbid direct contact with clients and public disclosure of clients’ names. This is usually imposed by clients rather than the outsourcing companies. For instance, Facebook-owner Meta, who is a client of Sama, asks workers to sign a non-disclosure agreement. Often, workers may not even know who their client is, what type of algorithmic system they are working on, or what their counterparts in other parts of the world are paid for the same job.
The arrangements of a company like Sama – low wages, secrecy, extraction of labour from vulnerable communities – is veered towards inequality. After all, this is ultimately affordable labour. Providing employment to minorities and slum youth may be empowering and uplifting to a point, but these workers are also comparatively inexpensive, with almost no relative bargaining power, leverage or resources to rebel.
Even the objective of data-labelling work felt extractive: it trains AI systems, which will eventually replace the very humans doing the training. But of the dozens of workers I spoke to over the course of two years, not one was aware of the implications of training their replacements, that they were being paid to hasten their own obsolescence.
— Madhumita Murgia, Code Dependent: Living in the Shadow of AI
70 notes
·
View notes
Text
ABOUT.
My name is Slim Ray.
I’m a former programmer turned horror writer and filmmaker. After nearly a decade in software development, I made the leap to follow my passion for storytelling.
My creative journey has led me to write and direct three short films. These projects taught me the intricacies of storytelling and strengthened my love for crafting narratives that leave an impact.
I’m captivated by the intersection of words and images. This fascination drives my visual experiments—a blend of AI, photography, and storytelling—documented on my blog, Project H.
I'm a bit of a social outsider and awkward IRL, so I've created a character for this blog. This character isn't me exactly, but more of a persona for this space.
I’m constantly learning and refining my craft. This blog serves as my research hub, a place to explore and develop ideas, and the testing ground for a storytelling framework I’m building—a passion project close to my heart.
Teaching is another love of mine. I’m working on online courses and guides to share what I’ve learned about storytelling—stay tuned for updates!
I use AI as a tool for research and editing. If that’s not your thing, no hard feelings, but I ask for respect in how I approach my process.
LINKS.
[Patreon]
31 notes
·
View notes