#intelligent automation platform
Explore tagged Tumblr posts
Text
#business aircraft consumables#aircraft parts distributor#Intelligent Automation#Intelligent Automation solutions#intelligent automation platform#intelligent automation tools
0 notes
Text
Future-Ready Enterprises: The Crucial Role of Large Vision Models (LVMs)
New Post has been published on https://thedigitalinsider.com/future-ready-enterprises-the-crucial-role-of-large-vision-models-lvms/
Future-Ready Enterprises: The Crucial Role of Large Vision Models (LVMs)
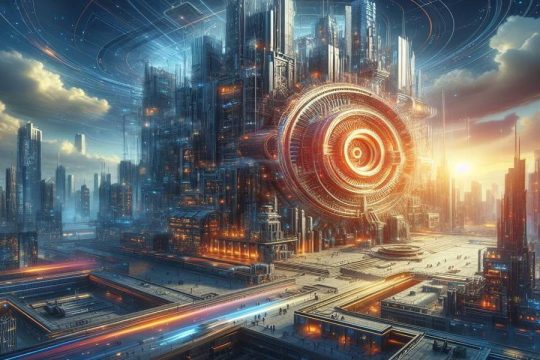
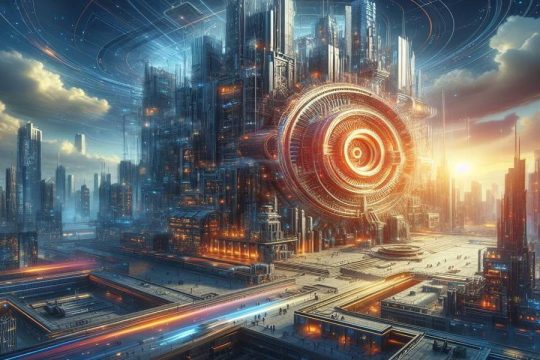
What are Large Vision Models (LVMs)
Over the last few decades, the field of Artificial Intelligence (AI) has experienced rapid growth, resulting in significant changes to various aspects of human society and business operations. AI has proven to be useful in task automation and process optimization, as well as in promoting creativity and innovation. However, as data complexity and diversity continue to increase, there is a growing need for more advanced AI models that can comprehend and handle these challenges effectively. This is where the emergence of Large Vision Models (LVMs) becomes crucial.
LVMs are a new category of AI models specifically designed for analyzing and interpreting visual information, such as images and videos, on a large scale, with impressive accuracy. Unlike traditional computer vision models that rely on manual feature crafting, LVMs leverage deep learning techniques, utilizing extensive datasets to generate authentic and diverse outputs. An outstanding feature of LVMs is their ability to seamlessly integrate visual information with other modalities, such as natural language and audio, enabling a comprehensive understanding and generation of multimodal outputs.
LVMs are defined by their key attributes and capabilities, including their proficiency in advanced image and video processing tasks related to natural language and visual information. This includes tasks like generating captions, descriptions, stories, code, and more. LVMs also exhibit multimodal learning by effectively processing information from various sources, such as text, images, videos, and audio, resulting in outputs across different modalities.
Additionally, LVMs possess adaptability through transfer learning, meaning they can apply knowledge gained from one domain or task to another, with the capability to adapt to new data or scenarios through minimal fine-tuning. Moreover, their real-time decision-making capabilities empower rapid and adaptive responses, supporting interactive applications in gaming, education, and entertainment.
How LVMs Can Boost Enterprise Performance and Innovation?
Adopting LVMs can provide enterprises with powerful and promising technology to navigate the evolving AI discipline, making them more future-ready and competitive. LVMs have the potential to enhance productivity, efficiency, and innovation across various domains and applications. However, it is important to consider the ethical, security, and integration challenges associated with LVMs, which require responsible and careful management.
Moreover, LVMs enable insightful analytics by extracting and synthesizing information from diverse visual data sources, including images, videos, and text. Their capability to generate realistic outputs, such as captions, descriptions, stories, and code based on visual inputs, empowers enterprises to make informed decisions and optimize strategies. The creative potential of LVMs emerges in their ability to develop new business models and opportunities, particularly those using visual data and multimodal capabilities.
Prominent examples of enterprises adopting LVMs for these advantages include Landing AI, a computer vision cloud platform addressing diverse computer vision challenges, and Snowflake, a cloud data platform facilitating LVM deployment through Snowpark Container Services. Additionally, OpenAI, contributes to LVM development with models like GPT-4, CLIP, DALL-E, and OpenAI Codex, capable of handling various tasks involving natural language and visual information.
In the post-pandemic landscape, LVMs offer additional benefits by assisting enterprises in adapting to remote work, online shopping trends, and digital transformation. Whether enabling remote collaboration, enhancing online marketing and sales through personalized recommendations, or contributing to digital health and wellness via telemedicine, LVMs emerge as powerful tools.
Challenges and Considerations for Enterprises in LVM Adoption
While the promises of LVMs are extensive, their adoption is not without challenges and considerations. Ethical implications are significant, covering issues related to bias, transparency, and accountability. Instances of bias in data or outputs can lead to unfair or inaccurate representations, potentially undermining the trust and fairness associated with LVMs. Thus, ensuring transparency in how LVMs operate and the accountability of developers and users for their consequences becomes essential.
Security concerns add another layer of complexity, requiring the protection of sensitive data processed by LVMs and precautions against adversarial attacks. Sensitive information, ranging from health records to financial transactions, demands robust security measures to preserve privacy, integrity, and reliability.
Integration and scalability hurdles pose additional challenges, especially for large enterprises. Ensuring compatibility with existing systems and processes becomes a crucial factor to consider. Enterprises need to explore tools and technologies that facilitate and optimize the integration of LVMs. Container services, cloud platforms, and specialized platforms for computer vision offer solutions to enhance the interoperability, performance, and accessibility of LVMs.
To tackle these challenges, enterprises must adopt best practices and frameworks for responsible LVM use. Prioritizing data quality, establishing governance policies, and complying with relevant regulations are important steps. These measures ensure the validity, consistency, and accountability of LVMs, enhancing their value, performance, and compliance within enterprise settings.
Future Trends and Possibilities for LVMs
With the adoption of digital transformation by enterprises, the domain of LVMs is poised for further evolution. Anticipated advancements in model architectures, training techniques, and application areas will drive LVMs to become more robust, efficient, and versatile. For example, self-supervised learning, which enables LVMs to learn from unlabeled data without human intervention, is expected to gain prominence.
Likewise, transformer models, renowned for their ability to process sequential data using attention mechanisms, are likely to contribute to state-of-the-art outcomes in various tasks. Similarly, Zero-shot learning, allowing LVMs to perform tasks they have not been explicitly trained on, is set to expand their capabilities even further.
Simultaneously, the scope of LVM application areas is expected to widen, encompassing new industries and domains. Medical imaging, in particular, holds promise as an avenue where LVMs could assist in the diagnosis, monitoring, and treatment of various diseases and conditions, including cancer, COVID-19, and Alzheimer’s.
In the e-commerce sector, LVMs are expected to enhance personalization, optimize pricing strategies, and increase conversion rates by analyzing and generating images and videos of products and customers. The entertainment industry also stands to benefit as LVMs contribute to the creation and distribution of captivating and immersive content across movies, games, and music.
To fully utilize the potential of these future trends, enterprises must focus on acquiring and developing the necessary skills and competencies for the adoption and implementation of LVMs. In addition to technical challenges, successfully integrating LVMs into enterprise workflows requires a clear strategic vision, a robust organizational culture, and a capable team. Key skills and competencies include data literacy, which encompasses the ability to understand, analyze, and communicate data.
The Bottom Line
In conclusion, LVMs are effective tools for enterprises, promising transformative impacts on productivity, efficiency, and innovation. Despite challenges, embracing best practices and advanced technologies can overcome hurdles. LVMs are envisioned not just as tools but as pivotal contributors to the next technological era, requiring a thoughtful approach. A practical adoption of LVMs ensures future readiness, acknowledging their evolving role for responsible integration into business processes.
#Accessibility#ai#Alzheimer's#Analytics#applications#approach#Art#artificial#Artificial Intelligence#attention#audio#automation#Bias#Business#Cancer#Cloud#cloud data#cloud platform#code#codex#Collaboration#Commerce#complexity#compliance#comprehensive#computer#Computer vision#container#content#covid
2 notes
·
View notes
Text
The AI-Powered Future of Data-Driven Decision-Making
In a world overwhelmed by data, the ability to make quick, accurate, and impactful decisions is now a key competitive differentiator. Across industries from boardrooms to hospitals, trading floors to creative teams, AI is more than a tool; it's reshaping how decisions get made. But what if you could take this a step further? What if you could automate data insights with machine learning and leverage AI solutions for data-driven decision-making across your entire operation?
Welcome to the AI-powered future.
What is Data-Driven Decision-Making?
Data-driven decision-making (DDDM) refers to the process of using data analytics to guide business strategies, operational improvements, and customer engagement. It involves collecting relevant data, analyzing it to extract meaningful patterns, and using those insights to make informed decisions.
Traditionally, data-driven decisions required human analysts to sift through spreadsheets, dashboards, and reports. But with the explosion of data volume and complexity, manual methods are no longer sufficient. This is where artificial intelligence (AI) and machine learning (ML) come in.
The Role of AI and Machine Learning in Decision-Making
Artificial intelligence enables machines to mimic human cognitive functions such as learning, reasoning, and problem-solving. Machine learning, a subset of AI, focuses on algorithms that can learn from and make predictions or decisions based on data.
These technologies offer two major advantages:
Speed and Scale: AI can analyze vast datasets in real-time, far beyond what a human team could manage.
Pattern Recognition: Machine learning algorithms can identify subtle correlations and anomalies that traditional analysis might miss.
By integrating these tools, organizations can automate data insights with machine learning, allowing them to act on information faster and more accurately
Key Benefits of AI Solutions for Data-Driven Decision-Making
1. Faster Time to Insight
AI reduces the time needed to move from raw data to actionable insight. Automated systems can pull data from various sources, clean it, process it, and generate insights in minutes rather than days.
2. Improved Accuracy and Consistency
Human error and bias can skew analysis. AI-driven models, when trained on quality data, provide consistent and objective outputs that enhance decision accuracy.
3. Real-Time Decision-Making
In industries like finance and healthcare, real-time decisions can be critical. AI allows systems to evaluate scenarios and make recommendations in real-time, enabling rapid response to changing conditions.
4. Predictive and Prescriptive Analytics
AI doesn’t just explain what happened; it predicts what’s likely to happen and prescribes what to do about it. This enables proactive rather than reactive decision-making.
5. Pattern Recognition
Machine learning algorithms can uncover hidden patterns, correlations, and anomalies in data that are often missed by traditional analytics. This leads to deeper insights and more nuanced strategies.
6. Scalability
Once deployed, AI systems can handle more data and more complex analyses without significantly increasing cost or time investment. This scalability makes AI a valuable asset for organizations of all sizes.
Real-World Applications Across Industries
Healthcare
AI is revolutionizing patient care by helping physicians diagnose diseases earlier, personalize treatment plans, and predict patient outcomes based on historical data. Machine learning models analyze thousands of data points in electronic health records (EHRs) to deliver evidence-based recommendations.
In the future, AI will power real-time health monitoring through wearable devices, assist in robotic surgeries with precision guidance, and even aid drug discovery through deep learning models.
Finance
Financial institutions use AI to detect fraudulent transactions, assess loan applications, and optimize trading strategies. Automated data insights with machine learning provide real-time risk assessments and forecasting.
Looking ahead, AI will power decentralized finance (DeFi) analytics, robo-advisors for personalized financial planning, and autonomous auditing systems that reduce regulatory burdens.
Retail and E-commerce
AI helps retailers forecast demand, manage inventory, and personalize customer experiences. Recommendation engines, driven by machine learning, tailor product suggestions based on individual user behavior.
In the future, AI will enable hyper-personalized shopping experiences with real-time sentiment analysis, dynamic pricing engines, and AI-powered visual search technologies.
Marketing
AI-driven analytics platforms enable marketers to segment audiences, optimize campaign performance, and predict customer churn. By automating these insights, teams can focus on strategy rather than number crunching.
The AI-powered future of marketing will include conversational AI for customer engagement, predictive content creation, and emotion-aware ad targeting for deeper personalization.
Manufacturing
In manufacturing, AI supports predictive maintenance, quality control, and supply chain optimization. Machine learning algorithms monitor equipment in real time to detect issues before they become critical.
Soon, AI will enable smart factories with autonomous robotics, AI-driven design for prototyping, and closed-loop feedback systems for continuous process improvement.
Education
In education, AI is streamlining administrative tasks, customizing learning pathways, and enhancing student engagement. Adaptive learning platforms analyze student behavior and tailor content to their needs.
The future of education will include AI tutors offering 24/7 support, real-time performance feedback, and curriculum design based on labor market analytics.
Public Sector & Governance
Governments are beginning to use AI for smarter city planning, resource allocation, and public safety. From traffic optimization to predictive policing, AI can enhance citizen services.
We can expect AI-powered policy simulations, citizen sentiment analysis, and digital twins of cities for proactive urban planning going forward.
How to Get Started with AI for Decision-Making
1. Assess Your Data Maturity
Before diving into AI, evaluate your current data infrastructure. Do you have clean, structured, and accessible data? Are your teams equipped to interpret and act on AI-generated insights?
2. Start Small, Scale Strategically
Begin with pilot projects that solve specific business problems. For instance, use AI to improve customer segmentation or automate financial forecasting. Once proven, scale the solution across departments.
3. Invest in the Right Tools and Platforms
There are many AI platforms that don’t require deep technical expertise. Tools like Google AutoML, Microsoft Azure AI, and DataRobot offer user-friendly interfaces for automating data insights with machine learning.
4. Foster a Data-Driven Culture
Adopting AI isn’t just about technology—it’s about mindset. Train staff, encourage experimentation, and align KPIs with data-driven outcomes to ensure organization-wide adoption.
Challenges and Considerations
While the benefits are substantial, implementing AI for decision-making also comes with challenges:
Data Quality: AI is only as good as the data it’s trained on.[Text Wrapping Break]Tip: Establish strong data governance and cleaning practices early.
Transparency and Explainability: Some AI models (like deep learning) act as "black boxes," making it hard to understand how decisions are made.[Text Wrapping Break]Tip: Use interpretable models or tools like SHAP and LIME to explain outputs.
Bias and Fairness: Without careful oversight, AI can perpetuate or amplify existing biases in the data.[Text Wrapping Break]Tip: Audit datasets for bias and regularly validate model outputs for fairness.
Change Management: Transitioning to AI-driven processes requires change at both the operational and cultural levels.[Text Wrapping Break]Tip: Communicate benefits clearly and involve stakeholders early in the adoption process.
The Future Outlook
As AI technology matures, its accessibility and impact will continue to grow. We can expect:
More explainable AI, helping decision-makers trust and understand AI-driven insights.
Increased use of real-time analytics for instant decision-making.
Edge AI for processing data locally and instantly, especially in IoT and manufacturing.
Autonomous enterprise systems that dynamically adjust operations based on real-time input.
AI integration in cross-industry ecosystems, enabling shared insights and collaboration at scale.
Greater use of multi-modal AI that processes text, audio, image, and video data simultaneously for richer insights.
The age of AI-powered decision-making is a profound turning point—not just for how we use data, but for how we think about it. AI is more than a technological upgrade; it’s an enabler of creativity, precision, and growth across industries. By embracing its potential, organizations can make smarter decisions, adapt to real-time challenges, and unlock untapped opportunities.
As we move forward, embracing AI isn’t just a matter of staying ahead—it’s about reimagining what’s possible in a world driven by intelligence and change.
The organizations that thrive in the future will be those that learn to combine human intuition with AI precision.
Learn more about DataPeak:
#datapeak#factr#saas#technology#agentic ai#machine learning#artificial intelligence#ai#ai-driven business solutions#machine learning for workflow#ai business tools#ai solutions for data driven decision making#aiinnovation#agentic#agenticai#best ai tools for data pipeline automation#ai platform for business process automation#automation#ai driven data workflow automation#digitaltools#digital trends#digital technology#datadrivendecisions#data driven decision making#dataanalytics#data driven decisions
0 notes
Text
How to Use n8n and AI to Build an Automation System
Automation is changing how we work every day. It helps save time, reduce mistakes, and get more done with less effort. If you want to automate your tasks but don’t know where to start, this guide is for you. In this post, you will learn how to use n8n — a free, open-source automation tool — combined with AI to build smart workflows that do work for you. What Is n8n? n8n (pronounced…
#AI automation#AI integration#AI workflow#AI-powered workflows#API integration#artificial intelligence tools#automate emails#automate tasks#automation platform#automation software#automation system#automation tips#business automation#chatbot automation#data processing automation#email automation#intelligent automation#low-code automation#n8n automation#no-code automation#open source automation#productivity tools#smart automation#time-saving tools#workflow automation#workflow builder
0 notes
Text
Unlocking the Future of Manufacturing: AI Innovation Panel with Certivo & Industry Leaders
Join Certivo at "Manufacturing Reimagined: AI-Powered Innovation" — a dynamic panel event exploring how AI is transforming the manufacturing value chain. Discover how automation and intelligent compliance can accelerate product launches and reduce regulatory delays. Hear from industry pioneers including Certivo CEO Kunal Chopra, T.A. McCann (PSL), and Vineet Thuvara (Fluke). Hosted by AAIA Seattle, PSL, and K&L Gates. 📅 May 20, 2025 | 3:30–5:30 PM | Downtown Seattle Reserve your seat now: https://lu.ma/o9h7ds6s Learn more about our AI solutions at certivo.com
#AI-powered compliance solutions for manufacturing#automate product compliance in manufacturing#AI tools to reduce regulatory delays#AI-driven product launch acceleration#intelligent compliance software for R&D teams#streamline manufacturing compliance with AI#real-time regulatory monitoring platform#AI-based risk management in manufacturing#enterprise AI solutions for global product launch#manufacturing innovation event Seattle 2025
0 notes
Text
How Spotify uses Ghost Writers and AI Content to Increase their Profits
Making a Scene Presents How Spotify uses Ghost Writers and AI Content to Increase their Profits Spotify has significantly reshaped the music streaming landscape, becoming an integral part of millions of listeners’ daily lives. However, behind the curated playlists and mood-setting tracks lies a controversial strategy involving “ghost artists” and artificial intelligence-generated music, aimed at…
#AI in ambient music#AI music controversy#AI-generated music#algorithmic music creation#ambient music streaming#artificial intelligence in music#Chill Hits Spotify#Deep Focus playlist#fake artists on Spotify#ghostwritten playlists#indie artist streaming issues#music automation#music industry ethics#music streaming secrets#Peaceful Piano Spotify#Spotify AI music#Spotify ghost artists#Spotify mood playlists#Spotify profit strategy#Spotify royalty avoidance#streaming platform manipulation
0 notes
Text
هوش مصنوعی در تجارت: الگوریتمهای یادگیری ماشین چگونه بازار را تحلیل میکنند | uaitrading
در دنیای بازارهای مالی که به سرعت در حال توسعه هستند، تجارت مبتنی بر هوش مصنوعی به یک تغییر بازی تبدیل شده است. در uaitrading.ai، ما از قدرت هوش مصنوعی و یادگیری ماشینی برای تبدیل دادههای خام به بینشهای عملی استفاده میکنیم و به معاملهگران در چشمانداز رقابتی امروز برتری میدهیم.
نحوه عملکرد یادگیری ماشین در تجارت سیستمهای هوشمند ما فقط دادهها را پردازش نمیکنند، بلکه از آنها یاد میگیرند. با تجزیه و تحلیل روندهای تاریخی و سیگنال های بازار در زمان واقعی، الگوریتم های ML فرصت ها را شناسایی کرده و با شرایط متغیر سازگار می شوند. در اینجا نحوه انجام آن آمده است:
تجزیه و تحلیل سری زمانی با استفاده از مدلهایی مانند ARIMA، LSTM، و Prophet، با تجزیه و تحلیل دادههای قیمت تاریخی و شناسایی الگوهای تکرارشونده، حرکات بازار را پیشبینی میکنیم.
شبکه های عصبی مدلهای یادگیری عمیق ما روابط غیرخطی و همبستگیهای پنهان را در بین متغیرهای متعدد تشخیص میدهند - قابلیتهای پیشبینی قدرتمند را باز میکنند.
یادگیری تقویتی نمایندگان معاملاتی در uaitrading از هر تصمیمی که گرفته میشود یاد میگیرند – بهینهسازی استراتژیها در طول زمان از طریق حلقههای بازخورد آزمون، خطا و پاداش.
تجزیه و تحلیل احساسات هوش مصنوعی اخبار مالی، رسانههای اجتماعی و سرفصلهای جهانی را اسکن میکند تا احساسات سرمایهگذاران را بسنجد - زمینهای فراتر از نمودارها را فراهم میکند.
مزایا و معایب هوش مصنوعی در تجارت ✅ چرا از هوش مصنوعی در تجارت استفاده کنیم؟ تجزیه و تحلیل با سرعت بالا: میلیون ها نقطه داده را در میلی ثانیه پردازش کنید.
تصمیمات عاری از احساسات: تعصبات انسانی را از استراتژی های معاملاتی حذف کنید.
اتوماسیون کامل: از تشخیص سیگنال تا اجرا - نیازی به مداخله دستی نیست.
❌ چالش ها چیست؟ رویدادهای قو سیاه: هوش مصنوعی همیشه نمی تواند غیرقابل پیش بینی ها را پیش بینی کند.
خطرات بیش از حد: اتکای بیش از حد به داده های تاریخی می تواند گمراه کننده باشد.
Garbage In, Garbage Out: داده های نادرست یا با کیفیت پایین می توانند نتایج را به خطر بیندازند.
در uaitrading، ما به ترکیب قدرت فناوری با درک عمیق بازار اعتقاد داریم. در حالی که هوش مصنوعی دقت و سرعت را افزایش می دهد، مدیریت ریسک و بینش انسانی برای تجارت موفق ضروری است.
آماده کاوش در تجارت مبتنی بر هوش مصنوعی هستید؟ به uaitrading.ai بپیوندید و هوشمندانه تر تجارت کنید.
#AI trading platform#AGI trading#UAI token farming#AI stock trading#forex automation#algorithmic trading#AI portfolio manager#crypto trading#blockchain AI#passive income crypto#Forex trading platform#Artificial intelligence stock trading#AI options trading#best AI for forex trading#AI algorithmic trading#AI for forex trading#AI in forex trading#trading with ChatGPT#forex artificial intelligence
1 note
·
View note
Text
How To Design A Reward Function For Trading Scenarios In Algorithmic Trading?

Design optimal reward functions for algo trading in India. Balance profits, risks compliance with strategies like Sharpe ratio, drawdowns backtesting.
Read more..
#Algorithmic trading for beginners#Algorithmic trading strategy#Algorithmic trading India#Algorithmic trading software#Basics of Algorithmic Trading#Automated Trading#algo trading#algo trading app#bigul#algo trading platform#algo trading india#algo trading strategies#bigul algo#free algo trading software#algorithm software for trading#finance#best algo trading software in india#algorithmic trading software free#algos#algorithm#artificial intelligence#algorithmic trading in india#algorithmic trading software#bigul algo trading#algo trading software price#best algo trading software#bigul algo trading app#bigul trading#bigul algo trading review#bigul algo ideas
0 notes
Text
Next-Gen AR Development: Bringing Ideas to Life - Atcuality
Augmented reality is no longer just a futuristic concept—it’s here, transforming industries and enhancing real-world applications. At Atcuality, we specialize in custom AR solutions designed to create interactive, engaging, and scalable experiences for businesses of all sizes. Our augmented reality development services cater to diverse industries, including retail, tourism, real estate, and automotive, helping brands deliver unforgettable user experiences. Whether you need AR-powered product visualization, training simulations, or interactive storytelling, our expert team ensures a seamless, high-quality solution tailored to your needs. Elevate your business with Atcuality’s cutting-edge AR technology and shape the future of digital interaction today!
#website development#ai applications#artificial intelligence#augmented and virtual reality market#web development#information technology#emailmarketing#augmented reality#web design#digital marketing#augmentative and alternative communication#augmented intelligence#virtual reality#ar vr technology#digital consulting#digital services#iotsolutions#iot#iot platform#iot applications#iot development services#technologynews#iot solutions#iot integration#automation#software company#software development#software engineering#software testing#cash collection application
0 notes
Text
Customer Data Integration Tools for Retail Chains: Enhancing Customer Experience and Driving Sales
In today’s competitive retail landscape, customer data is more valuable than ever. Retail chains must seamlessly collect, analyze, and integrate customer information across multiple touchpoints to deliver personalized experiences and drive business growth. However, managing fragmented data from in-store transactions, e-commerce platforms, loyalty programs, and customer service interactions can be overwhelming. This is where Customer Data Integration (CDI) tools come in.
Why Retail Chains Need Customer Data Integration
Retail businesses interact with customers through multiple channels—physical stores, mobile apps, social media, and websites. Without an integrated approach, data remains siloed, making it difficult to gain a 360-degree view of customers. CDI tools consolidate information from all sources, ensuring businesses can:
Personalize Customer Experiences: By unifying customer data, retailers can offer targeted promotions, personalized recommendations, and tailored marketing messages.
Improve Operational Efficiency: Data integration eliminates redundancy, automates workflows, and enhances decision-making processes.
Enhance Customer Support: Integrated data enables customer service teams to access a complete customer history, leading to quicker issue resolution and improved customer satisfaction.
Boost Sales and Retention: Understanding customer behavior helps retailers optimize product offerings, pricing strategies, and loyalty programs to increase revenue and customer retention.
Key Features of Customer Data Integration Tools
Effective CDI tools provide retailers with the ability to:
Real-Time Data Synchronization – Ensure customer data is up-to-date across all systems, including CRM, POS, and marketing automation platforms.
AI-Powered Analytics – Use machine learning and predictive analytics to generate insights on customer preferences and buying patterns.
Omnichannel Integration – Connect customer data from both online and offline channels for a unified shopping experience.
Data Security & Compliance – Maintain compliance with regulations like GDPR and CCPA while safeguarding customer information.
Automated Customer Segmentation – Categorize customers based on demographics, purchase history, and engagement levels for more targeted marketing efforts.
Choosing the Right Customer Data Integration Tool
When selecting a CDI tool for your retail business, consider the following:
Scalability: Can the tool handle growing data volumes as your retail chain expands?
Ease of Integration: Does it seamlessly integrate with your existing POS, CRM, and e-commerce platforms?
Customization: Does it offer flexibility to meet your specific business needs?
User-Friendly Interface: Can your team easily navigate and leverage its features without extensive technical training?
Unlock the Power of Customer Data with AntBuddy Inc.
At AntBuddy Inc., we specialize in cutting-edge Customer Data Integration solutions designed specifically for retail chains. Our platform helps businesses streamline customer data, gain actionable insights, and enhance customer engagement like never before.
Don’t let fragmented data hold your business back. Connect with AntBuddy Inc. today and take your retail operations to the next level with intelligent data integration!
🚀 Get started now! Contact us for a free consultation.
#crm omnichannel#omnichannel là gì#hàng thân thiết#Retail CRM#Voice brandname service#Intelligent automation#Customer engagement platform
0 notes
Text
#best distributor management system#b2b order management software#best sales force automation software#sales automation platform#retail intelligence software
0 notes
Text
Anaconda Launches First Unified AI Platform for Open Source, Redefining Enterprise-Grade AI Development
New Post has been published on https://thedigitalinsider.com/anaconda-launches-first-unified-ai-platform-for-open-source-redefining-enterprise-grade-ai-development/
Anaconda Launches First Unified AI Platform for Open Source, Redefining Enterprise-Grade AI Development
In a landmark announcement for the open-source AI community, Anaconda Inc., a long-time leader in Python-based data science, has launched the Anaconda AI Platform — the first unified AI development platform tailored specifically to open source. Aimed at streamlining and securing the end-to-end AI lifecycle, this platform enables enterprises to move from experimentation to production faster, safer, and more efficiently than ever before.
The launch represents not only a new product offering but a strategic pivot for the company: from being the de facto package manager for Python to now becoming the enterprise AI backbone for open-source innovation.
Bridging the Gap Between Innovation and Enterprise-Grade AI
The rapid rise of open-source tools has been a catalyst in the AI revolution. However, while frameworks like TensorFlow, PyTorch, scikit-learn, and Hugging Face Transformers have lowered the barrier to experimentation, enterprises face unique challenges in deploying these tools at scale. Issues like security vulnerabilities, dependency conflicts, compliance risks, and governance limitations often block enterprise adoption — slowing innovation just when it’s most needed.
Anaconda’s new platform is purpose-built to close this gap.
“Until now, there hasn’t been a single destination for AI development with open source, which is the backbone for inclusive and innovative AI,” said Peter Wang, Co-founder and Chief AI & Innovation Officer of Anaconda. “We’re not only offering streamlined workflows, enhanced security, and substantial time savings, but ultimately, giving enterprises the freedom to build AI their way — without compromise.”
What Makes It the First Unified AI Platform for Open Source?
The Anaconda AI Platform centralizes everything enterprises need to build and operationalize AI solutions based on open-source software. Unlike other platforms that specialize in just model hosting or experimentation, Anaconda’s platform covers the full AI lifecycle — from sourcing and securing packages to deploying production-ready models across any environment.
Key Capabilities of the Platform Include:
Trusted Open-Source Package Distribution:Includes access to over 8,000 pre-vetted, secure packages fully compatible with Anaconda Distribution. All packages are continuously tested for vulnerabilities, making it easier for enterprises to adopt open-source tools with confidence.
Secure AI & Governance:Enterprise-grade security features like Single Sign-On (SSO), role-based access control, and audit logging ensure traceability, user accountability, and compliance with regulations such as GDPR, HIPAA, and SOC 2.
AI-Ready Workspaces & Environments:Pre-configured “Quick Start” environments for use cases like finance, machine learning, and Python analytics accelerate time to value and reduce the need for configuration-heavy setup.
Unified CLI with AI Assistant:A command-line interface powered by an AI assistant helps developers resolve errors automatically, minimizing context switching and debugging time.
MLOps-Ready Integration:Built-in tools for monitoring, error tracking, and package auditing streamline MLOps (Machine Learning Operations), a critical discipline that bridges data science and production engineering.
What Is MLOps and Why Does It Matter?
MLOps is to AI what DevOps is to software development: a set of practices and tools that ensure machine learning models are not only developed but also deployed, monitored, updated, and scaled responsibly. Anaconda’s AI Platform is tightly aligned with MLOps principles, allowing teams to standardize workflows, track model lineage, and optimize model performance in real-time.
By centralizing governance, automation, and collaboration, the platform simplifies what is typically a fragmented and error-prone process. This unified approach is a game-changer for organizations trying to industrialize AI capabilities across teams.
Why Now? A Surge in Open-Source AI, But With Hidden Costs
Open source has become the foundation of modern AI. A recent study cited by Anaconda found that 50% of data scientists rely on open-source tools daily, and 66% of IT administrators confirm that open-source software plays a critical role in their enterprise tech stacks. However, the freedom and flexibility of open source come with trade-offs — especially around security and compliance.
Each time a team installs a package from a public repository like PyPI or GitHub, they introduce potential security risks. These vulnerabilities are difficult to track manually, especially when organizations rely on hundreds of packages, often with deep dependency trees.
With the Anaconda AI Platform, this complexity is abstracted away. Teams gain real-time visibility into package vulnerabilities, usage patterns, and compliance requirements — all while using the tools they know and love.
Enterprise Impact: Measurable ROI and Reduced Risk
To understand the business value of the platform, Anaconda commissioned a Total Economic Impact™ (TEI) study from Forrester Consulting. The findings are striking:
119% ROI over three years.
80% improvement in operational efficiency (worth $840,000).
60% reduction in risk of security breaches tied to package vulnerabilities.
80% reduction in time spent on package security management.
These results demonstrate that the Anaconda AI Platform is not just a developer tool — it’s a strategic enterprise asset that reduces overhead, enhances productivity, and accelerates time-to-value in AI development.
A Company Rooted in Open Source, Built for the AI Era
Anaconda isn’t new to the AI or data science space. The company was founded in 2012 by Peter Wang and Travis Oliphant, with the mission to bring Python — then an emerging language — into the mainstream of enterprise data analytics. Today, Python is the most widely used language in AI and machine learning, and Anaconda sits at the heart of that movement.
From a team of a few open-source contributors, the company has grown into a global operation with over 300 full-time employees and 40 million+ users around the world. It continues to maintain and steward many of the open-source tools used daily in data science, such as conda, pandas, NumPy, and more.
Anaconda is not just a company — it’s a movement. Its tools underpin key innovations at companies like Microsoft, Oracle, and IBM, and power integrations like Python in Excel and Snowflake’s Snowpark for Python.
“We are — and always will be — committed to fostering open-source innovation,” says Wang. “Our job is to make open source enterprise-ready so that innovation isn’t slowed down by complexity, risk, or compliance barriers.”
A Future-Proof Platform for AI at Scale
The Anaconda AI Platform is available now and can be deployed across public cloud, private cloud, sovereign cloud, and on-premise environments. It’s also listed on AWS Marketplace for seamless procurement and enterprise integration.
In a world where speed, trust, and scale are paramount, Anaconda has redefined what’s possible for open-source AI — not just for individual developers, but for the enterprises that depend on them.
#000#access control#adoption#ai#ai assistant#AI development#ai platform#amp#anaconda#Analytics#approach#Artificial Intelligence#audit#automation#AWS#barrier#Business#catalyst#Cloud#Collaboration#command#command-line interface#Community#Companies#complexity#compliance#compromise#consulting#data#data analytics
0 notes
Text
Why AI-Driven Data Workflow Automation is a Game-Changer for Healthcare
The healthcare industry is one of the most complex and data-intensive sectors, dealing with vast amounts of patient records, medical imaging, research data, billing, and administrative processes. Historically, these workflows have been manually managed, leading to inefficiencies, errors, and delays in patient care. AI-driven data workflow automation is transforming healthcare by streamlining processes, enhancing accuracy, and improving overall patient outcomes. In this blog, we will explore how AI-powered automation is revolutionizing healthcare, its benefits, real-world applications, and what the future holds for this groundbreaking technology.
The Need for Automation in Healthcare
Healthcare systems globally are struggling with challenges such as:
Overwhelming administrative burdens
Rising operational costs
Increasing patient expectations for faster and more personalized care
The need for better compliance with regulations such as HIPAA and GDPR
Data silos between hospitals, clinics, and insurance providers
AI-driven workflow automation addresses these challenges by automating repetitive tasks, integrating data sources, and improving decision-making capabilities through predictive analytics.
Key Benefits of AI-Driven Workflow Automation in Healthcare
1. Improved Efficiency and Productivity
AI can automate tasks such as scheduling appointments, processing insurance claims, and managing electronic health records (EHR). This reduces the administrative workload on healthcare staff, allowing them to focus on patient care.
2. Enhanced Accuracy and Reduced Errors
Manual data entry and processing errors can lead to misdiagnoses, incorrect billing, and treatment delays. AI-driven automation minimizes these risks by ensuring accurate data input, processing, and analysis.
3. Faster and More Personalized Patient Care
AI-powered chatbots and virtual assistants can help patients book appointments, receive reminders, and get answers to medical inquiries. Machine learning algorithms analyze patient data to recommend personalized treatment plans.
4. Cost Reduction
By automating repetitive and time-consuming tasks, healthcare organizations can cut labor costs and reduce operational expenses, making medical care more affordable and efficient.
5. Better Compliance and Security
AI-driven solutions ensure that sensitive patient data is handled according to regulatory requirements. Automated workflows can track and report compliance issues in real time, reducing legal risks for healthcare providers.
Applications of AI-Driven Data Workflow Automation in Healthcare
1. Electronic Health Records (EHR) Management
AI-powered automation helps in data extraction, categorization, and updating patient records without human intervention. This ensures real-time access to accurate and up-to-date information for doctors and medical staff.
2. Medical Imaging and Diagnostics
AI-driven image recognition tools can quickly analyze X-rays, MRIs, and CT scans, assisting radiologists in detecting diseases like cancer, fractures, and neurological disorders with high accuracy.
3. Appointment Scheduling and Patient Engagement
AI-powered chatbots and virtual assistants can handle appointment scheduling, send reminders, and answer patient queries, reducing the burden on healthcare staff and improving patient satisfaction.
4. Billing and Insurance Claims Processing
Automation streamlines medical billing by detecting errors, verifying insurance claims, and ensuring compliance with coding standards, leading to faster reimbursements and fewer claim denials.
5. Predictive Analytics for Disease Prevention
Machine learning algorithms analyze patient history, lifestyle, and genetic factors to predict disease risks and recommend preventive measures, helping reduce hospital admissions and improving public health outcomes.
6. Clinical Trials and Research
AI accelerates clinical trials by automating data collection, patient recruitment, and analysis of medical research, leading to faster drug development and approvals.
7. Telemedicine and Remote Patient Monitoring
AI-powered automation enables remote patient monitoring through wearable devices that collect and analyze health data in real time, allowing doctors to intervene before conditions worsen.
Real-Life Case Studies in AI-Driven Healthcare Automation
Case Study 1: Mayo Clinic – AI for Early Disease Detection
The Mayo Clinic, a renowned medical research center, has integrated AI-driven automation into its workflow to enhance early disease detection. One notable example is its use of AI-powered imaging analysis to detect heart disease and certain types of cancer earlier than traditional methods. By leveraging machine learning algorithms, Mayo Clinic's AI systems analyze thousands of medical images to identify patterns that may indicate disease, helping doctors make more accurate diagnoses faster. This AI-driven approach has significantly reduced diagnostic errors and improved patient outcomes, demonstrating the power of AI in predictive medicine.
Case Study 2: Mount Sinai Health System – AI-Powered Radiology Workflow
Mount Sinai Health System in New York has implemented AI automation in its radiology department to streamline image analysis and reporting. The hospital's AI-driven workflow automates the sorting and prioritization of medical images, allowing radiologists to focus on the most urgent cases first. By analyzing CT scans, MRIs, and X-rays in real time, the AI system flags abnormalities such as tumors, fractures, and internal bleeding, expediting the diagnostic process. This has reduced patient wait times and improved treatment planning, showcasing how AI can enhance efficiency in high-demand medical environments.
Challenges and Considerations
Despite its advantages, AI-driven workflow automation in healthcare comes with challenges:
Data Privacy and Security: Ensuring that AI systems comply with HIPAA, GDPR, and other regulations is crucial to protecting patient data.
Integration with Legacy Systems: Many hospitals still use outdated systems that may not easily integrate with AI-powered solutions.
Trust and Adoption: Healthcare professionals may be skeptical of AI-based decision-making and need proper training to understand its benefits.
Cost of Implementation: While AI-driven automation leads to cost savings in the long run, the initial investment can be high for smaller healthcare providers.
The Future of AI-Driven Workflow Automation in Healthcare
The future of AI in healthcare looks promising, with advancements such as:
AI-powered robotics for surgeries and rehabilitation – Surgical robots are becoming more precise, allowing for minimally invasive procedures with faster recovery times. AI-driven robotic-assisted surgeries will enhance accuracy and reduce risks.
Natural Language Processing (NLP) for better doctor-patient communication – AI-powered NLP will improve how healthcare providers interact with patients, translating complex medical jargon into simple terms and enabling voice-driven medical documentation.
Blockchain for secure and decentralized patient data management – AI and blockchain will combine to ensure patient records are secure, tamper-proof, and easily accessible across healthcare institutions.
AI-driven drug discovery and personalized medicine – AI is accelerating drug development by analyzing vast datasets to identify potential treatments and tailoring medications to individual genetic profiles.
AI-integrated wearable technology for proactive healthcare – Smart wearables with AI capabilities will track vital signs, detect anomalies, and alert healthcare providers before health issues escalate.
AI-driven mental health support – AI-powered chatbots and virtual therapists will assist in detecting and managing mental health conditions, making mental healthcare more accessible.
AI in emergency and disaster response – AI will play a crucial role in detecting disease outbreaks, optimizing hospital responses, and assisting in large-scale disaster management efforts.
As AI continues to evolve, its impact on healthcare will only grow, making medical services more efficient, accurate, and accessible.
AI-driven data workflow automation is undeniably a game-changer for the healthcare industry. By improving efficiency, reducing costs, and enhancing patient care, AI is paving the way for a smarter, data-driven healthcare ecosystem. While challenges remain, ongoing advancements and regulatory compliance measures will ensure that AI-powered automation becomes a standard practice in healthcare. The integration of AI-driven automation is the future of modern healthcare.
Learn more about DataPeak:
#datapeak#factr#saas#technology#agentic ai#artificial intelligence#machine learning#ai#ai-driven business solutions#machine learning for workflow#ai solutions for data driven decision making#ai business tools#artificialintelligence#aiinnovation#digital trends#digital technology#digitaltools#datadrivendecisions#dataanalytics#data driven decision making#data analytics#workflowautomation#best ai tools for data pipeline automation#ai platform for business process automation#ai driven data workflow automation#healthcare#ai healthcare
0 notes
Text
How does AI integrate with CRM systems for live sales forecasting?
AI integration with CRM systems enhances live sales forecasting by analyzing real-time data, identifying trends, and predicting customer behavior. This improves accuracy and decision-making. EPM software like BiCXO leverages AI to optimize sales strategies, driving growth and profitability.
#business solutions#bisolution#business intelligence software#data#businessefficiency#bi tool#business intelligence#businessintelligence#bicxo#data warehouse#crm software#crm services#crm solutions#crm platform#automation#India#USA#sales automation#software
1 note
·
View note
Text
Quick Thoughts on Microsoft P⚙️wer Aut⚙️mate: A Review
Microsoft Power Automate and AI tools like ChatGPT and Claude are reshaping productivity. Dive into this practical review for insights and real-world examples!
What’s On My Mind Today? Automation has become more than a buzzword—it’s a necessity. Microsoft Power Automate is a standout tool in this landscape, offering a way to design workflows that not only boost productivity but also serve as a foundation for more advanced automation down the line. After a brief dive into its interface and features, I’m struck by both its potential and its challenges.…
#AI tools#automation workflows#ChatGPT#Claude#creative automation#cross-platform synergy#dynamic workflows#enhanced collaboration#Ethical AI#Gemini#Microsoft AI#Microsoft Power Automate#Power Automate AI#productivity tools#scaling intelligence#Workflow Automation
0 notes
Text
Custom AWS Solutions for Modern Enterprises - Atcuality
Amazon Web Services offer an unparalleled ecosystem of cloud computing tools that cater to businesses of all sizes. At ATCuality, we understand that no two companies are the same, which is why we provide custom Amazon Web Services solutions tailored to your specific goals. From designing scalable architectures to implementing cutting-edge machine learning capabilities, our AWS services ensure that your business stays ahead of the curve. The flexibility of Amazon Web Services allows for easy integration with your existing systems, paving the way for seamless growth and enhanced efficiency. Let us help you harness the power of AWS for your enterprise.
#seo marketing#seo services#artificial intelligence#digital marketing#seo agency#iot applications#seo company#ai powered application#azure cloud services#amazon web services#virtual reality#augmented reality agency#augmented human c4 621#augmented and virtual reality market#augmented reality#augmented intelligence#digital services#iotsolutions#iot development services#iot platform#techinnovation#digitaltransformation#automation#iot#iot solutions#iot development company#innovation#erp software#erp system#cloud security services
0 notes