#automated data analytics
Explore tagged Tumblr posts
Text
AI Analytics Explained: How it Works and Industry Use Cases
AI analytics, a powerful fusion of artificial intelligence and data analytics, has become a cornerstone in unlocking deeper insights from vast datasets. The synergy between these two transformative technologies revolutionizes the way organizations extract value from their data, leading to increased efficiency and strategic decision-making.
At the core of AI analytics is the automated data analytics process. Traditional data analytics involves manual data preparation, exploration, and interpretation, which can be time-consuming and prone to human error. AI steps in to automate these tasks, utilizing machine learning algorithms to streamline the entire analytics pipeline. Automated data analytics, facilitated by AI, accelerates the speed at which insights are generated, allowing organizations to respond rapidly to evolving trends and challenges.
One key component of AI analytics is machine learning, which enables systems to learn from data patterns and make predictions or recommendations. In industry use cases, predictive maintenance is a stellar example. AI analytics processes sensor data to predict equipment failures before they occur, enabling proactive maintenance and minimizing downtime in sectors like manufacturing and logistics.
Natural language processing (NLP) is another facet of AI analytics, transforming the way users interact with data. NLP allows individuals to pose queries in everyday language, and AI-driven systems interpret and analyze the data to provide relevant insights. This capability is particularly valuable in customer service analytics, where automated systems can understand and respond to customer inquiries, enhancing user experiences.
Image recognition, a subset of AI analytics, finds applications in various industries such as healthcare and retail. In healthcare, AI-driven image analysis assists in the rapid diagnosis of medical imaging, improving the speed and accuracy of patient care. In retail, Data Analytics Tools processes images to understand customer behavior, optimize product placement, and enhance the overall shopping experience.
Fraud detection showcases the prowess of AI analytics in the financial sector. By analyzing patterns and anomalies in transaction data, AI algorithms can identify potentially fraudulent activities in real-time, bolstering security and protecting organizations and consumers alike.
AI analytics is a game-changer, automating data analytics processes and unlocking new possibilities across industries. From predictive maintenance to natural language processing and image recognition, the applications of AI analytics are diverse and transformative. As organizations continue to embrace automated data analytics with AI at its core, they position themselves at the forefront of innovation, ready to harness the full potential of their data for strategic advantage.
#Analytics Automation#Automated Data Analytics#data analytics platform#Automate Data Analysis#data analytics software
1 note
·
View note
Text
#marketing mix modeling#no code ai#automated data analytics#marketing mix modeling optimization#cpg marketing mix model#marketing mix model solution#no code ml#machine learning#marketing mix modeling mmm#no code machine learning
0 notes
Text
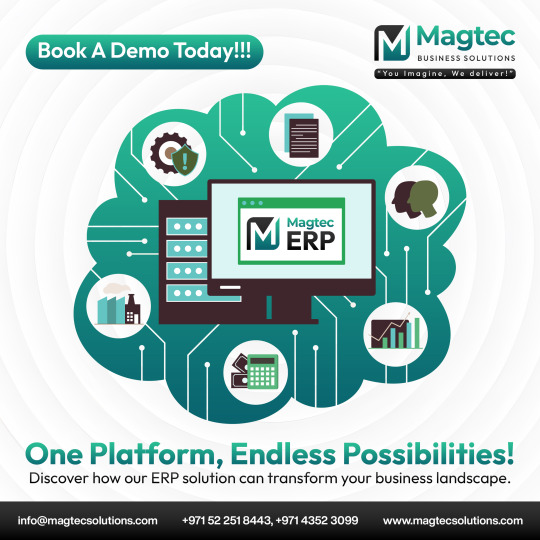
Transform your business with Magtec ERP! 🌐✨ Discover endless possibilities on a single platform. Book a demo today and see how we can elevate your operations to the next level! 🚀📈
#magtec#magtecerp#magtecsolutions#erp#businesssolutions#digitaltransformation#innovation#technology#growth#efficiency#productivity#cloud#automation#management#software#enterprise#success#analytics#customization#scalability#integration#teamwork#collaboration#strategy#data#support#consulting#businessdevelopment#transformation#leadership
3 notes
·
View notes
Text
Acadecraft Partners with Wadhwani Foundation's Government Digital Transformation Initiative to Develop eLearning Courses
#digitaltransformation#technology#innovation#business#digitalmarketing#ai#digital#artificialintelligence#software#machinelearning#automation#businessgrowth#tech#iot#techinnovation#bigdata#cybersecurity#cloud#data#cloudcomputing#smallbusiness#customerexperience#marketing#sap#webdevelopment#erp#blockchain#analytics#ecommerce#datascience
2 notes
·
View notes
Text
Top 5 DeepSeek AI Features Powering Industry Innovation
Table of Contents1. The Problem: Why Legacy Tools Can’t Keep Up2. What Makes DeepSeek AI Unique?3. 5 Game-Changing DeepSeek AI Features (with Real Stories)3.1 Adaptive Learning Engine3.2 Real-Time Anomaly Detection3.3 Natural Language Reports3.4 Multi-Cloud Sync3.5 Ethical AI Auditor4. How These Features Solve Everyday Challenges5. Step-by-Step: Getting Started with DeepSeek AI6. FAQs: Your…
#affordable AI solutions#AI automation#AI for educators#AI for entrepreneurs#AI for non-techies#AI for small business#AI in manufacturing#AI innovation 2024#AI time management#business growth tools#data-driven decisions#DeepSeek AI Features#ethical AI solutions#healthcare AI tools#no-code AI tools#Predictive Analytics#real-time analytics#remote work AI#retail AI features#startup AI tech
2 notes
·
View notes
Text
French initiative for responsible AI leaders - AI News
New Post has been published on https://thedigitalinsider.com/french-initiative-for-responsible-ai-leaders-ai-news/
French initiative for responsible AI leaders - AI News
ESSEC Business School and Accenture have announced the launch of a new initiative, ‘AI for Responsible Leadership,’ which marks the 10th anniversary of the establishment of the role of Chair at ESSEC, titled the ESSEC Accenture Strategic Business Analytics Chair.
The initiative aims to encourage the use of artificial intelligence by leaders in ways that are responsible and ethical, and that lead to high levels of professional performance. It aims to provide current and future leaders with the skills they require when faced with challenges in the future; economic, environmental, or social.
Several organisations support the initiative, including institutions, businesses, and specialised groups, including ESSEC Metalab for Data, Technology & Society, and Accenture Research.
Executive Director of the ESSEC Metalab, Abdelmounaim Derraz, spoke of the collaboration, saying, “Technical subjects are continuing to shake up business schools, and AI has opened up opportunities for collaboration between partner companies, researchers, and other members of the ecosystem (students, think tanks, associations, [and] public service).”
ESSEC and Accenture aim to integrate perspectives from multiple fields of expertise, an approach that is a result of experimentation in the decade the Chair has existed.
The elements of the initiative include workshops and talks designed to promote the exchange of knowledge and methods. It will also include a ‘barometer’ to help track AI’s implementation and overall impact on responsible leadership.
The initiative will engage with a network of institutions and academic publications, and an annual Grand Prix will recognise projects that focus on and explore the subject of AI and leadership.
Fabrice Marque, founder of the initiative and the current ESSEC Accenture Strategics Business Analytics Chair, said, “For years, we have explored the potential of using data and artificial intelligence in organisations. The synergies we have developed with our partners (Accenture, Accor, Dataiku, Engie, Eurofins, MSD, Orange) allowed us to evaluate and test innovative solutions before deploying them.
“With this initiative, we’re taking a major step: bringing together an engaged ecosystem to sustainably transform how leaders think, decide, and act in the face of tomorrow’s challenges. Our ambition is clear: to make AI a lever for performance, innovation and responsibility for […] leaders.”
Managing Director at Accenture and sponsor of the ESSEC/Accenture Chair and initiative, Aurélien Bouriot, said, “The ecosystem will benefit from the resources that Accenture puts at its disposal, and will also benefit our employees who participate.”
Laetitia Cailleteau, Managing Director at Accenture and leader of Responsible AI & Generative AI for Europe, highlighted the importance of future leaders understanding all aspects of AI.
“AI is a pillar of the ongoing industrial transformation. Tomorrow’s leaders must understand the technical, ethical, and human aspects and risks – and know how to manage them. In this way, they will be able to maximise value creation and generate a positive impact for the organisation, its stakeholders and society as a whole.”
Image credit: Wikimedia Commons
See also: Microsoft and OpenAI probe alleged data theft by DeepSeek
Want to learn more about AI and big data from industry leaders? Check out AI & Big Data Expo taking place in Amsterdam, California, and London. The comprehensive event is co-located with other leading events including Intelligent Automation Conference, BlockX, Digital Transformation Week, and Cyber Security & Cloud Expo.
Explore other upcoming enterprise technology events and webinars powered by TechForge here.
#accenture#ai#ai & big data expo#ai news#amp#Analytics#anniversary#approach#artificial#Artificial Intelligence#automation#Big Data#Business#business analytics#california#Cloud#Collaboration#Companies#comprehensive#conference#cyber#cyber security#data#data theft#deepseek#deploying#Digital Transformation#economic#education#employees
3 notes
·
View notes
Text
what u think, to much colour, or less?
https://sdesignt.threadless.com/
#tshirt#animals#design#rainbow#computer#Innovation#AI#Blockchain#Crypto#Tech#Digital#Data#BigData#Automation#Cloud#Cybersecurity#Startup#Entrepreneur#Leadership#Marketing#Business#Ecommerce#Content#Performance#Development#Research#Analytics#Growth#Productivity#Trend
4 notes
·
View notes
Text

𝐀𝐫𝐞 𝐘𝐨𝐮 𝐑𝐞𝐚𝐝𝐲 𝐭𝐨 𝐑𝐞𝐯𝐨𝐥𝐮𝐭𝐢𝐨𝐧𝐢𝐳𝐞 𝐘𝐨𝐮𝐫 𝐁𝐮𝐬𝐢𝐧𝐞𝐬𝐬? Discover Microsoft Dynamics 365 Business Central's game-changing power. 𝐃𝐢𝐬𝐜𝐨𝐯𝐞𝐫 𝟏𝟎 𝐠𝐚𝐦𝐞-𝐜𝐡𝐚𝐧𝐠𝐢𝐧𝐠 𝐟𝐞𝐚𝐭𝐮𝐫𝐞𝐬: ❇ Cloud-Based ERP ❇ Boosted Productivity ❇ Cost Avoidance ❇ Seamless Microsoft Integration ❇ Scalability ❇ Data-Driven Insights ❇ Simplified Customization ❇ Enhanced Employee Experience ❇ Revenue Growth Enablement ❇ Cost Transparency 𝗦𝘁𝗿𝗲𝗮𝗺𝗹𝗶𝗻𝗲 𝗼𝗽𝗲𝗿𝗮𝘁𝗶𝗼𝗻𝘀, 𝗮𝗺𝗽𝗹𝗶𝗳𝘆 𝗲𝗳𝗳𝗶𝗰𝗶𝗲𝗻𝗰𝘆 𝗮𝗻𝗱 𝗽𝗿𝗼𝗽𝗲𝗹 𝗴𝗿𝗼𝘄𝘁𝗵!
#DigitalTransformation#data analytics#custom software development#cloudcomputing#it consulting#artificial intelligence#automation#datascience#transformation
2 notes
·
View notes
Text
How Large Language Models (LLMs) are Transforming Data Cleaning in 2024
Data is the new oil, and just like crude oil, it needs refining before it can be utilized effectively. Data cleaning, a crucial part of data preprocessing, is one of the most time-consuming and tedious tasks in data analytics. With the advent of Artificial Intelligence, particularly Large Language Models (LLMs), the landscape of data cleaning has started to shift dramatically. This blog delves into how LLMs are revolutionizing data cleaning in 2024 and what this means for businesses and data scientists.
The Growing Importance of Data Cleaning
Data cleaning involves identifying and rectifying errors, missing values, outliers, duplicates, and inconsistencies within datasets to ensure that data is accurate and usable. This step can take up to 80% of a data scientist's time. Inaccurate data can lead to flawed analysis, costing businesses both time and money. Hence, automating the data cleaning process without compromising data quality is essential. This is where LLMs come into play.
What are Large Language Models (LLMs)?
LLMs, like OpenAI's GPT-4 and Google's BERT, are deep learning models that have been trained on vast amounts of text data. These models are capable of understanding and generating human-like text, answering complex queries, and even writing code. With millions (sometimes billions) of parameters, LLMs can capture context, semantics, and nuances from data, making them ideal candidates for tasks beyond text generation—such as data cleaning.
To see how LLMs are also transforming other domains, like Business Intelligence (BI) and Analytics, check out our blog How LLMs are Transforming Business Intelligence (BI) and Analytics.

Traditional Data Cleaning Methods vs. LLM-Driven Approaches
Traditionally, data cleaning has relied heavily on rule-based systems and manual intervention. Common methods include:
Handling missing values: Methods like mean imputation or simply removing rows with missing data are used.
Detecting outliers: Outliers are identified using statistical methods, such as standard deviation or the Interquartile Range (IQR).
Deduplication: Exact or fuzzy matching algorithms identify and remove duplicates in datasets.
However, these traditional approaches come with significant limitations. For instance, rule-based systems often fail when dealing with unstructured data or context-specific errors. They also require constant updates to account for new data patterns.
LLM-driven approaches offer a more dynamic, context-aware solution to these problems.

How LLMs are Transforming Data Cleaning
1. Understanding Contextual Data Anomalies
LLMs excel in natural language understanding, which allows them to detect context-specific anomalies that rule-based systems might overlook. For example, an LLM can be trained to recognize that “N/A” in a field might mean "Not Available" in some contexts and "Not Applicable" in others. This contextual awareness ensures that data anomalies are corrected more accurately.
2. Data Imputation Using Natural Language Understanding
Missing data is one of the most common issues in data cleaning. LLMs, thanks to their vast training on text data, can fill in missing data points intelligently. For example, if a dataset contains customer reviews with missing ratings, an LLM could predict the likely rating based on the review's sentiment and content.
A recent study conducted by researchers at MIT (2023) demonstrated that LLMs could improve imputation accuracy by up to 30% compared to traditional statistical methods. These models were trained to understand patterns in missing data and generate contextually accurate predictions, which proved to be especially useful in cases where human oversight was traditionally required.
3. Automating Deduplication and Data Normalization
LLMs can handle text-based duplication much more effectively than traditional fuzzy matching algorithms. Since these models understand the nuances of language, they can identify duplicate entries even when the text is not an exact match. For example, consider two entries: "Apple Inc." and "Apple Incorporated." Traditional algorithms might not catch this as a duplicate, but an LLM can easily detect that both refer to the same entity.
Similarly, data normalization—ensuring that data is formatted uniformly across a dataset—can be automated with LLMs. These models can normalize everything from addresses to company names based on their understanding of common patterns and formats.
4. Handling Unstructured Data
One of the greatest strengths of LLMs is their ability to work with unstructured data, which is often neglected in traditional data cleaning processes. While rule-based systems struggle to clean unstructured text, such as customer feedback or social media comments, LLMs excel in this domain. For instance, they can classify, summarize, and extract insights from large volumes of unstructured text, converting it into a more analyzable format.
For businesses dealing with social media data, LLMs can be used to clean and organize comments by detecting sentiment, identifying spam or irrelevant information, and removing outliers from the dataset. This is an area where LLMs offer significant advantages over traditional data cleaning methods.
For those interested in leveraging both LLMs and DevOps for data cleaning, see our blog Leveraging LLMs and DevOps for Effective Data Cleaning: A Modern Approach.

Real-World Applications
1. Healthcare Sector
Data quality in healthcare is critical for effective treatment, patient safety, and research. LLMs have proven useful in cleaning messy medical data such as patient records, diagnostic reports, and treatment plans. For example, the use of LLMs has enabled hospitals to automate the cleaning of Electronic Health Records (EHRs) by understanding the medical context of missing or inconsistent information.
2. Financial Services
Financial institutions deal with massive datasets, ranging from customer transactions to market data. In the past, cleaning this data required extensive manual work and rule-based algorithms that often missed nuances. LLMs can assist in identifying fraudulent transactions, cleaning duplicate financial records, and even predicting market movements by analyzing unstructured market reports or news articles.
3. E-commerce
In e-commerce, product listings often contain inconsistent data due to manual entry or differing data formats across platforms. LLMs are helping e-commerce giants like Amazon clean and standardize product data more efficiently by detecting duplicates and filling in missing information based on customer reviews or product descriptions.

Challenges and Limitations
While LLMs have shown significant potential in data cleaning, they are not without challenges.
Training Data Quality: The effectiveness of an LLM depends on the quality of the data it was trained on. Poorly trained models might perpetuate errors in data cleaning.
Resource-Intensive: LLMs require substantial computational resources to function, which can be a limitation for small to medium-sized enterprises.
Data Privacy: Since LLMs are often cloud-based, using them to clean sensitive datasets, such as financial or healthcare data, raises concerns about data privacy and security.

The Future of Data Cleaning with LLMs
The advancements in LLMs represent a paradigm shift in how data cleaning will be conducted moving forward. As these models become more efficient and accessible, businesses will increasingly rely on them to automate data preprocessing tasks. We can expect further improvements in imputation techniques, anomaly detection, and the handling of unstructured data, all driven by the power of LLMs.
By integrating LLMs into data pipelines, organizations can not only save time but also improve the accuracy and reliability of their data, resulting in more informed decision-making and enhanced business outcomes. As we move further into 2024, the role of LLMs in data cleaning is set to expand, making this an exciting space to watch.
Large Language Models are poised to revolutionize the field of data cleaning by automating and enhancing key processes. Their ability to understand context, handle unstructured data, and perform intelligent imputation offers a glimpse into the future of data preprocessing. While challenges remain, the potential benefits of LLMs in transforming data cleaning processes are undeniable, and businesses that harness this technology are likely to gain a competitive edge in the era of big data.
#Artificial Intelligence#Machine Learning#Data Preprocessing#Data Quality#Natural Language Processing#Business Intelligence#Data Analytics#automation#datascience#datacleaning#large language model#ai
2 notes
·
View notes
Text
From Zero to Hero: Mastering Data Analytics for Transformation
The concept of "data" has enormous significance in the modern digital world. The enormous amount of information produced every day has revolutionized the way we view the world and move through it. The data analytics industry is one of the major players in this revolution. Let's set out on an adventure to discover the fundamentals of data analytics, consider their importance, and understand how they affect different facets of our lives.
Understanding Data Analytics Analyzing massive data sets to find hidden patterns, correlations, trends, and insights is at the heart of data analytics. It's like sorting through a gold mine of knowledge to find the gold nuggets that will help you make decisions and solve problems. Data analytics isn't just about statistics and figures; it's about turning raw data into compelling narratives that encourage informed decisions.
Why Data Analysis Is Important
The capacity to make sense of all the data in the globe has changed the game. Data analytics enables companies, groups, and people to comprehend their operations, clients, and environments more thoroughly. Here are a few main arguments in favor of data analytics:
Making Informed Decisions: Data-driven insights allow for more precise and knowledgeable decision-making. Companies might modify their tactics in light of current developments and customer preferences.
Enhanced Efficiency: Data analytics uncovers bottlenecks and inefficiencies in processes and operations, allowing for focused changes.
Predictive Skills: By analyzing past data, predictive models can predict future patterns and aid organizations in becoming ready for the future.
Customer insights: Data analytics reveals the preferences and habits of customers, enabling more individualized interactions and higher levels of customer satisfaction.
Risk Management: Identifying potential risks and fraud patterns is made easier with data analytics, reducing vulnerabilities and losses.
Scientific Discoveries: In fields like healthcare and research, data analytics aids in uncovering groundbreaking insights and advancements.
The Data Analytics Process
Data analytics involves a systematic approach that follows several key steps:
Defining Objectives: Clearly state the objectives and issues you hope to resolve through data analysis.
Data Collection: Collect pertinent data from a variety of sources, making sure it is reliable and representative. Data Cleaning: Involves altering it to ensure accurate analysis.
Exploratory Analysis: Investigate the data in depth to find patterns, trends, and early insights.
Modeling: Building statistical models or algorithms to forecast outcomes or comprehend correlations in the data is known as modeling.
Interpretation: Draw conclusions from your analysis's findings and connect them to your original goals.
Visualization: To make your findings easier to understand, present them using visual tools like charts, graphs, and dashboards.
Action: Implement the learnings from data analysis to inspire positive changes and actions.
Data analytics has become an effective means for turning data into useful insights. By bridging the gap between information overload and useful knowledge, it facilitates decision-making, ignites innovation, and opens doors. A fascinating and fruitful trip may be had by anyone interested in learning more about data analytics, whether they are a business owner, researcher, or just curious. So, take part in uncovering the secrets of the data and grasp the power of data analytics to transform. If you need help implementing data analytics in your organization or if you want to learn more, you should consult professionals or sign up for specialized courses. For instance, the ACTE Institute offers comprehensive data analytics training courses that can provide you the knowledge and skills you need.
7 notes
·
View notes
Text
1 note
·
View note
Text
IoT in Action: Transforming Industries with Intelligent Connectivity
The Power of Connectivity
The Internet of Things (IoT) has become a cornerstone of innovation, as it reimagines industries and redefines the way business is conducted. In bridging the physical and digital worlds, IoT enables seamless connectivity, smarter decision-making, and unprecedented efficiency. Today, in the competitive landscape, intelligent connectivity is no longer just a technology advancement; for businesses wanting to be relevant and continue to thrive, it is now a strategic imperative.
IoT is not simply about connecting devices; it’s about creating ecosystems that work collaboratively to drive value. With industries relying heavily on real-time data and actionable insights, IoT-powered connectivity has become the backbone of operational excellence and growth. Let’s explore how this transformative technology is revolutionizing key sectors, with a focus on how businesses can leverage it effectively.
Applications of IoT in Key Industries
1.Smart Manufacturing: Efficiency Through Connectivity
Manufacturing has embraced IoT as a tool to streamline operations and boost productivity. By embedding sensors in machinery and integrating real-time monitoring systems, manufacturers can:
Predict and Prevent Downtime: IoT-enabled predictive maintenance reduces unplanned outages, saving time and money.
Optimize Resource Allocation: Smart systems track inventory, raw materials, and energy consumption, ensuring optimal usage.
Enhance Quality Control: Real-time data from production lines helps identify defects early, maintaining high-quality standards.
Example: A global automotive manufacturer integrated IoT sensors into its assembly lines, reducing equipment downtime by 25% and improving production efficiency by 30%. The ability to monitor machinery health in real time transformed their operations, delivering significant cost savings.
2.Healthcare: Improve Patient Outcomes
In healthcare, IoT has been a game-changer in enabling connected medical devices and systems that enhance patient care and operational efficiency. The main applications include:
Remote Patient Monitoring: Devices track vital signs in real time, allowing healthcare providers to offer timely interventions.
Smart Hospital Systems: IoT-enabled equipment and sensors optimize resource utilization, from patient beds to medical supplies.
Data-Driven Decisions: IoT integrates patient data across systems, providing actionable insights for personalized treatment plans.
Example: A major hospital has put into operation IoT-enabled wearables for chronic disease management. This solution reduced the number of readmissions to hospitals by 20% and empowered patients to take an active role in their health.
3.Retail: Revolutionizing Customer Experiences
IoT is revolutionizing retail through increased customer interaction and streamlined operations. Connected devices and smart analytics allow retailers to:
Personalize Shopping Experiences: IoT systems track customer preferences, offering tailored recommendations in real time.
Improve Inventory Management: Smart shelves and sensors keep stock levels optimal, reducing wastage and improving availability.
Enable Smooth Transactions: IoT-driven payment systems make checkout easier and much faster, increasing customers’ convenience
Example: A retail chain leveraged IoT to integrate smart shelves that automatically update inventory data. This reduced out-of-stock situations by 40%, improving customer satisfaction and driving higher sales.
Role of Intelligent Connectivity in Business Transformation
Intelligent connectivity lies at the heart of IoT’s transformative potential. By connecting devices, systems, and processes, businesses can:
Accelerate Decision-Making: Real-time data sharing enables faster, more informed decisions, giving companies a competitive edge.
It increases collaboration by allowing smooth communication between departments and teams, making the entire system more efficient.
Adapt to Market Dynamics: IoT enables companies to respond quickly to changes in demand, supply chain disruptions, or operational challenges.
Intelligent connectivity is not just about technology; it’s about creating value by aligning IoT solutions with business objectives. This strategic approach guarantees that IoT investments will deliver measurable outcomes, from cost savings to improved customer loyalty.
How Tudip Technologies Powers Intelligent Connectivity
Tudip Technologies specializes in designing and implementing IoT solutions that drive meaningful transformation for businesses. With a focus on innovation and collaboration, Tudip ensures that its clients achieve operational excellence through intelligent connectivity.
Tailored Solution for Every Business Industry
Tudip understands that no two businesses are alike. By customizing IoT strategies to address specific challenges, Tudip helps clients unlock the full potential of connectivity. Examples include:
Smart Supply Chains: Implementing IoT systems that provide real-time visibility into inventory and logistics, reducing delays and improving efficiency.
Energy Management: Developing IoT frameworks to monitor and optimize energy usage, driving sustainability and cost savings.
Healthcare Innovations: Designing networked medical devices that allow remote patient monitoring and data integration without a hitch.
The Future of Connected Systems
The demand for intelligent connectivity will keep increasing as the industries continue to evolve. Emerging trends in IoT include edge computing, 5G networks, and AI-powered analytics, which promise to redefine possibilities for connected ecosystems.
Businesses that embrace these advancements stand to gain:
Greater Resilience: IoT enables adaptive systems that can withstand market fluctuations and operational challenges.
Enhanced Innovation: Connected technologies open doors to new business models, revenue streams, and customer experiences.
Sustainable Growth: IoT optimizes resources and processes, contributing to long-term environmental and economic sustainability.
The future belongs to those who see connectivity not just as a technological tool but as a strategic enabler of transformation. The right partner will help businesses transform IoT from a concept into a competitive advantage.
Conclusion: Embracing Intelligent Connectivity with Tudip
IoT is not just changing the way businesses operate—it’s redefining what’s possible. From manufacturing and healthcare to retail and beyond, intelligent connectivity is driving innovation, efficiency, and growth across industries.
Tudip Technologies is at the forefront of this transformation, offering customized IoT solutions that deliver real results. By prioritizing collaboration, adaptability, and measurable outcomes, Tudip ensures that its clients stay ahead in an increasingly connected world.
Now is the time to embrace the power of IoT and unlock its potential for your business. With Tudip as your partner, the journey to intelligent connectivity is not just achievable—it’s inevitable.
Click the link below to learn more about the blog IoT in Action: Transforming Industries with Intelligent Connectivity https://tudip.com/blog-post/iot-in-action-transforming-industries-with-intelligent-connectivity/
#Tudip#IoT#intelligent connectivity#real-time data#predictive maintenance#smart manufacturing#remote patient monitoring#healthcare IoT#retail IoT#smart shelves#supply chain optimization#edge computing#AI-powered analytics#5G networks#industrial IoT#connected devices#digital transformation#operational efficiency#business intelligence#automation#data-driven decision-making#IoT solutions#smart systems#enterprise IoT#IoT-powered connectivity#sustainable growth#technology innovation#machine learning#cloud computing#smart sensors
0 notes
Text
#marketing mix modeling#no code ai#marketing mix modeling mmm#marketing mix modeling optimization#no code machine learning#automated data analytics#cpg marketing mix model#no code ml#marketing mix model solution#machine learning
0 notes
Text
Qatar Partners With Scale AI for AI-Powered Digital Transformation of Government Services
#digitaltransformation#technology#innovation#business#digitalmarketing#ai#digital#artificialintelligence#software#machinelearning#automation#businessgrowth#tech#iot#techinnovation#bigdata#cybersecurity#cloud#data#cloudcomputing#smallbusiness#customerexperience#marketing#sap#webdevelopment#erp#blockchain#analytics#ecommerce#datascience
2 notes
·
View notes
Text
Ultimate Guide to DeepSeek AI for Business Growth
Table of Contents of DeepSeek AI for Business Growth1. Introduction: Why AI is Essential for Modern Business Growth2. What Is DeepSeek AI?3. Top 5 DeepSeek AI Tools for Scaling Businesses3.1 Demand Forecasting Engine3.2 Customer Lifetime Value (CLV) Predictor3.3 Automated Supply Chain Optimizer3.4 Dynamic Pricing Module3.5 Sentiment Analysis Hub4. How DeepSeek AI Reduces Costs and Boosts…
#AI automation 2024#AI budgeting#AI business growth#AI for non-tech teams#AI for startups#AI implementation guide#AI in retail#AI supply chain#Business Intelligence#cost reduction strategies#data-driven decisions#DeepSeek AI#enterprise AI adoption#fintech AI solutions#generative AI for business#Predictive Analytics#ROI optimization#scaling with AI#SME AI tools#startup scaling
2 notes
·
View notes
Text
Future-Ready Enterprises: The Crucial Role of Large Vision Models (LVMs)
New Post has been published on https://thedigitalinsider.com/future-ready-enterprises-the-crucial-role-of-large-vision-models-lvms/
Future-Ready Enterprises: The Crucial Role of Large Vision Models (LVMs)
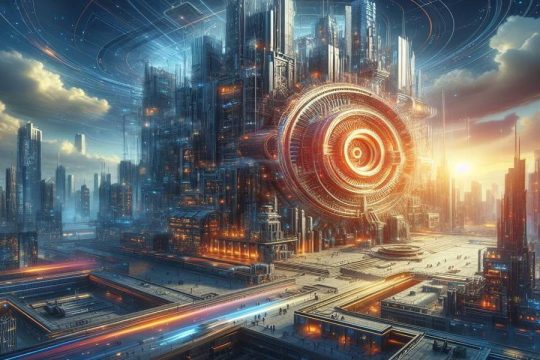
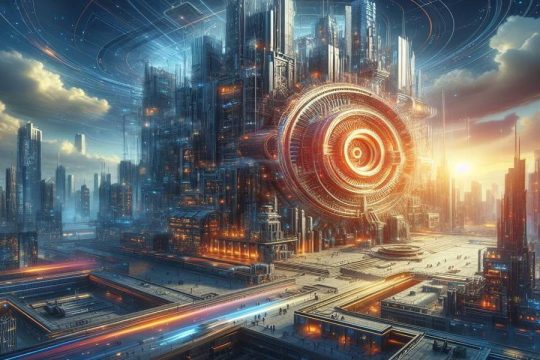
What are Large Vision Models (LVMs)
Over the last few decades, the field of Artificial Intelligence (AI) has experienced rapid growth, resulting in significant changes to various aspects of human society and business operations. AI has proven to be useful in task automation and process optimization, as well as in promoting creativity and innovation. However, as data complexity and diversity continue to increase, there is a growing need for more advanced AI models that can comprehend and handle these challenges effectively. This is where the emergence of Large Vision Models (LVMs) becomes crucial.
LVMs are a new category of AI models specifically designed for analyzing and interpreting visual information, such as images and videos, on a large scale, with impressive accuracy. Unlike traditional computer vision models that rely on manual feature crafting, LVMs leverage deep learning techniques, utilizing extensive datasets to generate authentic and diverse outputs. An outstanding feature of LVMs is their ability to seamlessly integrate visual information with other modalities, such as natural language and audio, enabling a comprehensive understanding and generation of multimodal outputs.
LVMs are defined by their key attributes and capabilities, including their proficiency in advanced image and video processing tasks related to natural language and visual information. This includes tasks like generating captions, descriptions, stories, code, and more. LVMs also exhibit multimodal learning by effectively processing information from various sources, such as text, images, videos, and audio, resulting in outputs across different modalities.
Additionally, LVMs possess adaptability through transfer learning, meaning they can apply knowledge gained from one domain or task to another, with the capability to adapt to new data or scenarios through minimal fine-tuning. Moreover, their real-time decision-making capabilities empower rapid and adaptive responses, supporting interactive applications in gaming, education, and entertainment.
How LVMs Can Boost Enterprise Performance and Innovation?
Adopting LVMs can provide enterprises with powerful and promising technology to navigate the evolving AI discipline, making them more future-ready and competitive. LVMs have the potential to enhance productivity, efficiency, and innovation across various domains and applications. However, it is important to consider the ethical, security, and integration challenges associated with LVMs, which require responsible and careful management.
Moreover, LVMs enable insightful analytics by extracting and synthesizing information from diverse visual data sources, including images, videos, and text. Their capability to generate realistic outputs, such as captions, descriptions, stories, and code based on visual inputs, empowers enterprises to make informed decisions and optimize strategies. The creative potential of LVMs emerges in their ability to develop new business models and opportunities, particularly those using visual data and multimodal capabilities.
Prominent examples of enterprises adopting LVMs for these advantages include Landing AI, a computer vision cloud platform addressing diverse computer vision challenges, and Snowflake, a cloud data platform facilitating LVM deployment through Snowpark Container Services. Additionally, OpenAI, contributes to LVM development with models like GPT-4, CLIP, DALL-E, and OpenAI Codex, capable of handling various tasks involving natural language and visual information.
In the post-pandemic landscape, LVMs offer additional benefits by assisting enterprises in adapting to remote work, online shopping trends, and digital transformation. Whether enabling remote collaboration, enhancing online marketing and sales through personalized recommendations, or contributing to digital health and wellness via telemedicine, LVMs emerge as powerful tools.
Challenges and Considerations for Enterprises in LVM Adoption
While the promises of LVMs are extensive, their adoption is not without challenges and considerations. Ethical implications are significant, covering issues related to bias, transparency, and accountability. Instances of bias in data or outputs can lead to unfair or inaccurate representations, potentially undermining the trust and fairness associated with LVMs. Thus, ensuring transparency in how LVMs operate and the accountability of developers and users for their consequences becomes essential.
Security concerns add another layer of complexity, requiring the protection of sensitive data processed by LVMs and precautions against adversarial attacks. Sensitive information, ranging from health records to financial transactions, demands robust security measures to preserve privacy, integrity, and reliability.
Integration and scalability hurdles pose additional challenges, especially for large enterprises. Ensuring compatibility with existing systems and processes becomes a crucial factor to consider. Enterprises need to explore tools and technologies that facilitate and optimize the integration of LVMs. Container services, cloud platforms, and specialized platforms for computer vision offer solutions to enhance the interoperability, performance, and accessibility of LVMs.
To tackle these challenges, enterprises must adopt best practices and frameworks for responsible LVM use. Prioritizing data quality, establishing governance policies, and complying with relevant regulations are important steps. These measures ensure the validity, consistency, and accountability of LVMs, enhancing their value, performance, and compliance within enterprise settings.
Future Trends and Possibilities for LVMs
With the adoption of digital transformation by enterprises, the domain of LVMs is poised for further evolution. Anticipated advancements in model architectures, training techniques, and application areas will drive LVMs to become more robust, efficient, and versatile. For example, self-supervised learning, which enables LVMs to learn from unlabeled data without human intervention, is expected to gain prominence.
Likewise, transformer models, renowned for their ability to process sequential data using attention mechanisms, are likely to contribute to state-of-the-art outcomes in various tasks. Similarly, Zero-shot learning, allowing LVMs to perform tasks they have not been explicitly trained on, is set to expand their capabilities even further.
Simultaneously, the scope of LVM application areas is expected to widen, encompassing new industries and domains. Medical imaging, in particular, holds promise as an avenue where LVMs could assist in the diagnosis, monitoring, and treatment of various diseases and conditions, including cancer, COVID-19, and Alzheimer’s.
In the e-commerce sector, LVMs are expected to enhance personalization, optimize pricing strategies, and increase conversion rates by analyzing and generating images and videos of products and customers. The entertainment industry also stands to benefit as LVMs contribute to the creation and distribution of captivating and immersive content across movies, games, and music.
To fully utilize the potential of these future trends, enterprises must focus on acquiring and developing the necessary skills and competencies for the adoption and implementation of LVMs. In addition to technical challenges, successfully integrating LVMs into enterprise workflows requires a clear strategic vision, a robust organizational culture, and a capable team. Key skills and competencies include data literacy, which encompasses the ability to understand, analyze, and communicate data.
The Bottom Line
In conclusion, LVMs are effective tools for enterprises, promising transformative impacts on productivity, efficiency, and innovation. Despite challenges, embracing best practices and advanced technologies can overcome hurdles. LVMs are envisioned not just as tools but as pivotal contributors to the next technological era, requiring a thoughtful approach. A practical adoption of LVMs ensures future readiness, acknowledging their evolving role for responsible integration into business processes.
#Accessibility#ai#Alzheimer's#Analytics#applications#approach#Art#artificial#Artificial Intelligence#attention#audio#automation#Bias#Business#Cancer#Cloud#cloud data#cloud platform#code#codex#Collaboration#Commerce#complexity#compliance#comprehensive#computer#Computer vision#container#content#covid
2 notes
·
View notes