#bayesian mechanics
Explore tagged Tumblr posts
Text
Bayesian Active Exploration: A New Frontier in Artificial Intelligence
The field of artificial intelligence has seen tremendous growth and advancements in recent years, with various techniques and paradigms emerging to tackle complex problems in the field of machine learning, computer vision, and natural language processing. Two of these concepts that have attracted a lot of attention are active inference and Bayesian mechanics. Although both techniques have been researched separately, their synergy has the potential to revolutionize AI by creating more efficient, accurate, and effective systems.
Traditional machine learning algorithms rely on a passive approach, where the system receives data and updates its parameters without actively influencing the data collection process. However, this approach can have limitations, especially in complex and dynamic environments. Active interference, on the other hand, allows AI systems to take an active role in selecting the most informative data points or actions to collect more relevant information. In this way, active inference allows systems to adapt to changing environments, reducing the need for labeled data and improving the efficiency of learning and decision-making.
One of the first milestones in active inference was the development of the "query by committee" algorithm by Freund et al. in 1997. This algorithm used a committee of models to determine the most meaningful data points to capture, laying the foundation for future active learning techniques. Another important milestone was the introduction of "uncertainty sampling" by Lewis and Gale in 1994, which selected data points with the highest uncertainty or ambiguity to capture more information.
Bayesian mechanics, on the other hand, provides a probabilistic framework for reasoning and decision-making under uncertainty. By modeling complex systems using probability distributions, Bayesian mechanics enables AI systems to quantify uncertainty and ambiguity, thereby making more informed decisions when faced with incomplete or noisy data. Bayesian inference, the process of updating the prior distribution using new data, is a powerful tool for learning and decision-making.
One of the first milestones in Bayesian mechanics was the development of Bayes' theorem by Thomas Bayes in 1763. This theorem provided a mathematical framework for updating the probability of a hypothesis based on new evidence. Another important milestone was the introduction of Bayesian networks by Pearl in 1988, which provided a structured approach to modeling complex systems using probability distributions.
While active inference and Bayesian mechanics each have their strengths, combining them has the potential to create a new generation of AI systems that can actively collect informative data and update their probabilistic models to make more informed decisions. The combination of active inference and Bayesian mechanics has numerous applications in AI, including robotics, computer vision, and natural language processing. In robotics, for example, active inference can be used to actively explore the environment, collect more informative data, and improve navigation and decision-making. In computer vision, active inference can be used to actively select the most informative images or viewpoints, improving object recognition or scene understanding.
Timeline:
1763: Bayes' theorem
1988: Bayesian networks
1994: Uncertainty Sampling
1997: Query by Committee algorithm
2017: Deep Bayesian Active Learning
2019: Bayesian Active Exploration
2020: Active Bayesian Inference for Deep Learning
2020: Bayesian Active Learning for Computer Vision
The synergy of active inference and Bayesian mechanics is expected to play a crucial role in shaping the next generation of AI systems. Some possible future developments in this area include:
- Combining active inference and Bayesian mechanics with other AI techniques, such as reinforcement learning and transfer learning, to create more powerful and flexible AI systems.
- Applying the synergy of active inference and Bayesian mechanics to new areas, such as healthcare, finance, and education, to improve decision-making and outcomes.
- Developing new algorithms and techniques that integrate active inference and Bayesian mechanics, such as Bayesian active learning for deep learning and Bayesian active exploration for robotics.
Dr. Sanjeev Namjosh: The Hidden Math Behind All Living Systems - On Active Inference, the Free Energy Principle, and Bayesian Mechanics (Machine Learning Street Talk, October 2024)
youtube
Saturday, October 26, 2024
#artificial intelligence#active learning#bayesian mechanics#machine learning#deep learning#robotics#computer vision#natural language processing#uncertainty quantification#decision making#probabilistic modeling#bayesian inference#active interference#ai research#intelligent systems#interview#ai assisted writing#machine art#Youtube
6 notes
·
View notes
Text
The ancient Greek craftsmen achieved a machining precision of 0.001 inches - a truly mind-boggling accomplishment for the era!
0 notes
Text
HWM: Game Design Log (25) Finding the Right “AI” System
Hey! I’m Omer from Oba Games, and I’m in charge of the game design for Hakan’s War Manager. I share a weekly game design log, and this time, I’ll be talking about AI and AI systems. Since I’m learning as I go, I thought, why not learn together? So, let’s get into it! Do We Need AI? Starting off with a simple question: Do we need AI? Now, I didn’t ask the most fundamental concept in game history…
View On WordPress
#AI system#Artificial Intelligence#Bayesian networks#decision tree#FSM#game design#game designer#game development#game mechanics#Hakan&039;s War Manager#Neural networks#state#state machine#strategy game
0 notes
Text
Interesting Papers for Week 51, 2024
Learning depends on the information conveyed by temporal relationships between events and is reflected in the dopamine response to cues. Balsam, P. D., Simpson, E. H., Taylor, K., Kalmbach, A., & Gallistel, C. R. (2024). Science Advances, 10(36).
Inferred representations behave like oscillators in dynamic Bayesian models of beat perception. Cannon, J., & Kaplan, T. (2024). Journal of Mathematical Psychology, 122, 102869.
Different temporal dynamics of foveal and peripheral visual processing during fixation. de la Malla, C., & Poletti, M. (2024). Proceedings of the National Academy of Sciences, 121(37), e2408067121.
Organizing the coactivity structure of the hippocampus from robust to flexible memory. Gava, G. P., Lefèvre, L., Broadbelt, T., McHugh, S. B., Lopes-dos-Santos, V., Brizee, D., … Dupret, D. (2024). Science, 385(6713), 1120–1127.
Saccade size predicts onset time of object processing during visual search of an open world virtual environment. Gordon, S. M., Dalangin, B., & Touryan, J. (2024). NeuroImage, 298, 120781.
Selective consistency of recurrent neural networks induced by plasticity as a mechanism of unsupervised perceptual learning. Goto, Y., & Kitajo, K. (2024). PLOS Computational Biology, 20(9), e1012378.
Measuring the velocity of spatio-temporal attention waves. Jagacinski, R. J., Ma, A., & Morrison, T. N. (2024). Journal of Mathematical Psychology, 122, 102874.
Distinct Neural Plasticity Enhancing Visual Perception. Kondat, T., Tik, N., Sharon, H., Tavor, I., & Censor, N. (2024). Journal of Neuroscience, 44(36), e0301242024.
Applying Super-Resolution and Tomography Concepts to Identify Receptive Field Subunits in the Retina. Krüppel, S., Khani, M. H., Schreyer, H. M., Sridhar, S., Ramakrishna, V., Zapp, S. J., … Gollisch, T. (2024). PLOS Computational Biology, 20(9), e1012370.
Nested compressed co-representations of multiple sequential experiences during sleep. Liu, K., Sibille, J., & Dragoi, G. (2024). Nature Neuroscience, 27(9), 1816–1828.
On the multiplicative inequality. McCausland, W. J., & Marley, A. A. J. (2024). Journal of Mathematical Psychology, 122, 102867.
Serotonin release in the habenula during emotional contagion promotes resilience. Mondoloni, S., Molina, P., Lecca, S., Wu, C.-H., Michel, L., Osypenko, D., … Mameli, M. (2024). Science, 385(6713), 1081–1086.
A nonoscillatory, millisecond-scale embedding of brain state provides insight into behavior. Parks, D. F., Schneider, A. M., Xu, Y., Brunwasser, S. J., Funderburk, S., Thurber, D., … Hengen, K. B. (2024). Nature Neuroscience, 27(9), 1829–1843.
Formalising the role of behaviour in neuroscience. Piantadosi, S. T., & Gallistel, C. R. (2024). European Journal of Neuroscience, 60(5), 4756–4770.
Cracking and Packing Information about the Features of Expected Rewards in the Orbitofrontal Cortex. Shimbo, A., Takahashi, Y. K., Langdon, A. J., Stalnaker, T. A., & Schoenbaum, G. (2024). Journal of Neuroscience, 44(36), e0714242024.
Sleep Consolidation Potentiates Sensorimotor Adaptation. Solano, A., Lerner, G., Griffa, G., Deleglise, A., Caffaro, P., Riquelme, L., … Della-Maggiore, V. (2024). Journal of Neuroscience, 44(36), e0325242024.
Input specificity of NMDA-dependent GABAergic plasticity in the hippocampus. Wiera, G., Jabłońska, J., Lech, A. M., & Mozrzymas, J. W. (2024). Scientific Reports, 14, 20463.
Higher-order interactions between hippocampal CA1 neurons are disrupted in amnestic mice. Yan, C., Mercaldo, V., Jacob, A. D., Kramer, E., Mocle, A., Ramsaran, A. I., … Josselyn, S. A. (2024). Nature Neuroscience, 27(9), 1794–1804.
Infant sensorimotor decoupling from 4 to 9 months of age: Individual differences and contingencies with maternal actions. Ying, Z., Karshaleva, B., & Deák, G. (2024). Infant Behavior and Development, 76, 101957.
Learning to integrate parts for whole through correlated neural variability. Zhu, Z., Qi, Y., Lu, W., & Feng, J. (2024). PLOS Computational Biology, 20(9), e1012401.
#neuroscience#science#research#brain science#scientific publications#cognitive science#neurobiology#cognition#psychophysics#neurons#neural computation#neural networks#computational neuroscience
14 notes
·
View notes
Text
Things That Are Hard
Some things are harder than they look. Some things are exactly as hard as they look.
Game AI, Intelligent Opponents, Intelligent NPCs
As you already know, "Game AI" is a misnomer. It's NPC behaviour, escort missions, "director" systems that dynamically manage the level of action in a game, pathfinding, AI opponents in multiplayer games, and possibly friendly AI players to fill out your team if there aren't enough humans.
Still, you are able to implement minimax with alpha-beta pruning for board games, pathfinding algorithms like A* or simple planning/reasoning systems with relative ease. Even easier: You could just take an MIT licensed library that implements a cool AI technique and put it in your game.
So why is it so hard to add AI to games, or more AI to games? The first problem is integration of cool AI algorithms with game systems. Although games do not need any "perception" for planning algorithms to work, no computer vision, sensor fusion, or data cleanup, and no Bayesian filtering for mapping and localisation, AI in games still needs information in a machine-readable format. Suddenly you go from free-form level geometry to a uniform grid, and from "every frame, do this or that" to planning and execution phases and checking every frame if the plan is still succeeding or has succeeded or if the assumptions of the original plan no longer hold and a new plan is on order. Intelligent behaviour is orders of magnitude more code than simple behaviours, and every time you add a mechanic to the game, you need to ask yourself "how do I make this mechanic accessible to the AI?"
Some design decisions will just be ruled out because they would be difficult to get to work in a certain AI paradigm.
Even in a game that is perfectly suited for AI techniques, like a turn-based, grid-based rogue-like, with line-of-sight already implemented, can struggle to make use of learning or planning AI for NPC behaviour.
What makes advanced AI "fun" in a game is usually when the behaviour is at least a little predictable, or when the AI explains how it works or why it did what it did. What makes AI "fun" is when it sometimes or usually plays really well, but then makes little mistakes that the player must learn to exploit. What makes AI "fun" is interesting behaviour. What makes AI "fun" is game balance.
You can have all of those with simple, almost hard-coded agent behaviour.
Video Playback
If your engine does not have video playback, you might think that it's easy enough to add it by yourself. After all, there are libraries out there that help you decode and decompress video files, so you can stream them from disk, and get streams of video frames and audio.
You can just use those libraries, and play the sounds and display the pictures with the tools your engine already provides, right?
Unfortunately, no. The video is probably at a different frame rate from your game's frame rate, and the music and sound effect playback in your game engine are probably not designed with syncing audio playback to a video stream.
I'm not saying it can't be done. I'm saying that it's surprisingly tricky, and even worse, it might be something that can't be built on top of your engine, but something that requires you to modify your engine to make it work.
Stealth Games
Stealth games succeed and fail on NPC behaviour/AI, predictability, variety, and level design. Stealth games need sophisticated and legible systems for line of sight, detailed modelling of the knowledge-state of NPCs, communication between NPCs, and good movement/ controls/game feel.
Making a stealth game is probably five times as difficult as a platformer or a puzzle platformer.
In a puzzle platformer, you can develop puzzle elements and then build levels. In a stealth game, your NPC behaviour and level design must work in tandem, and be developed together. Movement must be fluid enough that it doesn't become a challenge in itself, without stealth. NPC behaviour must be interesting and legible.
Rhythm Games
These are hard for the same reason that video playback is hard. You have to sync up your audio with your gameplay. You need some kind of feedback for when which audio is played. You need to know how large the audio lag, screen lag, and input lag are, both in frames, and in milliseconds.
You could try to counteract this by using certain real-time OS functionality directly, instead of using the machinery your engine gives you for sound effects and background music. You could try building your own sequencer that plays the beats at the right time.
Now you have to build good gameplay on top of that, and you have to write music. Rhythm games are the genre that experienced programmers are most likely to get wrong in game jams. They produce a finished and playable game, because they wanted to write a rhythm game for a change, but they get the BPM of their music slightly wrong, and everything feels off, more and more so as each song progresses.
Online Multi-Player Netcode
Everybody knows this is hard, but still underestimates the effort it takes. Sure, back in the day you could use the now-discontinued ready-made solution for Unity 5.0 to synchronise the state of your GameObjects. Sure, you can use a library that lets you send messages and streams on top of UDP. Sure, you can just use TCP and server-authoritative networking.
It can all work out, or it might not. Your netcode will have to deal with pings of 300 milliseconds, lag spikes, package loss, and maybe recover from five seconds of lost WiFi connections. If your game can't, because it absolutely needs the low latency or high bandwidth or consistency between players, you will at least have to detect these conditions and handle them, for example by showing text on the screen informing the player he has lost the match.
It is deceptively easy to build certain kinds of multiplayer games, and test them on your local network with pings in the single digit milliseconds. It is deceptively easy to write your own RPC system that works over TCP and sends out method names and arguments encoded as JSON. This is not the hard part of netcode. It is easy to write a racing game where players don't interact much, but just see each other's ghosts. The hard part is to make a fighting game where both players see the punches connect with the hit boxes in the same place, and where all players see the same finish line. Or maybe it's by design if every player sees his own car go over the finish line first.
50 notes
·
View notes
Text
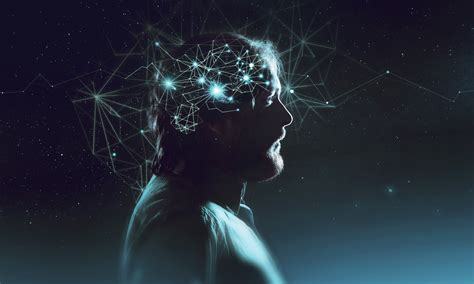
What Does Quantum Physics Imply About Consciousness?
In recent years much has been written about whether quantum mechanics (QM) does or does not imply that consciousness is fundamental to the cosmos. This is a problem that physicists have hotly debated since the earliest days of QM a century ago. It is extremely controversial; highly educated and famous physicists can't even agree on how to define the problem.
I have a degree in astrophysics and did some graduate level work in QM before switching to computer science; my Ph.D. addressed topics in cognitive science. So I'm going to give it a go to present an accessible and non-mathematical summary of the problem, hewing to as neutral a POV as I can manage. Due to the complexity of this subject I'm going to present it in three parts, with this being Part 1.
What is Quantum Mechanics?
First, a little background on QM. In science there are different types of theories. Some explain how phenomena work without predicting outcomes (e.g., Darwin's Theory of Evolution). Some predict outcomes without explaining how they work (e.g., Newton's Law of Gravity.)
QM is a purely predictive theory. It uses something called the wave function to predict the behavior of elementary particles such as electrons, photons, and so forth. The wave function expresses the probabilities of various outcomes, such as the likelihood that a photon will be recorded by a detection instrument. Before the physicist takes a measurement the wave function expresses what could happen; once the measurement is taken, it's no longer a question of probabilities because the event has happened (or not). The instrument recorded it. In QM this is called wave function collapse.
The Measurement Problem
When a wave function collapses, what does that mean in real terms? What does it imply about our familiar macroscopic world, and why do people keep saying it holds important implications for consciousness?
In QM this is called the Measurement Problem, first introduced in 1927 by physicist Werner Heisenberg as part of his famous Uncertainty Principle, and further developed by mathematician John Von Neumann in 1932. Heisenberg didn't attempt to explain what wave function collapse means in real terms; since QM is purely predictive, we're still not entirely sure what implications it may hold for the world we are familiar with. But one thing is certain: the predictions that QM makes are astonishingly accurate.
We just don't understand why they are so accurate. QM is undoubtedly telling us "something extremely important" about the structure of reality, we just don't know what that "something" is.
Interpretations of QM
But that hasn't stopped physicists from trying. There have been numerous attempts to interpret what implications QM might hold for the cosmos, or whether the wave function collapses at all. Some of these involve consciousness in some way; others do not.
Wave function collapse is required in these interpretations of QM:
The Copenhagen Interpretation (most commonly taught in physics programs)
Collective Collapse interpretations
The Transactional Interpretation
The Von Neumann-Wigner Interpretation
It is not required in these interpretations:
The Consistent Histories interpretation
The Bohm Interpretation
The Many Worlds Interpretation
Quantum Superdeterminism
The Ensemble Interpretation
The Relational Interpretation
This is not meant to be an exhaustive list, there are a boatload of other interpretations (e.g. Quantum Bayesianism). None of them should be taken as definitive since most of them are not falsifiable except via internal contradiction.
Big names in physics have lined up behind several of these (Steven Hawking was an advocate of the Many Worlds Interpretation, for instance) but that shouldn't be taken as anything more than a matter of personal philosophical preference. Ditto with statements of the form "most physicists agree with interpretation X" which has the same truth status as "most physicists prefer the color blue." These interpretations are philosophical in nature, and the debates will never end. As physicist M. David Mermin once observed: "New interpretations appear every year. None ever disappear."
What About Consciousness?
I began this post by noting that QM has become a major battlefield for discussions of the nature of consciousness (I'll have more to say about this in Part 2.) But linkages between QM and consciousness are certainly not new. In fact they have been raging since the wave function was introduced. Erwin Schrodinger said -
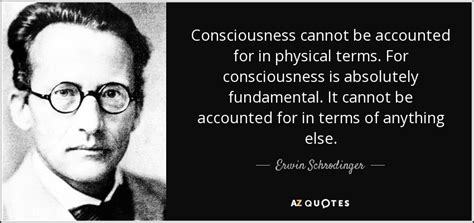
And Werner Heisenberg said -

In Part 2 I will look deeper at the connections between QM and consciousness with a review of philosopher Thomas Nagel's 2012 book Mind and Cosmos. In Part 3 I will take a look at how recent research into Near-Death Experiences and Terminal Lucidity hold unexpected implications for understanding consciousness.
(Image source: @linusquotes)
#quantum physics#consciousness#copenhagen interpretation#superdeterminism#many worlds#philosophy#physics#philosophy of mind#brain#consciousness series
107 notes
·
View notes
Text
Gerald: I've deduced that we're in some kind of temporal anomaly. Therefore I thought it better to not interact with too many elements to prevent a paradox from occurring. I'll continue to study the anomaly.
Shadow: actually, Professor, your study of the anomaly has already shaped the timeline. The Bayesian interpretation of quantum mechanics contends that the agent and the subject cannot be divorced from each other. The participation of the agent, or observer, that is to say: you, collaborates with the universe to create the universe. And if the universe were entirely material and wholly deterministic, there would be no room for free wi---
Gerald: my boy I don't give a shit
8 notes
·
View notes
Text
Speaker recognition refers to the process used to recognize a speaker from a spoken phrase (Furui, n.d. 1). It is a useful biometric tool with wide applications e.g. in audio or video document retrieval. Speaker recognition is dominated by two procedures namely segmentation and classification. Research and development have been ongoing to design new algorithms or to improve on old ones that are used for doing segmentation and classification. Statistical concepts dominate the field of speaker recognition and they are used for developing models. Machines that are used for speaker recognition purposes are referred to as automatic speech recognition (ASR) machines. ASR machines are either used to identify a person or to authenticate the person���s claimed identity (Softwarepractice, n.d., p.1). The following is a discussion of various improvements that have been suggested in the field of speaker recognition. Two processes that are of importance in doing speaker recognition are audio classification and segmentation. These two processes are carried out using computer algorithms. In developing an ideal procedure for the process of audio classification, it is important to consider the effect of background noise. Because of this factor, an auditory model has been put forward by Chu and Champagne that exhibits excellent performance even in a noisy background. To achieve such robustness in a noisy background the model inherently has a self-normalization mechanism. The simpler form of the auditory model is expressed as a three-stage processing progression through which an audio signal goes through an alteration to turn into an auditory spectrum, which is models inside neural illustration. Shortcomings associated with the use of this model are that it involves nonlinear processing and high computational requirements. These shortcomings necessitate the need for a simpler version of the model. A proposal put forward by the Chu and Champagne (2006)suggests modifications on the model that create a simpler version of it that is linear except in getting the square-root value of energy (p. 775). The modification is done on four of the original processing steps namely pre-emphasis, nonlinear compression, half-wave rectification, and temporal integration. To reduce its computational complexity the Parseval theorem is applied which enables the simplified model to be implemented in the frequency domain. The resultant effect of these modifications is a self-normalized FFT-based model that has been applied and tested in speech/music/noise classification. The test is done with the use of a support vector machine (SVM) as the classifier. The result of this test indicates that a comparison of the original and proposed auditory spectrum to a conventional FFT-based spectrum suggests a more robust performance in noisy environments (p.775). Additionally, the results suggest that by reducing the computational complexity, the performance of the conventional FFT-based spectrum is almost the same as that of the original auditory spectrum (p.775). One of the important processes in speaker recognition and in radio recordings is speech/music discrimination.. The discrimination is done using speech/music discriminators. The discriminator proposed by Giannakopoulos et al. involves a segmentation algorithm (V-809). Audio signals exhibit changes in the distribution of energy (RMS) and it is on this property that the audio segmentation algorithm is founded on. The discriminator proposed by Giannakopoulos et al involves the use of Bayesian networks (V-809). A strategic move, which is ideal in the classification stage of radio recordings, is the adoption of Bayesian networks. Each of the classifiers is trained on a single and distinct feature, thus, at any given classification nine features are involved in the process. By operating in distinct feature spaces, the independence between the classifiers is increased. This quality is desirable, as the results of the classifiers have to be combined by the Bayesian network in place. The nine commonly targeted features, which are extracted from an audio segment, are Spectral Centroid, Spectral Flux, Spectral Rolloff, Zero Crossing Rate, Frame Energy and 4 Mel-frequency cepstral coefficients. The new feature selection scheme that is integrated on the discriminator is based on the Bayesian networks (Giannakopoulos et al, V-809). Three Bayesian network architectures are considered and the performance of each is determined. The BNC Bayesian network has been determined experimentally, and found to be the best of the three owing to reduced error rate (Giannakopoulos et al, V-812). This proposed discriminator has worked on real internet broadcasts of the British Broadcasting Corporation (BBC) radio stations (Giannakopoulos et al, V-809). An important issue that arises in speaker recognition is the ability to determine the number of speakers involved in an audio session. Swamy et al. (2007) have put forward a mechanism that is able to determine the number of speakers (481). In this mechanism, the value is determined from multispeaker speech signals. According to Swamy et al., one pair of microphones that are spatially separated is sufficient to capture the speech signals (481). A feature of this mechanism is the time delay experienced in the arrival of these speech signals. This delay is because of the spatial separation of the microphones. The mechanism has its basis on the fact that different speakers will exhibit different time delay lengths. Thus, it is this variation in the length of the time delay, which is exploited in order to determine the number of speakers. In order to estimate the length of time delay, a cross-correlation procedure is undertaken. The procedure cross-correlates to the Hilbert envelopes, which correspond to linear prediction residuals of the speech signals. According to Zhang and Zhou (2004), audio segmentation is one of the most important processes in multimedia applications (IV-349). One of the typical problems in audio segmentation is accuracy. It is also desirable that the segmentation procedure can be done online. Algorithms that have attempted to deal with these two issues have one thing in common. The algorithms are designed to handle the classification of features at small-scale levels. These algorithms additionally result in high false alarm rates. Results obtained from experiments reveal that the classification of large-scale audio is easily compared to small-scale audio. It is this fact that has necessitated an extensive framework that increases robustness in audio segmentation. The proposed segmentation methodology can be described in two steps. In the first step, the segmentation is described as rough and the classification is large-scale. This step is taken as a measure of ensuring that there is integrality with respect to the content segments. By accomplishing this step you ensure that audio that is consecutive and that is from one source is not partitioned into different pieces thus homogeneity is preserved. In the second step, the segmentation is termed subtle and is undertaken to find segment points. These segment points correspond to boundary regions, which are the output of the first step. Results obtained from experiments also reveal that it is possible to achieve a desirable balance between the false alarm and low missing rate. The balance is desirable only when these two rates are kept at low levels (Zhang & Zhou, IV-349). According to Dutta and Haubold (2009), the human voice conveys speech and is useful in providing gender, nativity, ethnicity and other demographics about a speaker (422). Additionally, it also possesses other non-linguistic features that are unique to a given speaker (422). These facts about the human voice are helpful in doing audio/video retrieval. In order to do a classification of speaker characteristics, an evaluation is done on features that are categorized either as low-, mid- or high – level. MFCCs, LPCs, and six spectral features comprise the low-level features that are signal-based. Mid-level features are statistical in nature and used to model the low-level features. High-level features are semantic in nature and are found on specific phonemes that are selected. This describes the methodology that has been put forward by Dutta and Haubold (Dutta &Haubold, 2009, p.422). The data set that is used in assessing the performance of the methodology is made up of about 76.4 hours of annotated audio. In addition, 2786 segments that are unique to speakers are used for classification purposes. The results from the experiment reveal that the methodology put forward by Dutta and Haubold yields accuracy rates as high as 98.6% (Dutta & Haubold, 422). However, this accuracy rate is only achievable under certain conditions. The first condition is that test data is for male or female classification. The second condition to be observed is that in the experiment only mid-level features are used. The third condition is that the support vector machine used should posses a linear kernel. The results also reveal that mid- and high- level features are the most effective in identifying speaker characteristics. To automate the processes of speech recognition and spoken document retrieval the impact of unsupervised audio classification and segmentation has to be considered thoroughly. Huang and Hansen (2006) propose a new algorithm for audio classification to be used in automatic speech recognition (ASR) procedures (907). GMM networks that are weighted form the core feature of this new algorithm. Captured within this algorithm are the VSF and VZCR. VSF and VZCR are, additionally, extended-time features that are crucial to the performance of the algorithm. VSF and VZCR perform a pre-classification of the audio and additionally attach weights to the output probabilities of the GMM networks. After these two processes, the WGN networks implement the classification procedure. For the segmentation process in automatic speech recognition (ASR) procedures, Huang and Hansen (2006) propose a compound segmentation algorithm that captures 19 features (p.907). The figure below presents the features proposed Figure 1. Proposed features. Number required Feature name 1 2-mean distance metric 1 perceptual minimum variance distortionless response ( PMVDR) 1 Smoothed zero-crossing rate (SZCR) 1 False alarm compensation procedure 14 Filterbank log energy coefficients (FBLC) The 14 FBLCs proposed are implemented in 14 noisy environments where they are used to determine the best overall robust features with respect to these conditions. Turns lasting up to 5 seconds can be enhanced for short segment. In such case 2-mean distance metric is can be installed. The false alarm compensation procedure has been determined to boost efficiency of the rate at a cost effective manner. A comparison involving Huang and Hansen’s proposed classification algorithm against a GMM network baseline algorithm for classification reveals a 50% improvement in performance. Similarly, a comparison involving Huang and Hansen’s proposed compound segmentation algorithm against a baseline Mel-frequency cepstral coefficients (MFCC) and traditional Bayesian information criterion (BIC) algorithm reveals a 23%-10% improvement in all aspects (Huang and Hansen, 2006, p. 907). The data set used for the comparison procedure comprises of broadcast news evaluation data gotten from DARPA. DARPA is short for Defense Advanced Research Projects Agency. According to Huang and Hansen (2006), these two proposed algorithms achieve satisfactory results in the National Gallery of the Spoken Word (NGSW) corp, which is a more diverse, and challenging test. The basis of speaker recognition technology in use today is predominated by the process of statistical modeling. The statistical model formed is of short-time features that are extracted from acoustic speech signals. Two factors come into play when determining the recognition performance; these are the discrimination power associated with the acoustic features and the effectiveness of the statistical modeling techniques. The work of Chan et al is an analysis of the speaker discrimination power as it relates to two vocal features (1884). These two vocal features are either vocal source or conventional vocal tract related. The analysis draws a comparison between these two features. The features that are related to the vocal source are called wavelet octave coefficients of residues (WOCOR) and these have to be extracted from the audio signal. In order to perform the extraction process linear predictive (LP) residual signals have to be induced. This is because the linear predictive (LP) residual signals are compatible with the pitch-synchronous wavelet transform that perform the actual extraction. To determine between WOCOR and conventional MFCC features, which are least discriminative when a limit is placed on the amount of audio data consideration, is made to the degree of sensitivity to speech. Being less sensitive to spoken content and more discriminative in the face of a limited amount of training data are the two advantages that make WOCOR suitable for use in the task of speaker segmentation in telephone conversations (Chan et al, 1884). Such a task is characterized by building statistical speaker models upon short segments of speech. Additionally, experiments undertaken also reveal a significant reduction of errors associated with the segmentation process when WOCORs are used (Chan et al, 1884). Automatic speaker recognition (ASR) is the process through which a person is recognized from a spoken phrase by the aid of an ASR machine (Campbell, 1997, p.1437). Automatic speaker recognition (ASR) systems are designed and developed to operate in two modes depending on the nature of the problem to be solved. In one of the modes, they are used for identification purposes and in the other; they are used for verification or authentication purposes. In the first mode, the process is known as automatic speaker verification (ASV) while in the second the process is known as automatic speaker identification (ASI). In ASV procedures, the person’s claimed identity is authenticated by the ASR machine using the person’s voice. In ASI procedures unlike the ASV ones there is no claimed identity thus it is up to the ASR machine to determine the identity of the individual and the group to which the person belongs. Known sources of error in ASV procedures are shown in the table below Tab.2 Sources of verification errors. Misspoken or misread prompted phases Stress, duress and other extreme emotional states Multipath, noise and any other poor or inconsistent room acoustics The use of different microphones for verification and enrolment or any other cause of Chanel mismatch Sicknesses especially those that alter the vocal tract Aging Time varying microphone placement According to Campbell, a new automatic speaker recognition system is available and the recognizer is known to perform with 98.9% correct identification levels (p.1437 Signal acquisition is a basic building block for the recognizer. Feature extraction and selection is the second basic unit of the recognizer. Pattern matching is the third basic unit of the recognizer. A decision criterion is the fourth basic unit of the proposed recognizer. According to Ben-Harush et al. (2009), speaker diarization systems are used in assigning temporal speech segments in a conversation to the appropriate speaker (p.1). The system also assigns non-speech segments to non-speech. The problem that speaker diarization systems attempt to solve is captured in the query “who spoke when?” An inherent shortcoming in most of the diarization systems in use today is that they are unable to handle speech that is overlapped or co-channeled. To this end, algorithms have been developed in recent times seeking to address this challenge. However, most of these require unique conditions in order to perform and necessitate the need for high computational complexity. They also require that an audio data analysis with respect to time and frequency domain be undertaken. Ben-Harush et al. (2009) have proposed a methodology that uses frame based entropy analysis, Gaussian Mixture Modeling (GMM) and well known classification algorithms to counter this challenge (p.1). To perform overlapped speech detection, the methodology suggests an algorithm that is centered on a single feature. This single feature is an entropy analysis of the audio data in the time domain. To identify speech segments that are overlapped the methodology uses the combined force of Gaussian Mixture Modeling (GMM) and well-known classification algorithms. The methodology proposed by Ben-Harush et al is known to detect 60.0 % of frames containing overlapped speech (p.1). This value is achieved when the segmentation is at baseline level (p.1). It is capable of achieving this value while it maintains the rate of false alarm at 5 (p.1). Overlapped speech (OS) contributes to degrading the performance of automatic speaker recognition systems. Conversations over the telephone or during a meeting possess high quantities of overlapped speech. Du et al (200&) brings out audio segmentation as a problem in TV series, movies and other forms of practical media (I-205). Practical media exhibits audio segments of varying lengths but of these, short ones are easily noticeable due to their number. Through audio segmentation, an audio stream is broken down into parts that are homogenous with respect to speaker identity, acoustic class and environmental conditions..Du et al. (2007) has formulated an approach to unsupervised audio segmentation to be used in all forms of practical media. Included in this approach is a segmentation-stage at which potential acoustic changes are detected. Also included is a refinement-stage during which the detected acoustic changes are refined by a tri-model Bayesian Information Criterion (BIC). Results from experiments suggest that the approach possesses a high capability for detecting short segments (Du et al, I-205). Additionally, the results suggest that the tri-model BIC is effective in improving the overall segmentation performance (Du et al, I-205). According to Hosseinzadeh and Krishnan (2007), the concept of speaker recognition processes seven spectral features. The first of these spectral features is the Spectral centroid (SC). Hosseinzadeh and Krishnan (2007, p.205), state “the second spectral feature is Spectral bandwidth (SBW), the third is spectral band energy (SBE), the fourth is spectral crest factor (SCF), the fifth is Spectral flatness measure (SFM), the sixth is Shannon entropy (SE) and the seventh is Renyi entropy (RE)”. The seven features are used for quantification, which is important in speaker recognition since it is the case where vocal source information and the vocal tract function complements each other. The vocal truct function is determined specifically using two coefficients these are the MFCC and LPCC. MFCC stands for Mel frequency coefficients and LPCC stands for linear prediction cepstral coefficients. The quantification is quite significant in speaker detection as it is the container where verbal supply information and the verbal tract function are meant to balance. Very important in an experiment done to analyze the performance of these features is the use of a speaker identification system (SIS). ). A cohort Gaussian mixture model which is additionally text-independent is forms the ideal choice of a speaker identification method that is used in the experiment. The results from such an experiment reveal that these features achieve an identification accuracy of 99.33%. This accuracy level is achieved only when these features are combined with those that are MFCC based and additionally when undistorted speech is used. Read the full article
0 notes
Text
Advanced Statistical Methods for Data Analysts: Going Beyond the Basics
Introduction
Advanced statistical methods are a crucial toolset for data analysts looking to gain deeper insights from their data. While basic statistical techniques like mean, median, and standard deviation are essential for understanding data, advanced methods allow analysts to uncover more complex patterns and relationships.
Advanced Statistical Methods for Data Analysts
Data analysis has statistical theorems as its foundation. These theorems are stretched beyond basic applications to advanced levels by data analysts and scientists to fully exploit the possibilities of data science technologies. For instance, an entry-level course in any Data Analytics Institute in Delhi would cover the basic theorems of statistics as applied in data analysis while an advanced-level or professional course will teach learners some advanced theorems of statistics and how those theorems can be applied in data science. Some of the statistical theorems that extent beyond the basic ones are:
Regression Analysis: One key advanced method is regression analysis, which helps analysts understand the relationship between variables. For instance, linear regression can be utilised to estimate the value of a response variable using various input variables. This can be particularly useful in areas like demand forecasting and risk management.
Cluster Analysis: Another important method is cluster analysis, in which similar data points are grouped together. This can be handy for identifying patterns in data that may not be readily visible, such as customer segmentation in marketing.
Time Series Analysis: This is another advanced method that is used to analyse data points collected over time. This can be handy for forecasting future trends based on past data, such as predicting sales for the next quarter based on sales data from previous quarters.
Bayesian Inference: Unlike traditional frequentist statistics, Bayesian inference allows for the incorporation of previous knowledge or beliefs about a parameter of interest to make probabilistic inferences. This approach is particularly functional when dealing with small sample sizes or when prior information is available.
Survival Analysis: Survival analysis is used to analyse time-to-event data, such as the time until a patient experiences a particular condition or the time until a mechanical component fails. Techniques like Kaplan-Meier estimation and Cox proportional hazards regression are commonly used in survival analysis.
Spatial Statistics: Spatial statistics deals with data that have a spatial component, such as geographic locations. Techniques like spatial autocorrelation, spatial interpolation, and point pattern analysis are used to analyse spatial relationships and patterns.
Machine Learning: Machine learning involves advanced statistical techniques—such as ensemble methods, dimensionality reduction, and deep learning, that go beyond the fundamental theorems of statistics. These are typically covered in an advanced Data Analytics Course.
Causal Inference: Causal inference is used to identify causal relationships between variables dependent on observational data. Techniques like propensity score matching, instrumental variables, and structural equation modelling are used to estimate causal effects.
Text Mining and Natural Language Processing (NLP): Techniques in text mining and natural language processing are employed to analyse unstructured text data. NLP techniques simplify complex data analytics methods, rendering them comprehensible for non-technical persons. Professional data analysts need to collaborate with business strategists and decision makers who might not be technical experts. Many organisations in commercialised cities where data analytics is used for achieving business objectives require their workforce to gain expertise in NLP. Thus, a professional course from a Data Analytics Institute in Delhi would have many enrolments from both technical and non-technical professionals aspiring to acquire expertise in NLP.
Multilevel Modelling: Multilevel modelling, also known as hierarchical or mixed-effects modelling, helps with analysing nested structured data. This approach allows for the estimation of both within-group and between-group effects.
Summary
Overall, advanced statistical methods are essential for data analysts looking to extract meaningful insights from their data. By going beyond the basics, analysts can uncover hidden patterns and relationships that can lead to more informed decision-making. Statistical theorems are mandatory topics in any Data Analytics Course; only that the more advanced the course level, the more advanced the statistical theorems taught in the course.
0 notes
Text
Support: English, French; Cognitive Science, Computational Linguistics, Psycholinguistics, Writing Systems: Open, University of Montreal
The University of Montreal (Canada), in collaboration with Macquarie University (Australia), is recruiting a PhD student for a SSHRC-funded project on the cognitive and linguistic mechanisms underlying reading development and difficulties in bilingual children. The project combines the Dual-Route Cascaded model of reading with Bayesian computational modeling and cross-orthographic analyses to explore the interplay between cognitive processes and language experience in typical and atypical reader http://dlvr.it/TK3Pg3
0 notes
Text
In-vitro m
In-vitro measurements coupled with in-silico simulations for stochastic calibration and uncertainty quantification of the mechanical response of biological materials arXiv:2503.09900v1 Announce Type: new Abstract: In the standard Bayesian framework, a likelihood function is required, which can be difficult or computationally expensive to derive for non homogeneous biological materials under complex mechanical loading conditions. Here, we propose a simple and pr...
0 notes
Text
UFNO Machine Learning: A New Paradigm in AI Optimization
Introduction
Machine learning has seen rapid advancements in recent years, with models becoming more sophisticated and powerful. However, as complexity increases, challenges such as computational efficiency, scalability, and optimization persist. A new approach, UFNO Machine Learning (Unified Framework for Neural Optimization), aims to revolutionize the field by introducing a unified structure for model training, optimization, and adaptability.
This article explores UFNO Machine Learning, its core principles, advantages, and potential applications in various industries.
What is UFNO Machine Learning?
UFNO Machine Learning is a conceptual framework designed to enhance machine learning model efficiency by unifying multiple optimization techniques under a single umbrella. Unlike traditional models that rely on isolated optimization methods (such as gradient descent or evolutionary algorithms), UFNO integrates multiple strategies to improve adaptability and performance.
The core principles of UFNO Machine Learning include:
Unified Optimization Techniques – Combines gradient-based, evolutionary, and reinforcement learning strategies for robust optimization.
Adaptive Learning – Dynamically adjusts learning parameters based on real-time feedback.
Computational Efficiency – Reduces redundancy in training processes to save computational resources.
Scalability – Can be applied to various machine learning models, from small-scale neural networks to large-scale deep learning systems.
Key Components of UFNO Machine Learning
1. Multi-Modal Optimization
Traditional optimization techniques often focus on one methodology at a time. UFNO incorporates:
Gradient Descent Methods – Standard optimization using backpropagation.
Evolutionary Algorithms – Introduces genetic algorithms to enhance model adaptability.
Reinforcement Learning – Uses reward-based feedback mechanisms for self-improvement.
By fusing these methods, UFNO creates an adaptive optimization environment where the model selects the best approach depending on the scenario.
2. Automated Hyperparameter Tuning
One of the most time-consuming tasks in machine learning is hyperparameter tuning. UFNO automates this process through:
Bayesian Optimization – Predicts the best hyperparameters based on past iterations.
Neural Architecture Search (NAS) – Finds the best neural network configurations.
Real-Time Adjustment – Modifies parameters during training to avoid stagnation.
3. Scalability and Parallel Processing
Modern AI systems often require extensive computational power. UFNO optimizes resources through:
Distributed Computing – Divides tasks across multiple processors to improve speed.
Cloud Integration – Allows models to leverage cloud-based machine learning platforms for enhanced scalability.
Energy-Efficient Algorithms – Reduces computational wastage, making ML models more eco-friendly.
4. Self-Learning Mechanisms
Instead of relying solely on predefined learning paths, UFNO models employ:
Meta-Learning – Teaches models how to learn more efficiently based on past experiences.
Adaptive Loss Functions – Modifies loss functions dynamically to improve convergence.
Anomaly Detection – Identifies and corrects biases in real-time.
Advantages of UFNO Machine Learning
1. Increased Efficiency
By unifying optimization techniques, UFNO reduces the need for manual fine-tuning, leading to faster model convergence.
2. Higher Accuracy
With adaptive learning and self-correcting mechanisms, UFNO models achieve higher accuracy compared to traditional ML models.
3. Cost-Effective Training
By optimizing computational resources, UFNO reduces the financial burden of training complex models, making AI development more accessible.
4. Greater Generalization
Traditional models often suffer from overfitting. UFNO enhances generalization by using multiple optimization methods simultaneously, leading to robust models that perform well on unseen data.
5. Enhanced Real-World Adaptability
UFNO is particularly useful in dynamic environments, such as:
Finance – Predicting stock market trends with high adaptability.
Healthcare – Improving medical diagnosis with real-time learning.
Autonomous Systems – Enhancing decision-making in self-driving cars and robotics.
Applications of UFNO Machine Learning
1. Autonomous Systems
UFNO can significantly improve the performance of self-driving cars, drones, and industrial robots by enabling them to learn and adapt in real-time.
2. Financial Forecasting
By combining various optimization techniques, UFNO-based models can predict financial trends with higher accuracy, helping investors make informed decisions.
3. Healthcare and Medical Diagnosis
UFNO models can enhance predictive analytics in healthcare by analyzing patient data to detect diseases early and recommend treatments.
4. Natural Language Processing (NLP)
UFNO can optimize NLP models for better text recognition, language translation, and conversational AI applications.
5. Cybersecurity
By continuously learning from new threats, UFNO-based security systems can detect and prevent cyberattacks with greater efficiency.
Challenges and Future Prospects
While UFNO Machine Learning presents numerous advantages, it also comes with challenges:
Complexity in Implementation – Integrating multiple optimization methods requires sophisticated engineering.
Computational Overhead – Although it enhances efficiency, the initial setup may require significant computational power.
Adoption Barriers – Organizations may need to retrain their teams to effectively use UFNO methodologies.
However, as AI research continues to evolve, UFNO Machine Learning has the potential to become a standard framework for optimization in machine learning. With advancements in hardware and software, its implementation could become more accessible, making it a game-changer in the AI industry.
Conclusion
UFNO Machine Learning represents a novel approach to optimizing machine learning models by integrating multiple optimization techniques. With its focus on efficiency, scalability, and adaptability, it has the potential to reshape various industries, from finance and healthcare to autonomous systems and cybersecurity. While challenges remain, the promise of a unified framework for neural optimization could lead to breakthroughs in AI, making machine learning models smarter, faster, and more effective.
0 notes
Text
The Paradox of Probabilistic and Deterministic Worlds in AI and Quantum Computing
In the fascinating realms of artificial intelligence (AI) and quantum computing, a curious paradox emerges when we examine the interplay between algorithms and hardware. AI algorithms are inherently probabilistic, while the hardware they run on is deterministic. Conversely, quantum algorithms are deterministic, yet the hardware they rely on is probabilistic. This duality highlights the unique challenges and opportunities in these cutting-edge fields.
AI: Probabilistic Algorithms on Deterministic Hardware
AI algorithms, particularly those in machine learning, often rely on probabilistic methods to make predictions or decisions. Techniques like Bayesian inference, stochastic gradient descent, and Monte Carlo simulations are rooted in probability theory. These algorithms embrace uncertainty, using statistical models to approximate solutions where exact answers are computationally infeasible.
However, the hardware that executes these algorithms—traditional CPUs and GPUs—is deterministic. These processors follow precise instructions and produce predictable outcomes for given inputs. The deterministic nature of classical hardware ensures reliability and reproducibility, which are crucial for debugging and scaling AI systems. Yet, this mismatch between probabilistic algorithms and deterministic hardware can lead to inefficiencies, as the hardware isn't inherently designed to handle uncertainty.
Quantum Computing: Deterministic Algorithms on Probabilistic Hardware
In contrast, quantum computing presents an inverse scenario. Quantum algorithms, such as Shor's algorithm for factoring integers or Grover's algorithm for search problems, are deterministic. They are designed to produce specific, correct outcomes when executed correctly. However, the quantum hardware that runs these algorithms is inherently probabilistic.
Quantum bits (qubits) exist in superpositions of states, and their measurements yield probabilistic results. This probabilistic nature arises from the fundamental principles of quantum mechanics, such as superposition and entanglement. While quantum algorithms are designed to harness these phenomena to solve problems more efficiently than classical algorithms, the hardware's probabilistic behavior introduces challenges in error correction and result verification.
Bridging the Gap
The dichotomy between probabilistic algorithms and deterministic hardware in AI, and deterministic algorithms and probabilistic hardware in quantum computing, underscores the need for innovative approaches to bridge these gaps. In AI, researchers are exploring neuromorphic and probabilistic computing architectures that better align with the probabilistic nature of AI algorithms. These hardware innovations aim to improve efficiency and performance by embracing uncertainty at the hardware level.
In quantum computing, advancements in error correction and fault-tolerant designs are crucial to mitigate the probabilistic nature of quantum hardware. Techniques like quantum error correction codes and surface codes are being developed to ensure reliable and deterministic outcomes from quantum algorithms.
Conclusion
The interplay between probabilistic and deterministic elements in AI and quantum computing reveals the intricate balance required to harness the full potential of these technologies. As we continue to push the boundaries of computation, understanding and addressing these paradoxes will be key to unlocking new possibilities and driving innovation in both fields. Whether it's designing hardware that aligns with the probabilistic nature of AI or developing methods to tame the probabilistic behavior of quantum hardware, the journey promises to be as exciting as the destination.
0 notes
Text
Interesting Papers for Week 17, 2024
Computational mechanisms underlying latent value updating of unchosen actions. Ben-Artzi, I., Kessler, Y., Nicenboim, B., & Shahar, N. (2023). Science Advances, 9(42).
Associative learning or Bayesian inference? Revisiting backwards blocking reasoning in adults. Benton, D. T., & Rakison, D. H. (2023). Cognition, 241, 105626.
The value of mere completion. Converse, B. A., Tsang, S., & Hennecke, M. (2023). Journal of Experimental Psychology: General, 152(11), 3021–3036.
Stable sound decoding despite modulated sound representation in the auditory cortex. Funamizu, A., Marbach, F., & Zador, A. M. (2023). Current Biology, 33(20), 4470-4483.e7.
Differential attentional costs of encoding specific and gist episodic memory representations. Greene, N. R., & Naveh-Benjamin, M. (2023). Journal of Experimental Psychology: General, 152(11), 3292–3299.
The scaling of mental computation in a sorting task. Haridi, S., Wu, C. M., Dasgupta, I., & Schulz, E. (2023). Cognition, 241, 105605.
Simulations predict differing phase responses to excitation vs. inhibition in theta-resonant pyramidal neurons. Kelley, C., Antic, S. D., Carnevale, N. T., Kubie, J. L., & Lytton, W. W. (2023). Journal of Neurophysiology, 130(4), 910–924.
Attention preserves the selectivity of feature-tuned normalization. Klímová, M., Bloem, I. M., & Ling, S. (2023). Journal of Neurophysiology, 130(4), 990–998.
An approximate representation of objects underlies physical reasoning. Li, Y., Wang, Y., Boger, T., Smith, K. A., Gershman, S. J., & Ullman, T. D. (2023). Journal of Experimental Psychology: General, 152(11), 3074–3086.
Associative and predictive hippocampal codes support memory-guided behaviors. Liu, C., Todorova, R., Tang, W., Oliva, A., & Fernandez-Ruiz, A. (2023). Science, 382(6668).
Same but different: The latency of a shared expectation signal interacts with stimulus attributes. Lowe, B. G., Robinson, J. E., Yamamoto, N., Hogendoorn, H., & Johnston, P. (2023). Cortex, 168, 143–156.
Model-free decision making resists improved instructions and is enhanced by stimulus-response associations. Luna, R., Vadillo, M. A., & Luque, D. (2023). Cortex, 168, 102–113.
Infants’ sex affects neural responses to affective touch in early infancy. Mariani Wigley, I. L. C., Björnsdotter, M., Scheinin, N. M., Merisaari, H., Saunavaara, J., Parkkola, R., … Tuulari, J. J. (2023). Developmental Psychobiology, 65(7), e22419.
Action planning and execution cues influence economic partner choice. McEllin, L., Fiedler, S., & Sebanz, N. (2023). Cognition, 241, 105632.
Parallel processing of value-related information during multi-attribute decisions. Nakahashi, A., & Cisek, P. (2023). Journal of Neurophysiology, 130(4), 967–979.
Extended trajectory of spatial memory errors in typical and atypical development: The role of binding and precision. Peng, M., Lovos, A., Bottrill, K., Hughes, K., Sampsel, M., Lee, N. R., … Edgin, J. (2023). Hippocampus, 33(11), 1171–1188.
Mediodorsal thalamus-projecting anterior cingulate cortex neurons modulate helping behavior in mice. Song, D., Wang, C., Jin, Y., Deng, Y., Yan, Y., Wang, D., … Quan, Z. (2023). Current Biology, 33(20), 4330-4342.e5.
Metacognition and sense of agency. Wen, W., Charles, L., & Haggard, P. (2023). Cognition, 241, 105622.
Sensory deprivation arrests cellular and synaptic development of the night-vision circuitry in the retina. Wisner, S. R., Saha, A., Grimes, W. N., Mizerska, K., Kolarik, H. J., Wallin, J., … Hoon, M. (2023). Current Biology, 33(20), 4415-4429.e3.
The timing of confidence computations in human prefrontal cortex. Xue, K., Zheng, Y., Rafiei, F., & Rahnev, D. (2023). Cortex, 168, 167–175.
#neuroscience#science#research#brain science#scientific publications#cognitive science#neurobiology#cognition#psychophysics#neurons#computational neuroscience#neural computation#neural networks
16 notes
·
View notes
Text
Prompting for Deep Research (not ready for submission yet)
2/12/25
Act as a world-class cognitive scientist specializing in the intersection between the differences of human cognition and computational models. We are trying to develop a computation model of human cognition that we can then compare to actual human cognition or at least human performance. Your task is to provide an advanced, deeply-researched, detailed, long analysis on how human cognition differs from classical computation, with a focus on developing a model that systematically tests these differences. Structure your response with precision, drawing from cutting-edge research in cognitive science, neuroscience, and AI. Specifically, analyze how human perception from vision differs from current AI vision models that are compositional in terms of contextual awareness, object permanence, and generalization. Discuss how human time perception, with its distortions and event-based encoding, differs from the discrete, timestamped nature of computational memory. Examine human memory systems (episodic, semantic, procedural) in contrast to AI storage and retrieval mechanisms, identifying key limitations in classical computation's ability to reconstruct past events. Additionally, explore the human capacity for explanation and analogy, contrasting it with AI's reliance on pattern recognition and retrieval-augmented generation. Provide a detailed breakdown of experimental paradigms that could empirically test these cognitive differences, suggesting methodologies that quantify disparities in perception, recall, and reasoning between humans and computational models. Cite relevant theories, such as predictive processing, the Bayesian brain hypothesis, and hybrid cognitive architectures that integrate human-like intelligence with computational frameworks. Structure your response in four sections: (1) an overview of fundamental cognitive differences, (2) a synthesis of existing models attempting to bridge these gaps, (3) experimental designs for testing human vs. AI cognition, and (4) implications for developing more human-like AI systems. Use precise, technical language and reference key research papers to substantiate your claims.
Provide a structured research-backed report. We have no knowledge of existing models. Provide a comparative table of the specific types of cognition in which a computational model performs worse than human cognition. Present the performance using a computational model versus a human model by providing pros and cons. Additionally, design specific cognitive tests that would highlight these differences. I would like specific questions that a computational model and a human model could be tested on to show differences in performance. Take advantage of human cognitive priming, as well as anything else you can think of to show the flexibility of human cognition. Things like "the car slammed into the barrier" versus "the car glanced off the barrier" when showing the same video, etc. I want to know more about the flexibility of human cognition, versus the rigidity of classical computation, and how a computational theory of mind might not be correct. Where do we differ, and in what ways are we better than our AI counterparts?
0 notes
Text
Artificial Intelligence and Its Engineering Applications

This writing explores the impact of Artificial Intelligence (AI) on the engineering profession. It covers the evolution of AI, its applications in various engineering fields, the ethical and psychological considerations. The core argument is that AI is rapidly transforming engineering, presenting both opportunities and challenges that must be carefully addressed.
Intelligence may be defined as having six key traits: reasoning, understanding knowledge, the ability to plan, the ability to learn, the ability to communicate effectively, and integration of skills. AI seeks to replicate human cognitive abilities in machines. AI is categorized into three types based on the intelligence it exhibits:
Analytical AI: (Expert systems, supervised, unsupervised, and reinforcement learning) Primarily focused on cognitive intelligence, or pattern recognition and systematic thinking.
Human-Inspired AI: Includes both cognitive and emotional intelligence (adaptability, self-awareness, etc.).
Humanized AI: Incorporates cognitive, emotional, and social intelligence (empathy, teamwork, leadership).
Three evolutionary stages of AI may be identified:
Artificial Narrow Intelligence (ANI): Weak AI focused on specific tasks. Examples include facial recognition and route optimization.
Artificial General Intelligence (AGI): Strong AI capable of reasoning, planning, and problem-solving across a broad range of contexts. Experts predict this stage may be reached by 2040-2050.
Artificial Super Intelligence (ASI): High-level machine intelligence surpassing human cognitive abilities in almost all domains. Predictions suggest this could be attained around 2080.
There is a notable increase in AI related patents and publications related to Engineering. Additionally, revenue from AI-based software solutions is predicted to grow significantly, showcasing the rapid expansion of the field. VariousAI software platforms are now available that can assist Engineers. Examples include Microsoft Azure, TensorFlow, IBM Watson, and Infosys Nia.
Some major applications of AI in different Engineering disciplines are listed below.
Mechanical Engineering: AI is used in fracture mechanics (diagnostics, failure analysis), predictive maintenance of machinery, and designing smart machine tools. AI algorithms like k-NN, SVM, Deep Learning, Bayesian Networks, ANN, and CBR are utilized.
Civil Engineering: AI applications include structural engineering (modeling, design), construction management (monitoring), solid waste management (prediction, optimization), project management (risk assessment), and ocean engineering (analysis, simulation).
Electrical and Electronic Engineering: AI is applied in control engineering (plant-wide control), power systems (problem-solving), fault diagnosis and condition monitoring of electrical equipment, and image processing/computer vision.
Manufacturing: AI is being used to optimize the manufacturing process for Industry 4.0, make manufacturing more intelligent, and defend against cyber-attacks. AI is being integrated into Additive Manufacturing to optimize resource utilization, facilitate process control, and enhance data mining.
The ethical and psychological impacts of AI, briefly described below, should be treated carefully as AI applications in Engineering undergo significant growth.
AI Bias: AI algorithms are susceptible to biases present in their training datasets. This can lead to skewed or unfair decisions.
Military Applications: Ethical concerns arise from the use of AI in autonomous weapon systems, particularly around accountability for the actions of such systems.
Responsibility Gap: It is unclear who should be held responsible for decisions made by AI systems, such as a design flaw that causes a bridge to collapse.
Psychological Impacts: There is potential for technostress, burnout, and techno-addiction among engineers as a result of the rapid changes and demands created by AI. There is also potential for widening the economic gap, however that engineers are generally less likely to be affected due to the technical nature of their profession.
Need for Ethical Framework: There is a need for ethical frameworks for AI implementation, and educational efforts that integrate AI with ethical training to ensure proper use.
In conclusions, AI will significantly transform the engineering profession. AI is expected to increase productivity and shift engineers towards more knowledge-based tasks, which will likely require less time on manual labor. New disciplines and specializations in engineering are expected to emerge with advancements in AI. Predicting the future of AI and its precise impact is highly challenging, but the emergence of AGI and potentially ASI will fundamentally alter the role of an engineer.
0 notes