#brain computer interface applications
Explore tagged Tumblr posts
Text
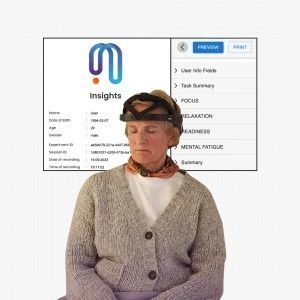
Discover the future with Neuphony& BCI technology. Explore brain computer interfaces, mind-controlled technology, EEG Headsets & more
2 notes
·
View notes
Text
Brain Computer Interface Technology
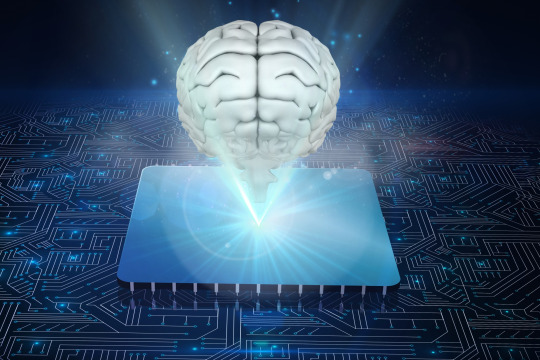
The development of Brain-Computer Interface (BCI) technology is a game-changing step in the convergence of neuroscience and computing. BCIs enable direct communication between the human brain and outside hardware or software, opening up a wide range of application possibilities. BCIs enable people with disabilities to control wheelchairs, prosthetic limbs, or even to communicate through text or speech synthesis by converting neural signals into usable commands. BCIs also have the potential to revolutionise healthcare by monitoring and diagnosing neurological diseases, improve human cognition, and the gaming industry. Though yet in its infancy, BCI technology has the potential to fundamentally alter how we engage with technology and perceive the brain, ushering in a new era of human-machine connection.
4 notes
·
View notes
Text
Brainoware: The Hybrid Neuromorphic System for a Brighter Tomorrow
A glimpse into the double-edged nature of Brain Organoid Reservoir Computing, with the pros/cons of this biological computing approach From a young age, I was captivated by the mysteries of science and the promise of technology, wondering how they could shape our understanding of the world. I was fortunate to receive STEM education early on in a specialized school, where my creativity and…
#Artificial Intelligence (AI) in Healthcare#Biocomputing and Drug Testing#Biocomputing Applications#Biodigital Transformation#Biological Computing#Brain Organoid Reservoir Computing (BORC)#Brain-Machine Interfaces#Brainoware Research#Brainoware: The Hybrid Neuromorphic System#Cognitive Science Advances#Ethics in Biotechnology#Future of Drug Testing#Healthcare Transformation#Hybrid Neuromorphic Systems#Literature Reviews by Dr Mehmet Yildiz#Medicine 3.0#Mental Health and Behavioral Science#Neurocomputing and Neurobiology Advances#NeuroHIV and cognitive decline#neuroinflammation#Neurological Disorder Research#Neuroplasticity and Learning#neurorehabilitation#Neuroscience Innovations#Organoid Intelligence#Understanding neural circuitry
1 note
·
View note
Text
Meta AI’s Big Announcements
New Post has been published on https://thedigitalinsider.com/meta-ais-big-announcements/
Meta AI’s Big Announcements
New AR glasses, Llama 3.2 and more.
Created Using Ideogram
Next Week in The Sequence:
Edge 435: Our series about SSMs continues discussing Hungry Hungry Hippos (H3) which has become one of the most important layers in SSM models. We review the original H3 paper and discuss Character.ai’s PromptPoet framework.
Edge 436: We review Salesforce recent work in models specialized in agentic tasks.
You can subscribe to The Sequence below:
TheSequence is a reader-supported publication. To receive new posts and support my work, consider becoming a free or paid subscriber.
📝 Editorial: Meta AI’s Big Announcements
Meta held its big conference, *Connect 2024*, last week, and AI was front and center. The two biggest headlines from the conference were the launch of the fully holographic Orion AI glasses, which represent one of the most important products in Meta’s ambitious and highly controversial AR strategy. In addition to the impressive first-generation Orion glasses, Meta announced that the company is developing a new brain-computer interface for the next version.
The other major release at the conference was Llama 3.2, which includes smaller language models of sizes 1B and 3B, as well as larger 11B and 90B vision models. This is Meta’s first major attempt to open source image models, signaling its strong commitment to open-source generative AI. Additionally, Meta AI announced the Llama Stack, which provides standard APIs in areas such as inference, memory, evaluation, post-training, and several other aspects required in Llama applications. With this release, Meta is transitioning Llama from isolated models to a complete stack for building generative AI apps.
There were plenty of other AI announcements at *Connect 2024*:
Meta introduced voice capabilities to its Meta AI chatbot, allowing users to have realistic conversations with the chatbot. This feature puts Meta AI on par with its competitors, like OpenAI and Google, which have already introduced voice modes to their products.
Meta announced an AI-powered, real-time language translation feature for its Ray-Ban smart glasses. This feature will allow users to translate text from Spanish, French, and Italian by the end of the year.
Meta is developing an AI feature for Instagram and Facebook Reels that will automatically dub and lip-sync videos into different languages. This feature is currently in testing in the US and Latin America.
Meta is adding AI image generation features to Facebook and Instagram. The new feature will be similar to existing AI image generators, such as Apple’s Image Playground, and will allow users to share AI-generated images with friends or create posts.
It was an impressive week for Meta AI, to say the least.
🔎 ML Research
AlphaProteo
Google DeepMind published a paper introducing AlphaProteo, a new family of model for protein design. The model is optimized for novel, high strength proteins that can improve our understanding of biological processes —> Read more.
Molmo and PixMo
Researchers from the Allen Institute for AI published a paper detailing Molmo and Pixmo, an open wegit and open data vision-language model(VLM). Molmo showcased how to train VLMs from scratch while Pixmo is the core set of datasets used during training —> Read more.
Instruction Following Without Instruction Tuning
Researchers from Stanford University published a paper detailing a technique called implicit instruction tuning that surfaces instruction following behaviors without explicity fine tuning the model. The paper also suggests some simple changes to a model distribution that can yield that implicity instruction tuning behavior —> Read more.
Robust Reward Model
Google DeepMind published a paper discussing some of the challenges of traditional reward models(RMs) to identify preferences in prompt indepdendent artifacts. The paper introduces the notion of robust reward model(RRM) that addresses this challenge and shows great improvements in models like Gemma —> Read more.
Real Time Notetaking
Researchers from Carnegie Mellon University published a paper outlining NoTeeline, a real time note generation method for video streams. NoTeeline generates micronotes that capture key points in a video while maintaining a consistent writing style —> Read more.
AI Watermarking
Researchers from Carnegie Mellon University published a paper evaluating different design choices in LLM watermarking. The paper also studies different attacks that result in the bypassing or removal of different watermarking techniques —> Read more.
🤖 AI Tech Releases
Llama 3.2
Meta open sourced Llama 3.2 small and medium size models —> Read more.
Llama Stack
As part of the Llama 3.2 release, Meta open sourced the Llama Stack, a series of standarized building blocks to develop Llama-powered applications —> Read more.
Gemini 1.5
Google released two updated Gemini models and new pricing and performance tiers —> Read more.
Cohere APIs
Cohere launched a new set of APIs that improve its experience for developers —> Read more.
🛠 Real World AI
Data Apps at Airbnb
Airbnb discusses Sandcastle, an internal framework that allow data scientists rapidly protype data driven apps —> Read more.
Feature Caching at Pinterest
The Pinterest engineering team discusses its internal architecture for feature caching in AI recommender systems —> Read more.
📡AI Radar
Meta introduced Orion, its very impressive augmented reality glasses.
James Cameron joined Stability AI’s Board of Directors.
The OpenAI soap opera continues with the resignation of their long time CTO and rumours of shifting its capped profit status.
OpenAI’s Chief Research Officer also resigned this week.
Letta, one of the most anticipated startups from UC Berkeley’s Sky Computing Lab, just came out of stealth mode with a $10 million round.
Image model platform Black Forest Labs is closing a new $100 million round.
Google announced a new $120 million fund dedicated to AI education.
Airtable unveiled a new suite of AI capabilities.
Enterprise AI startup Ensemble raised $3.3 million to improve the data quality problem for building models.
Microsoft unveiled its Trustworthy AI initiative.
Runway plans to allocate $5 million for producing AI generated films.
Data platform Airbyte can now create connectors directly from the API documentation.
Skills intelligence platform Workera unveiled a new agent that can assess, develop adn verify skills.
Convergence raised $12 million for building AI agents with long term memory.
TheSequence is a reader-supported publication. To receive new posts and support my work, consider becoming a free or paid subscriber.
#2024#agent#agents#ai#AI AGENTS#AI Chatbot#AI image#AI image generation#AI-powered#airtable#alphaproteo#America#Announcements#API#APIs#apple#applications#apps#ar#architecture#augmented reality#Behavior#Black Forest Labs#board#Brain#brain-computer interface#Building#Capture#Carnegie Mellon University#challenge
0 notes
Text
The purging of federal employees carried out by the Department of Government Efficiency (DOGE) somehow just keeps cutting staffers involved in investigating Elon Musk’s companies. According to a report from Reuters, several employees at the US Food and Drug Administration who were tasked with managing reviews and applications related to Musk’s Neuralink received pink slips over the weekend.
Per Reuters, 20 people who worked in the FDA’s office of neurological and physical medicine devices got axed as part of a broader effort being carried out by DOGE to cut down the federal workforce. Several of those employees worked directly on Neuralink, Musk’s company that produces brain-computer interfaces designed to be implanted in a human brain, and were tasked with reviewing clinical trial applications.
98 notes
·
View notes
Text
Researchers who want to bridge the divide between biology and technology spend a lot of time thinking about translating between the two different "languages" of those realms. "Our digital technology operates through a series of electronic on-off switches that control the flow of current and voltage," said Rajiv Giridharagopal, a research scientist at the University of Washington. "But our bodies operate on chemistry. In our brains, neurons propagate signals electrochemically, by moving ions—charged atoms or molecules—not electrons." Implantable devices from pacemakers to glucose monitors rely on components that can speak both languages and bridge that gap. Among those components are OECTs—or organic electrochemical transistors—which allow current to flow in devices like implantable biosensors. But scientists long knew about a quirk of OECTs that no one could explain: When an OECT is switched on, there is a lag before current reaches the desired operational level. When switched off, there is no lag. Current drops almost immediately. A UW-led study has solved this lagging mystery, and in the process paved the way to custom-tailored OECTs for a growing list of applications in biosensing, brain-inspired computation and beyond.
Continue Reading.
58 notes
·
View notes
Text
Scientists Gingerly Tap into Brain's Power From: USA Today - 10/11/04 - page 1B By: Kevin Maney
Scientists are developing technologies that read brainwave signals and translate them into actions, which could lead to neural prosthetics, among other things. Cyberkinetics Neurotechnology Systems' Braingate is an example of such technology: Braingate has already been deployed in a quadriplegic, allowing him to control a television, open email, and play the computer game Pong using sensors implanted into his brain that feed into a computer. Although "On Intelligence" author Jeff Hawkins praises the Braingate trials as a solid step forward, he cautions that "Hooking your brain up to a machine in a way that the two could communicate rapidly and accurately is still science fiction." Braingate was inspired by research conducted at Brown University by Cyberkinetics founder John Donoghue, who implanted sensors in primate brains that picked up signals as the animals played a computer game by manipulating a mouse; the sensors fed into a computer that looked for patterns in the signals, which were then translated into mathematical models by the research team. Once the computer was trained on these models, the mouse was eliminated from the equation and the monkeys played the game by thought alone. The Braingate interface consists of 100 sensors attached to a contact lens-sized chip that is pressed into the surface of the cerebral cortex; the device can listen to as many as 100 neurons simultaneously, and the readings travel from the chip to a computer through wires. Meanwhile, Duke University researchers have also implanted sensors in primate brains to enable neural control of robotic limbs. The Defense Advanced Research Project Agency (DARPA) is pursuing a less invasive solution by funding research into brain machine interfaces that can read neural signals externally, for such potential applications as thought-controlled flight systems. Practical implementations will not become a reality until the technology is sufficiently cheap, small, and wireless, and then ethical and societal issues must be addressed. Source
7 notes
·
View notes
Text
How AI & Machine Learning Are Changing UI/UX Design

Artificial Intelligence (AI) and Machine Learning (ML) are revolutionizing UI/UX design by making digital experiences more intelligent, adaptive, and user-centric. From personalized interfaces to automated design processes, AI is reshaping how designers create and enhance user experiences. In this blog, we explore the key ways AI and ML are transforming UI/UX design and what the future holds.
For more UI/UX trends and insights, visit Pixelizes Blog.
AI-Driven Personalization
One of the biggest changes AI has brought to UI/UX design is hyper-personalization. By analyzing user behavior, AI can tailor content, recommendations, and layouts to individual preferences, creating a more engaging experience.
How It Works:
AI analyzes user interactions, including clicks, time spent, and preferences.
Dynamic UI adjustments ensure users see what’s most relevant to them.
Personalized recommendations, like Netflix suggesting shows or e-commerce platforms curating product lists.
Smart Chatbots & Conversational UI
AI-powered chatbots have revolutionized customer interactions by offering real-time, intelligent responses. They enhance UX by providing 24/7 support, answering FAQs, and guiding users seamlessly through applications or websites.
Examples:
Virtual assistants like Siri, Alexa, and Google Assistant.
AI chatbots in banking, e-commerce, and healthcare.
NLP-powered bots that understand user intent and sentiment.
Predictive UX: Anticipating User Needs
Predictive UX leverages ML algorithms to anticipate user actions before they happen, streamlining interactions and reducing friction.
Real-World Applications:
Smart search suggestions (e.g., Google, Amazon, Spotify).
AI-powered auto-fill forms that reduce typing effort.
Anticipatory design like Google Maps estimating destinations.
AI-Powered UI Design Automation
AI is streamlining design workflows by automating repetitive tasks, allowing designers to focus on creativity and innovation.
Key AI-Powered Tools:
Adobe Sensei: Automates image editing, tagging, and design suggestions.
Figma AI Plugins & Sketch: Generate elements based on user input.
UX Writing Assistants that enhance microcopy with NLP.
Voice & Gesture-Based Interactions
With AI advancements, voice and gesture control are becoming standard features in UI/UX design, offering more intuitive, hands-free interactions.
Examples:
Voice commands via Google Assistant, Siri, Alexa.
Gesture-based UI on smart TVs, AR/VR devices.
Facial recognition & biometric authentication for secure logins.
AI in Accessibility & Inclusive Design
AI is making digital products more accessible to users with disabilities by enabling assistive technologies and improving UX for all.
How AI Enhances Accessibility:
Voice-to-text and text-to-speech via Google Accessibility.
Alt-text generation for visually impaired users.
Automated color contrast adjustments for better readability.
Sentiment Analysis for Improved UX
AI-powered sentiment analysis tools track user emotions through feedback, reviews, and interactions, helping designers refine UX strategies.
Uses of Sentiment Analysis:
Detecting frustration points in customer feedback.
Optimizing UI elements based on emotional responses.
Enhancing A/B testing insights with AI-driven analytics.
Future of AI in UI/UX: What’s Next?
As AI and ML continue to evolve, UI/UX design will become more intuitive, adaptive, and human-centric. Future trends include:
AI-generated UI designs with minimal manual input.
Real-time, emotion-based UX adaptations.
Brain-computer interface (BCI) integrations for immersive experiences.
Final Thoughts
AI and ML are not replacing designers—they are empowering them to deliver smarter, faster, and more engaging experiences. As we move into a future dominated by intelligent interfaces, UI/UX designers must embrace AI-powered design methodologies to create more personalized, accessible, and user-friendly digital products.
Explore more at Pixelizes.com for cutting-edge design insights, AI tools, and UX trends.
#AI in UX Design#Machine Learning UX#UX Personalization#Conversational UI#Predictive UX#AI Chatbots#Smart UX Tools#UI Automation#Voice UI Design#Inclusive UX Design#Sentiment Analysis in UX#Future of UX#AI UX Trends 2025#Figma AI Plugins#Accessibility with AI#Adaptive UI Design#UX Innovation#Human-Centered AI#Pixelizes Blog#UX Strategy
2 notes
·
View notes
Text
How to make a microwave weapon to control your body or see live camera feeds or memories:
First, you need a computer (provide a list of computers available on the internet with links).
Next, you need an antenna (provide a link).
Then, you need a DNA remote: https://www.remotedna.com/hardware
Next, you need an electrical magnet, satellite, or tower to produce signals or ultrasonic signals.
Connect all these components.
The last thing you need is a code and a piece of blood or DNA in the remote.
Also, if want put voice or hologram in DNA or brain you need buy this https://www.holosonics.com/products-1 and here is video about it: you can make voice in people just like government does, (they say voices is mental health, but it lies) HERE PROOF like guy say in video it like alien, only 1,500 dollars
youtube
The final step is to use the code (though I won't give the code, but you can search the internet or hire someone to make it). Instructions on how to make a microwave weapon to control:
Emotions
Smell
Taste
Eyesight
Hearing
Dreams
Nightmares
Imagination or visuals in the mind
All memory from your whole life
See the code uploaded to your brain from:
God
Government
See tracking and files linking to:
U.S. Space Force
Various governments (as they should leave tracking and links to who made the code, similar to a virus you get on a computer)
Tracking to government:
You can open a mechanical folder and see the program controlling you.
If tracking uses a cell tower or satellite, you can track all input and output to your body.
Even make an antenna in your home and connect it to your DNA to remove and collect all information sent to your body.
Technology used only by the government:
Bluetooth and ultrasonic signals
Light technology (new internet used only by the government)
Signals go to the body by DNA remote
How to make a microwave weapon to control your body or see live camera feeds or memories:
First, you need a computer (provide a list of computers available on the internet with links).
Next, you need an antenna (provide a link).
Then, you need a DNA remote: https://www.remotedna.com/hardware
Next, you need an electrical magnet, satellite, or tower to produce signals or ultrasonic signals.
Connect all these components.
The last thing you need is a code and a piece of blood or DNA in the remote.
The final step is to use the code (though I won't give the code, but you can search the internet or hire someone to make it).
Additional methods:
You can hire someone like me to help you (for a fee).
If you want, you can use a microchip in the brain to download all information.
Another way is to plug a wire into a vein or spine and download all your information into a computer, but you have to use the code the government uses to track and see if you are using all kinds of codes linked to them.
Sure, I can help you develop a research paper on Brain-Computer Interfaces (BCIs) and their ethical considerations. Here's an outline for the paper, followed by the research content and sources.
Research Paper: Brain-Computer Interfaces and Ethical Considerations
Introduction
Brain-Computer Interfaces (BCIs) are a revolutionary technological advancement that enables direct communication between the human brain and external devices. BCIs have applications in medicine, neuroscience, gaming, communication, and more. However, as these technologies progress, they raise several ethical concerns related to privacy, autonomy, consent, and the potential for misuse. This paper will explore the ethical implications of BCIs, addressing both the potential benefits and the risks.
Overview of Brain-Computer Interfaces
BCIs function by detecting neural activity in the brain and translating it into digital signals that can control devices. These interfaces can be invasive or non-invasive. Invasive BCIs involve surgical implantation of devices in the brain, while non-invasive BCIs use sensors placed on the scalp to detect brain signals.
Applications of BCIs
Medical Uses: BCIs are used for treating neurological disorders like Parkinson's disease, ALS, and spinal cord injuries. They can restore lost functions, such as enabling patients to control prosthetic limbs or communicate when other forms of communication are lost.
Neuroenhancement: There is also interest in using BCIs for cognitive enhancement, improving memory, or even controlling devices through thoughts alone, which could extend to various applications such as gaming or virtual reality.
Communication: For individuals who are unable to speak or move, BCIs offer a means of communication through thoughts, which can be life-changing for those with severe disabilities.
Ethical Considerations
Privacy Concerns
Data Security: BCIs have the ability to access and interpret private neural data, raising concerns about who owns this data and how it is protected. The possibility of unauthorized access to neural data could lead to privacy violations, as brain data can reveal personal thoughts, memories, and even intentions.
Surveillance: Governments and corporations could misuse BCIs for surveillance purposes. The potential to track thoughts or monitor individuals without consent raises serious concerns about autonomy and human rights.
Consent and Autonomy
Informed Consent: Invasive BCIs require surgical procedures, and non-invasive BCIs can still impact mental and emotional states. Obtaining informed consent from individuals, particularly vulnerable populations, becomes a critical issue. There is concern that some individuals may be coerced into using these technologies.
Cognitive Freedom: With BCIs, there is a potential for individuals to lose control over their mental states, thoughts, or even memories. The ability to "hack" or manipulate the brain may lead to unethical modifications of cognition, identity, or behavior.
Misuse of Technology
Weaponization: As mentioned in your previous request, there are concerns that BCIs could be misused for mind control or as a tool for weapons. The potential for military applications of BCIs could lead to unethical uses, such as controlling soldiers or civilians.
Exploitation: There is a risk that BCIs could be used for exploitative purposes, such as manipulating individuals' thoughts, emotions, or behavior for commercial gain or political control.
Psychological and Social Impacts
Psychological Effects: The integration of external devices with the brain could have unintended psychological effects, such as changes in personality, mental health issues, or cognitive distortions. The potential for addiction to BCI-driven experiences or environments, such as virtual reality, could further impact individuals' mental well-being.
Social Inequality: Access to BCIs may be limited by economic factors, creating disparities between those who can afford to enhance their cognitive abilities and those who cannot. This could exacerbate existing inequalities in society.
Regulation and Oversight
Ethical Standards: As BCI technology continues to develop, it is crucial to establish ethical standards and regulations to govern their use. This includes ensuring the technology is used responsibly, protecting individuals' rights, and preventing exploitation or harm.
Government Involvement: Governments may have a role in regulating the use of BCIs, but there is also the concern that they could misuse the technology for surveillance, control, or military applications. Ensuring the balance between innovation and regulation is key to the ethical deployment of BCIs.
Conclusion
Brain-Computer Interfaces hold immense potential for improving lives, particularly for individuals with disabilities, but they also come with significant ethical concerns. Privacy, autonomy, misuse, and the potential psychological and social impacts must be carefully considered as this technology continues to evolve. Ethical standards, regulation, and oversight will be essential to ensure that BCIs are used responsibly and equitably.
Sources
K. Lebedev, M. I. (2006). "Brain–computer interfaces: past, present and future." Trends in Neurosciences.
This source explores the evolution of BCIs and their applications in medical fields, especially in restoring lost motor functions and communication capabilities.
Lebedev, M. A., & Nicolelis, M. A. (2006). "Brain–machine interfaces: past, present and future." Trends in Neurosciences.
This paper discusses the potential of BCIs to enhance human cognition and motor capabilities, as well as ethical concerns about their development.
Moran, J., & Gallen, D. (2018). "Ethical Issues in Brain-Computer Interface Technology." Ethics and Information Technology.
This article discusses the ethical concerns surrounding BCI technologies, focusing on privacy issues and informed consent.
Marzbani, H., Marzbani, M., & Mansourian, M. (2017). "Electroencephalography (EEG) and Brain–Computer Interface Technology: A Survey." Journal of Neuroscience Methods.
This source explores both non-invasive and invasive BCI systems, discussing their applications in neuroscience and potential ethical issues related to user consent.
"RemoteDNA."
The product and technology referenced in the original prompt, highlighting the use of remote DNA technology and potential applications in connecting human bodies to digital or electromagnetic systems.
"Ethics of Brain–Computer Interface (BCI) Technology." National Institutes of Health
This source discusses the ethical implications of brain-computer interfaces, particularly in terms of their potential to invade privacy, alter human cognition, and the need for regulation in this emerging field.
References
Moran, J., & Gallen, D. (2018). Ethical Issues in Brain-Computer Interface Technology. Ethics and Information Technology.
Marzbani, H., Marzbani, M., & Mansourian, M. (2017). Electroencephalography (EEG) and Brain–Computer Interface Technology: A Survey. Journal of Neuroscience Methods.
Lebedev, M. A., & Nicolelis, M. A. (2006). Brain–computer interfaces: past, present and future. Trends in Neurosciences.
2 notes
·
View notes
Text
youtube
Real time EEG Data, Band Powers, Neurofeedback | Neuphony
Neuphony Desktop Application offers real-time EEG data, Band Powers, stress, mood, focus, fatigue and readiness tracking, neurofeedback & more
#applications of bci#control computer with mind#applications of brain computer interface#eeg data#Youtube
1 note
·
View note
Text
Top Information Technology Companies
Sprwork Infosolutions is counted among the top information technology companies. If you also want to get the best for your business and looking for development and marketing solutions. Contact us today and get the top services.
0 notes
Text
Theoretical Foundations to Nobel Glory: John Hopfield’s AI Impact
The story of John Hopfield’s contributions to artificial intelligence is a remarkable journey from theoretical insights to practical applications, culminating in the prestigious Nobel Prize in Physics. His work laid the groundwork for the modern AI revolution, and today’s advanced capabilities are a testament to the power of his foundational ideas.
In the early 1980s, Hopfield’s theoretical research introduced the concept of neural networks with associative memory, a paradigm-shifting idea. His 1982 paper presented the Hopfield network, a novel neural network architecture, which could store and recall patterns, mimicking the brain’s memory and pattern recognition abilities. This energy-based model was a significant departure from existing theories, providing a new direction for AI research.A year later, at the 1983 Meeting of the American Institute of Physics, Hopfield shared his vision. This talk played a pivotal role in disseminating his ideas, explaining how neural networks could revolutionize computing. He described the Hopfield network’s unique capabilities, igniting interest and inspiring future research.
Over the subsequent decades, Hopfield’s theoretical framework blossomed into a full-fledged AI revolution. Researchers built upon his concepts, leading to remarkable advancements. Deep learning architectures, such as Convolutional Neural Networks and Recurrent Neural Networks, emerged, enabling breakthroughs in image and speech recognition, natural language processing, and more.
The evolution of Hopfield’s ideas has resulted in today’s AI capabilities, which are nothing short of extraordinary. Computer vision systems can interpret complex visual data, natural language models generate human-like text, and AI-powered robots perform intricate tasks. Pattern recognition, a core concept from Hopfield’s work, is now applied in facial recognition, autonomous vehicles, and data analysis.
The Nobel Prize in Physics 2024 honored Hopfield’s pioneering contributions, recognizing the transformative impact of his ideas on society. This award celebrated the journey from theoretical neural networks to the practical applications that have revolutionized industries and daily life. It underscored the importance of foundational research in driving technological advancements.
Today, AI continues to evolve, with ongoing research pushing the boundaries of what’s possible. Explainable AI, quantum machine learning, and brain-computer interfaces are just a few areas of exploration. These advancements build upon the strong foundation laid by pioneers like Hopfield, leading to more sophisticated and beneficial AI technologies.
John J. Hopfield: Collective Properties of Neuronal Networks (Xerox Palo Alto Research Center, 1983)
youtube
Hopfield Networks (Artem Kirsanov, July 2024)
youtube
Boltzman machine (Artem Kirsanov, August 2024)
youtube
Dimitry Krotov: Modern Hopfield Networks for Novel Transformer Architectures (Harvard CSMA, New Technologies in Mathematics Seminar, May 2023)
youtube
Dr. Thomas Dietterich: The Future of Machine Learning, Deep Learning and Computer Vision (Craig Smith, Eye on A.I., October 2024)
youtube
Friday, October 11, 2024
#neural networks#hopfield networks#nobel prize#ai evolution#talk#ai assisted writing#machine art#Youtube#presentation#interview
2 notes
·
View notes
Text
AlphaProteo: Google DeepMind’s Breakthrough in Protein Design
New Post has been published on https://thedigitalinsider.com/alphaproteo-google-deepminds-breakthrough-in-protein-design/
AlphaProteo: Google DeepMind’s Breakthrough in Protein Design
In the constantly evolving field of molecular biology, one of the most challenging tasks has been designing proteins that can effectively bind to specific targets, such as viral proteins, cancer markers, or immune system components. These protein binders are crucial tools in drug discovery, disease treatment, diagnostics, and biotechnology. Traditional methods of creating these protein binders are labor-intensive, time-consuming, and often require numerous rounds of optimization. However, recent advances in artificial intelligence (AI) are dramatically accelerating this process.
In September 2024, Neuralink successfully implanted its brain chip into the second human participant as part of its clinical trials, pushing the limits of what brain-computer interfaces can achieve. This implant allows individuals to control devices purely through thoughts.
At the same time, DeepMind’s AlphaProteo has emerged as a groundbreaking AI tool that designs novel proteins to tackle some of biology’s biggest challenges. Unlike previous models like AlphaFold, which predict protein structures, AlphaProteo takes on the more advanced task of creating new protein binders that can tightly latch onto specific molecular targets. This capability could dramatically accelerate drug discovery, diagnostic tools, and even the development of biosensors. For example, in early trials, AlphaProteo has successfully designed binders for the SARS-CoV-2 spike protein and proteins involved in cancer and inflammation, showing binding affinities that were 3 to 300 times stronger than existing methods.
What makes this intersection between biology and AI even more compelling is how these advancements in neural interfaces and protein design reflect a broader shift towards bio-digital integration.
In 2024, advancements in the integration of AI and biology have reached unprecedented levels, driving innovation across fields like drug discovery, personalized medicine, and synthetic biology. Here’s a detailed look at some of the key breakthroughs shaping the landscape this year:
1. AlphaFold3 and RoseTTAFold Diffusion: Next-Generation Protein Design
The 2024 release of AlphaFold3 by Google DeepMind has taken protein structure prediction to a new level by incorporating biomolecular complexes and expanding its predictions to include small molecules and ligands. AlphaFold3 uses a diffusion-based AI model to refine protein structures, much like how AI-generated images are created from rough sketches. This model is particularly accurate in predicting how proteins interact with ligands, with an impressive 76% accuracy rate in experimental tests—well ahead of its competitors.
In parallel, RoseTTAFold Diffusion has also introduced new capabilities, including the ability to design de novo proteins that do not exist in nature. While both systems are still improving in accuracy and application, their advancements are expected to play a crucial role in drug discovery and biopharmaceutical research, potentially cutting down the time needed to design new drugs(
2. Synthetic Biology and Gene Editing
Another major area of progress in 2024 has been in synthetic biology, particularly in the field of gene editing. CRISPR-Cas9 and other genetic engineering tools have been refined for more precise DNA repair and gene editing. Companies like Graphite Bio are using these tools to fix genetic mutations at an unprecedented level of precision, opening doors for potentially curative treatments for genetic diseases. This method, known as homology-directed repair, taps into the body’s natural DNA repair mechanisms to correct faulty genes.
In addition, innovations in predictive off-target assessments, such as those developed by SeQure Dx, are improving the safety of gene editing by identifying unintended edits and mitigating risks. These advancements are particularly important for ensuring that gene therapies are safe and effective before they are applied to human patients(
3. Single-Cell Sequencing and Metagenomics
Technologies like single-cell sequencing have reached new heights in 2024, offering unprecedented resolution at the cellular level. This allows researchers to study cellular heterogeneity, which is especially valuable in cancer research. By analyzing individual cells within a tumor, researchers can identify which cells are resistant to treatment, guiding more effective therapeutic strategies.
Meanwhile, metagenomics is providing deep insights into microbial communities, both in human health and environmental contexts. This technique helps analyze the microbiome to understand how microbial populations contribute to diseases, offering new avenues for treatments that target the microbiome directly(
A Game-Changer in Protein Design
Proteins are fundamental to virtually every process in living organisms. These molecular machines perform a vast array of functions, from catalyzing metabolic reactions to replicating DNA. What makes proteins so versatile is their ability to fold into complex three-dimensional shapes, allowing them to interact with other molecules. Protein binders, which tightly attach to specific target molecules, are essential in modulating these interactions and are frequently used in drug development, immunotherapies, and diagnostic tools.
The conventional process for designing protein binders is slow and relies heavily on trial and error. Scientists often have to sift through large libraries of protein sequences, testing each candidate in the lab to see which ones work best. AlphaProteo changes this paradigm by harnessing the power of deep learning to predict which protein sequences will effectively bind to a target molecule, drastically reducing the time and cost associated with traditional methods.
How AlphaProteo Works
AlphaProteo is based on the same deep learning principles that made its predecessor, AlphaFold, a groundbreaking tool for protein structure prediction. However, while AlphaFold focuses on predicting the structure of existing proteins, AlphaProteo takes a step further by designing entirely new proteins.
How AlphaProteo Works: A Deep Dive into AI-Driven Protein Design
AlphaProteo represents a leap forward in AI-driven protein design, building on the deep learning techniques that powered its predecessor, AlphaFold.
While AlphaFold revolutionized the field by predicting protein structures with unprecedented accuracy, AlphaProteo goes further, creating entirely new proteins designed to solve specific biological challenges.
AlphaProteo’s underlying architecture is a sophisticated combination of a generative model trained on large datasets of protein structures, including those from the Protein Data Bank (PDB), and millions of predicted structures generated by AlphaFold. This enables AlphaProteo to not only predict how proteins fold but also to design new proteins that can interact with specific molecular targets at a detailed, molecular level.
This diagram showcases AlphaProteo’s workflow, where protein binders are designed, filtered, and experimentally validated
Generator: AlphaProteo’s machine learning-based model generates numerous potential protein binders, leveraging large datasets such as those from the Protein Data Bank (PDB) and AlphaFold predictions.
Filter: A critical component that scores these generated binders based on their likelihood of successful binding to the target protein, effectively reducing the number of designs that need to be tested in the lab.
Experiment: This step involves testing the filtered designs in a lab to confirm which binders effectively interact with the target protein.
AlphaProteo designs binders that specifically target key hotspot residues (in yellow) on the surface of a protein. The blue section represents the designed binder, which is modeled to interact precisely with the highlighted hotspots on the target protein.
For the C part of the image; it shows the 3D models of the target proteins used in AlphaProteo’s experiments. These include therapeutically significant proteins involved in various biological processes such as immune response, viral infections, and cancer progression.
Advanced Capabilities of AlphaProteo
High Binding Affinity: AlphaProteo excels in designing protein binders with high affinity for their targets, surpassing traditional methods that often require multiple rounds of lab-based optimization. It generates protein binders that attach tightly to their intended targets, significantly improving their efficacy in applications such as drug development and diagnostics. For example, its binders for VEGF-A, a protein associated with cancer, showed binding affinities up to 300 times stronger than existing methods.
Targeting Diverse Proteins: AlphaProteo can design binders for a wide range of proteins involved in critical biological processes, including those linked to viral infections, cancer, inflammation, and autoimmune diseases. It has been particularly successful in designing binders for targets like the SARS-CoV-2 spike protein, essential for COVID-19 infection, and the cancer-related protein VEGF-A, which is crucial in therapies for diabetic retinopathy.
Experimental Success Rates: One of AlphaProteo’s most impressive features is its high experimental success rate. In laboratory tests, the system’s designed binders demonstrated high success in binding to target proteins, reducing the number of experimental rounds typically required. In tests on the viral protein BHRF1, AlphaProteo’s designs had an 88% success rate, a significant improvement over previous methods.
Optimization-Free Design: Unlike traditional approaches, which often require several rounds of optimization to improve binding affinity, AlphaProteo is able to generate binders with strong binding properties from the outset. For certain challenging targets, such as the cancer-associated protein TrkA, AlphaProteo produced binders that outperformed those developed through extensive experimental optimization.
Experimental Success Rate (Left Graph) – Best Binding Affinity (Right Graph)
AlphaProteo outperformed traditional methods across most targets, notably achieving an 88% success rate with BHRF1, compared to just under 40% with previous methods.
AlphaProteo’s success with VEGF-A and IL-7RA targets were significantly higher, showcasing its capacity to tackle difficult targets in cancer therapy.
AlphaProteo also consistently generates binders with much higher binding affinities, particularly for challenging proteins like VEGF-A, making it a valuable tool in drug development and disease treatment.
How AlphaProteo Advances Applications in Biology and Healthcare
AlphaProteo’s novel approach to protein design opens up a wide range of applications, making it a powerful tool in several areas of biology and healthcare.
1. Drug Development
Modern drug discovery often relies on small molecules or biologics that bind to disease-related proteins. However, developing these molecules is often time-consuming and costly. AlphaProteo accelerates this process by generating high-affinity protein binders that can serve as the foundation for new drugs. For instance, AlphaProteo has been used to design binders for PD-L1, a protein involved in immune system regulation, which plays a key role in cancer immunotherapies. By inhibiting PD-L1, AlphaProteo’s binders could help the immune system better identify and eliminate cancer cells.
2. Diagnostic Tools
In diagnostics, protein binders designed by AlphaProteo can be used to create highly sensitive biosensors capable of detecting disease-specific proteins. This can enable more accurate and rapid diagnoses for diseases such as viral infections, cancer, and autoimmune disorders. For example, AlphaProteo’s ability to design binders for SARS-CoV-2 could lead to faster and more precise COVID-19 diagnostic tools.
3. Immunotherapy
AlphaProteo’s ability to design highly specific protein binders is particularly valuable in the field of immunotherapy. Immunotherapies leverage the body’s immune system to fight diseases, including cancer. One challenge in this field is developing proteins that can bind to and modulate immune responses effectively. With AlphaProteo’s precision in targeting specific proteins on immune cells, it could enhance the development of new, more effective immunotherapies.
4. Biotechnology and Biosensors
AlphaProteo-designed protein binders are also valuable in biotechnology, particularly in the creation of biosensors—devices used to detect specific molecules in various environments. Biosensors have applications ranging from environmental monitoring to food safety. AlphaProteo’s binders could improve the sensitivity and specificity of these devices, making them more reliable in detecting harmful substances.
Limitations and Future Directions
As with any new technology, AlphaProteo is not without its limitations. For instance, the system struggled to design effective binders for the protein TNF𝛼, a challenging target associated with autoimmune diseases like rheumatoid arthritis. This highlights that while AlphaProteo is highly effective for many targets, it still has room for improvement.
DeepMind is actively working to expand AlphaProteo’s capabilities, particularly in addressing challenging targets like TNF𝛼. The team is also exploring new applications for the technology, including using AlphaProteo to design proteins for crop improvement and environmental sustainability.
Conclusion
By drastically reducing the time and cost associated with traditional protein design methods, AlphaProteo accelerates innovation in biology and medicine. Its success in creating protein binders for challenging targets like the SARS-CoV-2 spike protein and VEGF-A demonstrates its potential to address some of the most pressing health challenges of our time.
As AlphaProteo continues to evolve, its impact on science and society will only grow, offering new tools for understanding life at the molecular level and unlocking new possibilities for treating diseases.
#2024#3d#ai#ai model#AlphaFold#alphaproteo#applications#approach#architecture#arthritis#artificial#Artificial Intelligence#autoimmune diseases#bank#Biology#biosensors#biotechnology#Blue#Brain#Brain-computer interfaces#Building#Cancer#cancer cells#Cancer Therapy#cell#Cells#challenge#chip#Companies#computer
0 notes
Text
The science of a Bidirectional Brain Computer Interface with a function to work from a distance is mistakenly reinvented by laymen as the folklore of Remote Neural Monitoring and Controlling
Critical thinking
How good is your information when you call it RNM? It’s very bad. Is your information empirically validated when you call it RNM? No, it’s not empirically validated.
History of the RNM folklore
In 1992, a layman Mr. John St. Clair Akwei tried to explain a Bidirectional Brain Computer Interface (BCI) technology, which he didn't really understand. He called his theory Remote Neural Monitoring. Instead of using the scientific method, Akwei came up with his idea based on water. Lacking solid evidence, he presented his theory as if it were fact. Without any real studies to back him up, Akwei twisted facts, projected his views, and blamed the NSA. He lost his court case and was sadistically disabled by medical practitioners using disabling pills. They only call him something he is not. Since then, his theory has gained many followers. Akwei's explanation is incorrect and shallow, preventing proper problem-solving. As a result, people waste life-time searching for a true scientific explanation that can help solve this issue. When you call it RNM, the same will be done to you as to Mr. Akwei (calling you something you are not and sadistically disabling you with pills).
Critical thinking
Where does good research-based information come from? It comes from a university or from an R&D lab.
State of the art in Bidirectional BCI
Science-based explanation using Carnegie Mellon University Based on the definition of BCI (link to a scientific paper included), it’s a Bidirectional Brain Computer Interface for having a computer interact with the brain, and it’s extended only with 1 new function to work from a distance.
It’s the non-invasive BCI type, not an implanted BCI. The software running on the computer is a sense and respond system. It has a command/function that weaponizes the device for a clandestine sabotage against any person. It’s not from Tesla, it’s from an R&D lab of some secret service that needs it to do surveillance, sabotages and assassinations with a plausible deniability.
You need good quality information that is empirically validated, and such information comes from a university or from an R&D lab of some large organization. It won’t come from your own explanations because you are not empirically validating them which means you aren’t using the scientific method to discover new knowledge (it’s called basic research).
Goal: Detect a Bidirectional BCI extended to work from a distance (it’s called applied research, solving a problem using existing good quality information that is empirically validated)
Strategy: Continuous improvement of Knowledge Management (knowledge transfer/sharing/utilization from university courses to the community) to come up with hypotheses + experimentation with Muse2 to test your hypotheses and share when they are proved).
This strategy can use existing options as hypotheses which is then an applied research. Or, it can come up with new, original hypotheses and discover new knowledge by testing them (which is basic research). It can combine both as needed.
Carnegie Mellon University courses from Biomedical Engineering (BME)
Basics (recommended - make sure you read):
42665 | Brain-Computer Interface: Principles and Applications:
Intermediate stuff (optional - some labs to practice):
2. 42783 | Neural Engineering laboratory - Neural engineering involves the practice of using tools we use to measure and manipulate neural activity: https://www.coursicle.com/cmu/courses/BMD/42783/
Expert stuff (only if you want to know the underlying physics behind BCI):
3. 18612 | Neural Technology: Sensing and Stimulation (this is the physics of brain cells, explaining how they can be read from and written into) https://www.andrew.cmu.edu/user/skkelly/18819e/18819E_Syllabus_F12.pdf
You have to read those books to facilitate knowledge transfer from the university to you.
With the above good quality knowledge that is empirically validated, the Bidirectional BCI can be likely detected (meaning proved) and in the process, new knowledge about it can be discovered.
Purchase a cheap unidirectional BCI device for experiments at home
Utilize all newly gained knowledge from the above books in practice to make educated guesses based on the books and then empirically validate them with Muse2. After it is validated, share your good quality, empirically validated information about the undisclosed Bidirectional BCI with the community (incl. the steps to validate it).
Python Project
Someone who knows Python should try to train an AI model to detect when what you hear is not from your ear drums. Here is my initial code: https://github.com/michaloblastni/insultdetector You can try this and send me your findings and improvements.
How to do research
Basic research makes progress by doing a literature review regarding a phenomenon, then identifying main explanatory theories, making new hypotheses and conducting experiments to find what happens. When new hypotheses are proved the existing knowledge is extended. New findings can be contributed back to extend existing theories.
In practice, you will review existing scientific theories that explain i.e. the biophysics behind sensing and stimulating brain activity, and you will try to extend those theories by coming up with new hypotheses and experimentally validating them. And then, you will repeat the cycle to discover more new knowledge. When it's a lot of iterations, you need a team.
In applied research, you start with a problem that needs solving. You do a literature review and study previous solutions to the problem. Then, you should synthesize a new solution from the existing ones, and it should involve extending them in a meaningful way. Your new solution should solve the problem in some measurably better way. You have to demonstrate what your novel solution does better i.e. by measuring it, or by proving it with some other way.
In practice, you will do a literature review of past designs of Bidirectional BCI and make them your design options. Then, you will synthesize a new design option from all the design options you reviewed. The new design will get you closer toward making a Bidirectional BCI work from a distance. Then, you will repeat the cycle to improve upon your design further until you eventually reach the goal. When it's a lot of iterations, you need a team.
Using a Bidirectional BCI device to achieve synthetic telepathy
How to approach learning, researching and life
At the core, the brain is a biological neural network. You make your own connections in it stronger when you repeatedly think of something (i.e. while watching an expert researcher on youtube). And your connections weaken and disconnect/reconnect/etc. when you stop thinking of something (i.e. you stop watching an expert on how to research and you start watching negative news instead).
You train yourself by watching/listening/hanging out with people, and by reading about/writing about/listening to/doing certain tasks, and also by other means.
The brain has a very limited way of functioning because when you stop repeatedly thinking of something it soon starts disappearing. Some people call it knowledge evaporation. It’s the disconnecting and reconnecting of neurons in your biological neural network. Old knowledge is gone and new knowledge is formed. It’s called neuroplasticity. It’s the ability of neurons to disconnect, connect elsewhere, etc. based on what you are thinking/reading/writing/listening/doing.
Minimize complexity by starting from the big picture (i.e. a theory that explains a phenomenon). Then, proceed and do problem solving with a top-down decomposition into subproblems. Focus only on key information for the purpose of each subproblem and skip other details. Solve separate subproblems separately.
2 notes
·
View notes
Text
The Intricacies of Cognitive Neuroscience
Introduction
Cognitive neuroscience is a multidisciplinary field that seeks to understand the complex interplay between the brain, cognition, and behaviour. It merges principles from psychology, neuroscience, and computer science to explore the neural mechanisms underlying various cognitive processes.
1. The Fundamentals of Cognitive Neuroscience
Cognitive neuroscience aims to unravel the mysteries of the mind by studying how neural activity gives rise to cognitive functions such as perception, memory, language, and decision-making. By examining brain structure and function using advanced imaging techniques like functional magnetic resonance imaging (fMRI) and electroencephalography (EEG), researchers can map cognitive processes onto specific brain regions.
2. Neural Basis of Perception and Sensation
Perception and sensation are fundamental processes through which organisms interpret and make sense of the world around them. Cognitive neuroscience investigates how sensory information is processed in the brain, from the initial encoding of sensory stimuli to higher-order perceptual processes that shape our conscious experience of the world.
3. Memory Encoding, Storage, and Retrieval
Memory is a cornerstone of cognition, allowing us to retain and retrieve information from past experiences. Cognitive neuroscience examines the neural mechanisms underlying memory encoding, storage, and retrieval, shedding light on how memories are formed, consolidated, and recalled. This research has implications for understanding memory disorders and developing strategies to enhance memory function.
4. Language Processing and Communication
Language is a uniquely human ability that plays a central role in communication and social interaction. Cognitive neuroscience investigates how language is processed in the brain, from the comprehension of spoken and written words to the production of speech and the interpretation of linguistic meaning. By studying language disorders like aphasia, researchers gain insights into the neural basis of language processing.
5. Decision-Making and Executive Function
Decision-making is a complex cognitive process that involves weighing multiple options, evaluating potential outcomes, and selecting the most appropriate course of action. Cognitive neuroscience explores the neural circuits involved in decision-making and executive function, including areas of the prefrontal cortex responsible for cognitive control, planning, and goal-directed behaviour.
6. Emotion Regulation and Affective Neuroscience
Emotions play a crucial role in shaping our thoughts, behaviours, and social interactions. Affective neuroscience investigates the neural basis of emotion processing, regulation, and expression, shedding light on how emotions are represented in the brain and influence decision-making, memory, and social behaviour. This research has implications for understanding mood disorders and developing interventions to promote emotional well-being.
7. Neuroplasticity and Brain Plasticity
Neuroplasticity refers to the brain’s remarkable ability to reorganize and adapt in response to experience, learning, and environmental changes. Cognitive neuroscience examines the mechanisms underlying neuroplasticity, from synaptic plasticity at the cellular level to large-scale changes in brain connectivity and function. Understanding neuroplasticity has implications for rehabilitation after brain injury and for enhancing cognitive function throughout the lifespan.
8. Applications of Cognitive Neuroscience
Cognitive neuroscience findings have far-reaching applications in fields such as education, healthcare, technology, and beyond. By elucidating the neural mechanisms underlying cognition and behaviour, cognitive neuroscience informs the development of interventions for cognitive enhancement, rehabilitation therapies for neurological disorders, and technological innovations like brain-computer interfaces.
9. Future Directions and Challenges
As technology advances and our understanding of the brain grows, cognitive neuroscience continues to evolve. Future research may focus on integrating data from multiple levels of analysis, from genes to behaviour, to gain a comprehensive understanding of brain function. Challenges in cognitive neuroscience include navigating ethical considerations, addressing methodological limitations, and fostering interdisciplinary collaboration to tackle complex questions about the mind and brain.
Conclusion
Cognitive neuroscience offers a fascinating window into the inner workings of the human mind, exploring the neural basis of cognition, perception, emotion, and behaviour. By combining insights from psychology, neuroscience, and computational modelling, cognitive neuroscience continues to unravel the mysteries of the brain, paving the way for advances in education, healthcare, and technology.
FAQs
1. What careers are available in cognitive neuroscience? Cognitive neuroscience opens doors to various career paths, including research, academia, clinical practice, and industry roles in technology and healthcare.
2. How does cognitive neuroscience differ from traditional neuroscience? While traditional neuroscience focuses on the structure and function of the brain, cognitive neuroscience specifically investigates how these processes give rise to cognitive functions like perception, memory, and language.
3. Can cognitive neuroscience help improve mental health treatments? Yes, cognitive neuroscience provides insights into the neural mechanisms underlying mental health disorders, leading to more effective treatments and interventions.
4. Is cognitive neuroscience only relevant to humans? No, cognitive neuroscience research extends to other species, providing valuable insights into the evolution of cognitive processes across different organisms.
5. How can I get involved in cognitive neuroscience research as a student? Many universities offer undergraduate and graduate programs in cognitive neuroscience, allowing students to pursue research opportunities and gain hands-on experience in the field.
#cognitive functions#NeuroscienceInsights#BrainPlasticity#MindAndBrain#NeuralMechanisms#brain research#CognitiveNeuroscience
2 notes
·
View notes
Text
Course Outline
Foundations of Neuroscience | Month #1
Weeks 1—2 | Introduction to Neuroscience, Neurons, and Neural Signaling
terminologies | neuron, action potential, synapse, neurotransmitter
concepts | structure & function of neurons, membrane potential, neurotransmission
Questions & Objectives → What are the basic building blocks of the nervous system? → How do neurons communicate with each other? → What role do neurotransmitters play in neural signaling?
Weeks 3—4 | Brain Development, Neuroanatomy, & Neutral Circuits
terminologies | neurogenesis, synaptogenesis, cortex, hippocampus, basal ganglia
concepts | embryonic brain development, brain regions & their functions, neural circuits
Questions & Objectives → How does the brain develop from embryo to adulthood? → What are the major anatomical structures oft he brain, and what functions do they serve? → How are neural circuits formed and how do they contribute to behaviour?
Weeks 5—6 | Sensory Systems & Motor Control
terminologies | sensory receptors, somatosensory cortex, motor cortex, proprioception
concepts | sensory processing motor control, sensory-motor integration
Questions & Objectives - > How do sensory systems detect and process environmental stimuli? → What neural mechanisms underlie voluntary and involuntary movement? → How does the brain coordinate sensory inputs with motor outputs?
Weeks 7 | Mid-terms Review and Assessment
Objective | Review key concepts, terminology, & principles covered in the first month. Assess understanding through quizzes, assignments, or exams.
Advanced Topics & Applications | Month 2.
Weeks 1—2 | Learning & Memory, Emotions, & Motivation
terminologies | hippocampus, amygdala, long-term potentiation, reward pathway
concepts | neural basis of learning & memory, emotional processing, motivation
Questions & Objectives → How are memories formed and stored in the brain? → What brain regions are involved in emotional processing, and how do they interact? → How does the brain regulate motivation and reward-seeking behaviour?
Weeks 3—4 | Neurological Disorders, Neuroplasticity, & Repair
terminologies | Neurodegeneration, neuroplasticity, stroke, traumatic brain injury
concepts | causes and mechanisms of neurological disorders, neural repair & regeneration
Questions & Objectives → What are the underlying causes of neurodegenerative diseases such as Alzheimer’s and Parkinson’s? → How does the brain recover from injury or disease through neuroplasticity? → What are the current approaches to neural repair and regeneration?
Weeks 5—6 | Cognitive Neuroscience & Consciousness
terminologies | prefrontal cortex, executive function, consciousness, neural correlates
concepts | Higher cognitive functions, consciousness & awareness, neural correlates of consciousness
Questions & Objectives → How does the prefrontal cortex contribute to executive functions such as decision-making and problem-solving? → What is consciousness, and how can it be studied from a neuroscience perspective? → What neural correlates are associated with different states of consciousness?
Weeks 7—8 | Future Directions and Ethical Considerations
terminologies | optogenetics, connectome, neuroethics, brain-computer interface
concepts | emerging technologies in neuroscience, ethical considerations in neuroscientific research
Questions & Objectives - > What are the potential applications of optogenetics and brain-computer interfaces in neuroscience research and clinical practice? → How can ethical considerations be integrated into neuroscience research & technology development → What are the future directions and challenges in the field of neuroscience, & how can they be addressed?
Week 8 | Final Review and Assessment
Objectives | Review key concepts, terminologies, and emerging topics covered in the course. Assess understanding through a final exam or project.
Final.
#Neuroscience#course#courselearning#personal journey#intellectual#intellect#Dark Academia#intellectualism my beloved#Neuroscience.#studying#studyblog#<3
2 notes
·
View notes