#data science with tableau
Explore tagged Tumblr posts
Text
Why Tableau is Essential in Data Science: Transforming Raw Data into Insights
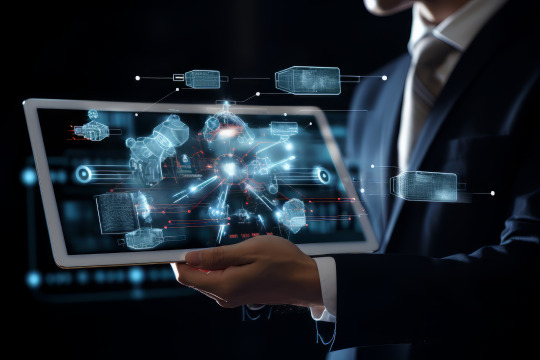
Data science is all about turning raw data into valuable insights. But numbers and statistics alone don’t tell the full story—they need to be visualized to make sense. That’s where Tableau comes in.
Tableau is a powerful tool that helps data scientists, analysts, and businesses see and understand data better. It simplifies complex datasets, making them interactive and easy to interpret. But with so many tools available, why is Tableau a must-have for data science? Let’s explore.
1. The Importance of Data Visualization in Data Science
Imagine you’re working with millions of data points from customer purchases, social media interactions, or financial transactions. Analyzing raw numbers manually would be overwhelming.
That’s why visualization is crucial in data science:
Identifies trends and patterns – Instead of sifting through spreadsheets, you can quickly spot trends in a visual format.
Makes complex data understandable – Graphs, heatmaps, and dashboards simplify the interpretation of large datasets.
Enhances decision-making – Stakeholders can easily grasp insights and make data-driven decisions faster.
Saves time and effort – Instead of writing lengthy reports, an interactive dashboard tells the story in seconds.
Without tools like Tableau, data science would be limited to experts who can code and run statistical models. With Tableau, insights become accessible to everyone—from data scientists to business executives.
2. Why Tableau Stands Out in Data Science
A. User-Friendly and Requires No Coding
One of the biggest advantages of Tableau is its drag-and-drop interface. Unlike Python or R, which require programming skills, Tableau allows users to create visualizations without writing a single line of code.
Even if you’re a beginner, you can:
✅ Upload data from multiple sources
✅ Create interactive dashboards in minutes
✅ Share insights with teams easily
This no-code approach makes Tableau ideal for both technical and non-technical professionals in data science.
B. Handles Large Datasets Efficiently
Data scientists often work with massive datasets—whether it’s financial transactions, customer behavior, or healthcare records. Traditional tools like Excel struggle with large volumes of data.
Tableau, on the other hand:
Can process millions of rows without slowing down
Optimizes performance using advanced data engine technology
Supports real-time data streaming for up-to-date analysis
This makes it a go-to tool for businesses that need fast, data-driven insights.
C. Connects with Multiple Data Sources
A major challenge in data science is bringing together data from different platforms. Tableau seamlessly integrates with a variety of sources, including:
Databases: MySQL, PostgreSQL, Microsoft SQL Server
Cloud platforms: AWS, Google BigQuery, Snowflake
Spreadsheets and APIs: Excel, Google Sheets, web-based data sources
This flexibility allows data scientists to combine datasets from multiple sources without needing complex SQL queries or scripts.
D. Real-Time Data Analysis
Industries like finance, healthcare, and e-commerce rely on real-time data to make quick decisions. Tableau’s live data connection allows users to:
Track stock market trends as they happen
Monitor website traffic and customer interactions in real time
Detect fraudulent transactions instantly
Instead of waiting for reports to be generated manually, Tableau delivers insights as events unfold.
E. Advanced Analytics Without Complexity
While Tableau is known for its visualizations, it also supports advanced analytics. You can:
Forecast trends based on historical data
Perform clustering and segmentation to identify patterns
Integrate with Python and R for machine learning and predictive modeling
This means data scientists can combine deep analytics with intuitive visualization, making Tableau a versatile tool.
3. How Tableau Helps Data Scientists in Real Life
Tableau has been adopted by the majority of industries to make data science more impactful and accessible. This is applied in the following real-life scenarios:
A. Analytics for Health Care
Tableau is deployed by hospitals and research institutions for the following purposes:
Monitor patient recovery rates and predict outbreaks of diseases
Analyze hospital occupancy and resource allocation
Identify trends in patient demographics and treatment results
B. Finance and Banking
Banks and investment firms rely on Tableau for the following purposes:
✅ Detect fraud by analyzing transaction patterns
✅ Track stock market fluctuations and make informed investment decisions
✅ Assess credit risk and loan performance
C. Marketing and Customer Insights
Companies use Tableau to:
✅ Track customer buying behavior and personalize recommendations
✅ Analyze social media engagement and campaign effectiveness
✅ Optimize ad spend by identifying high-performing channels
D. Retail and Supply Chain Management
Retailers leverage Tableau to:
✅ Forecast product demand and adjust inventory levels
✅ Identify regional sales trends and adjust marketing strategies
✅ Optimize supply chain logistics and reduce delivery delays
These applications show why Tableau is a must-have for data-driven decision-making.
4. Tableau vs. Other Data Visualization Tools
There are many visualization tools available, but Tableau consistently ranks as one of the best. Here’s why:
Tableau vs. Excel – Excel struggles with big data and lacks interactivity; Tableau handles large datasets effortlessly.
Tableau vs. Power BI – Power BI is great for Microsoft users, but Tableau offers more flexibility across different data sources.
Tableau vs. Python (Matplotlib, Seaborn) – Python libraries require coding skills, while Tableau simplifies visualization for all users.
This makes Tableau the go-to tool for both beginners and experienced professionals in data science.
5. Conclusion
Tableau has become an essential tool in data science because it simplifies data visualization, handles large datasets, and integrates seamlessly with various data sources. It enables professionals to analyze, interpret, and present data interactively, making insights accessible to everyone—from data scientists to business leaders.
If you’re looking to build a strong foundation in data science, learning Tableau is a smart career move. Many data science courses now include Tableau as a key skill, as companies increasingly demand professionals who can transform raw data into meaningful insights.
In a world where data is the driving force behind decision-making, Tableau ensures that the insights you uncover are not just accurate—but also clear, impactful, and easy to act upon.
#data science course#top data science course online#top data science institute online#artificial intelligence course#deepseek#tableau
3 notes
·
View notes
Text

Day 3/100 days of productivity | Wed 21 Feb, 2024
Long day at work, chatted with colleagues about career paths in data science, met with my boss for a performance evaluation (it went well!). Worked on writing some Quarto documentation for how to build one of my Tableau dashboards, since it keeps breaking and I keep referring to my notes 😳
In non-work productivity, I completed the ‘Logistic Regression as a Neural Network’ module of Andrew Ng’s Deep Learning course on Coursera and refreshed my understanding of backpropagation using the calculus chain rule. Fun!
#100 days of productivity#data science#deep learning#neural network#quarto#tableau#note taking#chain rule
3 notes
·
View notes
Text
#power bi course fees#Power BI#tableau#visualization#best data science courses to get a job#data analytics jobs
0 notes
Text
DATA ANALYTICS COURSE IN GANGTOK
Upgrade your career with DataMites Data Analytics Course in Gurgaon. Learn Python, SQL, Power BI, Tableau, and Machine Learning with expert-led training. Get hands-on experience, industry certification, and internship & job support. Limited-time 65% fee discount!
#Data Analytics Training Gangtok#Data Analytics Certification Gangtok#Best Data Analytics Course in Gangtok#Python for Data Analytics Gangtok#Power BI Training Gangtok#Tableau Course Gangtok#Data Science Training Gangtok#Machine Learning Course Gangtok#Internship in Data Analytics Gangtok
0 notes
Text
JUST PLACED AT PALLADIUM.
#data science#data analytics#business analytics#advance excel#power bi#tableau#sql#python#machine learning#ai technology
1 note
·
View note
Text

The Basics of Data Visualization | Why It Matters in Data Science
Data visualization is the art of representing data in a visual format, using graphs and charts. It eases the interpretation of complex datasets, enabling the viewer to recognize patterns, trends, and insights more quickly. In data science, data visualization enables a better communication of findings to allow for better decision-making. This form of communication comes into play by presenting information so that there is minimal space between raw data and useful insights. Data visualization tools also enable data scientists to easily identify outliers, relationships, and key metrics so that the overall analysis process is sped up while increasing audience engagement.
Importance of data visualization in data science
Data visualization is an integral part of data science as it involves translating data into a visual representation so that users can perceive the data being analyzed and report their insights. Different aspects of the importance of data visualization in the modern-day can be described as follows: Transforms data into its simplest form: Complex datasets are generally made easily understandable with the help of different visuals, such as graphs and charts, so that any pattern or trend can be easily laid out in front of the audiences. Unveils trends and patterns: This reveals the hidden insights like correlations or an anomaly-inducing trend, which serve as a precise basis for prediction and anomaly detection. Helps in exploration: Such interactive visuals are never short of discretionary privilege to dig deeper into the data, thus enhancing insights. Partial facilitating communication: Support is provided in the interpretation of complex findings to non-technical stakeholders and thus better decision-making. Supports better decision-making: A visual representation lets the decision be made quickly and correctly through informed integration of key insights. Facilitates storytelling: Data visualization tells a story that impresses, thus rendering the data relatable and actionable. Indicates areas for improvement: The visual nature of metric tracking makes it easy to pinpoint where issues exist and where improvements are necessary.
Interactive Dashboards: Tools such as Tableau and Power BI offer purpose-based dashboards so that users have the autonomy to explore their data and derive insight from it.
Benefits of Data Visualization in Data Science
Faster Data Processing: Visuals get processed faster compared to text or numbers when presented to the human brain. Enhanced Communication: This aids data scientists in presenting analytical insights in a way that the non-technical stakeholders can easily understand. Better Decision-Making: Has helped businesses to firmly base their decisions on the data. Find patterns and outliers: Visuals make the process of spotting the trends and anomalies in data much easier. Promotes data exploration: Interactive visualizations allow thorough exploration of data.
Data Visualization Tools: Simplifying Insights
Data visualization tools support converting data sets that contain high complexity into interactively clear visuals, allowing businesses to sift through data and uncover insights speedily to make quick business decisions. They offer the functionality of automation of chart-making; they pull data from data sources; they feature dashboards; and they provide templates.
Tools:
Tableau: Powerful yet user-friendly analytics.
Power BI: Microsoft’s tool for seamless reporting.
Looker Studio: Free tool for interactive dashboards.
QlikView: Intuitive data exploration.
Matplotlib: It is python based visualization, mostly used in data science
Plotly: Supports Python, R, and JavaScript.
Benefits:
Better Decisions: Easily identify trends and patterns.
Improved Communication: Present complex data clearly.
Efficiency: Automate visualization and reduce errors.
Real-Time Insights: Stay updated with live data.
Conclusion:
Data visualization is the approach that bridges the gap between raw data and insights. Transforming complex datasets into easy-to-understand graphs, charts, and dashboards allows faster data interpretation, clearer communication, and sounder decision-making. More fundamentally, data visualization creates the means to explore the underlying trends and patterns in the data and articulate a successful visualization-based narrative with it. It not only simplifies complex findings for non-technical stakeholders, but it's also a strong enablement to act on data-driven insights for businesses. The likes of Tableau, Power BI, and Matplotlib allow for the easiest process of visually appealing and interactive data representation. Suffice to say, data visualization is the lifeblood of modern-day enterprises and data scientists; a clear lens to cut through the unwieldy nature of big data empowers much smarter and more far-reaching decision-making..
1 note
·
View note
Text
Free Data Science Courses to Build Your Career Skills

Introduction
Data science is transforming industries, making it one of the most in-demand skills in today's job market. With the global data science platform market projected to grow from $95.3 billion in 2021 to $322.9 billion by 2026 at a staggering CAGR of 27.7%, professionals with data expertise are in high demand.
Free data science courses provide an excellent opportunity to upskill without financial barriers. They enable you to learn data science for free, covering everything from basic concepts to advanced techniques. This blog highlights top free resources, helping you kickstart or advance your career in this rapidly growing field.
Top Free Data Science Courses

Data Science is evolving rapidly, and finding quality resources for learning without paying is now easier than ever. Whether you’re new to Data Science or looking to expand your knowledge in specialised areas like Machine Learning or Data Visualisation, free online courses offer a fantastic opportunity to learn at your own pace.
Here’s a comprehensive guide to the top free Data Science courses available. Each course provides a unique focus, giving you options to build your Data Science skills effectively and affordably.
Applied Data Science by Future Learn
Future Learn’s Applied Data Science course collaborates with Coventry University, the Institute of Coding, and Birkbeck University to introduce students to the practical aspects of Data Science. This beginner-friendly course emphasises Data Visualisation, Machine Learning applications, and clustering techniques.
Key Features
User-Friendly Platform: Future Learn offers an intuitive experience with flexible schedules and engaging video-based learning.
Focus on Applied Data Science: Practical examples help users apply Data Science methods to real-world business cases.
Self-paced Learning: Students can start anytime and learn at their speed.
Certificate Option: Obtain a certificate for an additional 6,222 ₹.
Accessible Topics: Covers geolocation data, feature extraction, and introductory Machine Learning.
Data Science Professional Certificate by IBM on Coursera
The IBM Data Science Professional Certificate on Coursera offers an in-depth curriculum spanning nine courses. This specialisation takes about 11 months to complete. It includes a capstone project designed to simulate real-world data challenges, making it one of the most comprehensive free courses in Data Science.
Key Features
Nine-Course Structure: Covers everything from basic Data Science concepts to advanced SQL and Python.
Capstone Project: Provides a simulated real-world experience.
Certificate Upon Completion: Receive a shareable certificate on Coursera upon successful completion.
Excellent Instruction Quality: Taught by experienced IBM professionals.
Career-Boosting Potential: Many students use this course as a launchpad for Data Science careers.
Data Science Course by Pickl.AI
Pickl.AI’s Data Science Course is structured into 11 modules, covering everything from SQL and Tableau to Machine Learning techniques. With hands-on exercises and real-world case studies, this course is designed for beginners and professionals looking to advance in Data Science.
Key Features
Extensive Modules: Includes 462 lessons on SQL, Python, Machine Learning, and more.
Real-Life Case Studies: Learn through practical examples and case studies.
Certification Available: Certification offered upon completion, enhancing employability.
Focus on Data Science Tools: Access high-demand tools like Tableau and Power BI.
Flexible Pace: Allows learners to move through modules at their own speed.
Introduction to Data Science by Alison
Alison’s Introduction to Data Science course is a quick, impactful introduction to core Data Science concepts. It’s highly rated among working professionals and provides insights into data structuring, Machine Learning basics, and essential Data Science processes.
Key Features
Quick Overview: Completes in 1.5 to 3 hours, ideal for busy professionals.
Beginner-Friendly: No prior knowledge is required, making it accessible to everyone.
Certification Included: Receive a certificate upon completion, adding value to resumes.
Focus on Practical Skills: Learn data extraction, modelling, and scientific methods.
User-Friendly Platform: Alison is known for its ease of use and career-focused course design.
Data Science and Machine Learning with Python by Skillshare
Skillshare’s Data Science and Machine Learning with Python course offers an immersive look into Python, regression models, and ensemble learning, with a two-week free trial for new users. Frank Kane, an expert with over nine years of experience at Amazon and IMDB, leads this course, making it an excellent option for in-depth Python training.
Key Features
Access with Free Trial: Skillshare’s two-week free trial allows full access to the course.
Professional Instructor: Frank Kane brings practical experience and industry insights.
In-Depth Python Training: Covers foundational Python skills and data manipulation.
Beginner-Friendly: No prior knowledge is needed to start.
10-Hour Format: Perfect balance of depth and accessibility, making it manageable for beginners.
Conclusion
Free data science courses provide an excellent pathway to upskilling and breaking financial barriers. With platforms like Future Learn, IBM, and Pickl.AI offering specialised modules, learners can grasp core concepts, explore advanced techniques, and boost their career potential. Start today and embrace the growing demand for data science skills in this dynamic, evolving industry.
0 notes
Text
This tutorial video explains all types of filtering available in Tableau. Its uses with practical examples are shown.
0 notes
Text
Data Analytics Toolbox: Essential Skills to Master by 2025
As data continues to drive decision-making in every business, mastering data analytics becomes more important than ever for ambitious professionals. Students preparing to enter this dynamic sector must have a firm foundation in the necessary tools and abilities. Here, we describe the most important data analytics skills to learn in 2025, explain their significance, and provide a road map for building a versatile and relevant analytics toolkit.
1. Programming languages: Python and R
Python and R are the two most popular programming languages in data analytics, with each having distinct strengths and capabilities.
Python: The preferred language for data analysis, data manipulation, and machine learning, Python is well-known for its readability, adaptability, and extensive library. Libraries like Scikit-Learn for machine learning, NumPy for numerical calculations, and Pandas for data manipulation give analysts the strong tools they need to work effectively with big datasets.
R: Widely used in research and academia, R is used for data visualisation and statistical analysis. It is a strong choice for statistical analysis and for producing detailed, publication-ready visualizations thanks to its packages, like ggplot2 for visualization and dplyr for data processing.
Why It Matters: Students who are proficient in Python and R are able to manage a variety of analytical activities. While R's statistical capabilities can improve analysis, especially in professions that focus on research, Python is particularly useful for general-purpose data analytics.
2. Structured Query Language, or SQL
Data analysts can efficiently retrieve and manage data by using SQL, a fundamental ability for querying and maintaining relational databases.
SQL Fundamentals: Data analysts can manipulate data directly within databases by mastering the core SQL commands (SELECT, INSERT, UPDATE, and DELETE), which are necessary for retrieving and analyzing data contained in relational databases.
Advanced SQL Techniques: When working with structured data, SQL is a tremendous help. Proficiency in JOIN operations (for merging tables), window functions, and subqueries is essential for more complicated data chores.
Why It Matters: The main tool for retrieving and examining data kept in relational databases is SQL. Since almost all organizations store their data in SQL-based systems, analysts in nearly every data-focused position must be proficient in SQL.
3. Data Preparation and Cleaning
Cleaning, converting, and organizing data for analysis is known as "data wrangling," or data preparation, and it is an essential first step in the analytics process.
Managing Outliers and Missing Values: Accurate analysis relies on knowing how to handle outliers and missing values.
Data Transformation Techniques: By ensuring that data is in a format that machine learning algorithms can understand, abilities like normalization, standardization, and feature engineering serve to improve model accuracy.
Why It Matters: Analysts invest a lot of effort on cleaning and preparing data for any data analytics project. An accurate, reliable, and error-free analysis is guaranteed by efficient data preparation.
4. Visualization of Data
Complex datasets are transformed into understandable, relevant pictures through data visualization, which facilitates narrative and decision-making.
Visualization Libraries: Analysts may produce educational, expert-caliber charts, graphs, and interactive dashboards by learning to use tools like Matplotlib, Seaborn, Plotly (Python), and ggplot2 (R).
Data Storytelling: To effectively communicate findings, data analysts need to hone their storytelling abilities in addition to producing images. An effective analyst is able to create narratives from data that help decision-makers make decisions.
Why It Matters: Insights can be effectively communicated through visualizations. By becoming proficient in data visualization, analysts may communicate findings to stakeholders in a way that is compelling, accessible, and actionable.
5. Fundamentals of Machine Learning
Data analysts are finding that machine learning (ML) abilities are becoming more and more useful, especially as companies seek for predictive insights to gain a competitive edge.
Supervised and Unsupervised Learning: To examine and decipher patterns in data, analysts need to be familiar with the fundamentals of both supervised (such as regression and classification) and unsupervised (such as clustering and association) learning.
Well-known Machine Learning Libraries: Scikit-Learn (Python) and other libraries make basic ML models easily accessible, enabling analysts to create predictive models with ease.
Why It Matters: By offering deeper insights and predictive skills, machine learning may improve data analysis. This is especially important in industries where predicting trends is critical, such as marketing, e-commerce, finance, and healthcare.
6. Technologies for Big Data
As big data grows, businesses want analytics tools that can effectively manage enormous datasets. Big data tool knowledge has grown in popularity as a highly sought-after ability.
Hadoop and Spark: Working with big data at scale is made easier for analysts who are familiar with frameworks like Apache Hadoop and Apache Spark.
NoSQL databases: An analyst's capacity to handle unstructured and semi-structured data is enhanced by knowledge of NoSQL databases such as MongoDB and Cassandra.
Why It Matters: Data volumes in many businesses beyond the capacity of conventional processing. In order to meet industrial expectations, big data technologies give analysts the means to handle and examine enormous datasets.
7. Probability and Statistics
Accurately evaluating the findings of data analysis and drawing reliable conclusions require a solid foundation in probability and statistics.
Important Ideas: By understanding probability distributions, confidence intervals, and hypothesis testing, analysts can apply statistical concepts to actual data.
Useful Applications: Variance analysis, statistical significance, and sampling techniques are essential for data-driven decision-making.
Why It Is Important: Analysts can assess the reliability of their data, recognise trends, and formulate well-informed predictions with the use of statistical skills. Accurate and significant analysis is based on this knowledge.
8. Communication and Critical Thinking Soft Skills
Technical proficiency alone is insufficient. Proficient critical thinking and communication capabilities distinguish outstanding analysts.
Communication Skills: To ensure that their insights are understood and useful, analysts must effectively communicate their findings to both technical and non-technical audiences.
Problem-Solving: Critical thinking allows analysts to approach problems methodically, assessing data objectively and providing insightful solutions.
Why It Matters: In the end, data analytics is about making smarter decisions possible. Effective data interpreters and communicators close the gap between data and action, greatly enhancing an organization's value.
Conclusion: Developing a Diverse Skill Set for Success in Data Analytics
Both technical and soft skills must be dedicated in order to master data analytics. Students that master these skills will be at the forefront of the field, from core tools like SQL and visualization libraries to programming languages like Python and R. With data-driven professions becoming more prevalent across industries, these abilities make up a potent toolkit that can lead to fulfilling jobs and worthwhile projects.
These fundamental domains provide a solid basis for students who want to succeed in data analytics in 2025. Although mastery may be a difficult journey, every new skill you acquire will help you become a more proficient, adaptable, and effective data analyst.
Are you prepared to begin your data analytics career? Enrol in the comprehensive data analytics courses that CACMS Institute offers in Amritsar. With flexible scheduling to accommodate your hectic schedule and an industry-relevant curriculum that gives you the tools you need to succeed, our hands-on training programs are made to be successful.
In order to guarantee that you receive a well-rounded education that is suited for the demands of the modern workforce, our programs cover fundamental subjects including Python, R, SQL, Power BI, Tableau, Excel, Advanced Excel, and Data Analytics in Python.
Don't pass up this chance to improve your professional prospects! Please visit the link below or call +91 8288040281 for more information and to sign up for our data analytics courses right now!
#cacms institute#techskills#cacmsinstitute#techeducation#data analytics courses#data analytics training in amritsar#data analytics course#big data analytics#digital marketing training in amritsar#python courses in Amritsar#Python training in Amritsar#certification#data science course#tableau course in Amritsar
0 notes
Text
Industry-Leading Visualization with Tableau Data Visualization
1. User-Friendly Interface: The intuitive design allows anyone to easily start visualizing data.
2. Seamless Integration: Connects easily with various data sources for efficient analysis.
3. Quick Insights: Generate actionable insights within minutes.
4. Integrated Collaboration Tools: Share dashboards and insights with your team effortlessly.
5. Regular Updates: Tableau continuously evolves with new features and improvements.
Want to take your skills to the next level? Join our 1-day Tableau Bootcamp on 2nd November (Online)!
#tableau#tableau software#data visualization#data visualisation#dataviz#software#technology#cavillion#cavillion learning#data analytics#data#tableau online training#tableaudesktop#tableau dashboard#big data#business analytics#data analysis#data science#tableau course#salesforce#Tableau Tutorial#Tableau Visualization#Tableau Training#learn tableau#tableau certification#Tableau Visualisation
1 note
·
View note
Text
5 Powerful Programming Tools Every Data Scientist Needs

Data science is the field that involves statistics, mathematics, programming, and domain knowledge to extract meaningful insights from data. The explosion of big data and artificial intelligence has led to the use of specialized programming tools by data scientists to process, analyze, and visualize complex datasets efficiently.
Choosing the right tools is very important for anyone who wants to build a career in data science. There are many programming languages and frameworks, but some tools have gained popularity because of their robustness, ease of use, and powerful capabilities.
This article explores the top 5 programming tools in data science that every aspiring and professional data scientist should know.
Top 5 Programming Tools in Data Science
1. Python
Probably Python is the leading language used due to its versatility and simplicity together with extensive libraries. It applies various data science tasks, data cleaning, statistics, machine learning, and even deep learning applications.
Key Python Features for Data Science:
Packages & Framework: Pandas, NumPy, Matplotlib, Scikit-learn, TensorFlow, PyTorch
Easy to Learn; the syntax for programming is plain simple
High scalability; well suited for analyzing data at hand and enterprise business application
Community Support: One of the largest developer communities contributing to continuous improvement
Python's versatility makes it the go-to for professionals looking to be great at data science and AI.
2. R
R is another powerful programming language designed specifically for statistical computing and data visualization. It is extremely popular among statisticians and researchers in academia and industry.
Key Features of R for Data Science:
Statistical Computing: Inbuilt functions for complex statistical analysis
Data Visualization: Libraries like ggplot2 and Shiny for interactive visualizations
Comprehensive Packages: CRAN repository hosts thousands of data science packages
Machine Learning Integration: Supports algorithms for predictive modeling and data mining
R is a great option if the data scientist specializes in statistical analysis and data visualization.
3. SQL (Structured Query Language)
SQL is important for data scientists to query, manipulate, and manage structured data efficiently. The relational databases contain huge amounts of data; therefore, SQL is an important skill in data science.
Important Features of SQL for Data Science
Data Extraction: Retrieve and filter large datasets efficiently
Data Manipulation: Aggregate, join, and transform datasets for analysis
Database Management: Supports relational database management systems (RDBMS) such as MySQL, PostgreSQL, and Microsoft SQL Server
Integration with Other Tools: Works seamlessly with Python, R, and BI tools
SQL is indispensable for data professionals who handle structured data stored in relational databases.
4. Apache Spark
Apache Spark is the most widely utilized open-source, big data processing framework for very large-scale analytics and machine learning. It excels in performance for handling a huge amount of data that no other tool would be able to process.
Core Features of Apache Spark for Data Science:
Data Processing: Handle large datasets on high speed.
In-Memory Computation: Better performance in comparison to other disk-based systems
MLlib: A Built-in Machine Library for Scalable AI Models.
Compatibility with Other Tools: Supports Python (PySpark), R (SparkR), and SQL
Apache Spark is best suited for data scientists working on big data and real-time analytics projects.
5. Tableau
Tableau is one of the most powerful data visualization tools used in data science. Users can develop interactive and informative dashboards without needing extensive knowledge of coding.
Main Features of Tableau for Data Science:
Drag-and-Drop Interface: Suitable for non-programmers
Advanced Visualizations: Complex graphs, heatmaps, and geospatial data can be represented
Data Source Integration: Database, cloud storage, and APIs integration
Real-Time Analytics: Fast decision-making is achieved through dynamic reporting
Tableau is a very popular business intelligence and data storytelling tool used for making data-driven decisions available to non-technical stakeholders.
Data Science and Programming Tools in India
This led to India's emergence as one of the data science and AI hubs, which has seen most businesses, start-ups, and government organizations take significant investments into AI-driven solutions. The increase in demand for data scientists boosted the adoption rate of programming tools such as Python, R, SQL, and Apache Spark.
Government and Industrial Initiatives Gaining Momentum Towards Data Science Adoption in India
National AI Strategy: NITI Aayog's vision for AI driven economic transformation.
Digital India Initiative: This has promoted data-driven governance and integration of AI into public services.
AI Adoption in Enterprises: The big enterprises TCS, Infosys, and Reliance have been adopting AI for business optimisation.
Emerging Startups in AI & Analytics: Many Indian startups have been creating AI-driven products by using top data science tools.
Challenges to Data Science Growth in India
Some of the challenges in data science growth despite rapid advancements in India are:
Skill Gaps: Demand outstrips supply.
Data Privacy Issues: The emphasis lately has been on data protection laws such as the Data Protection Bill.
Infrastructure Constraint: Computational high-end resources are not accessible to all companies.
To bridge this skill gap, many online and offline programs assist students and professionals in learning data science from scratch through comprehensive training in programming tools, AI, and machine learning.
Kolkata Becoming the Next Data Science Hub
Kolkata is soon emerging as an important center for education and research in data science with its rich academic excellence and growth in the IT sector. Increasing adoption of AI across various sectors has resulted in businesses and institutions in Kolkata concentrating on building essential data science skills in professionals.
Academic Institutions and AI Education
Multiple institutions and private learning centers provide exclusive AI Courses Kolkata, dealing with the must-have programming skills such as Python, R, SQL, and Spark. Hands-on training sessions are provided by these courses about data analytics, machine learning, and AI.
Industries Using Data Science in Kolkata
Banking & Finance: Artificial intelligence-based risk analysis and fraud detection systems
Healthcare: Data-driven Predictive Analytics of patient care optimisation
E-Commerce & Retail: Customized recommendations & customer behavior analysis
EdTech: AI based adaptive learning environment for students.
Future Prospects of Data Science in Kolkata
Kolkata would find a vital place in India's data-driven economy because more and more businesses as well as educational institutions are putting money into AI and data science. The city of Kolkata is currently focusing strategically on technology education and research in AI for future innovations in AI and data analytics.
Conclusion
Over the years, with the discovery of data science, such programming tools like Python and R, SQL, Apache Spark, and Tableau have become indispensable in the world of professionals. They help in analyzing data, building AI models, and creating impactful visualizations.
Government initiatives and investments by the enterprises have seen India adapt rapidly to data science and AI, thus putting a high demand on skilled professionals. As a beginner, the doors are open with many educational programs to learn data science with hands-on experience using the most popular tools.
Kolkata is now emerging as a hub for AI education and innovation, which will provide world-class learning opportunities to aspiring data scientists. Mastery of these programming tools will help professionals stay ahead in the ever-evolving data science landscape.
0 notes
Text
#DataAnalysis #PowerBI #DataVisualization #BusinessIntelligence #DashboardDesign #DataInsights #DataScience #FreelanceGig #FiverrGig #DataExpert
#analytics#business intelligence#data analytics#sql#data analysis#data visualization#data science#data#power bi#tableau#excel
0 notes
Text
A Comprehensive Data Science Course in Hyderabad
Mastering data science is a career game-changer in a world where the new currency is data. Be it a professional with experience who is uninterested in a domain switch or a fresher who is super-enthusiastic about learning the world of data, a well-paced and drafted course is of immense value. From a land that promotes its tech ecosystem, Hyderabad furnishes a few of the best data science and AI upskilling programs to its dwellers. Here is all you need to know to find that perfect data science course in Hyderabad filled with the most important tools and technologies you should learn as part of your course.
Why a Data Science Course?
Hyderabad is now a hub for technology and innovation and, therefore, a great place to learn data science. Many data science institutes in Hyderabad offer holistic training combined with hands-on experience and industry-assimilated learning. Data science courses in Hyderabad sourced from illustrious faculty members, up-to-date tools, and a professional network will help you take off on your career and set the ball rolling to achieve excellence far beyond your imagination.
Key Components of a Data Science Course
The following is what is to be included in a proper data science course:
1- Managing and Manipulation of Data:
Knowing how to deal with data forms the core of data science. You learn SQL, vital in making queries and managing a relational database. Furthermore, MongoDB is usually taught to manipulate unstructured data.
2- Data Visualization:
The most important feature of these topics will always be visualization tools like Tableau and PowerBI, which are capable of translating complicated figures into easily understandable insights. A good course will train you on building interactive dashboards that may help drive decision processes.
3- Big Data Technologies:
Where volumes of data grow, handling big data tools becomes a much-needed skill. Often integrated within the courses is training in Big Data platforms like Hadoop or Spark Analytics, availing you of the chance to process and analyse big bulks of data with ease.
4- Time Series Analysis:
One of the most critical issues, especially in finance or economics, is to be able to identify trends and patterns over time. This subject should introduce you to the analysis of times series to make predictions based on historical information.
Introduction to Artificial Intelligence
Artificial Intelligence is among the foundational pillars of data science. Thus, in many courses, one should expect various features and techniques associated with AI:
1- Deep Learning with TensorFlow:
An approach to machine learning that exercises deeply to mimic the neuron activities in the human brain. Being an open-source library, TensorFlow is also extensively used to implement deep learning models. Any good course will equip you with practical hands-on application of this powerful tool.
2- Natural Language Processing:
This would be important for working with text data. It refers to the ability of computers to understand and generate human language. NLP techniques covered in these courses include various tasks such as text classification, sentiment analysis, and language translation.
3- Model Training and Deployment:
One should understand how to train and deploy models. The course's learnings will involve practical experience using cloud platforms to deploy machine learning models at scale—course content should be understood via AWS and GCP.
Top Best Data Science Course in Hyderabad
There are so many options that choosing the best data science training in Hyderabad can be tough. Here are a few things you should look into:
Curriculum: It should cover all the topics as mentioned above, along with their tools and techniques.
Faculty: Most of the courses are generally conducted by the ones currently working in the data sciences and AI field.
Hands-on Projects: The best way to learn data science is by actually doing it. Opt for a course that involves numerous hands-on projects along with case studies.
Placement Support: If you aim to start or shift your career, look for support from a strong placement team with evidence of many successful alumni in the field you desire.
Conclusion
The data science course in Hyderabad allows you to invest locally and globally in emerging sectors. Master critical tools like SQL, MongoDB, Tableau, Spark, TensorFlow for AI, and NLP to dive into challenges of this data-driven world. Up your skills or launch a new career with courses designed by industry experts to ensure you have the hands-on experience and knowledge that will set you apart.
1 note
·
View note
Text
DATA ANALYTICS COURSE IN KOTA
Elevate your career with DataMites Data Analytics Course in Dehradun. Master Python, SQL, Power BI, Tableau, and Machine Learning with expert-led training. Gain practical experience, industry certification, and internship & job support. Limited-time 65% fee discount!
#Data Analytics Training Kota#Data Analytics Certification Kota#Best Data Analytics Course in Kota#Python for Data Analytics Kota#Power BI Training Kota#Tableau Course Kota#Data Science Training Kota#Machine Learning Course Kota#Internship in Data Analytics Kota
0 notes
Text
What is Data Science? A Comprehensive Guide for Beginners

In today’s data-driven world, the term “Data Science” has become a buzzword across industries. Whether it’s in technology, healthcare, finance, or retail, data science is transforming how businesses operate, make decisions, and understand their customers. But what exactly is data science? And why is it so crucial in the modern world? This comprehensive guide is designed to help beginners understand the fundamentals of data science, its processes, tools, and its significance in various fields.
#Data Science#Data Collection#Data Cleaning#Data Exploration#Data Visualization#Data Modeling#Model Evaluation#Deployment#Monitoring#Data Science Tools#Data Science Technologies#Python#R#SQL#PyTorch#TensorFlow#Tableau#Power BI#Hadoop#Spark#Business#Healthcare#Finance#Marketing
0 notes
Text
Tableau A-Z: Hands-On Tableau Training for Data Science
0 notes