#data processing automation
Explore tagged Tumblr posts
Text
How to Use n8n and AI to Build an Automation System
Automation is changing how we work every day. It helps save time, reduce mistakes, and get more done with less effort. If you want to automate your tasks but don’t know where to start, this guide is for you. In this post, you will learn how to use n8n — a free, open-source automation tool — combined with AI to build smart workflows that do work for you. What Is n8n? n8n (pronounced…
#AI automation#AI integration#AI workflow#AI-powered workflows#API integration#artificial intelligence tools#automate emails#automate tasks#automation platform#automation software#automation system#automation tips#business automation#chatbot automation#data processing automation#email automation#intelligent automation#low-code automation#n8n automation#no-code automation#open source automation#productivity tools#smart automation#time-saving tools#workflow automation#workflow builder
0 notes
Text
i hate gen AI so much i wish crab raves upon it
#genuinely this shit is like downfall of humanity to me#what do you mean you have a compsci degree and are having chatgpt write basic code for you#what do you mean you are using it to come up with recipes#what do you mean you are talking to it 24/7 like it’s your friend#what do you mean you are RPing with it#what do you mean you use it instead of researching anything for yourself#what do you mean you’re using it to write your essays instead of just writing your essays#i feel crazy i feel insane on god on GOD#i would have gotten a different degree if i knew that half the jobs that exist now for my degree are all feeding into the fucking gen AI#slop machine#what’s worse is my work experience is very much ‘automation engineering’ which is NOT AI but#using coding/technology/databases to improve existing processes and make them easier and less tedious for people#to free them up to do things that involve more brainpower than tedious data entry/etc#SO ESPECIALLY so many of the jobs i would have been able to take with my work experience is now very gen AI shit and i just refuse to fuckin#do that shit?????
2 notes
·
View notes
Text
he literally just said on a rally (why is he even doing them still wtf) that he wants to bring the economy back to 1929 we're all so fucking screwed.... we're so fucking screwed
the social stuff can be mitigated... this can't, we're so screwed globally :|
#personal#i guess im not even thinking about job hopping for higher pay this year anymore lol#i'll eat other people's jobs with my automation scripts so that i'm not the layoff#i'm already getting added to the job eater team once im back from vacation.... cause i know some python and the job eating#software uses python for api data requests#like i'm the artist they chose to put on the /automation job eating/ team lol#like im literally the ONLY artist not in a true managerial role put on this team cause i can code a little...and translate to cs nerd#(and all the scripts that i've made at work are just adaptations of my gif automation process... so if#weird boyband special interest mixed with hypernumeracy type autism saves me and my husband from this stupidity#i'll be annoyed but grateful)
4 notes
·
View notes
Text
With Innrly | Streamline Your Hospitality Operations
Manage all your hotels from anywhere | Transformation without transition
Managing a hotel or a multi-brand portfolio can be overwhelming, especially when juggling multiple systems, reports, and data sources. INNRLY, a cutting-edge hotel management software, revolutionizes the way hospitality businesses operate by delivering intelligent insights and simplifying workflows—all without the need for system changes or upgrades. Designed for seamless integration and powerful automation, INNRLY empowers hotel owners and managers to make data-driven decisions and enhance operational efficiency.
Revolutionizing Hotel Management
In the fast-paced world of hospitality, efficiency is the cornerstone of success. INNRLY’s cloud-based platform offers a brand-neutral, user-friendly interface that consolidates critical business data across all your properties. Whether you manage a single boutique hotel or a portfolio of properties spanning different regions, INNRLY provides an all-in-one solution for optimizing performance and boosting productivity.
One Dashboard for All Your Properties:
Say goodbye to fragmented data and manual processes. INNRLY enables you to monitor your entire portfolio from a single dashboard, providing instant access to key metrics like revenue, occupancy, labor costs, and guest satisfaction. With this unified view, hotel managers can make informed decisions in real time.
Customizable and Scalable Solutions:
No two hospitality businesses are alike, and INNRLY understands that. Its customizable features adapt to your unique needs, whether you're running a small chain or managing an extensive enterprise. INNRLY grows with your business, ensuring that your operations remain efficient and effective.
Seamless Integration for Effortless Operations:
One of INNRLY’s standout features is its ability to integrate seamlessly with your existing systems. Whether it's your property management system (PMS), accounting software, payroll/labor management tools, or even guest feedback platforms, INNRLY pulls data together effortlessly, eliminating the need for system overhauls.
Automated Night Audits:
Tired of labor-intensive night audits? INNRLY’s Night Audit+ automates this crucial process, providing detailed reports that are automatically synced with your accounting software. It identifies issues such as declined credit cards or high balances, ensuring no problem goes unnoticed.
A/R and A/P Optimization:
Streamline your accounts receivable (A/R) and accounts payable (A/P) processes to improve cash flow and avoid costly mistakes. INNRLY’s automation reduces manual entry, speeding up credit cycles and ensuring accurate payments.
Labor and Cost Management:
With INNRLY, you can pinpoint inefficiencies, monitor labor hours, and reduce costs. Detailed insights into overtime risks, housekeeping minutes per room (MPR), and other labor metrics help you manage staff productivity effectively.
Empowering Data-Driven Decisions:
INNRLY simplifies decision-making by surfacing actionable insights through its robust reporting and analytics tools.
Comprehensive Reporting:
Access reports on your schedule, from detailed night audit summaries to trial balances and franchise billing reconciliations. Consolidated data across multiple properties allows for easy performance comparisons and trend analysis.
Benchmarking for Success:
Compare your properties' performance against industry standards or other hotels in your portfolio. Metrics such as ADR (Average Daily Rate), RevPAR (Revenue Per Available Room), and occupancy rates are presented in an easy-to-understand format, empowering you to identify strengths and areas for improvement.
Guest Satisfaction Insights:
INNRLY compiles guest feedback and satisfaction scores, enabling you to take prompt action to enhance the guest experience. Happy guests lead to better reviews and increased bookings, driving long-term success.
Key Benefits of INNRLY
Single Login, Full Control: Manage all properties with one login, saving time and reducing complexity.
Error-Free Automation: Eliminate manual data entry, reducing errors and increasing productivity.
Cost Savings: Pinpoint problem areas to reduce labor costs and optimize spending.
Enhanced Accountability: Hold each property accountable for issues flagged by INNRLY’s tools, supported by an optional Cash Flow Protection Team at the enterprise level.
Data Security: Protect your credentials and data while maintaining your existing systems.
Transforming Hospitality Without Transition
INNRLY’s philosophy is simple: transformation without transition. You don’t need to replace or upgrade your existing systems to benefit from INNRLY. The software integrates effortlessly into your current setup, allowing you to focus on what matters most—delivering exceptional guest experiences and achieving your business goals.
Who Can Benefit from INNRLY?
Hotel Owners:
For owners managing multiple properties, INNRLY offers a centralized platform to monitor performance, identify inefficiencies, and maximize profitability.
General Managers:
Simplify day-to-day operations with automated processes and real-time insights, freeing up time to focus on strategic initiatives.
Accounting Teams:
INNRLY ensures accurate financial reporting by syncing data across systems, reducing errors, and streamlining reconciliation processes.
Multi-Brand Portfolios:
For operators managing properties across different brands, INNRLY’s brand-neutral platform consolidates data, making it easy to compare and optimize performance.
Contact INNRLY Today
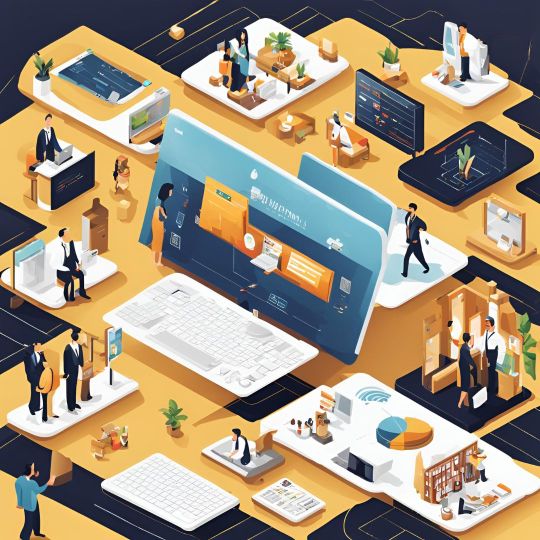
Ready to revolutionize your hotel management? Join the growing number of hospitality businesses transforming their operations with INNRLY.
Website: www.innrly.com
Email: [email protected]
Phone: 833-311-0777
#Innrly#Innrly Hotel Management Software#Bank Integrations in Hospitality Software#Tracking Hotel Compliance#hotel performance software#hotel portfolio software#Hotel Performance Management Software#hotel reconciliation software#Hotel Data Entry Software#accounting software hotels#hotel banking software#hospitality automated accounting software#hotel automation software hotel bookkeeping software#back office hotel accounting software#hospitality back office software#accounting hospitality software#Hotel Management Accounting Software#Hotel Accounting Software#Hospitality Accounting Software#Accounting Software for Hotels#Hotel Budgeting Software#Automate Night Audit Software#Automate Night Audit Process#Best Hotel Accounting Software#Best Accounting Software For Hotels#Financial & Hotel Accounting Software#Hospitality Accounting Solutions
2 notes
·
View notes
Text
How Large Language Models (LLMs) are Transforming Data Cleaning in 2024
Data is the new oil, and just like crude oil, it needs refining before it can be utilized effectively. Data cleaning, a crucial part of data preprocessing, is one of the most time-consuming and tedious tasks in data analytics. With the advent of Artificial Intelligence, particularly Large Language Models (LLMs), the landscape of data cleaning has started to shift dramatically. This blog delves into how LLMs are revolutionizing data cleaning in 2024 and what this means for businesses and data scientists.
The Growing Importance of Data Cleaning
Data cleaning involves identifying and rectifying errors, missing values, outliers, duplicates, and inconsistencies within datasets to ensure that data is accurate and usable. This step can take up to 80% of a data scientist's time. Inaccurate data can lead to flawed analysis, costing businesses both time and money. Hence, automating the data cleaning process without compromising data quality is essential. This is where LLMs come into play.
What are Large Language Models (LLMs)?
LLMs, like OpenAI's GPT-4 and Google's BERT, are deep learning models that have been trained on vast amounts of text data. These models are capable of understanding and generating human-like text, answering complex queries, and even writing code. With millions (sometimes billions) of parameters, LLMs can capture context, semantics, and nuances from data, making them ideal candidates for tasks beyond text generation—such as data cleaning.
To see how LLMs are also transforming other domains, like Business Intelligence (BI) and Analytics, check out our blog How LLMs are Transforming Business Intelligence (BI) and Analytics.

Traditional Data Cleaning Methods vs. LLM-Driven Approaches
Traditionally, data cleaning has relied heavily on rule-based systems and manual intervention. Common methods include:
Handling missing values: Methods like mean imputation or simply removing rows with missing data are used.
Detecting outliers: Outliers are identified using statistical methods, such as standard deviation or the Interquartile Range (IQR).
Deduplication: Exact or fuzzy matching algorithms identify and remove duplicates in datasets.
However, these traditional approaches come with significant limitations. For instance, rule-based systems often fail when dealing with unstructured data or context-specific errors. They also require constant updates to account for new data patterns.
LLM-driven approaches offer a more dynamic, context-aware solution to these problems.

How LLMs are Transforming Data Cleaning
1. Understanding Contextual Data Anomalies
LLMs excel in natural language understanding, which allows them to detect context-specific anomalies that rule-based systems might overlook. For example, an LLM can be trained to recognize that “N/A” in a field might mean "Not Available" in some contexts and "Not Applicable" in others. This contextual awareness ensures that data anomalies are corrected more accurately.
2. Data Imputation Using Natural Language Understanding
Missing data is one of the most common issues in data cleaning. LLMs, thanks to their vast training on text data, can fill in missing data points intelligently. For example, if a dataset contains customer reviews with missing ratings, an LLM could predict the likely rating based on the review's sentiment and content.
A recent study conducted by researchers at MIT (2023) demonstrated that LLMs could improve imputation accuracy by up to 30% compared to traditional statistical methods. These models were trained to understand patterns in missing data and generate contextually accurate predictions, which proved to be especially useful in cases where human oversight was traditionally required.
3. Automating Deduplication and Data Normalization
LLMs can handle text-based duplication much more effectively than traditional fuzzy matching algorithms. Since these models understand the nuances of language, they can identify duplicate entries even when the text is not an exact match. For example, consider two entries: "Apple Inc." and "Apple Incorporated." Traditional algorithms might not catch this as a duplicate, but an LLM can easily detect that both refer to the same entity.
Similarly, data normalization—ensuring that data is formatted uniformly across a dataset—can be automated with LLMs. These models can normalize everything from addresses to company names based on their understanding of common patterns and formats.
4. Handling Unstructured Data
One of the greatest strengths of LLMs is their ability to work with unstructured data, which is often neglected in traditional data cleaning processes. While rule-based systems struggle to clean unstructured text, such as customer feedback or social media comments, LLMs excel in this domain. For instance, they can classify, summarize, and extract insights from large volumes of unstructured text, converting it into a more analyzable format.
For businesses dealing with social media data, LLMs can be used to clean and organize comments by detecting sentiment, identifying spam or irrelevant information, and removing outliers from the dataset. This is an area where LLMs offer significant advantages over traditional data cleaning methods.
For those interested in leveraging both LLMs and DevOps for data cleaning, see our blog Leveraging LLMs and DevOps for Effective Data Cleaning: A Modern Approach.

Real-World Applications
1. Healthcare Sector
Data quality in healthcare is critical for effective treatment, patient safety, and research. LLMs have proven useful in cleaning messy medical data such as patient records, diagnostic reports, and treatment plans. For example, the use of LLMs has enabled hospitals to automate the cleaning of Electronic Health Records (EHRs) by understanding the medical context of missing or inconsistent information.
2. Financial Services
Financial institutions deal with massive datasets, ranging from customer transactions to market data. In the past, cleaning this data required extensive manual work and rule-based algorithms that often missed nuances. LLMs can assist in identifying fraudulent transactions, cleaning duplicate financial records, and even predicting market movements by analyzing unstructured market reports or news articles.
3. E-commerce
In e-commerce, product listings often contain inconsistent data due to manual entry or differing data formats across platforms. LLMs are helping e-commerce giants like Amazon clean and standardize product data more efficiently by detecting duplicates and filling in missing information based on customer reviews or product descriptions.

Challenges and Limitations
While LLMs have shown significant potential in data cleaning, they are not without challenges.
Training Data Quality: The effectiveness of an LLM depends on the quality of the data it was trained on. Poorly trained models might perpetuate errors in data cleaning.
Resource-Intensive: LLMs require substantial computational resources to function, which can be a limitation for small to medium-sized enterprises.
Data Privacy: Since LLMs are often cloud-based, using them to clean sensitive datasets, such as financial or healthcare data, raises concerns about data privacy and security.

The Future of Data Cleaning with LLMs
The advancements in LLMs represent a paradigm shift in how data cleaning will be conducted moving forward. As these models become more efficient and accessible, businesses will increasingly rely on them to automate data preprocessing tasks. We can expect further improvements in imputation techniques, anomaly detection, and the handling of unstructured data, all driven by the power of LLMs.
By integrating LLMs into data pipelines, organizations can not only save time but also improve the accuracy and reliability of their data, resulting in more informed decision-making and enhanced business outcomes. As we move further into 2024, the role of LLMs in data cleaning is set to expand, making this an exciting space to watch.
Large Language Models are poised to revolutionize the field of data cleaning by automating and enhancing key processes. Their ability to understand context, handle unstructured data, and perform intelligent imputation offers a glimpse into the future of data preprocessing. While challenges remain, the potential benefits of LLMs in transforming data cleaning processes are undeniable, and businesses that harness this technology are likely to gain a competitive edge in the era of big data.
#Artificial Intelligence#Machine Learning#Data Preprocessing#Data Quality#Natural Language Processing#Business Intelligence#Data Analytics#automation#datascience#datacleaning#large language model#ai
2 notes
·
View notes
Text
I need to pluck Trin and Vari out from my brain and get them helping me with this analysis :(
#stuff#it's 100% my own foolish fault#my move from excel to google sheets means that certain survey data analysis processes that I used to automate are now manual#aha. eyes hurty
2 notes
·
View notes
Text
Why AI-Driven Data Workflow Automation is a Game-Changer for Healthcare
The healthcare industry is one of the most complex and data-intensive sectors, dealing with vast amounts of patient records, medical imaging, research data, billing, and administrative processes. Historically, these workflows have been manually managed, leading to inefficiencies, errors, and delays in patient care. AI-driven data workflow automation is transforming healthcare by streamlining processes, enhancing accuracy, and improving overall patient outcomes. In this blog, we will explore how AI-powered automation is revolutionizing healthcare, its benefits, real-world applications, and what the future holds for this groundbreaking technology.
The Need for Automation in Healthcare
Healthcare systems globally are struggling with challenges such as:
Overwhelming administrative burdens
Rising operational costs
Increasing patient expectations for faster and more personalized care
The need for better compliance with regulations such as HIPAA and GDPR
Data silos between hospitals, clinics, and insurance providers
AI-driven workflow automation addresses these challenges by automating repetitive tasks, integrating data sources, and improving decision-making capabilities through predictive analytics.
Key Benefits of AI-Driven Workflow Automation in Healthcare
1. Improved Efficiency and Productivity
AI can automate tasks such as scheduling appointments, processing insurance claims, and managing electronic health records (EHR). This reduces the administrative workload on healthcare staff, allowing them to focus on patient care.
2. Enhanced Accuracy and Reduced Errors
Manual data entry and processing errors can lead to misdiagnoses, incorrect billing, and treatment delays. AI-driven automation minimizes these risks by ensuring accurate data input, processing, and analysis.
3. Faster and More Personalized Patient Care
AI-powered chatbots and virtual assistants can help patients book appointments, receive reminders, and get answers to medical inquiries. Machine learning algorithms analyze patient data to recommend personalized treatment plans.
4. Cost Reduction
By automating repetitive and time-consuming tasks, healthcare organizations can cut labor costs and reduce operational expenses, making medical care more affordable and efficient.
5. Better Compliance and Security
AI-driven solutions ensure that sensitive patient data is handled according to regulatory requirements. Automated workflows can track and report compliance issues in real time, reducing legal risks for healthcare providers.
Applications of AI-Driven Data Workflow Automation in Healthcare
1. Electronic Health Records (EHR) Management
AI-powered automation helps in data extraction, categorization, and updating patient records without human intervention. This ensures real-time access to accurate and up-to-date information for doctors and medical staff.
2. Medical Imaging and Diagnostics
AI-driven image recognition tools can quickly analyze X-rays, MRIs, and CT scans, assisting radiologists in detecting diseases like cancer, fractures, and neurological disorders with high accuracy.
3. Appointment Scheduling and Patient Engagement
AI-powered chatbots and virtual assistants can handle appointment scheduling, send reminders, and answer patient queries, reducing the burden on healthcare staff and improving patient satisfaction.
4. Billing and Insurance Claims Processing
Automation streamlines medical billing by detecting errors, verifying insurance claims, and ensuring compliance with coding standards, leading to faster reimbursements and fewer claim denials.
5. Predictive Analytics for Disease Prevention
Machine learning algorithms analyze patient history, lifestyle, and genetic factors to predict disease risks and recommend preventive measures, helping reduce hospital admissions and improving public health outcomes.
6. Clinical Trials and Research
AI accelerates clinical trials by automating data collection, patient recruitment, and analysis of medical research, leading to faster drug development and approvals.
7. Telemedicine and Remote Patient Monitoring
AI-powered automation enables remote patient monitoring through wearable devices that collect and analyze health data in real time, allowing doctors to intervene before conditions worsen.
Real-Life Case Studies in AI-Driven Healthcare Automation
Case Study 1: Mayo Clinic – AI for Early Disease Detection
The Mayo Clinic, a renowned medical research center, has integrated AI-driven automation into its workflow to enhance early disease detection. One notable example is its use of AI-powered imaging analysis to detect heart disease and certain types of cancer earlier than traditional methods. By leveraging machine learning algorithms, Mayo Clinic's AI systems analyze thousands of medical images to identify patterns that may indicate disease, helping doctors make more accurate diagnoses faster. This AI-driven approach has significantly reduced diagnostic errors and improved patient outcomes, demonstrating the power of AI in predictive medicine.
Case Study 2: Mount Sinai Health System – AI-Powered Radiology Workflow
Mount Sinai Health System in New York has implemented AI automation in its radiology department to streamline image analysis and reporting. The hospital's AI-driven workflow automates the sorting and prioritization of medical images, allowing radiologists to focus on the most urgent cases first. By analyzing CT scans, MRIs, and X-rays in real time, the AI system flags abnormalities such as tumors, fractures, and internal bleeding, expediting the diagnostic process. This has reduced patient wait times and improved treatment planning, showcasing how AI can enhance efficiency in high-demand medical environments.
Challenges and Considerations
Despite its advantages, AI-driven workflow automation in healthcare comes with challenges:
Data Privacy and Security: Ensuring that AI systems comply with HIPAA, GDPR, and other regulations is crucial to protecting patient data.
Integration with Legacy Systems: Many hospitals still use outdated systems that may not easily integrate with AI-powered solutions.
Trust and Adoption: Healthcare professionals may be skeptical of AI-based decision-making and need proper training to understand its benefits.
Cost of Implementation: While AI-driven automation leads to cost savings in the long run, the initial investment can be high for smaller healthcare providers.
The Future of AI-Driven Workflow Automation in Healthcare
The future of AI in healthcare looks promising, with advancements such as:
AI-powered robotics for surgeries and rehabilitation – Surgical robots are becoming more precise, allowing for minimally invasive procedures with faster recovery times. AI-driven robotic-assisted surgeries will enhance accuracy and reduce risks.
Natural Language Processing (NLP) for better doctor-patient communication – AI-powered NLP will improve how healthcare providers interact with patients, translating complex medical jargon into simple terms and enabling voice-driven medical documentation.
Blockchain for secure and decentralized patient data management – AI and blockchain will combine to ensure patient records are secure, tamper-proof, and easily accessible across healthcare institutions.
AI-driven drug discovery and personalized medicine – AI is accelerating drug development by analyzing vast datasets to identify potential treatments and tailoring medications to individual genetic profiles.
AI-integrated wearable technology for proactive healthcare – Smart wearables with AI capabilities will track vital signs, detect anomalies, and alert healthcare providers before health issues escalate.
AI-driven mental health support – AI-powered chatbots and virtual therapists will assist in detecting and managing mental health conditions, making mental healthcare more accessible.
AI in emergency and disaster response – AI will play a crucial role in detecting disease outbreaks, optimizing hospital responses, and assisting in large-scale disaster management efforts.
As AI continues to evolve, its impact on healthcare will only grow, making medical services more efficient, accurate, and accessible.
AI-driven data workflow automation is undeniably a game-changer for the healthcare industry. By improving efficiency, reducing costs, and enhancing patient care, AI is paving the way for a smarter, data-driven healthcare ecosystem. While challenges remain, ongoing advancements and regulatory compliance measures will ensure that AI-powered automation becomes a standard practice in healthcare. The integration of AI-driven automation is the future of modern healthcare.
Learn more about DataPeak:
#datapeak#factr#saas#technology#agentic ai#artificial intelligence#machine learning#ai#ai-driven business solutions#machine learning for workflow#ai solutions for data driven decision making#ai business tools#artificialintelligence#aiinnovation#digital trends#digital technology#digitaltools#datadrivendecisions#dataanalytics#data driven decision making#data analytics#workflowautomation#best ai tools for data pipeline automation#ai platform for business process automation#ai driven data workflow automation#healthcare#ai healthcare
0 notes
Text
0 notes
Text
How to Automate Document Processing for Your Business: A Step-by-Step Guide
Managing documents manually is one of the biggest time drains in business today. From processing invoices and contracts to organizing customer forms, these repetitive tasks eat up hours every week. The good news? Automating document processing is simpler (and more affordable) than you might think.
In this easy-to-follow guide, we’ll show you step-by-step how to automate document processing in your business—saving you time, reducing errors, and boosting productivity.
What You’ll Need
A scanner (if you still have paper documents)
A document processing software (like AppleTechSoft’s Document Processing Solution)
Access to your business’s document workflows (invoices, forms, receipts, etc.)
Step 1: Identify Documents You Want to Automate
Start by making a list of documents that take up the most time to process. Common examples include:
Invoices and bills
Purchase orders
Customer application forms
Contracts and agreements
Expense receipts
Tip: Prioritize documents that are repetitive and high volume.
Step 2: Digitize Your Paper Documents
If you’re still handling paper, scan your documents into digital formats (PDF, JPEG, etc.). Most modern document processing tools work best with digital files.
Quick Tip: Use high-resolution scans (300 DPI or more) for accurate data extraction.
Step 3: Choose a Document Processing Tool
Look for a platform that offers:
OCR (Optical Character Recognition) to extract text from scanned images
AI-powered data extraction to capture key fields like dates, names, and totals
Integration with your accounting software, CRM, or database
Security and compliance features to protect sensitive data
AppleTechSoft’s Document Processing Solution ticks all these boxes and more.
Step 4: Define Your Workflow Rules
Tell your software what you want it to do with your documents. For example:
Extract vendor name, date, and amount from invoices
Automatically save contracts to a shared folder
Send expense reports directly to accounting
Most tools offer an easy drag-and-drop interface or templates to set these rules up.
Step 5: Test Your Automation
Before going live, test the workflow with sample documents. Check if:
Data is extracted accurately
Documents are routed to the right folders or apps
Any errors or mismatches are flagged
Tweak your settings as needed.
Step 6: Go Live and Monitor
Once you’re confident in your workflow, deploy it for daily use. Monitor the automation for the first few weeks to ensure it works as expected.
Pro Tip: Set up alerts for any failed extractions or mismatches so you can quickly correct issues.
Bonus Tips for Success
Regularly update your templates as your document formats change
Train your team on how to upload and manage documents in the system
Schedule periodic reviews to optimize and improve your workflows
Conclusion
Automating document processing can transform your business operations—from faster invoicing to smoother customer onboarding. With the right tools and a clear plan, you can streamline your paperwork and focus on what matters most: growing your business.
Ready to get started? Contact AppleTechSoft today to explore our Document Processing solutions.
#document processing#business automation#workflow automation#AI tools#paperless office#small business tips#productivity hacks#digital transformation#AppleTechSoft#business technology#OCR software#data extraction#invoicing automation#business growth#time saving tips
1 note
·
View note
Text
Vision in Focus: The Art and Science of Computer Vision & Image Processing.
Sanjay Kumar Mohindroo Sanjay Kumar Mohindroo. skm.stayingalive.in An insightful blog post on computer vision and image processing, highlighting its impact on medical diagnostics, autonomous driving, and security systems.
Computer vision and image processing have reshaped the way we see and interact with the world. These fields power systems that read images, detect objects and analyze video…
#AI#Automated Image Recognition#Autonomous Driving#Collaboration#Community#Computer Vision#data#Discussion#Future Tech#Health Tech#Image Processing#Innovation#Medical Diagnostics#News#Object Detection#Privacy#Sanjay Kumar Mohindroo#Security Systems#Tech Ethics#tech innovation#Video Analysis
0 notes
Text
oof i just realized since i have a newer phone now and outlook app works on it, not only can i work on teams off my wrist, but i can do EMAILS off my wrist
#tbh i automated around like... 50% of my job away#i mean i still have to check the artwork and stuff it's not like my scripties can do my job for me#nor can my datamerge sets or my like.... resize one art.. automatically resizes all other garment size templates#and when i wfh i let the computer run and answer messages and texts on my phone#but now i don't even have to run over when i get an email!!!!!!!!!!!!!!!!!!!#my boss saw me do it a few times and i taught a few ppl in my dept my like... .lazy girl automation#AND he asked how i knew the things and i was like... oh no reason like i know this for no reason#until like i was there over a year..... and i was like UHHH i was REALLY into a kpop boyband with 9 members and wanted to make GIFS#for ALL NINE BOYS!! every performance... sometimes 2 perfs a day which is 4 x 9 x 2 gifs LOL#he looked at me like i was weird but i also sit in between the bts cubicle and the exo cubicle#i only have work stuff pinned up on my cube lol#BUT if you guys didn't know all my gifs are batch processed.... so i only do about half the work#i have a script to copy layers to all open documents which helps with coloring and watermarks#and then also.... a BUNCH of batch processes... like all i do is import crop and do base coloring#everything else my computer just runs for me now LMAO#personal#if i don't get a good raise this year... we're going to be implementing one of my data merge things for templates for a LOT of the pitch#boards and pages for sales................... SOOoooOOoO i'll sneak that shit into my portfolio and apply elsewhere to get a job hop bump#but i should get a good review lol
3 notes
·
View notes
Text
AI-Powered Business Analytics: Make Smarter Decisions, Faster
AI-Powered Business Analytics Make Smarter Decisions, Faster 💡 AI-powered analytics give you instant insights into what’s working and what’s not. Learn how to use AI to optimize business decisions. The Problem: Are You Guessing or Growing? Let’s be real—making business decisions based on gut feelings is like throwing darts blindfolded. Sure, you might hit the target occasionally, but most of…
#AI automation for business#AI business analytics#AI business optimization tools#AI customer insights#AI data analysis#AI data visualization#AI for advertising performance#AI for audience segmentation#AI for business intelligence#AI for business scalability#AI for customer retention#AI for customer segmentation#AI for demand prediction#AI for eCommerce analytics#AI for financial forecasting#AI for operational decision-making#AI for process optimization#AI for revenue optimization#AI for ROI maximization#AI for sales forecasting#AI for sales optimization#AI for small business growth#AI in corporate decision-making#AI in digital marketing#AI in marketing analytics#AI operational efficiency#AI performance tracking#AI predictive analytics#AI-driven business strategy#AI-driven competitive analysis
0 notes
Text

Satellite Data Processing and Data Analytics with AI-Based Intelligence Solutions.
APRIL (Advanced Pixel Research & Intelligence Lab), Azista's strategic business unit, uses state-of-the-art AI/ML algorithms to meet customer requirements for data processing and analytics. Our earth imaging satellites are capable of supporting various critical applications for civilian and defence purposes. our satellite imagery can help in agriculture, environment, transportation, mining, forestry, navigation, disaster management, urban planning and other government agencies.
APRIL specialises in satellite data processing and data analytics. APRIL works with diverse datasets to deliver actionable intelligence to strategic users and has developed proprietary software in accordance with DIPAC guidelines. Additionally, APRIL offers timely intelligence reports over specified Areas of Interest (AoI).
Our first satellite, "AFR," on June 13th, 2023. AFR is a wide-swath medium-resolution remote sensing satellite with various use cases for civilian and defence purposes.
For more information, contact us : https://www.april.azista.space/
#afr satellite#satellite data analytics#satellite imagery analytics#satellite image analytics#satellite image analysis system#test and automation solution#signal processing company
1 note
·
View note
Text
How ERP Systems Streamline Processes and Boost Efficiency
In today’s swiftly changing business environment, organisations are under increasing pressure to streamline their processes, increase their efficiency, and maintain their competitiveness. Enterprise Resource Planning (ERP) systems have emerged as a game-changer, radically altering how businesses function. This article will examine the critical role that ERP System plays in streamlining business…
#Business Automation#Centralized Data#Data Integration in ERP#Enterprise Resource Planning#ERP Benefits#ERP Collaboration#ERP for Efficiency#ERP Systems#Improving Business Operations#Productivity Boost with ERP#Streamlining Business Processes
0 notes
Text
Real-World Applications of AI in Data Analytics Across Industries
Artificial Intelligence (AI) has transformed the way businesses leverage data analytics, enabling organizations to gain deeper insights, improve decision-making, and enhance operational efficiency. Across various industries, AI-driven data analytics is revolutionizing processes, uncovering hidden patterns, and driving innovation. This article explores the real-world applications of AI in data analytics across multiple sectors, demonstrating its profound impact on modern business landscapes.
1. Healthcare: Enhancing Diagnostics and Personalized Medicine
The healthcare industry has significantly benefited from AI-powered data analytics, particularly in diagnostics and personalized medicine. AI-driven algorithms analyze vast datasets from electronic health records (EHRs), medical images, and genomic sequences to detect diseases at an early stage. For example, AI systems are being used to identify cancerous cells in radiology scans with higher accuracy than human radiologists. Additionally, predictive analytics help in forecasting disease outbreaks and patient deterioration, allowing for timely medical interventions. Personalized treatment plans are another crucial application, where AI assesses genetic data and medical history to recommend tailored therapies, improving patient outcomes.
2. Finance: Fraud Detection and Risk Management
In the financial sector, AI-powered data analytics is instrumental in fraud detection and risk management. Traditional fraud detection methods often fail to keep up with sophisticated cyber threats. AI algorithms analyze transaction patterns in real time, identifying anomalies that may indicate fraudulent activities. Machine learning models assess credit risk by evaluating an individual's financial behavior and history, enabling banks to make informed lending decisions. Additionally, AI aids in algorithmic trading, where vast datasets are processed to predict market trends and execute high-frequency trades, optimizing investment portfolios.
3. Retail: Enhancing Customer Experience and Inventory Management
Retail businesses use AI in data analytics to enhance customer experience and optimize inventory management. AI-powered recommendation engines analyze customer preferences, purchase history, and browsing behavior to provide personalized product suggestions, increasing sales and customer satisfaction. Predictive analytics help retailers forecast demand, ensuring optimal stock levels and reducing wastage. AI-driven chatbots and virtual assistants improve customer service by offering instant support and resolving queries efficiently. Furthermore, AI enables dynamic pricing strategies, adjusting product prices based on demand fluctuations, competitor pricing, and customer behavior.
4. Manufacturing: Predictive Maintenance and Supply Chain Optimization
AI-driven data analytics is transforming the manufacturing industry by enabling predictive maintenance and optimizing supply chains. Predictive maintenance uses AI to analyze data from sensors and IoT devices to detect potential equipment failures before they occur, reducing downtime and maintenance costs. In supply chain management, AI assesses historical data, market trends, and weather conditions to optimize logistics, ensuring timely deliveries and cost efficiency. Smart factories integrate AI-powered robotics and automation, enhancing production efficiency and minimizing errors.
5. Marketing: Advanced Customer Insights and Campaign Optimization
In marketing, AI is revolutionizing data analytics by providing advanced customer insights and optimizing campaigns. AI-driven sentiment analysis assesses social media and customer feedback, allowing brands to understand consumer emotions and preferences. Predictive analytics help marketers identify potential customers, improving targeting strategies. AI automates content personalization, ensuring the right messages reach the right audience at the right time. Additionally, AI enhances advertising performance by analyzing engagement metrics and adjusting campaign strategies in real-time for maximum ROI.
6. Transportation and Logistics: Route Optimization and Traffic Management
The transportation and logistics industry leverages AI-driven data analytics for route optimization and traffic management. AI algorithms process real-time traffic data, weather conditions, and historical patterns to determine the most efficient delivery routes, reducing fuel consumption and improving delivery times. Ride-sharing companies use AI to match drivers with passengers efficiently, minimizing wait times and optimizing fleet utilization. In urban planning, AI-powered traffic management systems analyze congestion patterns and suggest alternative routes to improve traffic flow and reduce emissions.
7. Energy Sector: Smart Grids and Renewable Energy Optimization
In the energy sector, AI is playing a crucial role in optimizing energy consumption and integrating renewable energy sources. Smart grids leverage AI to predict energy demand and supply fluctuations, ensuring efficient distribution and reducing power outages. AI-powered data analytics help in monitoring equipment health, preventing failures in power plants and transmission networks. Renewable energy companies use AI to forecast weather patterns and optimize solar and wind energy generation, improving sustainability efforts.
8. Education: Personalized Learning and Administrative Efficiency
The education sector is increasingly adopting AI-driven data analytics to enhance personalized learning and streamline administrative tasks. AI-powered learning platforms analyze student performance data to customize learning materials and provide personalized recommendations. Automated grading systems reduce the burden on educators, allowing them to focus on interactive teaching. Predictive analytics help institutions identify students at risk of dropping out, enabling timely interventions to improve retention rates. Additionally, AI assists in optimizing resource allocation and curriculum planning, enhancing overall educational effectiveness.
9. Real Estate: Property Valuation and Market Trends Analysis
AI-driven data analytics is reshaping the real estate industry by improving property valuation and market trend analysis. AI models assess various factors, such as location, market demand, historical pricing data, and economic indicators, to predict property values accurately. Real estate platforms use AI to recommend properties based on user preferences, streamlining the home-buying process. AI-powered chatbots assist customers in finding suitable listings and answering queries in real time, improving customer experience.
Conclusion
AI has become an indispensable tool in data analytics, revolutionizing industries by offering predictive insights, automation, and efficiency improvements. Whether in healthcare, finance, retail, or manufacturing, AI-driven data analytics enhances decision-making and drives innovation. Businesses that integrate AI for data analytics into their operations gain a competitive edge by harnessing the power of machine learning and automation. As advancements in AI and data analytics software continue to evolve, their impact across industries will only become more profound, shaping the future of business intelligence and strategic decision-making.
#data visualization softwares#data visualization tools#tools for data visualization#rpa software#robotic process automation#unified data analytics platform
0 notes