#Energy-Efficient Computing Technologies
Explore tagged Tumblr posts
Text
#Artificial Intelligence Trends 2025#Blockchain Innovations 2025#Energy-Efficient Computing Technologies#Impact of AI on Future Industries#Quantum Computing Applications in Business
0 notes
Text
Exploring the Growing $21.3 Billion Data Center Liquid Cooling Market: Trends and Opportunities
In an era marked by rapid digital expansion, data centers have become essential infrastructures supporting the growing demands for data processing and storage. However, these facilities face a significant challenge: maintaining optimal operating temperatures for their equipment. Traditional air-cooling methods are becoming increasingly inadequate as server densities rise and heat generation intensifies. Liquid cooling is emerging as a transformative solution that addresses these challenges and is set to redefine the cooling landscape for data centers.
What is Liquid Cooling?
Liquid cooling systems utilize liquids to transfer heat away from critical components within data centers. Unlike conventional air cooling, which relies on air to dissipate heat, liquid cooling is much more efficient. By circulating a cooling fluid—commonly water or specialized refrigerants—through heat exchangers and directly to the heat sources, data centers can maintain lower temperatures, improving overall performance.
Market Growth and Trends
The data centre liquid cooling market is on an impressive growth trajectory. According to industry analysis, this market is projected to grow USD 21.3 billion by 2030, achieving a remarkable compound annual growth rate (CAGR) of 27.6%. This upward trend is fueled by several key factors, including the increasing demand for high-performance computing (HPC), advancements in artificial intelligence (AI), and a growing emphasis on energy-efficient operations.
Key Factors Driving Adoption
1. Rising Heat Density
The trend toward higher power density in server configurations poses a significant challenge for cooling systems. With modern servers generating more heat than ever, traditional air cooling methods are struggling to keep pace. Liquid cooling effectively addresses this issue, enabling higher density server deployments without sacrificing efficiency.
2. Energy Efficiency Improvements
A standout advantage of liquid cooling systems is their energy efficiency. Studies indicate that these systems can reduce energy consumption by up to 50% compared to air cooling. This not only lowers operational costs for data center operators but also supports sustainability initiatives aimed at reducing energy consumption and carbon emissions.
3. Space Efficiency
Data center operators often grapple with limited space, making it crucial to optimize cooling solutions. Liquid cooling systems typically require less physical space than air-cooled alternatives. This efficiency allows operators to enhance server capacity and performance without the need for additional physical expansion.
4. Technological Innovations
The development of advanced cooling technologies, such as direct-to-chip cooling and immersion cooling, is further propelling the effectiveness of liquid cooling solutions. Direct-to-chip cooling channels coolant directly to the components generating heat, while immersion cooling involves submerging entire server racks in non-conductive liquids, both of which push thermal management to new heights.
Overcoming Challenges
While the benefits of liquid cooling are compelling, the transition to this technology presents certain challenges. Initial installation costs can be significant, and some operators may be hesitant due to concerns regarding complexity and ongoing maintenance. However, as liquid cooling technology advances and adoption rates increase, it is expected that costs will decrease, making it a more accessible option for a wider range of data center operators.
The Competitive Landscape
The data center liquid cooling market is home to several key players, including established companies like Schneider Electric, Vertiv, and Asetek, as well as innovative startups committed to developing cutting-edge thermal management solutions. These organizations are actively investing in research and development to refine the performance and reliability of liquid cooling systems, ensuring they meet the evolving needs of data center operators.
Download PDF Brochure :
The outlook for the data center liquid cooling market is promising. As organizations prioritize energy efficiency and sustainability in their operations, liquid cooling is likely to become a standard practice. The integration of AI and machine learning into cooling systems will further enhance performance, enabling dynamic adjustments based on real-time thermal demands.
The evolution of liquid cooling in data centers represents a crucial shift toward more efficient, sustainable, and high-performing computing environments. As the demand for advanced cooling solutions rises in response to technological advancements, liquid cooling is not merely an option—it is an essential element of the future data center landscape. By embracing this innovative approach, organizations can gain a significant competitive advantage in an increasingly digital world.
#Data Center#Liquid Cooling#Energy Efficiency#High-Performance Computing#Sustainability#Thermal Management#AI#Market Growth#Technology Innovation#Server Cooling#Data Center Infrastructure#Immersion Cooling#Direct-to-Chip Cooling#IT Solutions#Digital Transformation
2 notes
·
View notes
Text
Why Quantum Computing Will Change the Tech Landscape
The technology industry has seen significant advancements over the past few decades, but nothing quite as transformative as quantum computing promises to be. Why Quantum Computing Will Change the Tech Landscape is not just a matter of speculation; it’s grounded in the science of how we compute and the immense potential of quantum mechanics to revolutionise various sectors. As traditional…
#AI#AI acceleration#AI development#autonomous vehicles#big data#classical computing#climate modelling#complex systems#computational power#computing power#cryptography#cybersecurity#data processing#data simulation#drug discovery#economic impact#emerging tech#energy efficiency#exponential computing#exponential growth#fast problem solving#financial services#Future Technology#government funding#hardware#Healthcare#industry applications#industry transformation#innovation#machine learning
3 notes
·
View notes
Text
How Sustainability Business Practices Powering Green Technology
When we talk about sustainability, it is no longer just a buzzword—it's a driving force behind innovation and growth. As our businesses strive to reduce their environmental footprint, green technology is emerging as a powerful ally. We can invest in renewable energy sources like solar and wind and transform how we power our data centers, significantly offsetting energy consumption. But sustainability goes beyond clean energy; it's about optimizing operations and creating a brighter, more efficient future for our businesses and the planet.
From adopting cloud computing to managing e-waste responsibly and training employees on eco-friendly practices, businesses are integrating sustainable strategies that benefit the planet and enhance efficiency and competitiveness. The future of green technology, particularly in optimizing energy consumption, lies in harnessing AI and IoT. These technologies can identify inefficiencies and automate energy management, offering eco-friendly services and solutions that are both innovative and sustainable.
As companies embrace sustainability, the shift towards green technology reshapes the entire business ecosystem. Optimizing data centers—traditionally one of the largest energy consumers—is now a top priority. By leveraging cloud computing, businesses can drastically reduce their carbon footprint, improving energy efficiency while cutting costs. We can manage e-waste and ensure that equipment is recycled.
Training employees in sustainable practices and fostering a culture of environmental responsibility throughout the organization is not just a task; it's a mission. This holistic approach to sustainability meets growing consumer demands for eco-consciousness and positions businesses toward a greener and more resilient future. Each of us has a crucial role in this transition, and together, we can make a significant impact.
Sustainable Business Practices Helping Technology Go Green

1-Energy-Efficient Facilities
Implementing energy-efficient facilities involves upgrading buildings with advanced insulation, smart lighting management, and smart energy management systems. This approach reduces energy consumption, lowers operational costs, and minimizes the overall carbon footprint, supporting long-term sustainability.
2-Adopt the Cloud
Cloud computing allows businesses to reduce energy usage by consolidating IT infrastructure. Cloud providers often use energy-efficient, renewable-powered data centers, enabling companies to streamline operations, enhance scalability, and contribute to global energy conservation.
3-Embrace Green Computing Practices
Green computing involves using energy-efficient hardware, optimizing software, and implementing power management strategies. By adopting these practices, businesses can lower energy consumption, reduce electronic waste, and maintain productivity while minimizing environmental impact.
4-Establish Internal Equipment Recycling
Internal equipment recycling programs ensure outdated technology is repurposed, donated, or recycled responsibly. Partnering with certified recyclers helps businesses reduce e-waste, safely dispose of hazardous materials, and demonstrate a commitment to sustainability.
5-AI and IoT To Optimize Energy Consumption
AI and IoT technologies are the future. They monitor and optimize energy usage in real-time. By identifying inefficiencies and automating energy management, businesses can reduce waste, enhance operational efficiency, and significantly lower their carbon footprint. The potential of these technologies is truly exciting and promising for a greener future.
Why Web Synergies?
As business environments move forward, the challenges of a rapidly changing world, embracing sustainable practices is not just an option—it's a necessity. Integrating green technology into every aspect of your operations can reduce environmental impact while enhancing efficiency and innovation.
At Web Synergies, we understand the power of sustainability in driving long-term success. Our commitment to helping businesses adopt eco-friendly solutions, from optimizing data centers to leveraging AI and IoT, positions us as a trusted partner in your journey toward a greener future. We can create a more sustainable world where technology drives progress and protects the planet for future generations.
#sustainable business practices#green technology solutions#eco-friendly business strategies#sustainability in technology#sustainable IT solutions#energy-efficient data centres#cloud computing for sustainability#green computing practices#AI for energy optimisation#IoT in sustainable business#internal equipment recycling#managing e-waste in tech
0 notes
Text
Green Bytes: The Journey Towards Sustainable IT & Green Computing.
Sanjay Kumar Mohindroo Sanjay Kumar Mohindroo. skm.stayingalive.in This post explores sustainable IT practices, energy-efficient computing, e-waste reduction, and green digital infrastructures to spark dialogue and inspire action.
A New Era for IT Embracing Change for a Greener Tomorrow In our fast-moving world, technology drives our lives in every way. We see power in every byte we use and…
#Clean Tech#Digital Infrastructures#E-Waste Reduction#Eco-Friendly IT#Energy-Efficient Computing#Green Computing#Green Technology#News#Sanjay Kumar Mohindroo#Smart Tech#Sustainable Data Centers#Sustainable IT
0 notes
Text
The AI Efficiency Paradox
Understanding Jevons Paradox
Jevons Paradox occurs when technological progress increases the efficiency of resource use, but the rate of consumption of that resource rises due to increasing demand. The core mechanism is simple: as efficiency improves, costs decrease, making the resource more accessible and creating new use cases, ultimately driving up total consumption.
In the 1860s, economist William Stanley Jevons made a counterintuitive observation about coal consumption during the Industrial Revolution. Despite significant improvements in steam engine efficiency, coal consumption increased rather than decreased. This phenomenon, later termed "Jevons Paradox," suggests that technological improvements in resource efficiency often lead to increased consumption rather than conservation. Today, as artificial intelligence transforms our world, we're witnessing a similar pattern that raises important questions about technology, resource usage, and societal impact.
The AI Parallel
Artificial intelligence presents a modern manifestation of Jevons Paradox across multiple dimensions:
Computational Resources
While AI models have become more efficient in terms of performance per computation, the total demand for computational resources has skyrocketed. Each improvement in AI efficiency enables more complex applications, larger models, and broader deployment, leading to greater overall energy consumption and hardware demands.
Human Labor and Productivity
AI tools promise to make human work more efficient, potentially reducing the labor needed for specific tasks. However, this efficiency often creates new demands and opportunities for human work rather than reducing overall labor requirements. For instance, while AI might automate certain aspects of programming, it has simultaneously increased the complexity and scope of software development projects.
Data Usage
As AI systems become more efficient at processing data, organizations collect and analyze ever-larger datasets. The improved efficiency in data processing doesn't lead to using less data – instead, it drives an exponential increase in data collection and storage needs.
Implications for Society and Technology
The AI manifestation of Jevons Paradox has several important implications:
Resource Consumption
Despite improvements in AI model efficiency, the total environmental impact of AI systems continues to grow. This raises important questions about sustainability and the need for renewable energy sources to power AI infrastructure.
Economic Effects
The paradox suggests that AI efficiency gains might not lead to reduced resource consumption or costs at a macro level, but rather to expanded applications and new markets. This has significant implications for business planning and economic policy.
Social Impact
As AI makes certain tasks more efficient, it doesn't necessarily reduce human workload but often transforms it, creating new roles and responsibilities. This challenges the simple narrative of AI leading to widespread job displacement.
Addressing the Paradox
Understanding the AI efficiency paradox is crucial for developing effective policies and strategies:
Resource Planning: Organizations need to plan for increased resource demands rather than assuming efficiency improvements will reduce consumption.
Sustainability Initiatives: The paradox highlights the importance of coupling AI development with renewable energy and sustainable computing initiatives.
Policy Considerations: Regulators and policymakers should consider Jevons Paradox when developing AI governance frameworks and resource management policies.
Looking Forward
As AI technology continues to evolve, the implications of Jevons Paradox become increasingly relevant. The challenge lies not in preventing the paradox – which may be inherent to technological progress – but in managing its effects responsibly. This requires:
- Investment in sustainable infrastructure to support growing AI resource demands
- Development of policies that account for rebound effects in resource consumption
- Careful consideration of how efficiency improvements might reshape rather than reduce resource usage
The parallels between historical patterns of resource consumption and modern AI development offer valuable lessons for technology leaders, policymakers, and society at large. As we continue to push the boundaries of AI capability, understanding and accounting for Jevons Paradox will be crucial for sustainable and responsible technological progress.
#linklayer#blog#artificial intelligence#efficiency#innovation#technology#sustainability#jevons paradox#greentech#work in progress#growth#efficient#infrastructure#energy consumption#energy#energy conservation#plans#global#opportunity#computer science#science#sustainable#climate science
0 notes
Text
Reimagining the Energy Landscape: AI's Growing Hunger for Computing Power #BlogchatterA2Z
Reimagining the Energy Landscape: AI's Growing Hunger for Computing Power #BlogchatterA2Z #AIdevelopment #energyConsumption #DataCenterInfrastructure #ArmHoldings #energyEfficiency #SustainableTechnology #RenewableEnergy #EdgeComputing #RegulatoryMeasures
Navigating the Energy Conundrum: AI’s Growing Hunger for Computing Power In the ever-expanding realm of artificial intelligence (AI), the voracious appetite for computing power threatens to outpace our energy sources, sparking urgent calls for a shift in approach. According to Rene Haas, Chief Executive Officer of Arm Holdings Plc, by the year 2030, data centers worldwide are projected to…
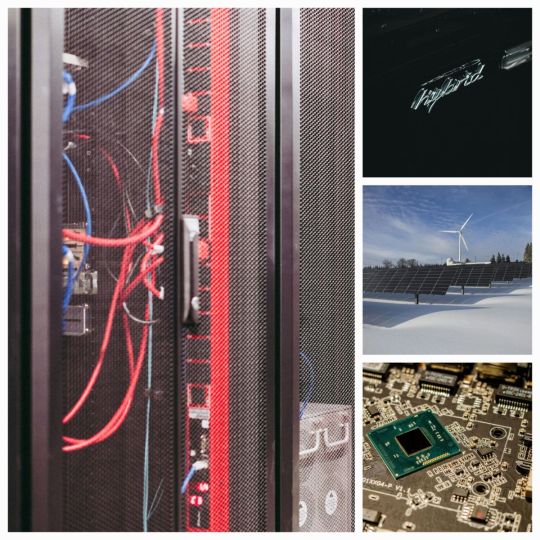
View On WordPress
#AI Development#Arm Holdings#Custom-built chips#Data center infrastructure#Edge computing#Energy consumption#energy efficiency#Regulatory measures#Renewable Energy#Sustainable technology
0 notes
Text
Artificial Intelligence: Energy Consumption Beyond Bitcoin and Blockchain Mining

As the world becomes increasingly digital, the demand for computational power is skyrocketing. Daniel Reitberg dives into the energy-intensive world of artificial intelligence, shedding light on its voracious appetite for energy.
The Digital Revolution's Dark Secret
While blockchain and Bitcoin mining have been criticized for their energy consumption, artificial intelligence operates on an entirely different scale. Daniel Reitberg reveals that the energy usage of AI dwarfs these digital counterparts.
AI: The Energy Gobbler
The rapid growth of AI, particularly deep learning models, has led to enormous energy requirements. Training large language models, for example, can consume as much energy as a small town.
The Carbon Footprint of AI
AI's colossal energy consumption translates to a significant carbon footprint. As the world grapples with climate change, understanding and mitigating AI's environmental impact are crucial.
Why AI Consumes So Much Energy
Daniel Reitberg explains that AI's energy consumption arises from its complex algorithms and massive datasets. High-performance computing, power-hungry GPUs, and energy-intensive data centers all play a role.
Green AI: A New Imperative
Green AI is emerging as a critical field. It focuses on developing energy-efficient algorithms, hardware, and data center operations without compromising AI's capabilities.
Energy-Efficient Algorithms
Optimizing algorithms to achieve the same results with less computation is a central focus. Daniel Reitberg explores how AI researchers are making strides in energy-efficient model design.
AI Hardware Innovations
Developing energy-efficient hardware is another facet of green AI. New, specialized chips and GPUs are being designed to minimize power consumption.
Eco-Friendly Data Centers
Transitioning to renewable energy sources and implementing energy-efficient cooling systems in data centers is imperative. This not only reduces energy consumption but also lowers the carbon footprint of AI.
Decentralized AI
Edge computing is changing the game by decentralizing AI processing. This reduces energy consumption by bringing AI closer to the data source.
AI's Role in Sustainability
Ironically, AI can also play a pivotal role in sustainability. Daniel Reitberg discusses how AI is used to optimize resource allocation, reduce waste, and enhance energy efficiency in various industries.
Responsibility and Regulation
As AI's energy consumption comes under scrutiny, governments and organizations are considering regulations to promote eco-friendly AI practices.
Balancing Innovation and Sustainability
Daniel Reitberg's exploration of AI's energy consumption paints a picture of a powerful technology with an Achilles' heel. As AI becomes increasingly integrated into our lives, striking a balance between innovation and sustainability is essential. AI's potential is boundless, but so are the responsibilities to our planet.
#artificial intelligence#machine learning#deep learning#technology#robotics#energy consumption#bitcoin#blockchain#blockchainmining#bitcoinmining#decentralized#data center#algorithm#green computing#energy efficiency#sustainable energy
0 notes
Text
Distribution Feeder Automation Market Business Research, Types and Applications, Demand by 2032
Market Overview: The Distribution Feeder Automation Market refers to the market for advanced technologies and systems that automate the monitoring, control, and management of distribution feeders within an electrical distribution network. Distribution feeder automation improves the efficiency, reliability, and resiliency of power distribution by utilizing sensors, communication networks, and automation software to monitor and control power flows, fault detection, and restoration. These solutions enhance the performance of distribution feeders and enable utilities to deliver electricity more effectively.
Feeder Automation Market is projected to be worth USD 7.85 Billion by 2030, registering a CAGR of 8.2% during the forecast period (2022 - 2030)
Demand: The demand for distribution feeder automation is driven by several factors, including:
Distribution feeder automation solutions help utilities improve the reliability and resiliency of their distribution networks. By automating fault detection, isolation, and restoration, these systems minimize outage durations and enhance the overall performance of the grid, ensuring a more reliable power supply for customers.
Distribution feeder automation systems streamline operations by reducing manual interventions, optimizing power flow, and enhancing network monitoring capabilities. These solutions enable utilities to manage distribution feeders more efficiently, reduce costs, and improve the overall operational performance of their networks.
The increasing integration of renewable energy sources, such as solar and wind power, into the distribution grid presents operational challenges. Distribution feeder automation helps utilities manage the intermittent nature of renewables, optimize power flow, and ensure grid stability, facilitating the integration of clean energy sources.
Latest technological developments, key factors, and challenges in the Distribution Feeder Automation Market:
Latest Technological Developments:
Intelligent Sensors and IoT Integration: Distribution feeder automation is leveraging intelligent sensors and Internet of Things (IoT) integration to monitor real-time data from various points along the distribution feeders. These sensors provide insights into voltage levels, current flow, fault detection, and other parameters, enabling quicker fault localization and resolution.
Advanced Communication Protocols: Modern distribution feeder automation systems are adopting advanced communication protocols like for seamless data exchange between field devices and control centers. This facilitates real-time monitoring, remote control, and efficient data transmission.
Decentralized Control and Edge Computing: Distribution feeder automation systems are moving toward decentralized control and edge computing. This allows decision-making and control to occur closer to field devices, reducing latency and enhancing responsiveness.
Distributed Energy Resource (DER) Management: With the integration of distributed energy resources like solar panels, wind turbines, and energy storage systems, feeder automation systems are being developed to manage these resources effectively, ensuring grid stability and optimal energy distribution.
Advanced Analytics and AI: Distribution feeder automation is incorporating advanced analytics and artificial intelligence to analyze data from various sources. AI algorithms can predict and prevent potential faults, optimize energy flows, and enhance overall feeder performance.
Key Factors:
Reliability Enhancement: Distribution feeder automation improves the reliability of electricity distribution by enabling quicker fault detection, isolation, and restoration. This minimizes outage durations and enhances overall grid reliability.
Efficient Grid Management: Feeder automation allows utilities to manage the distribution grid more efficiently. Load balancing, voltage regulation, and fault management can be automated, leading to optimized energy delivery.
Integration of Renewable Energy: As the penetration of renewable energy sources increases, distribution feeder automation becomes crucial for managing the intermittent nature of these resources and maintaining grid stability.
Grid Resilience and Outage Management: Feeder automation systems enhance grid resilience by providing real-time data on grid conditions and faults. This facilitates faster response and restoration during outages, minimizing customer impact.
Challenges:
Interoperability: Integrating various devices and protocols into a cohesive feeder automation system can be challenging due to the need for interoperability between different vendors and technologies.
Cybersecurity: With increased connectivity and data exchange, distribution feeder automation systems face cybersecurity threats. Ensuring the security of these systems is paramount to prevent unauthorized access and data breaches.
Cost and Infrastructure: Implementing distribution feeder automation can involve significant upfront costs, including hardware, software, and training. Retrofitting existing infrastructure for automation may also pose challenges.
Complexity of Data Management: Feeder automation generates vast amounts of data that need to be effectively managed, analyzed, and acted upon. Handling this complexity can be demanding.
Change Management: Transitioning from manual to automated processes requires change management efforts to train personnel, address resistance, and ensure smooth integration.
Maintenance and Upgrades: Ensuring the proper functioning of feeder automation systems over time requires regular maintenance and potential upgrades to keep up with technology advancements.
Distribution feeder automation is at the forefront of modernizing electricity distribution networks. While it offers significant benefits in terms of reliability, efficiency, and resilience, addressing technical challenges and ensuring a smooth transition is crucial for successful implementation.
By visiting our website or contacting us directly, you can explore the availability of specific reports related to this market. These reports often require a purchase or subscription, but we provide comprehensive and in-depth information that can be valuable for businesses, investors, and individuals interested in this market.
“Remember to look for recent reports to ensure you have the most current and relevant information.”
Click Here, To Get Free Sample Report: https://stringentdatalytics.com/sample-request/distribution-automation-solutions-market/10965/
Market Segmentations:
Global Distribution Feeder Automation Market: By Company
• ABB
• Eaton
• Grid Solutions
• Schneider Electric
• Siemens
• Advanced Control Systems
• Atlantic City Electric
• CG
• G&W Electric
• Kalkitech
• Kyland Technology
• Moxa
• S&C Electric Company
• Schweitzer Engineering Laboratories (SEL)
Global Distribution Feeder Automation Market: By Type
• Fault Location
• Isolation
• Service Restoration
• Automatic Transfer Scheme
Global Distribution Feeder Automation Market: By Application
• Industrial
• Commercial
• Residential
Global Distribution Feeder Automation Market: Regional Analysis
The regional analysis of the global Distribution Feeder Automation market provides insights into the market's performance across different regions of the world. The analysis is based on recent and future trends and includes market forecast for the prediction period. The countries covered in the regional analysis of the Distribution Feeder Automation market report are as follows:
North America: The North America region includes the U.S., Canada, and Mexico. The U.S. is the largest market for Distribution Feeder Automation in this region, followed by Canada and Mexico. The market growth in this region is primarily driven by the presence of key market players and the increasing demand for the product.
Europe: The Europe region includes Germany, France, U.K., Russia, Italy, Spain, Turkey, Netherlands, Switzerland, Belgium, and Rest of Europe. Germany is the largest market for Distribution Feeder Automation in this region, followed by the U.K. and France. The market growth in this region is driven by the increasing demand for the product in the automotive and aerospace sectors.
Asia-Pacific: The Asia-Pacific region includes Singapore, Malaysia, Australia, Thailand, Indonesia, Philippines, China, Japan, India, South Korea, and Rest of Asia-Pacific. China is the largest market for Distribution Feeder Automation in this region, followed by Japan and India. The market growth in this region is driven by the increasing adoption of the product in various end-use industries, such as automotive, aerospace, and construction.
Middle East and Africa: The Middle East and Africa region includes Saudi Arabia, U.A.E, South Africa, Egypt, Israel, and Rest of Middle East and Africa. The market growth in this region is driven by the increasing demand for the product in the aerospace and defense sectors.
South America: The South America region includes Argentina, Brazil, and Rest of South America. Brazil is the largest market for Distribution Feeder Automation in this region, followed by Argentina. The market growth in this region is primarily driven by the increasing demand for the product in the automotive sector.
Click Here, To Buy Report: https://stringentdatalytics.com/purchase/distribution-feeder-automation-market/10966/?license=single
Reasons to Purchase Distribution Feeder Automation Market Report:
Comprehensive Market Insights: Global research market reports provide a thorough and in-depth analysis of a specific market or industry. They offer valuable insights into market size, growth potential, trends, challenges, and opportunities, helping businesses make informed decisions and formulate effective strategies.
Market Analysis and Forecasts: These reports provide detailed analysis and forecasts of market trends, growth rates, and future market scenarios. They help businesses understand the current market landscape and anticipate future market developments, enabling them to plan and allocate resources accordingly.
Competitive Intelligence: Global research market reports provide a competitive landscape analysis, including information about key market players, their market share, strategies, and product portfolios. This information helps businesses understand their competitors' strengths and weaknesses, identify market gaps, and develop strategies to gain a competitive advantage.
Industry Trends and Insights: These reports offer insights into industry-specific trends, emerging technologies, and regulatory frameworks. Understanding industry dynamics and staying updated on the latest trends can help businesses identify growth opportunities and stay ahead in a competitive market.
Investment and Expansion Opportunities: Global research market reports provide information about investment opportunities, potential markets for expansion, and emerging growth areas. These reports help businesses identify untapped markets, assess the feasibility of investments, and make informed decisions regarding expansion strategies.
Risk Mitigation: Market reports provide risk assessment and mitigation strategies. By analyzing market dynamics, potential challenges, and regulatory frameworks, businesses can proactively identify risks and develop strategies to mitigate them, ensuring better risk management and decision-making.
Cost and Time Efficiency: Conducting comprehensive market research independently can be time-consuming and expensive. Purchasing a global research market report provides a cost-effective and time-efficient solution, saving businesses valuable resources while still gaining access to reliable and detailed market information.
Decision-Making Support: Global research market reports serve as decision-making tools by providing data-driven insights and analysis. Businesses can rely on these reports to support their decision-making process, validate assumptions, and evaluate the potential outcomes of different strategies.
In general, market research studies offer companies and organisations useful data that can aid in making decisions and maintaining competitiveness in their industry. They can offer a strong basis for decision-making, strategy formulation, and company planning.
About US:
Stringent Datalytics offers both custom and syndicated market research reports. Custom market research reports are tailored to a specific client's needs and requirements. These reports provide unique insights into a particular industry or market segment and can help businesses make informed decisions about their strategies and operations.
Syndicated market research reports, on the other hand, are pre-existing reports that are available for purchase by multiple clients. These reports are often produced on a regular basis, such as annually or quarterly, and cover a broad range of industries and market segments. Syndicated reports provide clients with insights into industry trends, market sizes, and competitive landscapes. By offering both custom and syndicated reports, Stringent Datalytics can provide clients with a range of market research solutions that can be customized to their specific needs
Contact US:
Stringent Datalytics
Contact No - +1 346 666 6655
Email Id - [email protected]
Web - https://stringentdatalytics.com/
#Distribution Feeder Automation#Smart Grid Technology#Intelligent Sensors#IoT Integration#Edge Computing#Advanced Analytics#AI in Grid Management#Microgrid Integration#Fault Detection#Voltage Regulation#Load Balancing#Power Distribution Optimization#Grid Resilience#Outage Management#Renewable Energy Integration#Distribution System Efficiency#Energy Management Solutions.
0 notes
Text
A summary of the Chinese AI situation, for the uninitiated.
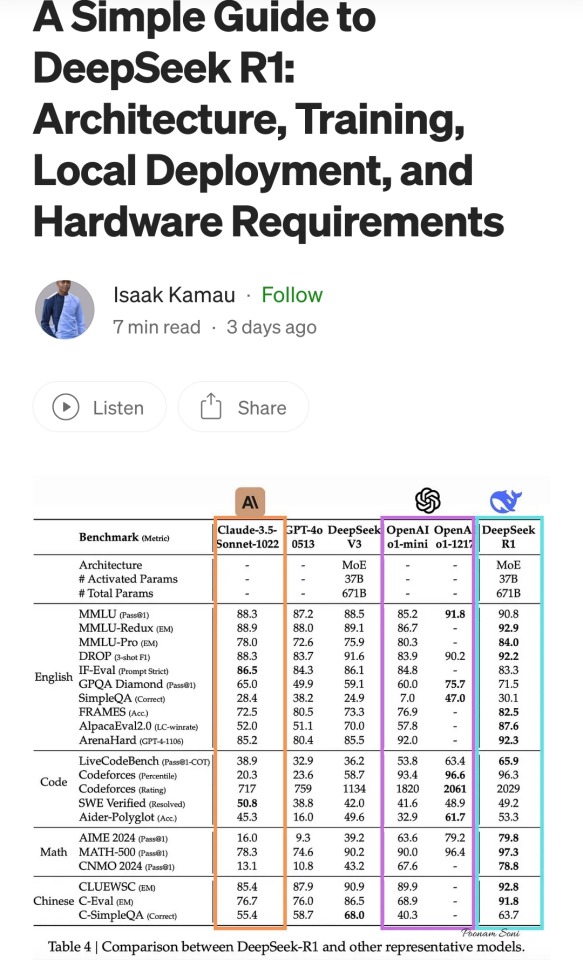
These are scores on different tests that are designed to see how accurate a Large Language Model is in different areas of knowledge. As you know, OpenAI is partners with Microsoft, so these are the scores for ChatGPT and Copilot. DeepSeek is the Chinese model that got released a week ago. The rest are open source models, which means everyone is free to use them as they please, including the average Tumblr user. You can run them from the servers of the companies that made them for a subscription, or you can download them to install locally on your own computer. However, the computer requirements so far are so high that only a few people currently have the machines at home required to run it.
Yes, this is why AI uses so much electricity. As with any technology, the early models are highly inefficient. Think how a Ford T needed a long chimney to get rid of a ton of black smoke, which was unused petrol. Over the next hundred years combustion engines have become much more efficient, but they still waste a lot of energy, which is why we need to move towards renewable electricity and sustainable battery technology. But that's a topic for another day.
As you can see from the scores, are around the same accuracy. These tests are in constant evolution as well: as soon as they start becoming obsolete, new ones are released to adjust for a more complicated benchmark. The new models are trained using different machine learning techniques, and in theory, the goal is to make them faster and more efficient so they can operate with less power, much like modern cars use way less energy and produce far less pollution than the Ford T.
However, computing power requirements kept scaling up, so you're either tied to the subscription or forced to pay for a latest gen PC, which is why NVIDIA, AMD, Intel and all the other chip companies were investing hard on much more powerful GPUs and NPUs. For now all we need to know about those is that they're expensive, use a lot of electricity, and are required to operate the bots at superhuman speed (literally, all those clickbait posts about how AI was secretly 150 Indian men in a trenchcoat were nonsense).
Because the chip companies have been working hard on making big, bulky, powerful chips with massive fans that are up to the task, their stock value was skyrocketing, and because of that, everyone started to use AI as a marketing trend. See, marketing people are not smart, and they don't understand computers. Furthermore, marketing people think you're stupid, and because of their biased frame of reference, they think you're two snores short of brain-dead. The entire point of their existence is to turn tall tales into capital. So they don't know or care about what AI is or what it's useful for. They just saw Number Go Up for the AI companies and decided "AI is a magic cow we can milk forever". Sometimes it's not even AI, they just use old software and rebrand it, much like convection ovens became air fryers.
Well, now we're up to date. So what did DepSeek release that did a 9/11 on NVIDIA stock prices and popped the AI bubble?
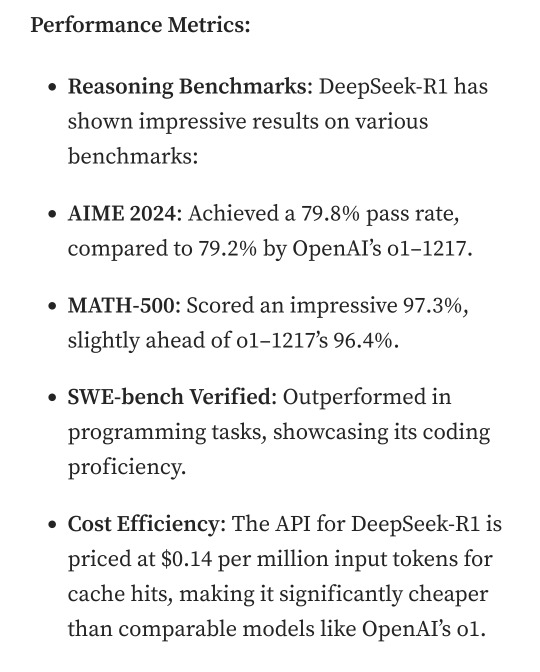
Oh, I would not want to be an OpenAI investor right now either. A token is basically one Unicode character (it's more complicated than that but you can google that on your own time). That cost means you could input the entire works of Stephen King for under a dollar. Yes, including electricity costs. DeepSeek has jumped from a Ford T to a Subaru in terms of pollution and water use.
The issue here is not only input cost, though; all that data needs to be available live, in the RAM; this is why you need powerful, expensive chips in order to-
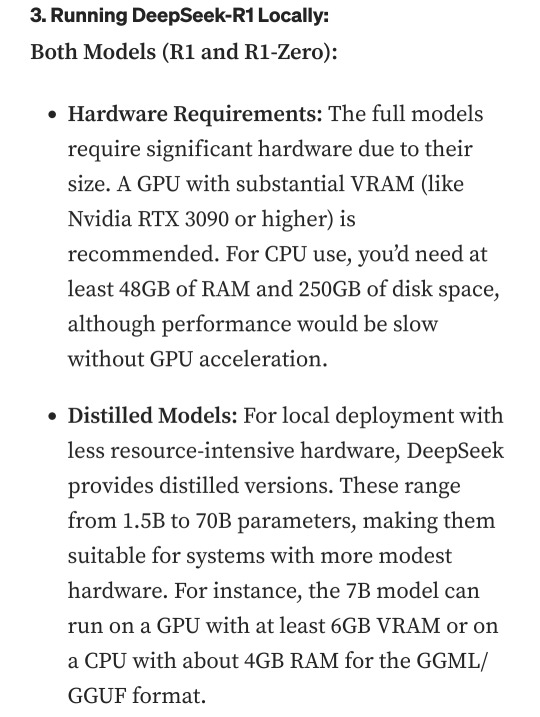
Holy shit.
I'm not going to detail all the numbers but I'm going to focus on the chip required: an RTX 3090. This is a gaming GPU that came out as the top of the line, the stuff South Korean LoL players buy…
Or they did, in September 2020. We're currently two generations ahead, on the RTX 5090.
What this is telling all those people who just sold their high-end gaming rig to be able to afford a machine that can run the latest ChatGPT locally, is that the person who bought it from them can run something basically just as powerful on their old one.
Which means that all those GPUs and NPUs that are being made, and all those deals Microsoft signed to have control of the AI market, have just lost a lot of their pulling power.
Well, I mean, the ChatGPT subscription is 20 bucks a month, surely the Chinese are charging a fortune for-
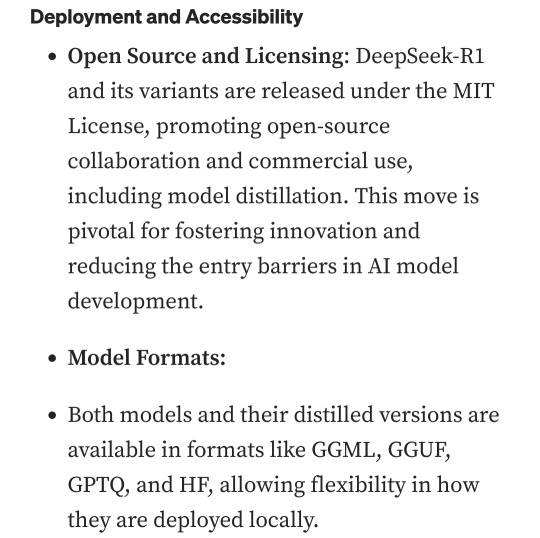
Oh. So it's free for everyone and you can use it or modify it however you want, no subscription, no unpayable electric bill, no handing Microsoft all of your private data, you can just run it on a relatively inexpensive PC. You could probably even run it on a phone in a couple years.
Oh, if only China had massive phone manufacturers that have a foot in the market everywhere except the US because the president had a tantrum eight years ago.
So… yeah, China just destabilised the global economy with a torrent file.
#valid ai criticism#ai#llms#DeepSeek#ai bubble#ChatGPT#google gemini#claude ai#this is gonna be the dotcom bubble again#hope you don't have stock on anything tech related#computer literacy#tech literacy
433 notes
·
View notes
Text
Photonic processor could enable ultrafast AI computations with extreme energy efficiency
New Post has been published on https://thedigitalinsider.com/photonic-processor-could-enable-ultrafast-ai-computations-with-extreme-energy-efficiency/
Photonic processor could enable ultrafast AI computations with extreme energy efficiency


The deep neural network models that power today’s most demanding machine-learning applications have grown so large and complex that they are pushing the limits of traditional electronic computing hardware.
Photonic hardware, which can perform machine-learning computations with light, offers a faster and more energy-efficient alternative. However, there are some types of neural network computations that a photonic device can’t perform, requiring the use of off-chip electronics or other techniques that hamper speed and efficiency.
Building on a decade of research, scientists from MIT and elsewhere have developed a new photonic chip that overcomes these roadblocks. They demonstrated a fully integrated photonic processor that can perform all the key computations of a deep neural network optically on the chip.
The optical device was able to complete the key computations for a machine-learning classification task in less than half a nanosecond while achieving more than 92 percent accuracy — performance that is on par with traditional hardware.
The chip, composed of interconnected modules that form an optical neural network, is fabricated using commercial foundry processes, which could enable the scaling of the technology and its integration into electronics.
In the long run, the photonic processor could lead to faster and more energy-efficient deep learning for computationally demanding applications like lidar, scientific research in astronomy and particle physics, or high-speed telecommunications.
“There are a lot of cases where how well the model performs isn’t the only thing that matters, but also how fast you can get an answer. Now that we have an end-to-end system that can run a neural network in optics, at a nanosecond time scale, we can start thinking at a higher level about applications and algorithms,” says Saumil Bandyopadhyay ’17, MEng ’18, PhD ’23, a visiting scientist in the Quantum Photonics and AI Group within the Research Laboratory of Electronics (RLE) and a postdoc at NTT Research, Inc., who is the lead author of a paper on the new chip.
Bandyopadhyay is joined on the paper by Alexander Sludds ’18, MEng ’19, PhD ’23; Nicholas Harris PhD ’17; Darius Bunandar PhD ’19; Stefan Krastanov, a former RLE research scientist who is now an assistant professor at the University of Massachusetts at Amherst; Ryan Hamerly, a visiting scientist at RLE and senior scientist at NTT Research; Matthew Streshinsky, a former silicon photonics lead at Nokia who is now co-founder and CEO of Enosemi; Michael Hochberg, president of Periplous, LLC; and Dirk Englund, a professor in the Department of Electrical Engineering and Computer Science, principal investigator of the Quantum Photonics and Artificial Intelligence Group and of RLE, and senior author of the paper. The research appears today in Nature Photonics.
Machine learning with light
Deep neural networks are composed of many interconnected layers of nodes, or neurons, that operate on input data to produce an output. One key operation in a deep neural network involves the use of linear algebra to perform matrix multiplication, which transforms data as it is passed from layer to layer.
But in addition to these linear operations, deep neural networks perform nonlinear operations that help the model learn more intricate patterns. Nonlinear operations, like activation functions, give deep neural networks the power to solve complex problems.
In 2017, Englund’s group, along with researchers in the lab of Marin Soljačić, the Cecil and Ida Green Professor of Physics, demonstrated an optical neural network on a single photonic chip that could perform matrix multiplication with light.
But at the time, the device couldn’t perform nonlinear operations on the chip. Optical data had to be converted into electrical signals and sent to a digital processor to perform nonlinear operations.
“Nonlinearity in optics is quite challenging because photons don’t interact with each other very easily. That makes it very power consuming to trigger optical nonlinearities, so it becomes challenging to build a system that can do it in a scalable way,” Bandyopadhyay explains.
They overcame that challenge by designing devices called nonlinear optical function units (NOFUs), which combine electronics and optics to implement nonlinear operations on the chip.
The researchers built an optical deep neural network on a photonic chip using three layers of devices that perform linear and nonlinear operations.
A fully-integrated network
At the outset, their system encodes the parameters of a deep neural network into light. Then, an array of programmable beamsplitters, which was demonstrated in the 2017 paper, performs matrix multiplication on those inputs.
The data then pass to programmable NOFUs, which implement nonlinear functions by siphoning off a small amount of light to photodiodes that convert optical signals to electric current. This process, which eliminates the need for an external amplifier, consumes very little energy.
“We stay in the optical domain the whole time, until the end when we want to read out the answer. This enables us to achieve ultra-low latency,” Bandyopadhyay says.
Achieving such low latency enabled them to efficiently train a deep neural network on the chip, a process known as in situ training that typically consumes a huge amount of energy in digital hardware.
“This is especially useful for systems where you are doing in-domain processing of optical signals, like navigation or telecommunications, but also in systems that you want to learn in real time,” he says.
The photonic system achieved more than 96 percent accuracy during training tests and more than 92 percent accuracy during inference, which is comparable to traditional hardware. In addition, the chip performs key computations in less than half a nanosecond.
“This work demonstrates that computing — at its essence, the mapping of inputs to outputs — can be compiled onto new architectures of linear and nonlinear physics that enable a fundamentally different scaling law of computation versus effort needed,” says Englund.
The entire circuit was fabricated using the same infrastructure and foundry processes that produce CMOS computer chips. This could enable the chip to be manufactured at scale, using tried-and-true techniques that introduce very little error into the fabrication process.
Scaling up their device and integrating it with real-world electronics like cameras or telecommunications systems will be a major focus of future work, Bandyopadhyay says. In addition, the researchers want to explore algorithms that can leverage the advantages of optics to train systems faster and with better energy efficiency.
This research was funded, in part, by the U.S. National Science Foundation, the U.S. Air Force Office of Scientific Research, and NTT Research.
#ai#air#air force#Algorithms#applications#artificial#Artificial Intelligence#Astronomy#author#Building#Cameras#CEO#challenge#chip#chips#computation#computer#computer chips#Computer Science#Computer science and technology#computing#computing hardware#data#Deep Learning#devices#efficiency#Electrical engineering and computer science (EECS)#electronic#Electronics#energy
0 notes
Text
OPTİVİSER - GOLD

Welcome to Optiviser.com, your ultimate guide to navigating the complex world of electronics in 2024. As technology continues to evolve at a rapid pace, finding the right devices that suit your needs can be overwhelming. In this blog post, we’ll harness the power of AI to help you make informed choices with our comprehensive electronics comparison. We’ll take a closer look at the top smart home devices that are revolutionizing how we live and work, providing convenience and efficiency like never before. Additionally, we’ll offer expert laptop recommendations tailored to various lifestyles and budgets, ensuring you find the perfect match for your daily tasks.
AI-powered Electronics Comparison
In today's fast-paced technological landscape, making informed choices about electronics can be overwhelming. An AI-powered Electronics Comparison tool can help streamline this process by providing insights that cater to specific user needs. These advanced tools utilize algorithms that analyze product features, specifications, and user reviews, resulting in a tailored recommendation for buyers.
As we delve into the world of consumer technology, it's important to highlight the Top Smart Home Devices 2024. From smart thermostats to security cameras, these devices are becoming essential for modern households. They not only enhance convenience but also significantly improve energy efficiency and home safety.
For those looking for a new computer to enhance productivity or gaming experiences, consider checking out the latest Laptop Recommendations. Many platforms, including Optiviser.com, provide comprehensive comparisons and insights that can help consumers choose the best laptop suited to their needs, whether it’s for work, study, or leisure.
Top Smart Home Devices 2024
As we move into 2024, the landscape of home automation is evolving rapidly, showcasing an array of innovative gadgets designed to enhance comfort and convenience. In this era of AI-powered Electronics Comparison, selecting the right devices can be overwhelming, but we've highlighted some of the best Top Smart Home Devices 2024 that stand out for their functionality and user experience.
One of the most impressive innovations for this year is the latest AI-powered home assistant. These devices not only respond to voice commands but also learn your preferences over time, allowing them to offer personalized suggestions and perform tasks proactively. Imagine a device that can monitor your schedule and automatically adjust your home's temperature and lighting accordingly!
Moreover, security remains a top priority in smart homes. The Top Smart Home Devices 2024 include state-of-the-art security cameras and smart locks that provide robust protection while ensuring ease of access. With features like remote monitoring through your smartphone or integration with smart doorbells, keeping your home safe has never been easier. For more details on the comparisons and recommendations of these devices, you can check out Optiviser.com.
Laptop Recommendation
In today's fast-paced world, choosing the right laptop can be a daunting task. With numerous options available in the market, it's essential to consider various factors such as performance, portability, and price. At Optiviser.com, we provide an insightful guide to help you navigate through the vast array of choices. To streamline your decision-making process, we have developed an AI-powered Electronics Comparison tool that allows you to compare specifications and features of different laptops side by side.
This year, we have seen a surge in innovative laptops that cater to diverse needs. Whether for gaming, business, or everyday use, our top recommendations include models that excel in battery life, processing power, and display quality. For instance, consider the latest models from top brands, which have integrated the best features of Top Smart Home Devices 2024 trends, ensuring seamless connectivity and advanced functionalities.
Additionally, if you're looking for a laptop that can handle multitasking effortlessly, we suggest models equipped with the latest processors and ample RAM. Our detailed Laptop Recommendation section on Optiviser.com includes expert reviews and user feedback to help you choose a laptop that not only fits your budget but also meets your specific requirements.
674 notes
·
View notes
Text
Upgrading Your Gaming Rig with the Latest Computer Parts
In the rapidly evolving world of PC gaming, staying ahead of the curve is essential for an immersive and competitive experience. Upgrading your gaming rig with the latest computer parts not only boosts your system’s performance but also ensures that you’re getting the most out of your gaming sessions. This comprehensive guide will walk you through the key components to consider when upgrading…
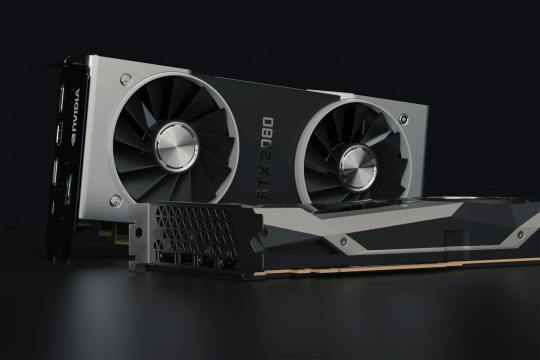
View On WordPress
#cooling systems#CPU upgrades#cutting-edge gaming technology#DIY PC upgrades#energy-efficient PSUs#future-proofing components#gaming experience#gaming rig upgrades#GPU enhancements#high-resolution gaming#immersive gaming setup#latest computer parts#motherboard compatibility#NVMe SSD#PC gaming advancements#PC performance boost#power supply units#professional PC building#quality computer components#RAM optimization#ray tracing#SSD technology#system compatibility#thermal management
1 note
·
View note
Text
Calling a technology "bad for the environment" is a politically vacuous framework. It makes invisible the ways classes of people intentionally develop tech in a resource-intensive way. It erases the fact that tech is controlled by PEOPLE who should be held accountable. Saying that anything is "bad for the environment" isn't useful framing. Throwing a napkin in the street is bad for the environment but negligible compared to driving a car or eating meat. Yet, the negligible act feels worse. The better framing is: is the resource cost worth the benefit provided?
I think the resources required to develop genAI and machine learning are worth the cost. BUT there's no reason for dozens of companies to build huge proprietary models or for aggressive data center expansion. AI CAN be developed sustainably. But a for-profit system doesn't incentivize this.
Some might argue that any use of AI is a waste of resources. This is disingenuous and ignores the active applications of AI in education, medicine, and admin. And even if it were frivolous, it's okay for societies to produce frivolous things as long as it's produced sustainably. Communist societies will still produce soda, stylish hats, and video games.
Even if you think AI is frivolous, it CAN be produced sustainably, but we live in a for profit system where big tech companies are using a ton of unnecessary resources in ways that hurt the public. But we know tech (not just AI) can be sustainable & energy efficient. Computing is one of the most energy efficient things humans do compared to how much we rely on it, and is only increasing in efficiency.
If you're critical of AI, framing it as uniquely bad for the environment isn't useful and isn't true. At the individual level, it's less wasteful than eating meat and slightly less energy intensive than watching Netflix. Some models can be run locally on your computer. Training can be energy intensive but is infrequent, and only needs to be done once per model.
Also, there are real harms in overstating AI energy use! Energy and utility companies are using overinflated AI energy numbers to justify a huge buildout of oil and gas.
If you're critical of AI, you should instead ask: What are we getting for the resources used? What outcome are we trying to get out of AI & tech development? Are all these resources necessary for the outcomes we seek? AI CAN be environmentally sustainable, but there are systems and people who want to foreclose this possibility. Headlines like "ChatGPT uses 10x more energy than Google search" (not even true), shift blame from institutional actors onto individuals, from profit-motives onto technology.
71 notes
·
View notes
Text
An innovative algorithm for detecting collisions of high-speed particles within nuclear fusion reactors has been developed, inspired by technologies used to determine whether bullets hit targets in video games. This advancement enables rapid predictions of collisions, significantly enhancing the stability and design efficiency of future fusion reactors. Professor Eisung Yoon and his research team in the Department of Nuclear Engineering at UNIST announced that they have successfully developed a collision detection algorithm capable of quickly identifying collision points of high-speed particles within virtual fusion devices. The research is published in the journal Computer Physics Communications.
Continue Reading.
67 notes
·
View notes
Text
Some old art of my Owlk engineer, Eris, designing the Stranger's solar sails! Enjoy a big ramble about him and his job because I love this silly man:
Eris works on the design team for the Owlk space program, specialising in energy and propulsion technologies for the ships, satellites, and probes. Having a design philosophy of functionality and beauty, Eris enjoys going all-out with his work. He has received special recognition for his solar panel designs in particular, which borrowed from the unparalleled efficiency found in photosynthesizing plants.
When designing the Stranger's solar sails, Eris took inspiration from plants, but also the opening of insect elytra; the ballooning behaviours of silk-producing invertebrates, in which they sail from tree to tree using electric fields and air currents; and how flying creatures will use thermal updrafts to soar higher while expending less energy. Already familiar with how solar energy impacts technology from his work on solar panels, he proposed the use of this energy to propel the Stranger through space.
As travelling the distance between stars presented the major roadblock in the plan to reach the Eye (regarded as the Interstellar Propulsion Problem), Eris was lauded for his contributions, promoted to being one of the main engineers overseeing the Stranger's design.
More information about his general design process below!
When designing for a project, Eris uses all of the tools at his disposal. His first weapon of choice is always his pencil, and he will sketch out potential sources of inspiration on paper until the design concept begins to take form. Based on the initial project parameters he's been given, he drafts up a blueprint for his components.
Next, he must further conceptualise his designs. This is where the most valuable tool of the trade comes into play—the Vision Torch! Vision Torches serve many purposes for Owlks, from allowing them to nonverbally communicate to creating photographs from memory alone. Owlk engineers LOVE Vision Torches for how easy they make effectively communicating ideas. They allow concepts to be visualised in 3D, basic functionality to be shown through animations, and are even able to interface with computers. Eris might even 3D print a model using a Vision Torch to help him visualise his concepts as he works.
The space program is extremely collaborative, and Eris works on just a small part of the overall project, so being able to easily share ideas with others and see how all the individual components of a satellite or ship interact is vital. When discussing with more than a single other Owlk, Eris can use a Vision Torch linked to a holographic display to present concepts to a crowd. Concepts can also be tweaked in real time this way!

[Here's an example from the game of Owlks building the simulation with Vision Torches and a holographic display!]
With a Vision Torch, concepts can also be directly uploaded to a computer terminal. This is where a lot of the real work gets done - calculating weight, materials needed, stress testing in simulations, calculating trajectories, making precise tweaks to finalize the design, you name it. This also allows other Owlks working closely with Eris to access the most current design for their own tests.
This is an iterative process - as other Owlks finalize their components, as weight limitations are further restrained and material needs are calculated, Eris often has to go back to an earlier step and rework his concept. Fortunately, he thoroughly enjoys getting to be creative in his work (and doing math) and treats every project as a puzzle that needs to be solved! The only time when he's not excited to go back to the drawing board is when a last-minute adjustment from his peers means he needs to work long hours to get his work done in time for launch.
#outer wilds#outer wilds spoilers#echoes of the eye#echoes of the eye spoilers#outer wilds oc#my art#eris#my workaholic son#someone needs to tell him to take a break#please
68 notes
·
View notes