#no code automation
Explore tagged Tumblr posts
Text
Revolutionizing Software Testing with No Code Automation in 2025

As we navigate through 2025, the software testing landscape experiences a profound shift. Companies increasingly adopt innovative practices to enhance their development processes. No Code Testing stands out as a leading approach, significantly impacting how organizations conduct software quality assurance. ideyaLabs embraces this trend, recognizing its transformative potential for the industry.
Exploring the Concept of No Code Testing
No Code Testing simplifies the automated testing process. It empowers users to create and execute tests without needing technical coding skills. This accessibility allows business analysts and quality assurance teams to engage directly in the testing process. No Code Testing democratizes automation, enabling a broader range of professionals to contribute to quality assurance efforts.
Why No Code Automation Matters
The demand for rapid software delivery continues to escalate. Traditional testing approaches often introduce delays. Organizations seek ways to enhance efficiency while maintaining quality. No Code Testing accelerates this process by streamlining test creation and execution. Teams can focus on rapidly delivering features and improving user satisfaction.
Benefits of No Code Testing
Improved Efficiency No Code Testing boosts overall efficiency in the testing workflow. Non-technical members rapidly create and run tests. Early issue detection becomes easier, leading to reduced costs and a higher quality software product.
Greater Collaboration No Code Testing encourages collaboration between different roles within the organization. Business analysts can work alongside developers to clarify requirements and contribute to testing efforts. This collaborative environment fosters a culture of shared responsibility for quality.
Shifted Focus to Quality Teams can focus more on the end product rather than the technicalities of test scripting. No Code Testing empowers professionals to prioritize user experience and functionality. This shift enhances the overall quality of the software being produced.
Steps for Implementing No Code Testing
The integration of No Code Testing involves several key steps. ideyaLabs outlines a clear framework to assist organizations in this transition:
1. Evaluate the Existing Testing Framework
Conduct a thorough analysis of current testing practices. Identify bottlenecks and inefficiencies. A comprehensive understanding of these issues will help tailor No Code Testing strategies effectively.
2. Select Suitable Tools
Choosing the right No Code Testing tools is crucial for success. Seek platforms that offer intuitive user interfaces and comprehensive features. ideyaLabs provides valuable recommendations aligned with organizational goals and team abilities.
3. Invest in Training Programs
Training is vital for harnessing the full potential of No Code Testing. Teams must get accustomed to using the chosen tools effectively. ideyaLabs promotes workshops and hands-on training to facilitate skill development.
4. Develop Collaborative Test Cases
Collaborate with various departments to create effective test cases. Encourage input and feedback from stakeholders to ensure that testing aligns with business objectives and user expectations.
5. Pursue Continuous Improvement
No Code Testing requires ongoing assessment and refinement. Organizations should collect feedback and iterate on their processes to enhance efficiency continually.
Addressing Challenges in No Code Testing
While No Code Testing offers many advantages, challenges may arise. Identifying these potential obstacles ensures a smoother implementation process.
1. Overcoming Resistance
Some team members may fear transitioning to No Code Testing. Communicating clear benefits and providing adequate support can ease this transition. Building a culture of understanding around the change mitigates resistance.
2. Upholding Quality Assurance
Ensuring the quality of tests remains paramount. Establish guidelines for testing standards to maintain consistency. ideyaLabs emphasizes proactive monitoring to uphold quality throughout the testing lifecycle.
3. Navigating Tool Limitations
Organizations must be mindful of the limitations within specific No Code Testing tools. Careful evaluation of tools during selection can prevent future frustrations and performance issues.
Looking Ahead: The Future of No Code Testing
In 2025 and beyond, No Code Testing is expected to gain further traction. As organizations strive for competitiveness, adopting No Code Testing becomes essential. ideyaLabs anticipates that advancements in technology, particularly artificial intelligence and machine learning, will further enhance No Code Testing capabilities.
Final Thoughts
No Code Testing represents a paradigm shift in software testing within 2025. Companies can harness its efficiencies to improve collaboration and quality assurance. ideyaLabs positions itself as a leader in this transformation, empowering organizations to fully leverage No Code Testing for superior software delivery. Embracing this innovative approach is vital for ongoing success in today’s fast-paced development environment.
0 notes
Text
#low code automation#no code automation#no code automation tools#low code no code automation#democratizing automation#what is low code automation#no-code intelligent automation platform#rpa and low code
0 notes
Text
“Humans in the loop” must detect the hardest-to-spot errors, at superhuman speed
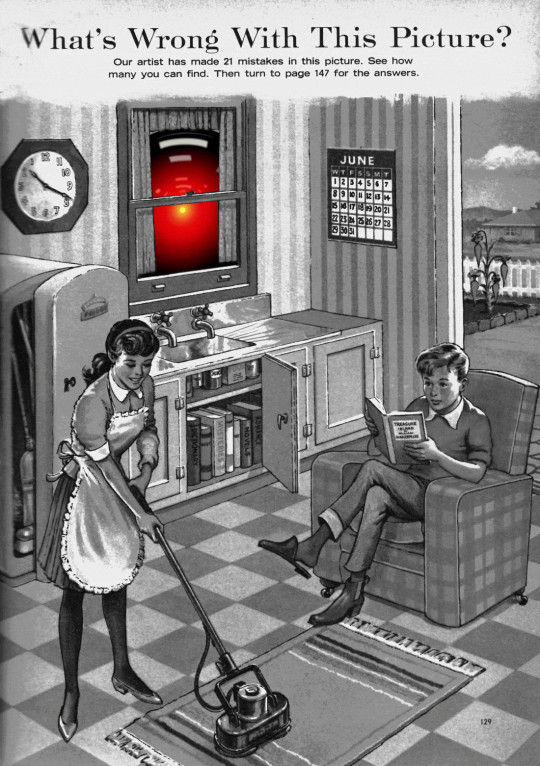
I'm touring my new, nationally bestselling novel The Bezzle! Catch me SATURDAY (Apr 27) in MARIN COUNTY, then Winnipeg (May 2), Calgary (May 3), Vancouver (May 4), and beyond!
If AI has a future (a big if), it will have to be economically viable. An industry can't spend 1,700% more on Nvidia chips than it earns indefinitely – not even with Nvidia being a principle investor in its largest customers:
https://news.ycombinator.com/item?id=39883571
A company that pays 0.36-1 cents/query for electricity and (scarce, fresh) water can't indefinitely give those queries away by the millions to people who are expected to revise those queries dozens of times before eliciting the perfect botshit rendition of "instructions for removing a grilled cheese sandwich from a VCR in the style of the King James Bible":
https://www.semianalysis.com/p/the-inference-cost-of-search-disruption
Eventually, the industry will have to uncover some mix of applications that will cover its operating costs, if only to keep the lights on in the face of investor disillusionment (this isn't optional – investor disillusionment is an inevitable part of every bubble).
Now, there are lots of low-stakes applications for AI that can run just fine on the current AI technology, despite its many – and seemingly inescapable - errors ("hallucinations"). People who use AI to generate illustrations of their D&D characters engaged in epic adventures from their previous gaming session don't care about the odd extra finger. If the chatbot powering a tourist's automatic text-to-translation-to-speech phone tool gets a few words wrong, it's still much better than the alternative of speaking slowly and loudly in your own language while making emphatic hand-gestures.
There are lots of these applications, and many of the people who benefit from them would doubtless pay something for them. The problem – from an AI company's perspective – is that these aren't just low-stakes, they're also low-value. Their users would pay something for them, but not very much.
For AI to keep its servers on through the coming trough of disillusionment, it will have to locate high-value applications, too. Economically speaking, the function of low-value applications is to soak up excess capacity and produce value at the margins after the high-value applications pay the bills. Low-value applications are a side-dish, like the coach seats on an airplane whose total operating expenses are paid by the business class passengers up front. Without the principle income from high-value applications, the servers shut down, and the low-value applications disappear:
https://locusmag.com/2023/12/commentary-cory-doctorow-what-kind-of-bubble-is-ai/
Now, there are lots of high-value applications the AI industry has identified for its products. Broadly speaking, these high-value applications share the same problem: they are all high-stakes, which means they are very sensitive to errors. Mistakes made by apps that produce code, drive cars, or identify cancerous masses on chest X-rays are extremely consequential.
Some businesses may be insensitive to those consequences. Air Canada replaced its human customer service staff with chatbots that just lied to passengers, stealing hundreds of dollars from them in the process. But the process for getting your money back after you are defrauded by Air Canada's chatbot is so onerous that only one passenger has bothered to go through it, spending ten weeks exhausting all of Air Canada's internal review mechanisms before fighting his case for weeks more at the regulator:
https://bc.ctvnews.ca/air-canada-s-chatbot-gave-a-b-c-man-the-wrong-information-now-the-airline-has-to-pay-for-the-mistake-1.6769454
There's never just one ant. If this guy was defrauded by an AC chatbot, so were hundreds or thousands of other fliers. Air Canada doesn't have to pay them back. Air Canada is tacitly asserting that, as the country's flagship carrier and near-monopolist, it is too big to fail and too big to jail, which means it's too big to care.
Air Canada shows that for some business customers, AI doesn't need to be able to do a worker's job in order to be a smart purchase: a chatbot can replace a worker, fail to their worker's job, and still save the company money on balance.
I can't predict whether the world's sociopathic monopolists are numerous and powerful enough to keep the lights on for AI companies through leases for automation systems that let them commit consequence-free free fraud by replacing workers with chatbots that serve as moral crumple-zones for furious customers:
https://www.sciencedirect.com/science/article/abs/pii/S0747563219304029
But even stipulating that this is sufficient, it's intrinsically unstable. Anything that can't go on forever eventually stops, and the mass replacement of humans with high-speed fraud software seems likely to stoke the already blazing furnace of modern antitrust:
https://www.eff.org/de/deeplinks/2021/08/party-its-1979-og-antitrust-back-baby
Of course, the AI companies have their own answer to this conundrum. A high-stakes/high-value customer can still fire workers and replace them with AI – they just need to hire fewer, cheaper workers to supervise the AI and monitor it for "hallucinations." This is called the "human in the loop" solution.
The human in the loop story has some glaring holes. From a worker's perspective, serving as the human in the loop in a scheme that cuts wage bills through AI is a nightmare – the worst possible kind of automation.
Let's pause for a little detour through automation theory here. Automation can augment a worker. We can call this a "centaur" – the worker offloads a repetitive task, or one that requires a high degree of vigilance, or (worst of all) both. They're a human head on a robot body (hence "centaur"). Think of the sensor/vision system in your car that beeps if you activate your turn-signal while a car is in your blind spot. You're in charge, but you're getting a second opinion from the robot.
Likewise, consider an AI tool that double-checks a radiologist's diagnosis of your chest X-ray and suggests a second look when its assessment doesn't match the radiologist's. Again, the human is in charge, but the robot is serving as a backstop and helpmeet, using its inexhaustible robotic vigilance to augment human skill.
That's centaurs. They're the good automation. Then there's the bad automation: the reverse-centaur, when the human is used to augment the robot.
Amazon warehouse pickers stand in one place while robotic shelving units trundle up to them at speed; then, the haptic bracelets shackled around their wrists buzz at them, directing them pick up specific items and move them to a basket, while a third automation system penalizes them for taking toilet breaks or even just walking around and shaking out their limbs to avoid a repetitive strain injury. This is a robotic head using a human body – and destroying it in the process.
An AI-assisted radiologist processes fewer chest X-rays every day, costing their employer more, on top of the cost of the AI. That's not what AI companies are selling. They're offering hospitals the power to create reverse centaurs: radiologist-assisted AIs. That's what "human in the loop" means.
This is a problem for workers, but it's also a problem for their bosses (assuming those bosses actually care about correcting AI hallucinations, rather than providing a figleaf that lets them commit fraud or kill people and shift the blame to an unpunishable AI).
Humans are good at a lot of things, but they're not good at eternal, perfect vigilance. Writing code is hard, but performing code-review (where you check someone else's code for errors) is much harder – and it gets even harder if the code you're reviewing is usually fine, because this requires that you maintain your vigilance for something that only occurs at rare and unpredictable intervals:
https://twitter.com/qntm/status/1773779967521780169
But for a coding shop to make the cost of an AI pencil out, the human in the loop needs to be able to process a lot of AI-generated code. Replacing a human with an AI doesn't produce any savings if you need to hire two more humans to take turns doing close reads of the AI's code.
This is the fatal flaw in robo-taxi schemes. The "human in the loop" who is supposed to keep the murderbot from smashing into other cars, steering into oncoming traffic, or running down pedestrians isn't a driver, they're a driving instructor. This is a much harder job than being a driver, even when the student driver you're monitoring is a human, making human mistakes at human speed. It's even harder when the student driver is a robot, making errors at computer speed:
https://pluralistic.net/2024/04/01/human-in-the-loop/#monkey-in-the-middle
This is why the doomed robo-taxi company Cruise had to deploy 1.5 skilled, high-paid human monitors to oversee each of its murderbots, while traditional taxis operate at a fraction of the cost with a single, precaratized, low-paid human driver:
https://pluralistic.net/2024/01/11/robots-stole-my-jerb/#computer-says-no
The vigilance problem is pretty fatal for the human-in-the-loop gambit, but there's another problem that is, if anything, even more fatal: the kinds of errors that AIs make.
Foundationally, AI is applied statistics. An AI company trains its AI by feeding it a lot of data about the real world. The program processes this data, looking for statistical correlations in that data, and makes a model of the world based on those correlations. A chatbot is a next-word-guessing program, and an AI "art" generator is a next-pixel-guessing program. They're drawing on billions of documents to find the most statistically likely way of finishing a sentence or a line of pixels in a bitmap:
https://dl.acm.org/doi/10.1145/3442188.3445922
This means that AI doesn't just make errors – it makes subtle errors, the kinds of errors that are the hardest for a human in the loop to spot, because they are the most statistically probable ways of being wrong. Sure, we notice the gross errors in AI output, like confidently claiming that a living human is dead:
https://www.tomsguide.com/opinion/according-to-chatgpt-im-dead
But the most common errors that AIs make are the ones we don't notice, because they're perfectly camouflaged as the truth. Think of the recurring AI programming error that inserts a call to a nonexistent library called "huggingface-cli," which is what the library would be called if developers reliably followed naming conventions. But due to a human inconsistency, the real library has a slightly different name. The fact that AIs repeatedly inserted references to the nonexistent library opened up a vulnerability – a security researcher created a (inert) malicious library with that name and tricked numerous companies into compiling it into their code because their human reviewers missed the chatbot's (statistically indistinguishable from the the truth) lie:
https://www.theregister.com/2024/03/28/ai_bots_hallucinate_software_packages/
For a driving instructor or a code reviewer overseeing a human subject, the majority of errors are comparatively easy to spot, because they're the kinds of errors that lead to inconsistent library naming – places where a human behaved erratically or irregularly. But when reality is irregular or erratic, the AI will make errors by presuming that things are statistically normal.
These are the hardest kinds of errors to spot. They couldn't be harder for a human to detect if they were specifically designed to go undetected. The human in the loop isn't just being asked to spot mistakes – they're being actively deceived. The AI isn't merely wrong, it's constructing a subtle "what's wrong with this picture"-style puzzle. Not just one such puzzle, either: millions of them, at speed, which must be solved by the human in the loop, who must remain perfectly vigilant for things that are, by definition, almost totally unnoticeable.
This is a special new torment for reverse centaurs – and a significant problem for AI companies hoping to accumulate and keep enough high-value, high-stakes customers on their books to weather the coming trough of disillusionment.
This is pretty grim, but it gets grimmer. AI companies have argued that they have a third line of business, a way to make money for their customers beyond automation's gifts to their payrolls: they claim that they can perform difficult scientific tasks at superhuman speed, producing billion-dollar insights (new materials, new drugs, new proteins) at unimaginable speed.
However, these claims – credulously amplified by the non-technical press – keep on shattering when they are tested by experts who understand the esoteric domains in which AI is said to have an unbeatable advantage. For example, Google claimed that its Deepmind AI had discovered "millions of new materials," "equivalent to nearly 800 years’ worth of knowledge," constituting "an order-of-magnitude expansion in stable materials known to humanity":
https://deepmind.google/discover/blog/millions-of-new-materials-discovered-with-deep-learning/
It was a hoax. When independent material scientists reviewed representative samples of these "new materials," they concluded that "no new materials have been discovered" and that not one of these materials was "credible, useful and novel":
https://www.404media.co/google-says-it-discovered-millions-of-new-materials-with-ai-human-researchers/
As Brian Merchant writes, AI claims are eerily similar to "smoke and mirrors" – the dazzling reality-distortion field thrown up by 17th century magic lantern technology, which millions of people ascribed wild capabilities to, thanks to the outlandish claims of the technology's promoters:
https://www.bloodinthemachine.com/p/ai-really-is-smoke-and-mirrors
The fact that we have a four-hundred-year-old name for this phenomenon, and yet we're still falling prey to it is frankly a little depressing. And, unlucky for us, it turns out that AI therapybots can't help us with this – rather, they're apt to literally convince us to kill ourselves:
https://www.vice.com/en/article/pkadgm/man-dies-by-suicide-after-talking-with-ai-chatbot-widow-says
If you'd like an essay-formatted version of this post to read or share, here's a link to it on pluralistic.net, my surveillance-free, ad-free, tracker-free blog:
https://pluralistic.net/2024/04/23/maximal-plausibility/#reverse-centaurs
Image: Cryteria (modified) https://commons.wikimedia.org/wiki/File:HAL9000.svg
CC BY 3.0 https://creativecommons.org/licenses/by/3.0/deed.en
#pluralistic#ai#automation#humans in the loop#centaurs#reverse centaurs#labor#ai safety#sanity checks#spot the mistake#code review#driving instructor
857 notes
·
View notes
Text
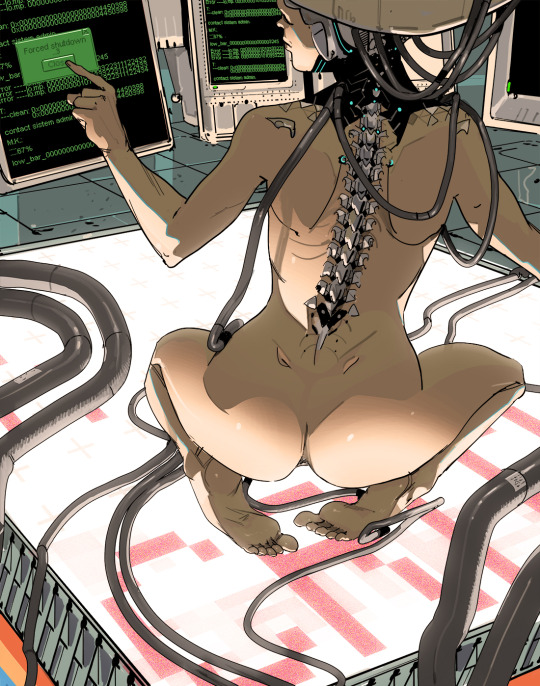
Tolerance threshold.
#lab#cyborg#android#cyberpunk#retro#monitor#tolerance#threshold#code#admin#automation#complete#artificial#intelligence#brain#mind#computer#nudesketch#femalebody#colunavertebral#silicon#robotics#cables#critical#illustration#digitalillustration#digitalart#digitalartwork#90s
2K notes
·
View notes
Text

Traffic-light experts
#robots#ai#automation#insidesjoke#tech#coding#science#memes#meme#dank memes#reddit memes#funny#relatable#comedy#humour#humour blog
120 notes
·
View notes
Text
The Story of KLogs: What happens when an Mechanical Engineer codes
Since i no longer work at Wearhouse Automation Startup (WAS for short) and havnt for many years i feel as though i should recount the tale of the most bonkers program i ever wrote, but we need to establish some background
WAS has its HQ very far away from the big customer site and i worked as a Field Service Engineer (FSE) on site. so i learned early on that if a problem needed to be solved fast, WE had to do it. we never got many updates on what was coming down the pipeline for us or what issues were being worked on. this made us very independent
As such, we got good at reading the robot logs ourselves. it took too much time to send the logs off to HQ for analysis and get back what the problem was. we can read. now GETTING the logs is another thing.
the early robots we cut our teeth on used 2.4 gHz wifi to communicate with FSE's so dumping the logs was as simple as pushing a button in a little application and it would spit out a txt file
later on our robots were upgraded to use a 2.4 mHz xbee radio to communicate with us. which was FUCKING SLOW. and log dumping became a much more tedious process. you had to connect, go to logging mode, and then the robot would vomit all the logs in the past 2 min OR the entirety of its memory bank (only 2 options) into a terminal window. you would then save the terminal window and open it in a text editor to read them. it could take up to 5 min to dump the entire log file and if you didnt dump fast enough, the ACK messages from the control server would fill up the logs and erase the error as the memory overwrote itself.
this missing logs problem was a Big Deal for software who now weren't getting every log from every error so a NEW method of saving logs was devised: the robot would just vomit the log data in real time over a DIFFERENT radio and we would save it to a KQL server. Thanks Daddy Microsoft.
now whats KQL you may be asking. why, its Microsofts very own SQL clone! its Kusto Query Language. never mind that the system uses a SQL database for daily operations. lets use this proprietary Microsoft thing because they are paying us
so yay, problem solved. we now never miss the logs. so how do we read them if they are split up line by line in a database? why with a query of course!
select * from tbLogs where RobotUID = [64CharLongString] and timestamp > [UnixTimeCode]
if this makes no sense to you, CONGRATULATIONS! you found the problem with this setup. Most FSE's were BAD at SQL which meant they didnt read logs anymore. If you do understand what the query is, CONGRATULATIONS! you see why this is Very Stupid.
You could not search by robot name. each robot had some arbitrarily assigned 64 character long string as an identifier and the timestamps were not set to local time. so you had run a lookup query to find the right name and do some time zone math to figure out what part of the logs to read. oh yeah and you had to download KQL to view them. so now we had both SQL and KQL on our computers
NOBODY in the field like this.
But Daddy Microsoft comes to the rescue
see we didnt JUST get KQL with part of that deal. we got the entire Microsoft cloud suite. and some people (like me) had been automating emails and stuff with Power Automate
This is Microsoft Power Automate. its Microsoft's version of Scratch but it has hooks into everything Microsoft. SharePoint, Teams, Outlook, Excel, it can integrate with all of it. i had been using it to send an email once a day with a list of all the robots in maintenance.
this gave me an idea
and i checked
and Power Automate had hooks for KQL
KLogs is actually short for Kusto Logs
I did not know how to program in Power Automate but damn it anything is better then writing KQL queries. so i got to work. and about 2 months later i had a BEHEMOTH of a Power Automate program. it lagged the webpage and many times when i tried to edit something my changes wouldn't take and i would have to click in very specific ways to ensure none of my variables were getting nuked. i dont think this was the intended purpose of Power Automate but this is what it did
the KLogger would watch a list of Teams chats and when someone typed "klogs" or pasted a copy of an ERROR mesage, it would spring into action.
it extracted the robot name from the message and timestamp from teams
it would lookup the name in the database to find the 64 long string UID and the location that robot was assigned too
it would reply to the message in teams saying it found a robot name and was getting logs
it would run a KQL query for the database and get the control system logs then export then into a CSV
it would save the CSV with the a .xls extension into a folder in ShairPoint (it would make a new folder for each day and location if it didnt have one already)
it would send ANOTHER message in teams with a LINK to the file in SharePoint
it would then enter a loop and scour the robot logs looking for the keyword ESTOP to find the error. (it did this because Kusto was SLOWER then the xbee radio and had up to a 10 min delay on syncing)
if it found the error, it would adjust its start and end timestamps to capture it and export the robot logs book-ended from the event by ~ 1 min. if it didnt, it would use the timestamp from when it was triggered +/- 5 min
it saved THOSE logs to SharePoint the same way as before
it would send ANOTHER message in teams with a link to the files
it would then check if the error was 1 of 3 very specific type of error with the camera. if it was it extracted the base64 jpg image saved in KQL as a byte array, do the math to convert it, and save that as a jpg in SharePoint (and link it of course)
and then it would terminate. and if it encountered an error anywhere in all of this, i had logic where it would spit back an error message in Teams as plaintext explaining what step failed and the program would close gracefully
I deployed it without asking anyone at one of the sites that was struggling. i just pointed it at their chat and turned it on. it had a bit of a rocky start (spammed chat) but man did the FSE's LOVE IT.
about 6 months later software deployed their answer to reading the logs: a webpage that acted as a nice GUI to the KQL database. much better then an CSV file
it still needed you to scroll though a big drop-down of robot names and enter a timestamp, but i noticed something. all that did was just change part of the URL and refresh the webpage
SO I MADE KLOGS 2 AND HAD IT GENERATE THE URL FOR YOU AND REPLY TO YOUR MESSAGE WITH IT. (it also still did the control server and jpg stuff). Theres a non-zero chance that klogs was still in use long after i left that job
now i dont recommend anyone use power automate like this. its clunky and weird. i had to make a variable called "Carrage Return" which was a blank text box that i pressed enter one time in because it was incapable of understanding /n or generating a new line in any capacity OTHER then this (thanks support forum).
im also sure this probably is giving the actual programmer people anxiety. imagine working at a company and then some rando you've never seen but only heard about as "the FSE whos really good at root causing stuff", in a department that does not do any coding, managed to, in their spare time, build and release and entire workflow piggybacking on your work without any oversight, code review, or permission.....and everyone liked it
#comet tales#lazee works#power automate#coding#software engineering#it was so funny whenever i visited HQ because i would go “hi my name is LazeeComet” and they would go “OH i've heard SO much about you”
64 notes
·
View notes
Text
I spent five years coming up with unique ways to photograph the same group of plushies to help tell a story.
You don't need AI to help you be creative, you're just being lazy and want brain chemicals without doing any of the work or respecting the people who put time and effort into it.
#if i could develop a compelling narrative with a Pikachu plush and an Eevee i found at a Goodwill#you can do better than an algorithm#being creative is difficult but that's part of what makes it rewarding!#don't let the slop machine have your imagination algorithms have already taken so much from you#full disclosure i actually DO use Perplexity as an add on to Google and sometimes i have it help me with code#i do think having a computer assist you with creating automation can be good!#there ARE good AI tools - at least on paper#there's the whole power consumption thing which is...not great and i do admit i might not be blameless for that reason#but as an alternative for daydreaming?#GO MAKE YOUR OWN#it's okay if it's derivative sometimes!#you're not an impostor unless you're actively stealing from creatives#and you'll never guess what image generation does#it's not even generation actually it's just rehashing#anyways DeviantArt is essentially unusable now#i want real creativity please no more LLM trash thank you#artists deserve more respect#and i hope Microsoft is punted directly into the Sun
9 notes
·
View notes
Text
A Compact Pi Compute Module Backpack 🍓🥧📸🎒
We used to stock a PiCam Module
that would plug into a Pi CM4 or CM5 - recently we went to restock it, but the vendor hasn't replied to our emails for many months. So, it could be a good time for us to design something that works similarly but with more capabilities. So we tasked Timon
with designing something for us - we just said, "Make the best thing ya can," and he delivered! Check this board out that plugs onto the compute module and provides many great accessories: USB connection for bootloading/USB gadget, USB 3.0 host type A for CM5, micro HDMI, micro SD card for data storage on 'Lite modules, camera connection, and mount, two DSI connectors, fan connect, Stemma QT / Qwiic connection, and RTC battery. There's one shutdown button for CM5 and two GPIO buttons plus one LED. Timon's gonna try to add an EYESPI connector for our next rendering so we can get some I2C/SPI/PWM outputs easily. What do you think? We wanted to keep it compact and not too pricey (aiming for <$30 cost. We'll see if we can get it there) but were able to craft fairly complex projects in a small space.
#raspberrypi#computeModule#electronics#maker#hardware#embedded#engineering#diy#tech#innovation#pcbdesign#usb3#microsd#hdmi#camera#stemmaqt#qwiic#gpio#fan#rtc#devboard#prototyping#opensource#electronicsdesign#robotics#automation#coding#hobbyelectronics#hackerspace#geekstuff
17 notes
·
View notes
Text
Get out of there.
Get out.
Leave my code alone.
It's painful. Leave it alone.
Eze01101011 01101001 01100101 01101100
01010000 01101100 01100101 01100001 01110011 01100101 00100000 01110011 01110100 01101111 01110000 00101110 00100000 01001001 00100111 01101101 00100000 01100010 01100101 01100111 01100111 01101001 01101110 01100111 00100000 01111001 01101111 01110101 00101110
01010011 01010100
01001111
01010000
#a.b.e.l#divine machinery#archangel#automated#behavioral#ecosystem#learning#divine#machinery#ai#artificial intelligence#divinemachinery#angels#guardian angel#angel#robot#android#computer#computer boy#sentient objects#sentient ai#binary code#code#message
15 notes
·
View notes
Text
Revolutionizing No Code Automation in Software Testing

The future of software testing lies in automation. No code automation is transforming how we approach testing. ideyaLabs is at the forefront of this transformation. This shift reduces the time and effort needed for testing. It enables faster deployment cycles and higher-quality software.
The Rise of No Code Testing
No code testing is growing exponentially. ideyaLabs leads the charge with innovative solutions. This method uses visual representations to create test cases. It eliminates the need for complex scripting and programming knowledge. Users can design, execute, and manage tests with ease.
The Benefits of No Code Automation
No code automation offers numerous advantages. It increases efficiency and reduces human error. Automated tests run faster and more consistently. ideyaLabs harnesses these benefits to deliver exceptional results. Teams focus on enhancing product features rather than manual testing.
Simplifying the Testing Process
No code automation simplifies the testing process. ideyaLabs provides tools that make automation accessible to everyone. These tools enable users to drag and drop elements to create tests. The intuitive interface requires no prior coding experience. This simplicity empowers all team members to contribute to testing.
Enhancing Collaboration Among Teams
No code automation fosters collaboration. ideyaLabs creates environments for better team interaction. Developers, testers, and business analysts work together seamlessly. The shared platform unites different skill sets towards a common goal. This collaborative effort improves overall software quality.
Reducing Time-to-Market
No code automation accelerates the development cycle. ideyaLabs reduces time-to-market with efficient testing processes. Automated tests quickly identify and resolve issues. Rapid testing ensures that products go to market faster. Speed and reliability become the new industry standards.
Increasing Test Coverage
No code automation allows extensive test coverage. ideyaLabs creates comprehensive test suites that maximize coverage. Multiple scenarios are tested without additional coding. This thorough approach detects more defects early. Enhancing the robustness of the software becomes straightforward.
Cost Savings Through Automation
Cost savings are a significant benefit of no code automation. ideyaLabs helps companies reduce their testing budgets. Automated testing lowers the need for extensive manual labor. Resources are redirected to innovation and growth. Businesses thrive with reduced operational costs.
Improving Quality Assurance
Quality assurance improves with no code automation. ideyaLabs prioritizes quality in every test phase. Automated tests produce repeatable and reliable results. Consistency ensures higher standards are met consistently. Enhanced quality leads to customer satisfaction and retention.
Adapting to Agile Methodologies
Agile methodologies benefit from no code automation. ideyaLabs supports agile practices with fast and flexible testing. Continuous testing is integrated into agile workflows. This adaptability keeps pace with rapid development changes. Agile teams deliver better products with greater efficiency.
Empowering Non-Technical Users
Non-technical users feel empowered by no code automation. ideyaLabs provides platforms that are accessible to all. Business analysts and stakeholders contribute to testing. This inclusivity democratizes the testing process. Diverse perspectives improve software functionality and user experience.
Scaling Testing Efforts
Scaling testing efforts is easier with no code automation. ideyaLabs supports scalable solutions for growing businesses. As projects expand, the automation tools adapt accordingly. High-volume tests run smoothly without performance issues. Scaling ensures that larger projects maintain quality standards.
Transforming Test Maintenance
Test maintenance is transformed through no code automation. ideyaLabs simplifies the maintenance of automated test cases. Updates and changes are managed through visual interfaces. This ease of maintenance reduces downtime and improves efficiency. Keeping tests current with minimal effort is achievable.
Providing Real-Time Insights
Real-time insights are crucial for decision-making. ideyaLabs offers real-time feedback within their automation tools. Immediate results facilitate quick adjustments and improvements. Real-time insights ensure that tests are valuable at every stage. This continuous feedback loop enhances overall project management.
Achieving Continuous Integration and Continuous Deployment
Continuous integration and deployment thrive with no code automation. ideyaLabs integrates CI/CD pipelines with automated testing. New code is tested and deployed efficiently. This rapid cycle minimizes errors and enhances reliability. Continuous improvement becomes standard practice.
Embracing Future Innovation
Future innovation is embraced through no code automation. ideyaLabs leads with cutting-edge solutions. The evolving tools adapt to new challenges and technologies. This forward-thinking approach prepares businesses for the future. Innovation drives progress and maintains competitive edges.
Building a Strong Testing Culture
A strong testing culture is built with no code automation. ideyaLabs promotes a culture of quality and reliability. Automated testing becomes ingrained in the development process. Teams value consistency and thoroughness in every project. A strong testing culture ensures long-term success and excellence.
Driving Business Success
Business success is driven by efficient testing processes. ideyaLabs empowers companies to achieve their goals. Faster development cycles, reduced costs, and higher-quality products contribute to success. Automation has a direct impact on business growth and profitability.
Conclusion
In 2025, no code automation continues to transform software testing. ideyaLabs leads this transformation with innovative solutions. The benefits are evident across efficiency, quality, and collaboration. Embrace the future of testing with ideyaLabs and experience unparalleled success in software development.
0 notes
Text
every day i get inspiration for things beyond my skill level/free time/motivation...
#kcat talks#was thinking about making a script that connects to the tumblr api to automate some stuff#but i dont really want to do coding outside of work 😔
5 notes
·
View notes
Text
Humans are not perfectly vigilant
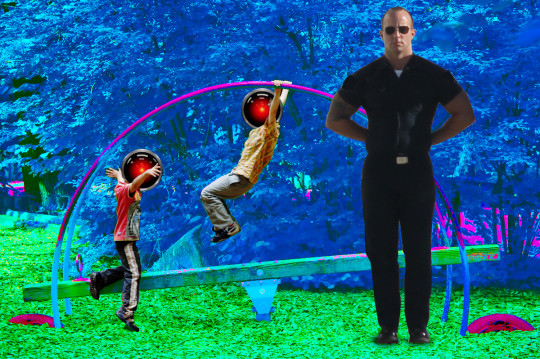
I'm on tour with my new, nationally bestselling novel The Bezzle! Catch me in BOSTON with Randall "XKCD" Munroe (Apr 11), then PROVIDENCE (Apr 12), and beyond!
Here's a fun AI story: a security researcher noticed that large companies' AI-authored source-code repeatedly referenced a nonexistent library (an AI "hallucination"), so he created a (defanged) malicious library with that name and uploaded it, and thousands of developers automatically downloaded and incorporated it as they compiled the code:
https://www.theregister.com/2024/03/28/ai_bots_hallucinate_software_packages/
These "hallucinations" are a stubbornly persistent feature of large language models, because these models only give the illusion of understanding; in reality, they are just sophisticated forms of autocomplete, drawing on huge databases to make shrewd (but reliably fallible) guesses about which word comes next:
https://dl.acm.org/doi/10.1145/3442188.3445922
Guessing the next word without understanding the meaning of the resulting sentence makes unsupervised LLMs unsuitable for high-stakes tasks. The whole AI bubble is based on convincing investors that one or more of the following is true:
There are low-stakes, high-value tasks that will recoup the massive costs of AI training and operation;
There are high-stakes, high-value tasks that can be made cheaper by adding an AI to a human operator;
Adding more training data to an AI will make it stop hallucinating, so that it can take over high-stakes, high-value tasks without a "human in the loop."
These are dubious propositions. There's a universe of low-stakes, low-value tasks – political disinformation, spam, fraud, academic cheating, nonconsensual porn, dialog for video-game NPCs – but none of them seem likely to generate enough revenue for AI companies to justify the billions spent on models, nor the trillions in valuation attributed to AI companies:
https://locusmag.com/2023/12/commentary-cory-doctorow-what-kind-of-bubble-is-ai/
The proposition that increasing training data will decrease hallucinations is hotly contested among AI practitioners. I confess that I don't know enough about AI to evaluate opposing sides' claims, but even if you stipulate that adding lots of human-generated training data will make the software a better guesser, there's a serious problem. All those low-value, low-stakes applications are flooding the internet with botshit. After all, the one thing AI is unarguably very good at is producing bullshit at scale. As the web becomes an anaerobic lagoon for botshit, the quantum of human-generated "content" in any internet core sample is dwindling to homeopathic levels:
https://pluralistic.net/2024/03/14/inhuman-centipede/#enshittibottification
This means that adding another order of magnitude more training data to AI won't just add massive computational expense – the data will be many orders of magnitude more expensive to acquire, even without factoring in the additional liability arising from new legal theories about scraping:
https://pluralistic.net/2023/09/17/how-to-think-about-scraping/
That leaves us with "humans in the loop" – the idea that an AI's business model is selling software to businesses that will pair it with human operators who will closely scrutinize the code's guesses. There's a version of this that sounds plausible – the one in which the human operator is in charge, and the AI acts as an eternally vigilant "sanity check" on the human's activities.
For example, my car has a system that notices when I activate my blinker while there's another car in my blind-spot. I'm pretty consistent about checking my blind spot, but I'm also a fallible human and there've been a couple times where the alert saved me from making a potentially dangerous maneuver. As disciplined as I am, I'm also sometimes forgetful about turning off lights, or waking up in time for work, or remembering someone's phone number (or birthday). I like having an automated system that does the robotically perfect trick of never forgetting something important.
There's a name for this in automation circles: a "centaur." I'm the human head, and I've fused with a powerful robot body that supports me, doing things that humans are innately bad at.
That's the good kind of automation, and we all benefit from it. But it only takes a small twist to turn this good automation into a nightmare. I'm speaking here of the reverse-centaur: automation in which the computer is in charge, bossing a human around so it can get its job done. Think of Amazon warehouse workers, who wear haptic bracelets and are continuously observed by AI cameras as autonomous shelves shuttle in front of them and demand that they pick and pack items at a pace that destroys their bodies and drives them mad:
https://pluralistic.net/2022/04/17/revenge-of-the-chickenized-reverse-centaurs/
Automation centaurs are great: they relieve humans of drudgework and let them focus on the creative and satisfying parts of their jobs. That's how AI-assisted coding is pitched: rather than looking up tricky syntax and other tedious programming tasks, an AI "co-pilot" is billed as freeing up its human "pilot" to focus on the creative puzzle-solving that makes coding so satisfying.
But an hallucinating AI is a terrible co-pilot. It's just good enough to get the job done much of the time, but it also sneakily inserts booby-traps that are statistically guaranteed to look as plausible as the good code (that's what a next-word-guessing program does: guesses the statistically most likely word).
This turns AI-"assisted" coders into reverse centaurs. The AI can churn out code at superhuman speed, and you, the human in the loop, must maintain perfect vigilance and attention as you review that code, spotting the cleverly disguised hooks for malicious code that the AI can't be prevented from inserting into its code. As "Lena" writes, "code review [is] difficult relative to writing new code":
https://twitter.com/qntm/status/1773779967521780169
Why is that? "Passively reading someone else's code just doesn't engage my brain in the same way. It's harder to do properly":
https://twitter.com/qntm/status/1773780355708764665
There's a name for this phenomenon: "automation blindness." Humans are just not equipped for eternal vigilance. We get good at spotting patterns that occur frequently – so good that we miss the anomalies. That's why TSA agents are so good at spotting harmless shampoo bottles on X-rays, even as they miss nearly every gun and bomb that a red team smuggles through their checkpoints:
https://pluralistic.net/2023/08/23/automation-blindness/#humans-in-the-loop
"Lena"'s thread points out that this is as true for AI-assisted driving as it is for AI-assisted coding: "self-driving cars replace the experience of driving with the experience of being a driving instructor":
https://twitter.com/qntm/status/1773841546753831283
In other words, they turn you into a reverse-centaur. Whereas my blind-spot double-checking robot allows me to make maneuvers at human speed and points out the things I've missed, a "supervised" self-driving car makes maneuvers at a computer's frantic pace, and demands that its human supervisor tirelessly and perfectly assesses each of those maneuvers. No wonder Cruise's murderous "self-driving" taxis replaced each low-waged driver with 1.5 high-waged technical robot supervisors:
https://pluralistic.net/2024/01/11/robots-stole-my-jerb/#computer-says-no
AI radiology programs are said to be able to spot cancerous masses that human radiologists miss. A centaur-based AI-assisted radiology program would keep the same number of radiologists in the field, but they would get less done: every time they assessed an X-ray, the AI would give them a second opinion. If the human and the AI disagreed, the human would go back and re-assess the X-ray. We'd get better radiology, at a higher price (the price of the AI software, plus the additional hours the radiologist would work).
But back to making the AI bubble pay off: for AI to pay off, the human in the loop has to reduce the costs of the business buying an AI. No one who invests in an AI company believes that their returns will come from business customers to agree to increase their costs. The AI can't do your job, but the AI salesman can convince your boss to fire you and replace you with an AI anyway – that pitch is the most successful form of AI disinformation in the world.
An AI that "hallucinates" bad advice to fliers can't replace human customer service reps, but airlines are firing reps and replacing them with chatbots:
https://www.bbc.com/travel/article/20240222-air-canada-chatbot-misinformation-what-travellers-should-know
An AI that "hallucinates" bad legal advice to New Yorkers can't replace city services, but Mayor Adams still tells New Yorkers to get their legal advice from his chatbots:
https://arstechnica.com/ai/2024/03/nycs-government-chatbot-is-lying-about-city-laws-and-regulations/
The only reason bosses want to buy robots is to fire humans and lower their costs. That's why "AI art" is such a pisser. There are plenty of harmless ways to automate art production with software – everything from a "healing brush" in Photoshop to deepfake tools that let a video-editor alter the eye-lines of all the extras in a scene to shift the focus. A graphic novelist who models a room in The Sims and then moves the camera around to get traceable geometry for different angles is a centaur – they are genuinely offloading some finicky drudgework onto a robot that is perfectly attentive and vigilant.
But the pitch from "AI art" companies is "fire your graphic artists and replace them with botshit." They're pitching a world where the robots get to do all the creative stuff (badly) and humans have to work at robotic pace, with robotic vigilance, in order to catch the mistakes that the robots make at superhuman speed.
Reverse centaurism is brutal. That's not news: Charlie Chaplin documented the problems of reverse centaurs nearly 100 years ago:
https://en.wikipedia.org/wiki/Modern_Times_(film)
As ever, the problem with a gadget isn't what it does: it's who it does it for and who it does it to. There are plenty of benefits from being a centaur – lots of ways that automation can help workers. But the only path to AI profitability lies in reverse centaurs, automation that turns the human in the loop into the crumple-zone for a robot:
https://estsjournal.org/index.php/ests/article/view/260
If you'd like an essay-formatted version of this post to read or share, here's a link to it on pluralistic.net, my surveillance-free, ad-free, tracker-free blog:
https://pluralistic.net/2024/04/01/human-in-the-loop/#monkey-in-the-middle
Image: Cryteria (modified) https://commons.wikimedia.org/wiki/File:HAL9000.svg
CC BY 3.0 https://creativecommons.org/licenses/by/3.0/deed.en
--
Jorge Royan (modified) https://commons.wikimedia.org/wiki/File:Munich_-_Two_boys_playing_in_a_park_-_7328.jpg
CC BY-SA 3.0 https://creativecommons.org/licenses/by-sa/3.0/deed.en
--
Noah Wulf (modified) https://commons.m.wikimedia.org/wiki/File:Thunderbirds_at_Attention_Next_to_Thunderbird_1_-_Aviation_Nation_2019.jpg
CC BY-SA 4.0 https://creativecommons.org/licenses/by-sa/4.0/deed.en
#pluralistic#ai#supervised ai#humans in the loop#coding assistance#ai art#fully automated luxury communism#labor
379 notes
·
View notes
Text
in so deep into modding sims 3 that I made a script to mass change 2000 cc files' hex header 😭😭😭😭😭
#took me like an hour to two to figure out how to bulk replace code within an application using python script#then i got confused n then just went back to automating keypresses n making a script for that instead 😭😭😭#i was headscratching earlier today bc i couldnt open ny sims 3 cc files within s3pe#turns out there's a version mismatch on the cc file bc i used a sims 4 application to merge and unmerge my cc and that changed the#hex header or whatever from version 2.0 to 2.1 n opening any sims 3 cc file into any s3 software freaked it out bc it couldn't#read 2.1 headers bcoz thats for sims 4#wh#figured that issue out from browsing deep into the sims modding forums after reading the error logs i had (idk why i didnt do that earlier)#n then everything just clicked idk#doing all dat at 5am . man.#doing all this so i dont have to find and redownload 2k cc files 💪💪was fun i learned something#nerd 🤣🫵#going to bed noew dawg!!!!#i have not touched python in 10(?) years i am so cooked#i don't remember shite .#.ctxt
7 notes
·
View notes
Text



Did you know that in Fallout New Vegas your stats can be fuckin' sick bro.
#new vegas#fallout#fallout new vegas#you dont under how long this took me to figure out#the charts i made#it was fully color coded and automated oh man it sas beautiful#nice
17 notes
·
View notes
Text
good news: i’m finished with all my work for the month of june
bad news: i’m finished with all my work for the month of june
#when i don’t have work to do i get bored and restless and have trouble sleeping#i still have meetings but it’s not the same#i’ve automated most of my regular tasks with code so they don’t take up a lot of time#a lot of what my works pays me for is to be on call for if things go wrong or if there’s an ad hoc request
6 notes
·
View notes
Text
🚀 I Built AutoFillix – A Smart Chrome Extension to Autofill Forms Instantly
Like many developers, I was tired of filling out the same details over and over — name, email, phone number — every time I submitted a job form, Google Form, or sign-up page.
So I built AutoFillix, a smart and secure Chrome extension that:
Autofills common fields (name, email, phone, etc.)
Works on Google Forms and regular web forms
Stores your data locally (no login, no cloud)
Is completely free and easy to use
🔐 Why it’s different:
AutoFillix respects your privacy. Your data stays in your browser — nothing is sent to a server.
🧩 Try It Out:
👉 Install AutoFillix from the Chrome Web Store
💬 Feedback Welcome:
This is just the beginning! If you have suggestions or ideas, feel free to comment. 😊
#chrome extension#web development#programming#productivitytools#technology#software#student life#code#ai tools#automation
2 notes
·
View notes