#Computation
Explore tagged Tumblr posts
Text

William J. Mitchell, The Logic of Architecture Design, Computation and Cognition, A Vocabulary of Stair Motifs (After Thiis Evensen, 1988)
#William J. Mitchell#stair#architecture#design#art#vocabulary#a vocabulary of stair motifs#the logic of architecture design#computation#cognition
738 notes
·
View notes
Text
Is it possible to deduce the shape of a drum from the sounds it makes? This is the kind of question that Iosif Polterovich, a professor in the Department of Mathematics and Statistics at Université de Montréal, likes to ask. Polterovich uses spectral geometry, a branch of mathematics, to understand physical phenomena involving wave propagation. Last summer, Polterovich and his international collaborators—Nikolay Filonov, Michael Levitin and David Sher—proved a special case of a famous conjecture in spectral geometry formulated in 1954 by the eminent Hungarian-American mathematician George Pólya. The conjecture bears on the estimation of the frequencies of a round drum or, in mathematical terms, the eigenvalues of a disk.
Continue Reading.
86 notes
·
View notes
Text
Why there's no intelligence in Artificial Intelligence
You can blame it all on Turing. When Alan Turing invented his mathematical theory of computation, what he really tried to do was to construct a mechanical model for the processes actual mathematicians employ when they prove a mathematical theorem. He was greatly influenced by Kurt Gödel and his incompleteness theorems. Gödel developed a method to decode logical mathematical statements as numbers and in that way was able to manipulate these statements algebraically. After Turing managed to construct a model capable of performing any arbitrary computation process (which we now call "A Universal Turing Machine") he became convinced that he discovered the way the human mind works. This conviction quickly infected the scientific community and became so ubiquitous that for many years it was rare to find someone who argued differently, except on religious grounds.
There was a good reason for adopting the hypothesis that the mind is a computation machine. This premise was following the extremely successful paradigm stating that biology is physics (or, to be precise, biology is both physics and chemistry, and chemistry is physics), which reigned supreme over scientific research since the eighteenth century. It was already responsible for the immense progress that completely transformed modern biology, biochemistry, and medicine. Turing seemed to supply a solution, within this theoretical framework, for the last large piece in the puzzle. There was now a purely mechanistic model for the way brain operation yields all the complex repertoire of human (and animal) behavior.
Obviously, not every computation machine is capable of intelligent conscious thought. So, where do we draw the line? For instance, at what point can we say that a program running on a computer understands English? Turing provided a purely behavioristic test: a computation understands a language if by conversing with it we cannot distinguish it from a human.
This is quite a silly test, really. It doesn't provide any clue as to what actually happens within the artificial "mind"; it assumes that the external behavior of an entity completely encapsulates its internal state; it requires "man in the loop" to provide the final ruling; it does not state for how long and on what level should this conversation be held. Such a test may serve as a pragmatic common-sense method to filter out obvious failures, but it brings us not an ounce closer to understanding conscious thinking.
Still, the Turing Test stuck. If anyone tried to question the computational model of the mind, he was then confronted with the unavoidable question: what else can it be? After all, biology is physics, and therefore the brain is just a physical machine. Physics is governed by equations, which are all, in theory, computable (at least approximately, with errors being as small as one wishes). So, short of conjuring supernatural soul that magically produces a conscious mind out of biological matter, there can be no other solution.

Nevertheless, not everyone conformed to the new dogma. There were two tiers of reservations to computational Artificial Intelligence. The first, maintained, for example, by the Philosopher John Searl, didn't object to idea that a computation device may, in principle, emulate any human intellectual capability. However, claimed Searl, a simulation of a conscious mind is not conscious in itself.
To demonstrate this point Searl envisioned a person who doesn't know a single word in Chinese, sitting in a secluded room. He receives Chinese texts from the outside through a small window and is expected to return responses in Chinese. To do that he uses written manuals that contain the AI algorithm which incorporates a comprehensive understanding of the Chinese language. Therefore, a person fluent in Chinese that converses with the "room" shall deduce, based on Turing Test, that it understands the language. However, in fact there's no one there but a man using a printed recipe to convert an input message he doesn't understands to an output message he doesn't understands. So, who in the room understands Chinese?
The next tier of opposition to computationalism was maintained by the renowned physicist and mathematician Roger Penrose, claiming that the mind has capabilities which no computational process can reproduce. Penrose considered a computational process that imitates a human mathematician. It analyses mathematical conjecture of a certain type and tries to deduce the answer to that problem. To arrive at a correct answer the process must employ valid logical inferences. The quality of such computerized mathematician is measured by the scope of problems it can solve.
What Penrose proved is that such a process can never verify in any logically valid way that its own processing procedures represent valid logical deductions. In fact, if it assumes, as part of its knowledge base, that its own operations are necessarily logically valid, then this assumption makes them invalid. In other words, a computational machine cannot be simultaneously logically rigorous and aware of being logically rigorous.
A human mathematician, on the other hand, is aware of his mental processes and can verify for himself that he is making correct deductions. This is actually an essential part of his profession. It follows that, at least with respect to mathematicians, cognitive functions cannot be replicated computationally.
Neither Searl's position nor Penrose's was accepted by the mainstream, mainly because, if not computation, "what else can it be?". Penrose's suggestion that mental processes involve quantum effects was rejected offhandedly, as "trying to explicate one mystery by swapping it with another mystery". And the macroscopic hot, noisy brain seemed a very implausible place to look for quantum phenomena, which typically occur in microscopic, cold and isolated systems.
Fast forward several decades. Finaly, it seemed as though the vision of true Artificial Intelligence technology started bearing fruits. A class of algorithms termed Deep Neural Networks (DNN) achieved, at last, some human-like capabilities. It managed to identify specific objects in pictures and videos, generate photorealistic images, translate voice to text, and support a wide variety of other pattern recognition and generation tasks. Most impressively, it seemed to have mastered natural language and could partake in an advanced discourse. The triumph of computational AI appeared more feasible than ever. Or was it?
During my years as undergraduate and graduate student I sometimes met fellow students who, at first impression, appeared to be far more conversant in the academic courses subject matter than me. They were highly confident and knew a great deal about things that were only briefly discussed in lectures. Therefore, I was vastly surprised when it turned out they were not particularly good students, and that they usually scored worse than me in the exams. It took me some time to realize that these people hadn't really possessed a better understanding of the curricula. They just adopted the correct jargon, employed the right words, so that, to the layperson ears, they had sounded as if they knew what they were talking about.
I was reminded of these charlatans when I encountered natural language AIs such as Chat GPT. At first glance, their conversational abilities seem impressive – fluent, elegant and decisive. Their style is perfect. However, as you delve deeper, you encounter all kinds of weird assertions and even completely bogus statements, uttered with absolute confidence. Whenever their knowledge base is incomplete, they just fill the gap with fictional "facts". And they can't distinguish between different levels of source credibility. They're like Idiot Savants – superficially bright, inherently stupid.
What confuses so many people with regard to AIs is that they seem to pass the (purely behavioristic) Turing Test. But behaviorism is a fundamentally non-scientific viewpoint. At the core, computational AIs are nothing but algorithms that generates a large number of statistical heuristics from enormous data sets.
There is an old anecdote about a classification AI that was supposed to distinguish between friendly and enemy tanks. Although the AI performed well with respect to the database, it failed miserably in field tests. Finely, the developers figured out the source of the problem. Most of the friendly tanks' images in the database were taken during good weather and with fine lighting conditions. The enemy tanks were mostly photographed in cloudy, darker weather. The AI simply learned to identify the environmental condition.
Though this specific anecdote is probably an urban legend, it illustrates the fact that AIs don't really know what they're doing. Therefore, attributing intelligence to Arificial Intelligence algorithms is a misconception. Intelligence is not the application of a complicated recipe to data. Rather, it is a self-critical analysis that generates meaning from input. Moreover, because intelligence requires not only understanding of the data and its internal structure, but also inner-understanding of the thought processes that generate this understanding, as well as an inner-understanding of this inner-understanding (and so forth), it can never be implemented using a finite set of rules. There is something of the infinite in true intelligence and in any type of conscious thought.
But, if not computation, "what else can it be?". The substantial progress made in quantum theory and quantum computation revived the old hypothesis by Penrose that the working of the mind is tightly coupled to the quantum nature of the brain. What had been previously regarded as esoteric and outlandish suddenly became, in light of recent advancements, a relevant option.
During the last thirty years, quantum computation has been transformed from a rather abstract idea made by the physicist Richard Feynman into an operational technology. Several quantum algorithms were shown to have a fundamental advantage over any corresponding classical algorithm. Some tasks that are extremely hard to fulfil through standard computation (for example, factorization of integers to primes) are easy to achieve quantum mechanically. Note that this difference between hard and easy is qualitative rather than quantitative. It's independent of which hardware and how much resources we dedicate to such tasks.
Along with the advancements in quantum computation came a surging realization that quantum theory is still an incomplete description of nature, and that many quantum effects cannot be really resolved form a conventional materialistic viewpoint. This understanding was first formalized by John Stewart Bell in the 1960s and later on expanded by many other physicists. It is now clear that by accepting quantum mechanics, we have to abandon at least some deep-rooted philosophical perceptions. And it became even more conceivable that any comprehensive understanding of the physical world should incorporate a theory of the mind that experiences it. It's only stands to reason that, if the human mind is an essential component of a complete quantum theory, then the quantum is an essential component of the workings of the mind. If that's the case, then it's clear that a classical algorithm, sophisticated as it may be, can never achieve true intelligence. It lacks an essential physical ingredient that is vital for conscious, intelligent thinking. Trying to simulate such thinking computationally is like trying to build a Perpetuum Mobile or chemically transmute lead into gold. You might discover all sorts of useful things along the way, but you would never reach your intended goal. Computational AIs shall never gain true intelligence. In that respect, this technology is a dead end.
#physics#ai#artificial intelligence#Alan Turing#computation#science#quantum physics#mind and body#John Searl#Roger Penrose
20 notes
·
View notes
Text


17.Dec.2024
Honestly treating my PhD like my engineering undergrad is the most fun thing ever. I’m not working as hard as I did as a baby engineer, but learning stuff I find incredibly fascinating is so much FUN! You’re telling me I get to code, debug, play with new tools, collect and play with data, then just keep learning whatever I want?? This is a treat. It’s stuff like this that makes me feel lucky. Always the student, sometimes the teacher, never the expert 😉
#studyblr#gradblr#phdblr#phd life#phdjourney#engineerblr#phd student#psychblr#cognitive science#computation
9 notes
·
View notes
Text
N64 architecture
#nintendo 64#nintendo#hardware#programming#tech#CPU#GPU#RAM#computation#MIPS#SGI#silicon graphics#NEC#90s#vintage computing
33 notes
·
View notes
Quote
The Golem hypothesis raises important questions: if life can be made from materials unlike those that gave rise to life as we know it, what are the shared principles that give rise to all living things? What are the universal properties of life-supporting chemistry?
Is life a complex computational process? | Aeon Essays
8 notes
·
View notes
Text
I want to make this piece of software. I want this piece of software to be a good piece of software. As part of making it a good piece of software, i want it to be fast. As part of making it fast, i want to be able to paralellize what i can. As part of that paralellization, i want to use compute shaders. To use compute shaders, i need some interface to graphics processors. After determining that Vulkan is not an API that is meant to be used by anybody, i decided to use OpenGL instead. In order for using OpenGL to be useful, i need some way to show the results to the user and get input from the user. I can do this by means of the Wayland API. In order to bridge the gap between Wayland and OpenGL, i need to be able to create an OpenGL context where the default framebuffer is the same as the Wayland surface that i've set to be a window. I can do this by means of EGL. In order to use EGL to create an OpenGL context, i need to select a config for the context.
Unfortunately, it just so happens that on my Linux partition, the implementation of EGL does not support the config that i would need for this piece of software.
Therefore, i am going to write this piece of software for 9front instead, using my 9front partition.
#Update#Programming#Technology#Wayland#OpenGL#Computers#Operating systems#EGL (API)#Windowing systems#3D graphics#Wayland (protocol)#Computer standards#Code#Computer graphics#Standards#Graphics#Computing standards#3D computer graphics#OpenGL API#EGL#Computer programming#Computation#Coding#OpenGL graphics API#Wayland protocol#Implementation of standards#Computational technology#Computing#OpenGL (API)#Process of implementation of standards
9 notes
·
View notes
Text
youtube
I am here to tell you about a silly accomplishment of mine, via the medium of an even sillier song.
(The full video, paper etc are linked from https://www.toothycat.net/~hologram/Magic/ , but I like the silly song best. And it's only 2 minutes long.)
#magic the gathering#turing machine#computation#silly songs#gilbert and sullivan#parody song#mtg#magic microcontroller#Youtube
3 notes
·
View notes
Text
“Thinking can be best understood in terms of representational structures in the mind and computational procedures that operate on those structures.”
3 notes
·
View notes
Text

Aula de Introdução a Ciência da Computação : )
Aprendendo a programar em Python
2 notes
·
View notes
Text

William J. Mitchell, The Logic of Architecture Design, Computation and Cognition, A Vocabulary of Roof Themes (After Thiis Evensen, 1988)
#art#design#architecture#William J. Mitchell#the logic of architecture design#computation and cognition#computation#a vocabulary of roof themes#roof#vocabulary#Thiis Evensen
61 notes
·
View notes
Text


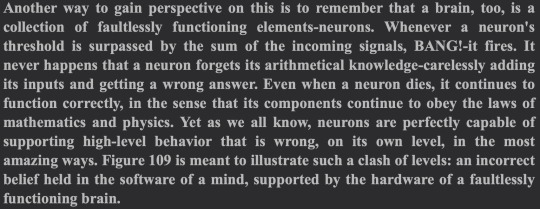
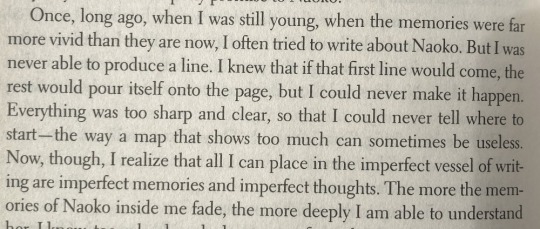



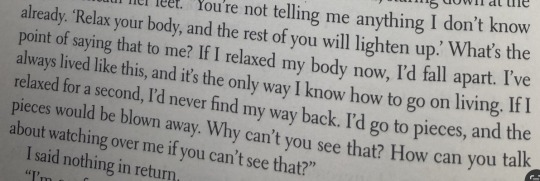
body/flesh as an inescapable filter through which all information is sent before it is committed to memory, perfection of physical function as dissociated from the imperfection of the symbols that emerge at the highest level of abstraction
murakami / hofstadter
10 notes
·
View notes
Text
You can compute someone’s neural pathways using their patterns of speech. If a computer running AI software can be programmed to do this then who can be trusted with this technology? It can also be expanded beyond speech and used to compute what someone most commonly thinks about.
1 note
·
View note
Text
nothing funnier to me than when AI does math wrong. like I get why it happens, it's a language model that's treating the numbers you feed it as words rather than integers and then giving you an answer based on how those words typically appear in a block of text instead of actually performing a calculation. but the one thing computers are genuinely incredible at. you fucked up a perfectly good calculator is what you did, look at it it's got hallucinations
#hilarious to me every time.#a computer exponentially more powerful than the equipment needed to put people on the moon can't count the Ns in ''mayonnaise''
91K notes
·
View notes
Text
Computer Science major here, it's not working because the computer doesn't respect you. download viruses on it to remind it who's boss.
follow for more tits
68K notes
·
View notes