#probabilistic
Explore tagged Tumblr posts
Text
Lead Engineer - Probabilistic Design - Aerospace Research
Job title: Lead Engineer – Probabilistic Design – Aerospace Research Company: GE Aerospace Job description: design, inverse modeling, engineering ***ysis model validation for GE Aerospace and U.S. government projects…, energy transition, and the future of flight throughout the GE Aerospace company. You will also work with U.S. government… Expected salary: $90000 – 175000 per year Location:…
0 notes
Text
"One popular strategy is to enter an emotional spiral. Could that be the right approach? We contacted several researchers who are experts in emotional spirals to ask them, but none of them were in a state to speak with us."
Probalistic Uncertainty [Explained]
Transcript Under the Cut
[A table titled "Coping With Probabilistic Uncertainty", with two columns labeled "Scenario" and "How to think about it in an emptionally healthy way". The boxes in the Scenario column contains text followed by a rectangle split into two parts; the left part is a smiley face, the right part is a frowny face.]
Row 1, column 1: "Good outcome more likely". The smiley face portion of the rectangle is about 75%. Row 1, column 2: "Recognize that the bad outcome is possible, but be reassured that the odds are in your favor".
Row 2, column 1: "Bad outcome more likely". The smiley face portion of the rectangle is about 25%. Row 2, column 2: "Prepare for the bad outcome while remembering that the future isn't certain and hope is justified".
Row 3, column 1: "Precisely 50/50". The rectangle is split in half. Row 3, column 2: "????? N/A ????"
4K notes
·
View notes
Text
Not the Monty Hall problem 😔
#I understand the probabilistic nature of the problem and i accept it#but it just feels wrong to me. i accept that facts don't care about my feelings. but the facts also don't change em
23 notes
·
View notes
Text
Bullshit engines.
I mean, I don’t suppose many people following this blog strongly disagree.
#they are not search engines#they are not the new improved version! they are something else!#they are probabilistic text generators#they produce something plausible based on the data set they were trained on#there is no knowledge or understanding involved
13 notes
·
View notes
Text
finally getting around to watching a playthrough of mouthwashing, this better be as sick as all the posting ive seen for it has made it out to be
#the cryptid speaks#based solely on the first 5 minutes of this watch it will be#this is everyone's heads up that this may be the next hit media to overwhelm my blog#who knows! well; probabilistically -
10 notes
·
View notes
Text
The Illusion of Complexity: Binary Exploitation in Engagement-Driven Algorithms
Abstract:
This paper examines how modern engagement algorithms employed by major tech platforms (e.g., Google, Meta, TikTok, and formerly Twitter/X) exploit predictable human cognitive patterns through simplified binary interactions. The prevailing perception that these systems rely on sophisticated personalization models is challenged; instead, it is proposed that such algorithms rely on statistical generalizations, perceptual manipulation, and engineered emotional reactions to maintain continuous user engagement. The illusion of depth is a byproduct of probabilistic brute force, not advanced understanding.
1. Introduction
Contemporary discourse often attributes high levels of sophistication and intelligence to the recommendation and engagement algorithms employed by dominant tech companies. Users report instances of eerie accuracy or emotionally resonant suggestions, fueling the belief that these systems understand them deeply. However, closer inspection reveals a more efficient and cynical design principle: engagement maximization through binary funneling.
2. Binary Funneling and Predictive Exploitation
At the core of these algorithms lies a reductive model: categorize user reactions as either positive (approval, enjoyment, validation) or negative (disgust, anger, outrage). This binary schema simplifies personalization into a feedback loop in which any user response serves to reinforce algorithmic certainty. There is no need for genuine nuance or contextual understanding; rather, content is optimized to provoke any reaction that sustains user attention.
Once a user engages with content —whether through liking, commenting, pausing, or rage-watching— the system deploys a cluster of categorically similar material. This recurrence fosters two dominant psychological outcomes:
If the user enjoys the content, they may perceive the algorithm as insightful or ���smart,” attributing agency or personalization where none exists.
If the user dislikes the content, they may continue engaging in a doomscroll or outrage spiral, reinforcing the same cycle through negative affect.
In both scenarios, engagement is preserved; thus, profit is ensured.
3. The Illusion of Uniqueness
A critical mechanism in this system is the exploitation of the human tendency to overestimate personal uniqueness. Drawing on techniques long employed by illusionists, scammers, and cold readers, platforms capitalize on common patterns of thought and behavior that are statistically widespread but perceived as rare by individuals.
Examples include:
Posing prompts or content cues that seem personalized but are statistically predictable (e.g., "think of a number between 1 and 50 with two odd digits” → most select 37).
Triggering cognitive biases such as the availability heuristic and frequency illusion, which make repeated or familiar concepts appear newly significant.
This creates a reinforcing illusion: the user feels “understood” because the system has merely guessed correctly within a narrow set of likely options. The emotional resonance of the result further conceals the crude probabilistic engine behind it.
4. Emotional Engagement as Systemic Currency
The underlying goal is not understanding, but reaction. These systems optimize for time-on-platform, not user well-being or cognitive autonomy. Anger, sadness, tribal validation, fear, and parasocial attachment are all equally useful inputs. Through this lens, the algorithm is less an intelligent system and more an industrialized Skinner box: an operant conditioning engine powered by data extraction.
By removing the need for interpretive complexity and relying instead on scalable, binary psychological manipulation, companies minimize operational costs while maximizing monetizable engagement.
5. Black-Box Mythology and Cognitive Deference
Compounding this problem is the opacity of these systems. The “black-box” nature of proprietary algorithms fosters a mythos of sophistication. Users, unaware of the relatively simple statistical methods in use, ascribe higher-order reasoning or consciousness to systems that function through brute-force pattern amplification.
This deference becomes part of the trap: once convinced the algorithm “knows them,” users are less likely to question its manipulations and more likely to conform to its outputs, completing the feedback circuit.
6. Conclusion
The supposed sophistication of engagement algorithms is a carefully sustained illusion. By funneling user behavior into binary categories and exploiting universally predictable psychological responses, platforms maintain the appearance of intelligent personalization while operating through reductive, low-cost mechanisms. Human cognition —biased toward pattern recognition and overestimation of self-uniqueness— completes the illusion without external effort. The result is a scalable system of emotional manipulation that masquerades as individualized insight.
In essence, the algorithm does not understand the user; it understands that the user wants to be understood, and it weaponizes that desire for profit.
#ragebait tactics#mass psychology#algorithmic manipulation#false agency#click economy#social media addiction#illusion of complexity#engagement bait#probabilistic targeting#feedback loops#psychological nudging#manipulation#user profiling#flawed perception#propaganda#social engineering#social science#outrage culture#engagement optimization#cognitive bias#predictive algorithms#black box ai#personalization illusion#pattern exploitation#ai#binary funnelling#dopamine hack#profiling#Skinner box#dichotomy
3 notes
·
View notes
Text
Bayesian Active Exploration: A New Frontier in Artificial Intelligence
The field of artificial intelligence has seen tremendous growth and advancements in recent years, with various techniques and paradigms emerging to tackle complex problems in the field of machine learning, computer vision, and natural language processing. Two of these concepts that have attracted a lot of attention are active inference and Bayesian mechanics. Although both techniques have been researched separately, their synergy has the potential to revolutionize AI by creating more efficient, accurate, and effective systems.
Traditional machine learning algorithms rely on a passive approach, where the system receives data and updates its parameters without actively influencing the data collection process. However, this approach can have limitations, especially in complex and dynamic environments. Active interference, on the other hand, allows AI systems to take an active role in selecting the most informative data points or actions to collect more relevant information. In this way, active inference allows systems to adapt to changing environments, reducing the need for labeled data and improving the efficiency of learning and decision-making.
One of the first milestones in active inference was the development of the "query by committee" algorithm by Freund et al. in 1997. This algorithm used a committee of models to determine the most meaningful data points to capture, laying the foundation for future active learning techniques. Another important milestone was the introduction of "uncertainty sampling" by Lewis and Gale in 1994, which selected data points with the highest uncertainty or ambiguity to capture more information.
Bayesian mechanics, on the other hand, provides a probabilistic framework for reasoning and decision-making under uncertainty. By modeling complex systems using probability distributions, Bayesian mechanics enables AI systems to quantify uncertainty and ambiguity, thereby making more informed decisions when faced with incomplete or noisy data. Bayesian inference, the process of updating the prior distribution using new data, is a powerful tool for learning and decision-making.
One of the first milestones in Bayesian mechanics was the development of Bayes' theorem by Thomas Bayes in 1763. This theorem provided a mathematical framework for updating the probability of a hypothesis based on new evidence. Another important milestone was the introduction of Bayesian networks by Pearl in 1988, which provided a structured approach to modeling complex systems using probability distributions.
While active inference and Bayesian mechanics each have their strengths, combining them has the potential to create a new generation of AI systems that can actively collect informative data and update their probabilistic models to make more informed decisions. The combination of active inference and Bayesian mechanics has numerous applications in AI, including robotics, computer vision, and natural language processing. In robotics, for example, active inference can be used to actively explore the environment, collect more informative data, and improve navigation and decision-making. In computer vision, active inference can be used to actively select the most informative images or viewpoints, improving object recognition or scene understanding.
Timeline:
1763: Bayes' theorem
1988: Bayesian networks
1994: Uncertainty Sampling
1997: Query by Committee algorithm
2017: Deep Bayesian Active Learning
2019: Bayesian Active Exploration
2020: Active Bayesian Inference for Deep Learning
2020: Bayesian Active Learning for Computer Vision
The synergy of active inference and Bayesian mechanics is expected to play a crucial role in shaping the next generation of AI systems. Some possible future developments in this area include:
- Combining active inference and Bayesian mechanics with other AI techniques, such as reinforcement learning and transfer learning, to create more powerful and flexible AI systems.
- Applying the synergy of active inference and Bayesian mechanics to new areas, such as healthcare, finance, and education, to improve decision-making and outcomes.
- Developing new algorithms and techniques that integrate active inference and Bayesian mechanics, such as Bayesian active learning for deep learning and Bayesian active exploration for robotics.
Dr. Sanjeev Namjosh: The Hidden Math Behind All Living Systems - On Active Inference, the Free Energy Principle, and Bayesian Mechanics (Machine Learning Street Talk, October 2024)
youtube
Saturday, October 26, 2024
#artificial intelligence#active learning#bayesian mechanics#machine learning#deep learning#robotics#computer vision#natural language processing#uncertainty quantification#decision making#probabilistic modeling#bayesian inference#active interference#ai research#intelligent systems#interview#ai assisted writing#machine art#Youtube
6 notes
·
View notes
Text
Legal scholars claiming causation when all they have is weak ass correlation with massive signs of it being spurious, endogenous or caused by a missing variable is what will give me an ulcer.
Or a very complex and nuanced villain origin story, as the jurist who turned against her own peers and companions, screaming "YOU ARE THE ONE MAKING ME DO THIS" to them, as she puts on the dark cloak of political science, now forever tormented by her torn identity and a massive imposter syndrome.
#adventures in academia#I want to like this book#I like a lot of things about this book#but you cannot give me one (one) table of descriptive statistics#and tell me this is proof of causation#also I will start ranting about how qualitative methods are based on set theory and deterministic causality#so using stats does not wooooooork because they have a probabilistic approach to causality#STOP PLEASE
13 notes
·
View notes
Note
I want to know about books 11, 22, 33, 44 and 55 💫
Thanks for asking! Lol, typically for me, every one of these is a mystery, but they're all pretty different!
11. Light Thickens, Ngaio Marsh - The final Roderick Alleyn mystery (not that they need to be read in order). This does actually have some characters return from a mystery set (and written) several years before this one; they have aged, but Alleyn apparently has not. It's a good stand-alone mystery set in a play house (one of Marsh's favorite things to write about), with various references to Macbeth.
22. The Redeemers, Ace Atkins - This is book 5 in the Quinn Colson mystery series, I spent a lot of the beginning of last year tearing through them (11 total), slowed down only by waiting for library holds to come in. The sort of arc of the series (which does take place over 10 or so years, each book set ~when it was published) is that former Army Ranger Quinn Colson comes back to his hometown in Mississippi and then runs for sheriff so as to get rid of the old corrupt sheriff - and then takes down a crime lord, and has to quit being sheriff, and gets voted back in, and another crime lord takes over... Anyway, they're grittier/more violent than a lot of the mysteries I read, but I was hooked. All the characters felt very well-rounded - all the good guys have significant flaws, but I love them anyway, and (almost) all the bad guys have moments where they're sympathetic, if not redeemable.
33. The Night She Died, Dorothy Simpson - OK, so I can't think of anything particularly wrong with this book, but I forgot I read it until looking #33 up for this list. The first in yet another mystery series (published 1980, set in England), and it was...fine? I didn't read any others in the series, but I did finish the book, so it was gripping enough for that!
44. One, Two, Buckle My Shoe, Agatha Christie - A Poirot book; Poirot goes to the dentist, and then later in the day, the dentist is found murdered! If you've read any Poirot stuff, then you have an idea where things go from there. This was a reread, I like the more domestic Christie books (as opposed to international intrigue).
55. Relic, Douglas Preston with Lincoln Child - This is also first in a series, called the Pendergast series. I actually remember why I read this - I saw several books from the series in the library, and was intrigued, so when I got home I found the first one on Libby. Honestly not sure that "mystery" is the best description - maybe a combo of horror and thriller and some supernatural elements. I did like this first one - it's gripping, and Pendergast is a charismatic character. There's some funky pseudoscience in this one (think Jurassic Park) to explain some pretty fantastic things, but it's made to sound reasonable; I read two more in the series, but when it looked like Pendergast was actually starting to time travel with the power of his mind in the third one, I decided not to read the other 19(!).
(Send me a number 1 - 206 and I'll tell you about a book I read in 2023!)
#books of 2023#I promise I read other genres - but probabilistically this makes sense#gonna tag the books now#light thickens#ngaio marsh#quinn colson#ace atkins#the night she died#dorothy simpson#one two buckle my shoe#agatha christie#relic#douglas preston#lincoln child
6 notes
·
View notes
Text
I didn't want to distract from the excellent article about the woman doing great work on Wikipedia nazi articles, but it reminded me of my current crusade that I need Wikipedia gurus to help me fix.
Probabilistic genotyping is a highly contentious form of forensic science. They use an algorithm to say if someone's DNA was on the scene of a crime for mixtures that are too complex to be analyzed by hand.
Let's learn more by going to the Wikipedia page.
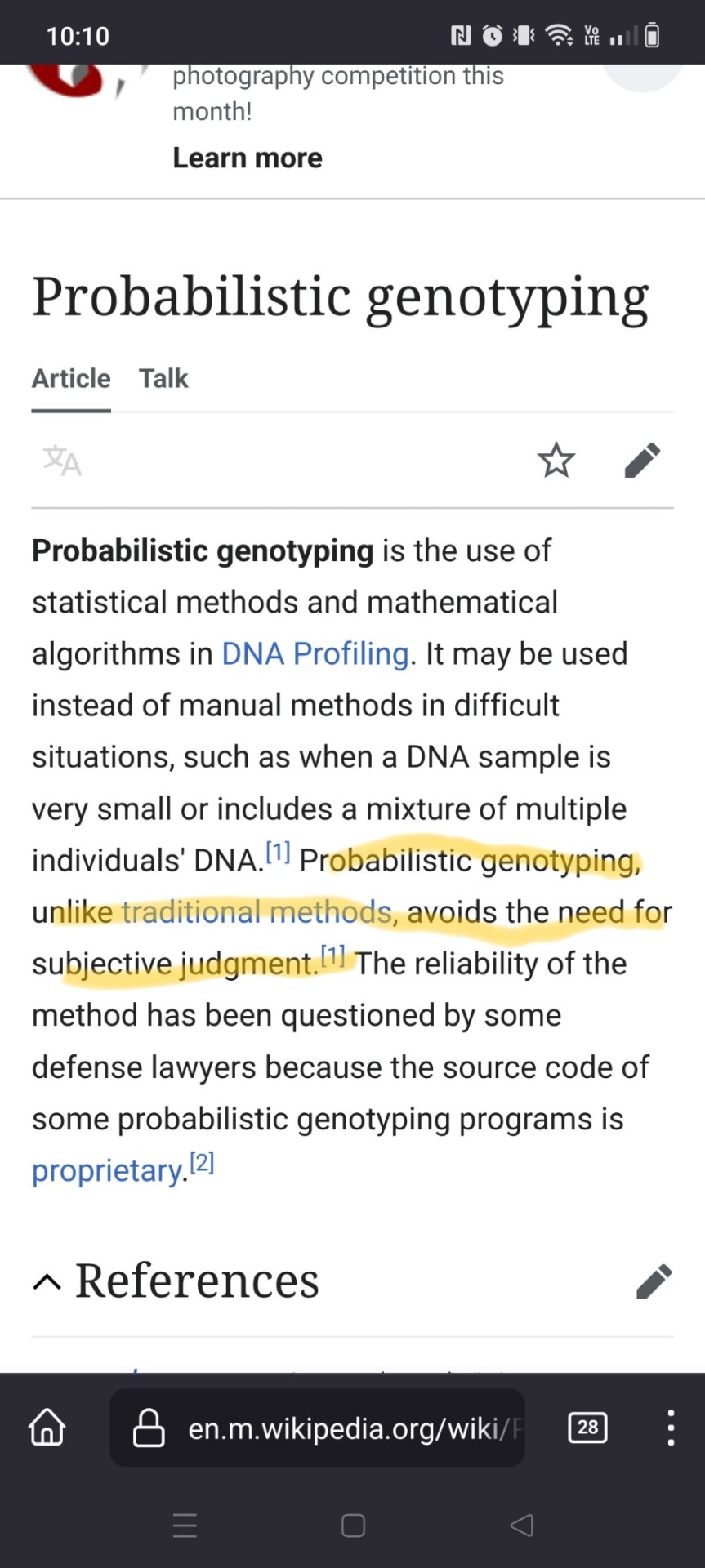
Oh that's good, it's less subjective. Sure defense attorneys question it, but they question all sorts of science.
Let's go to the cited article to learn more.

Well that doesn't seem like it probably supports the Wikipedia assertion. Let's skim through to the conclusion section of the article.
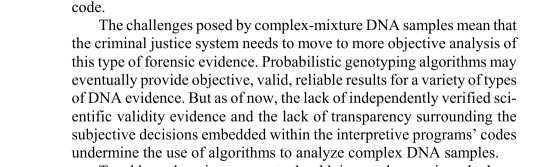
Well shit.
Also the article talks about how STRmix, one of these popular programs, has allowed defense attorneys to look at the algorithm. That's true! We hired an expert who was allowed to look at millions of lines of code! For 4 hours. With a pen and paper.
Junk science is all over the place in courtrooms and it's insane that allegedly objective places like Wikipedia endorse it so blindly. I am biting.
#wikipedia#forensic science#probabilistic genotyping#criminal justice#people who are good at wikipedia i need your help#criminal law
2 notes
·
View notes
Text
X marks the unknown in algebra – but X's origins are a math mystery

2 notes
·
View notes
Text
see this is why you need a derivatives structurer, you tranche the product - you sell the equity portion (the money) to a hedge fund for 99.9 cents on the dollar, then you hold an auction for the gender change part! thanks financialization
as a cis guy, when presented with the "99% you get a ton of money, 1% you turn into a girl" it honestly would be dumb to not hit that button until it breaks. like ok now i have 100 bajillion dollars and gender dysphoria. big deal. i have all the money in the world to turn me back into a guy. like with that kind of money i could have obama do me a phalloplasty. he wouldnt be able to do it as he isnt a surgeon but the point still stands
#imagining a world where jane street has a desk specifically for these weird probabilistic buttons#like yeah thats a gender one. we put those in the gender change fund. we have an aging desk and a teleportation desk too
82K notes
·
View notes
Text
Ask A Genius 1255: Total Crap
Scott Douglas Jacobsen: I found an article in Mother Jones about AI becoming as powerful as human cognition by 2025 worth reading. The article is from 2013. The article was scaled up in search results somehow. Some parts of it could have been written ten years later. It uses Moore’s law from 2013 to predict that the number of calculations per second in the fastest computers will eventually be on…
#AI cognition limits#iterative AI integration#probabilistic language processing#Rick Rosner#Scott Douglas Jacobsen
0 notes
Text

It’s not a binding circle guys
#I’m a RF electrical engineer#and it is a little bit magic#yeah let’s blast power into this metal rod and it’ll transmit information#you gotta make the metal strip on top of the other metal strip this long or else your signal gets fucked#it is understandable magic even though it gets very probabilistic at times#but it adds whimsy to the suffering#I’d like to also propose the smith chart as an addition here
119K notes
·
View notes
Text
The Role of MDM in Enhancing Data Quality
In today’s data-centric business world, organizations rely on accurate and consistent data to drive decision-making. One of the key components to ensuring data quality is Master Data Management (MDM). MDM plays a pivotal role in consolidating, managing, and maintaining high-quality data across an organization’s various systems and processes. As businesses grow and data volumes expand, the need for efficient data quality measures becomes critical. This is where techniques like deterministic matching and probabilistic matching come into play, allowing MDM systems to manage and reconcile records effectively.
Understanding Data Quality and Its Importance
Data quality refers to the reliability, accuracy, and consistency of data used across an organization. Poor data quality can lead to incorrect insights, flawed decision-making, and operational inefficiencies. For example, a customer database with duplicate records or inaccurate information can result in misguided marketing efforts, customer dissatisfaction, and even compliance risks.
MDM addresses these challenges by centralizing an organization’s key data—referred to as "master data"—such as customer, product, and supplier information. With MDM in place, organizations can standardize data, remove duplicates, and resolve inconsistencies. However, achieving high data quality requires sophisticated data matching techniques.
Deterministic Matching in MDM
Deterministic matching is a method used by MDM systems to match records based on exact matches of predefined identifiers, such as email addresses, phone numbers, or customer IDs. In this approach, if two records have the same value for a specific field, such as an identical customer ID, they are considered a match.
Example: Let’s say a retailer uses customer IDs to track purchases. Deterministic matching will easily reconcile records where the same customer ID appears in different systems, ensuring that all transactions are linked to the correct individual.
While deterministic matching is highly accurate when unique identifiers are present, it struggles with inconsistencies. Minor differences, such as a typo in an email address or a missing middle name, can prevent records from being matched correctly. For deterministic matching to be effective, the data must be clean and standardized.
Probabilistic Matching in MDM
In contrast, probabilistic matching offers a more flexible and powerful solution for reconciling records that may not have exact matches. This technique uses algorithms to calculate the likelihood that two records refer to the same entity, even if the data points differ. Probabilistic matching evaluates multiple attributes—such as name, address, and date of birth—and assigns a weight to each attribute based on its reliability.
Example: A bank merging customer data from multiple sources might have a record for "John A. Doe" in one system and "J. Doe" in another. Probabilistic matching will compare not only the names but also other factors, such as addresses and phone numbers, to determine whether these records likely refer to the same person. If the combined data points meet a predefined probability threshold, the records will be merged.
Probabilistic matching is particularly useful in MDM when dealing with large datasets where inconsistencies, misspellings, or missing information are common. It can also handle scenarios where multiple records contain partial data, making it a powerful tool for improving data quality in complex environments.
The Role of Deterministic and Probabilistic Matching in MDM
Both deterministic and probabilistic matching are integral to MDM systems, but their application depends on the specific needs of the organization and the quality of the data.
Use Cases for Deterministic Matching: This technique works best in environments where data is clean and consistent, and where reliable, unique identifiers are available. For example, in industries like healthcare or finance, where Social Security numbers, patient IDs, or account numbers are used, deterministic matching provides quick, highly accurate results.
Use Cases for Probabilistic Matching: Probabilistic matching excels in scenarios where data is prone to errors or where exact matches aren’t always possible. In retail, marketing, or customer relationship management, customer information often varies across platforms, and probabilistic matching is crucial for linking records that may not have consistent data points.
Enhancing Data Quality with MDM
Master Data Management, when coupled with effective matching techniques, significantly enhances data quality by resolving duplicate records, correcting inaccuracies, and ensuring consistency across systems. The combination of deterministic and probabilistic matching allows businesses to achieve a more holistic view of their data, which is essential for:
Accurate Reporting and Analytics: High-quality data ensures that reports and analytics are based on accurate information, leading to better business insights and decision-making.
Improved Customer Experience: Consolidating customer data allows businesses to deliver a more personalized and seamless experience across touchpoints.
Regulatory Compliance: Many industries are subject to stringent data regulations. By maintaining accurate records through MDM, businesses can meet compliance requirements and avoid costly penalties.
Real-World Application of MDM in Enhancing Data Quality
Many industries rely on MDM to enhance data quality, especially in sectors where customer data plays a critical role.
Retail: Retailers use MDM to unify customer data across online and offline channels. With probabilistic matching, they can create comprehensive profiles even when customer names or contact details vary across systems.
Healthcare: In healthcare, ensuring accurate patient records is crucial for treatment and care. Deterministic matching helps link patient IDs, while probabilistic matching can reconcile records with missing or inconsistent data, ensuring no critical information is overlooked.
Financial Services: Banks and financial institutions rely on MDM to manage vast amounts of customer and transaction data. Both deterministic and probabilistic matching help ensure accurate customer records, reducing the risk of errors in financial reporting and regulatory compliance.
Conclusion
Master Data Management plays a crucial role in enhancing data quality across organizations by centralizing, standardizing, and cleaning critical data. Techniques like deterministic and probabilistic matching ensure that MDM systems can effectively reconcile records, even in complex and inconsistent datasets. By improving data quality, businesses can make better decisions, provide enhanced customer experiences, and maintain regulatory compliance.
In today’s competitive market, ensuring data accuracy isn’t just a best practice—it’s a necessity. Through the proper application of MDM and advanced data matching techniques, organizations can unlock the full potential of their data, paving the way for sustainable growth and success.
0 notes
Text
Book Summary of How to Decide by Annie Duke
How to Decide by Annie Duke is a practical guide on mastering decision-making. Whether in daily life or high-stakes situations, Duke helps you develop better decision skills, teaching you to embrace uncertainty and make smarter, more confident choices. Overview of the Author: Annie DukeThe Purpose of How to DecideKey Themes in How to Decide by Annie DukeDecision-Making as a SkillThe Role of…
#Annie Duke#cognitive biases#decision frameworks#decision process#decision skills#decision-making#How to Decide book#personal growth#probabilistic thinking#uncertainty
0 notes