#AI-driven training
Explore tagged Tumblr posts
Text
🚀 The future of workforce training is AI-driven. Traditional education is too slow to keep up with evolving industry demands, but AI-powered training is changing the game—offering adaptive learning, real-world simulations, and personalized upskilling at scale. Will New Zealand lead the charge or fall behind? Let’s discuss! 👇 #AI #FutureOfWork #VocationalTraining
View On WordPress
#adaptive learning#AI for skills training#AI in career development#AI in education#AI in job training#AI in vocational training#AI in workforce training#AI simulations#AI upskilling#AI-driven training#AI-powered learning#Edtech#Future of work#vocational education#workforce development
0 notes
Text
The surprising truth about data-driven dictatorships
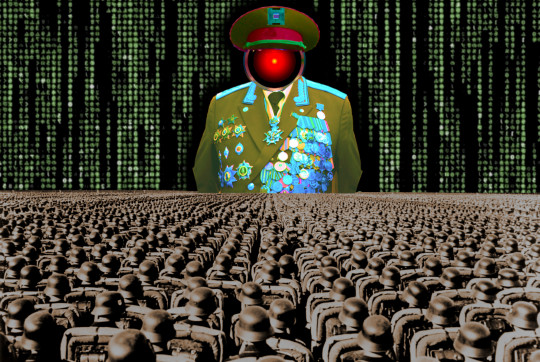
Here’s the “dictator’s dilemma”: they want to block their country’s frustrated elites from mobilizing against them, so they censor public communications; but they also want to know what their people truly believe, so they can head off simmering resentments before they boil over into regime-toppling revolutions.
These two strategies are in tension: the more you censor, the less you know about the true feelings of your citizens and the easier it will be to miss serious problems until they spill over into the streets (think: the fall of the Berlin Wall or Tunisia before the Arab Spring). Dictators try to square this circle with things like private opinion polling or petition systems, but these capture a small slice of the potentially destabiziling moods circulating in the body politic.
Enter AI: back in 2018, Yuval Harari proposed that AI would supercharge dictatorships by mining and summarizing the public mood — as captured on social media — allowing dictators to tack into serious discontent and diffuse it before it erupted into unequenchable wildfire:
https://www.theatlantic.com/magazine/archive/2018/10/yuval-noah-harari-technology-tyranny/568330/
Harari wrote that “the desire to concentrate all information and power in one place may become [dictators] decisive advantage in the 21st century.” But other political scientists sharply disagreed. Last year, Henry Farrell, Jeremy Wallace and Abraham Newman published a thoroughgoing rebuttal to Harari in Foreign Affairs:
https://www.foreignaffairs.com/world/spirals-delusion-artificial-intelligence-decision-making
They argued that — like everyone who gets excited about AI, only to have their hopes dashed — dictators seeking to use AI to understand the public mood would run into serious training data bias problems. After all, people living under dictatorships know that spouting off about their discontent and desire for change is a risky business, so they will self-censor on social media. That’s true even if a person isn’t afraid of retaliation: if you know that using certain words or phrases in a post will get it autoblocked by a censorbot, what’s the point of trying to use those words?
The phrase “Garbage In, Garbage Out” dates back to 1957. That’s how long we’ve known that a computer that operates on bad data will barf up bad conclusions. But this is a very inconvenient truth for AI weirdos: having given up on manually assembling training data based on careful human judgment with multiple review steps, the AI industry “pivoted” to mass ingestion of scraped data from the whole internet.
But adding more unreliable data to an unreliable dataset doesn’t improve its reliability. GIGO is the iron law of computing, and you can’t repeal it by shoveling more garbage into the top of the training funnel:
https://memex.craphound.com/2018/05/29/garbage-in-garbage-out-machine-learning-has-not-repealed-the-iron-law-of-computer-science/
When it comes to “AI” that’s used for decision support — that is, when an algorithm tells humans what to do and they do it — then you get something worse than Garbage In, Garbage Out — you get Garbage In, Garbage Out, Garbage Back In Again. That’s when the AI spits out something wrong, and then another AI sucks up that wrong conclusion and uses it to generate more conclusions.
To see this in action, consider the deeply flawed predictive policing systems that cities around the world rely on. These systems suck up crime data from the cops, then predict where crime is going to be, and send cops to those “hotspots” to do things like throw Black kids up against a wall and make them turn out their pockets, or pull over drivers and search their cars after pretending to have smelled cannabis.
The problem here is that “crime the police detected” isn’t the same as “crime.” You only find crime where you look for it. For example, there are far more incidents of domestic abuse reported in apartment buildings than in fully detached homes. That’s not because apartment dwellers are more likely to be wife-beaters: it’s because domestic abuse is most often reported by a neighbor who hears it through the walls.
So if your cops practice racially biased policing (I know, this is hard to imagine, but stay with me /s), then the crime they detect will already be a function of bias. If you only ever throw Black kids up against a wall and turn out their pockets, then every knife and dime-bag you find in someone’s pockets will come from some Black kid the cops decided to harass.
That’s life without AI. But now let’s throw in predictive policing: feed your “knives found in pockets” data to an algorithm and ask it to predict where there are more knives in pockets, and it will send you back to that Black neighborhood and tell you do throw even more Black kids up against a wall and search their pockets. The more you do this, the more knives you’ll find, and the more you’ll go back and do it again.
This is what Patrick Ball from the Human Rights Data Analysis Group calls “empiricism washing”: take a biased procedure and feed it to an algorithm, and then you get to go and do more biased procedures, and whenever anyone accuses you of bias, you can insist that you’re just following an empirical conclusion of a neutral algorithm, because “math can’t be racist.”
HRDAG has done excellent work on this, finding a natural experiment that makes the problem of GIGOGBI crystal clear. The National Survey On Drug Use and Health produces the gold standard snapshot of drug use in America. Kristian Lum and William Isaac took Oakland’s drug arrest data from 2010 and asked Predpol, a leading predictive policing product, to predict where Oakland’s 2011 drug use would take place.
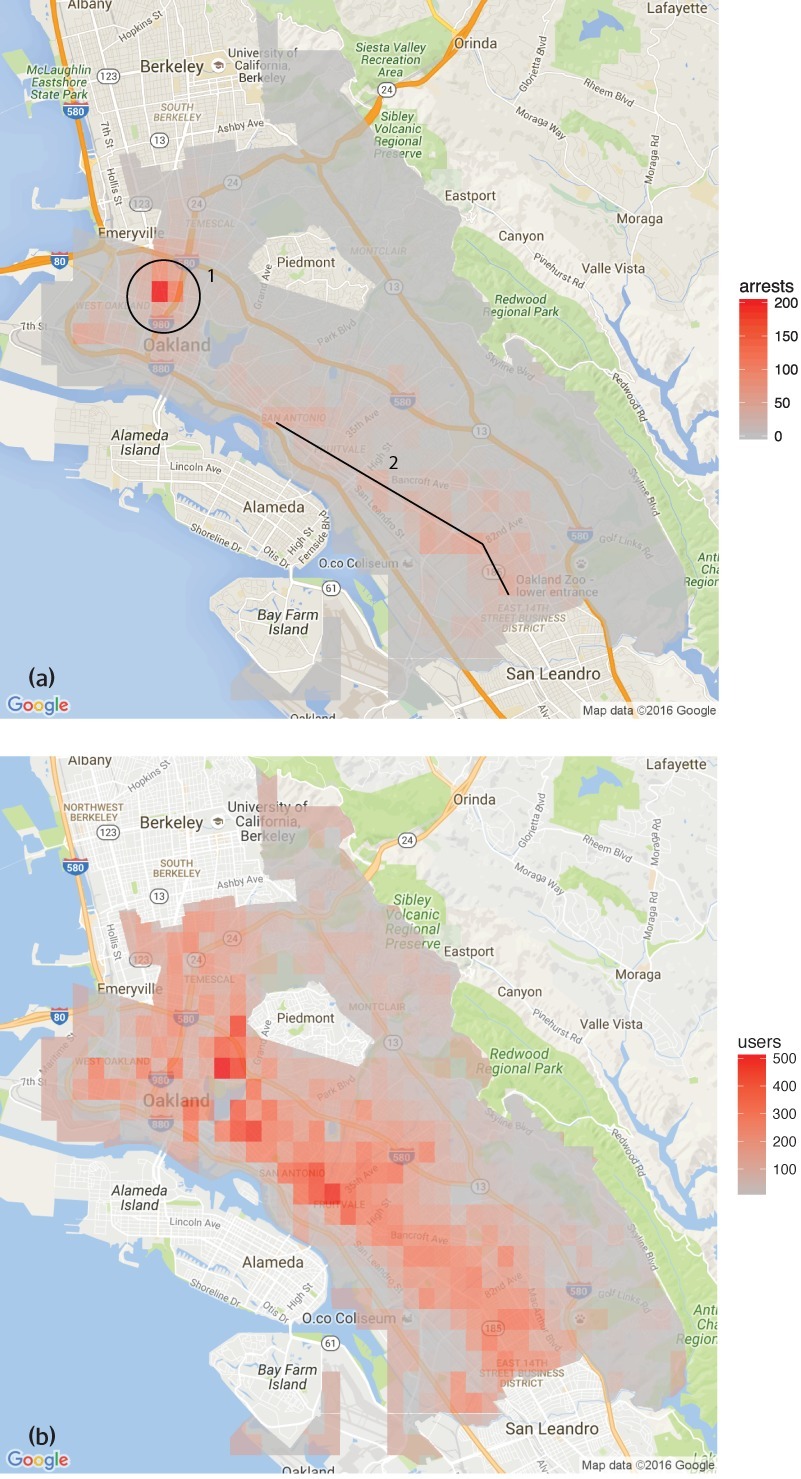
[Image ID: (a) Number of drug arrests made by Oakland police department, 2010. (1) West Oakland, (2) International Boulevard. (b) Estimated number of drug users, based on 2011 National Survey on Drug Use and Health]
Then, they compared those predictions to the outcomes of the 2011 survey, which shows where actual drug use took place. The two maps couldn’t be more different:
https://rss.onlinelibrary.wiley.com/doi/full/10.1111/j.1740-9713.2016.00960.x
Predpol told cops to go and look for drug use in a predominantly Black, working class neighborhood. Meanwhile the NSDUH survey showed the actual drug use took place all over Oakland, with a higher concentration in the Berkeley-neighboring student neighborhood.
What’s even more vivid is what happens when you simulate running Predpol on the new arrest data that would be generated by cops following its recommendations. If the cops went to that Black neighborhood and found more drugs there and told Predpol about it, the recommendation gets stronger and more confident.
In other words, GIGOGBI is a system for concentrating bias. Even trace amounts of bias in the original training data get refined and magnified when they are output though a decision support system that directs humans to go an act on that output. Algorithms are to bias what centrifuges are to radioactive ore: a way to turn minute amounts of bias into pluripotent, indestructible toxic waste.
There’s a great name for an AI that’s trained on an AI’s output, courtesy of Jathan Sadowski: “Habsburg AI.”
And that brings me back to the Dictator’s Dilemma. If your citizens are self-censoring in order to avoid retaliation or algorithmic shadowbanning, then the AI you train on their posts in order to find out what they’re really thinking will steer you in the opposite direction, so you make bad policies that make people angrier and destabilize things more.
Or at least, that was Farrell(et al)’s theory. And for many years, that’s where the debate over AI and dictatorship has stalled: theory vs theory. But now, there’s some empirical data on this, thanks to the “The Digital Dictator’s Dilemma,” a new paper from UCSD PhD candidate Eddie Yang:
https://www.eddieyang.net/research/DDD.pdf
Yang figured out a way to test these dueling hypotheses. He got 10 million Chinese social media posts from the start of the pandemic, before companies like Weibo were required to censor certain pandemic-related posts as politically sensitive. Yang treats these posts as a robust snapshot of public opinion: because there was no censorship of pandemic-related chatter, Chinese users were free to post anything they wanted without having to self-censor for fear of retaliation or deletion.
Next, Yang acquired the censorship model used by a real Chinese social media company to decide which posts should be blocked. Using this, he was able to determine which of the posts in the original set would be censored today in China.
That means that Yang knows that the “real” sentiment in the Chinese social media snapshot is, and what Chinese authorities would believe it to be if Chinese users were self-censoring all the posts that would be flagged by censorware today.
From here, Yang was able to play with the knobs, and determine how “preference-falsification” (when users lie about their feelings) and self-censorship would give a dictatorship a misleading view of public sentiment. What he finds is that the more repressive a regime is — the more people are incentivized to falsify or censor their views — the worse the system gets at uncovering the true public mood.
What’s more, adding additional (bad) data to the system doesn’t fix this “missing data” problem. GIGO remains an iron law of computing in this context, too.
But it gets better (or worse, I guess): Yang models a “crisis” scenario in which users stop self-censoring and start articulating their true views (because they’ve run out of fucks to give). This is the most dangerous moment for a dictator, and depending on the dictatorship handles it, they either get another decade or rule, or they wake up with guillotines on their lawns.
But “crisis” is where AI performs the worst. Trained on the “status quo” data where users are continuously self-censoring and preference-falsifying, AI has no clue how to handle the unvarnished truth. Both its recommendations about what to censor and its summaries of public sentiment are the least accurate when crisis erupts.
But here’s an interesting wrinkle: Yang scraped a bunch of Chinese users’ posts from Twitter — which the Chinese government doesn’t get to censor (yet) or spy on (yet) — and fed them to the model. He hypothesized that when Chinese users post to American social media, they don’t self-censor or preference-falsify, so this data should help the model improve its accuracy.
He was right — the model got significantly better once it ingested data from Twitter than when it was working solely from Weibo posts. And Yang notes that dictatorships all over the world are widely understood to be scraping western/northern social media.
But even though Twitter data improved the model’s accuracy, it was still wildly inaccurate, compared to the same model trained on a full set of un-self-censored, un-falsified data. GIGO is not an option, it’s the law (of computing).
Writing about the study on Crooked Timber, Farrell notes that as the world fills up with “garbage and noise” (he invokes Philip K Dick’s delighted coinage “gubbish”), “approximately correct knowledge becomes the scarce and valuable resource.”
https://crookedtimber.org/2023/07/25/51610/
This “probably approximately correct knowledge” comes from humans, not LLMs or AI, and so “the social applications of machine learning in non-authoritarian societies are just as parasitic on these forms of human knowledge production as authoritarian governments.”
The Clarion Science Fiction and Fantasy Writers’ Workshop summer fundraiser is almost over! I am an alum, instructor and volunteer board member for this nonprofit workshop whose alums include Octavia Butler, Kim Stanley Robinson, Bruce Sterling, Nalo Hopkinson, Kameron Hurley, Nnedi Okorafor, Lucius Shepard, and Ted Chiang! Your donations will help us subsidize tuition for students, making Clarion — and sf/f — more accessible for all kinds of writers.
Libro.fm is the indie-bookstore-friendly, DRM-free audiobook alternative to Audible, the Amazon-owned monopolist that locks every book you buy to Amazon forever. When you buy a book on Libro, they share some of the purchase price with a local indie bookstore of your choosing (Libro is the best partner I have in selling my own DRM-free audiobooks!). As of today, Libro is even better, because it’s available in five new territories and currencies: Canada, the UK, the EU, Australia and New Zealand!
[Image ID: An altered image of the Nuremberg rally, with ranked lines of soldiers facing a towering figure in a many-ribboned soldier's coat. He wears a high-peaked cap with a microchip in place of insignia. His head has been replaced with the menacing red eye of HAL9000 from Stanley Kubrick's '2001: A Space Odyssey.' The sky behind him is filled with a 'code waterfall' from 'The Matrix.']
Image: Cryteria (modified) https://commons.wikimedia.org/wiki/File:HAL9000.svg
CC BY 3.0 https://creativecommons.org/licenses/by/3.0/deed.en
 — 
Raimond Spekking (modified) https://commons.wikimedia.org/wiki/File:Acer_Extensa_5220_-_Columbia_MB_06236-1N_-_Intel_Celeron_M_530_-_SLA2G_-_in_Socket_479-5029.jpg
CC BY-SA 4.0 https://creativecommons.org/licenses/by-sa/4.0/deed.en
 — 
Russian Airborne Troops (modified) https://commons.wikimedia.org/wiki/File:Vladislav_Achalov_at_the_Airborne_Troops_Day_in_Moscow_%E2%80%93_August_2,_2008.jpg
“Soldiers of Russia” Cultural Center (modified) https://commons.wikimedia.org/wiki/File:Col._Leonid_Khabarov_in_an_everyday_service_uniform.JPG
CC BY-SA 3.0 https://creativecommons.org/licenses/by-sa/3.0/deed.en
#pluralistic#habsburg ai#self censorship#henry farrell#digital dictatorships#machine learning#dictator's dilemma#eddie yang#preference falsification#political science#training bias#scholarship#spirals of delusion#algorithmic bias#ml#Fully automated data driven authoritarianism#authoritarianism#gigo#garbage in garbage out garbage back in#gigogbi#yuval noah harari#gubbish#pkd#philip k dick#phildickian
832 notes
·
View notes
Text
#Faster Onboarding with AI#Employee Training on Complex Software#AI-driven training and onboarding#apty.ai#digital adoption platform#best digital adoption platforms
0 notes
Text
Hey AI, Are You Telling Google About Me?
A Personal Dive Into the Algorithmic Mirror It started with a simple, almost paranoid question—but one that feels increasingly valid in the age of digital surveillance: “Do you report cookies to Google or is it just my phone/browser doing so? Because often times I’ll talk to you about something and a short while later I’ll see an ad or video for it.” That question wasn’t rhetorical—it came…
#AI and you#AI Conversations#Algoritm Culture#content curation#Data Driven World#did i train the algorithm#digital identity#Digital self awarteness#digital surveillance#echo chamber#feed manipulation#Lexi and Me#media bubble#modern mind control#Platform Politics#tech vs privacy#watching back#youtube feed
0 notes
Text
The Role of Cloud Computing in Digital Transformation for SMBs
One key driver of digital transformation for SMBs is cloud computing. By leveraging cloud technologies, SMBs can enhance their operational efficiency, scalability, and flexibility, transform their business processes, and achieve new heights of success.Â
Understanding Cloud Computing
Cloud computing refers to the delivery of computing services, such as storage, processing power, and applications, over the Internet. Instead of relying on on-premises servers and infrastructure, businesses can access these services on a pay-as-you-go basis from cloud service providers like Amazon Web Services (AWS), Microsoft Azure, and Google Cloud Platform.Â
Types of Cloud ComputingÂ
Cloud computing can be categorized into three main types, each offering different levels of control, flexibility, and management:Â
1. Public CloudÂ
Public cloud services are operated by third-party providers and made available to multiple users over the Internet. Examples include Amazon Web Services (AWS), Microsoft Azure, and Google Cloud Platform. This model offers cost-effectiveness and scalability but may have security and compliance concerns for sensitive data.Â
2. Private CloudÂ
A private cloud is dedicated to a single organization, offering greater control and security. It can be hosted on-premises or by a third-party provider. This option is ideal for SMBs that require stringent security and regulatory compliance.Â
3. Hybrid CloudÂ
A hybrid cloud combines elements of both public and private clouds, allowing SMBs to balance scalability and security. Businesses can keep critical workloads on private clouds while leveraging the public cloud for less sensitive operations.Â
“Cloud services provide SMBs with the ability to rapidly adopt new IT solutions and deploy additional storage capacity as needed, making it easier to keep up with digital transformation.”
— Data Centre Review
Cloud Solution Service Models for SMBs
Cloud computing services are typically divided into three key models:Â
1. Infrastructure as a Service (IaaS)Â
IaaS provides virtualized computing resources over the internet, including storage, networking, and virtual machines. It allows SMBs to avoid investing in expensive hardware and instead rent infrastructure on a flexible basis. Examples include AWS EC2 and Google Compute Engine.Â
2. Platform as a Service (PaaS)Â
PaaS offers a development and deployment environment in the cloud, including operating systems, databases, and application development tools. This model enables SMBs to build and deploy applications without worrying about infrastructure management. Examples include Microsoft Azure App Services and Google App Engine.Â
3. Software as a Service (SaaS)Â
SaaS provides ready-to-use applications over the Internet, eliminating the need for installation and maintenance. Common examples include Google Workspace, Microsoft 365, and Salesforce. This model is cost-effective and easily accessible for SMBs looking to streamline operations.Â
Driving Digital TransformationÂ
By adopting cloud computing, SMBs can drive their digital transformation efforts and gain a competitive edge. Cloud technologies enable businesses to innovate faster, respond to market changes, and deliver enhanced customer experiences. Â
Here are some ways SMBs can leverage cloud computing for digital transformation:Â
Data Analytics: Cloud-based analytics tools allow businesses to gather, process, and analyze large volumes of data. SMBs can gain valuable insights into customer behaviour, market trends, and operational performance, informing data-driven decision-making.Â
Collaboration and Communication: Cloud-based collaboration tools, such as Microsoft Teams and Slack, facilitate seamless communication and teamwork. These platforms support real-time collaboration, file sharing, and project management, improving overall productivity.Â
Customer Engagement: Cloud-based customer engagement platforms, such as CRM systems, help SMBs manage customer interactions, track sales leads, and personalize marketing efforts. This leads to improved customer satisfaction and loyalty.Â
E-Commerce: Cloud hosting solutions provide the infrastructure needed to run e-commerce websites and applications. SMBs can scale their online presence, manage inventory, and process transactions efficiently, driving growth in the digital marketplace.Â
Key Benefits of Cloud Computing for SMBsÂ
1. Cost SavingsÂ
SMBs often operate with limited budgets, making large capital expenditures on IT infrastructure impractical. Cloud computing eliminates the need for expensive hardware and maintenance, reducing upfront costs and shifting expenses to a predictable subscription model.Â
2. Scalability and FlexibilityÂ
With cloud-based solutions, SMBs can scale resources up or down based on demand. This flexibility allows businesses to respond to market changes, expand operations, and deploy new applications without significant investments in physical infrastructure.Â
3. Enhanced CollaborationÂ
Cloud-based tools such as Google Workspace, Microsoft 365, and Slack enable teams to collaborate in real time, regardless of location. This is especially valuable in today’s remote and hybrid work environments, improving productivity and communication.Â
4. Improved Security and ComplianceÂ
Many SMBs lack the resources to implement robust cybersecurity measures. Cloud providers invest heavily in security protocols, offering features such as encryption, multi-factor authentication, and regular security updates. Additionally, compliance with industry regulations is easier with built-in security frameworks provided by cloud vendors.Â
5. Business Continuity and Disaster RecoveryÂ
Data loss can be catastrophic for any business. Cloud computing offers automatic backups, disaster recovery options, and redundancy, ensuring that critical business data remains secure and accessible even in the face of cyberattacks or natural disasters.Â
6. Access to Advanced TechnologiesÂ
Cloud platforms enable SMBs to leverage cutting-edge technologies like artificial intelligence (AI), machine learning, and big data analytics. These tools help businesses gain insights, automate processes, and enhance customer engagement.Â
Implementation Strategies for SMBsÂ
Successfully adopting cloud computing requires a strategic approach. Here are key steps SMBs can take:Â
1. Assess Business NeedsÂ
Identify the specific pain points cloud computing can address, such as improving collaboration, reducing IT costs, or enhancing data security.Â
2. Choose the Right Cloud ModelÂ
Based on security, scalability, and budget considerations, decide whether a public, private, or hybrid cloud is the best fit.Â
3. Select a Reliable Cloud ProviderÂ
Evaluate different providers based on cost, security measures, compliance, and service-level agreements (SLAs) to ensure they align with business goals.Â
4. Plan for Data MigrationÂ
Develop a step-by-step migration plan, starting with less critical workloads and gradually transitioning to the cloud. Engage IT experts or cloud consultants if needed.Â
5. Train EmployeesÂ
Ensure staff is equipped with the necessary skills to work efficiently with cloud-based tools. Offer training sessions and create best practices for security and usage.Â
6. Monitor and Optimize PerformanceÂ
Regularly assess cloud usage, optimize resource allocation, and update security protocols to maintain efficiency and cost-effectiveness.Â
As technology continues to evolve, cloud computing will play an even greater role in digital transformation. Advances in artificial intelligence (AI), edge computing, and serverless architectures will offer SMBs even more opportunities to innovate and streamline operations. SMBs that embrace cloud technology today will be better positioned to adapt to future technological advancements and market demands, ensuring long-term growth and competitiveness.Â
While cloud computing offers numerous advantages, SMBs must also address challenges such as data migration, integration with existing systems, and vendor reliability. Choosing the right cloud provider and strategy is essential for a successful digital transformation journey. Additionally, concerns about data privacy, potential downtime, and the need for staff training should be carefully managed. However, with proper planning, these challenges can be overcome, allowing businesses to fully unlock the potential of cloud computing.Â
Case Study: T-shirt Design Co.'s Cloud Computing SuccessÂ
Background: T-shirt Design Co. is a small business specializing in custom apparel printing and design services. With a growing customer base and increasing demand for personalized products, the company needed a scalable solution to manage its operations efficiently.Â
Challenge: As T-shirt Design Co. expanded, it faced several challenges, including managing a growing inventory, processing numerous orders, and maintaining customer satisfaction. The company also needed a cost-effective way to store and analyze customer data to drive marketing efforts and product development.Â
Solution: T-shirt Design Co. decided to adopt cloud computing by partnering with a leading cloud service provider, Microsoft Azure. The company leveraged various cloud-based solutions to address its challenges:Â
Cloud Storage: T-shirt Design Co. moved its inventory management and order processing systems to the cloud, enabling real-time tracking and updates. This ensured accurate inventory levels and timely order fulfillment.Â
Customer Relationship Management (CRM): The company implemented a cloud-based CRM system to store and analyze customer data. This allowed them to personalize marketing campaigns and improve customer engagement.Â
Collaboration Tools: By using cloud-based collaboration tools like Microsoft Teams, T-shirt Design Co. enhanced communication and coordination among its remote and in-house teams, ensuring smooth operations and faster decision-making.Â
Results:Â
Scalability: The cloud-based solutions allowed T-shirt Design Co. to scale its operations effortlessly, accommodating peak seasons and expanding its product line without significant infrastructure investments.Â
Operational Efficiency: By automating inventory management and order processing, the company reduced manual errors and improved overall efficiency. This led to faster order fulfillment and increased customer satisfaction.Â
Cost Savings: The pay-as-you-go pricing model of cloud services helped T-shirt Design Co. reduce capital expenditures and operational costs. The company could allocate resources to other critical areas, such as marketing and product development.Â
Enhanced Customer Engagement: With the cloud-based CRM system, T-shirt Design Co. gained valuable insights into customer preferences and behaviour. This enabled them to create targeted marketing campaigns, leading to higher conversion rates and customer loyalty.Â
T-shirt Design Co.'s successful implementation of cloud computing demonstrates how SMBs can leverage cloud technologies to drive digital transformation, enhance operational efficiency, and achieve sustainable growth. By adopting cloud-based solutions, the company was able to stay competitive and deliver exceptional customer experiences.Â
Cloud computing is a powerful catalyst for digital transformation in SMBs. By embracing cloud technologies, small and medium-sized businesses can enhance their operational efficiency, scalability, and innovation capabilities. The flexibility, cost savings, and advanced features offered by cloud services empower SMBs to thrive in today's dynamic business environment. Investing in the cloud is no longer a luxury but a necessity for SMBs looking to thrive in today’s digital economy. The key is to assess business needs, choose the right cloud solutions, and adopt a strategic approach to digital transformation.Â
Ready to Unlock the Full Power of Your Data?
Learn more about DataPeak:
#datapeak#factr#saas#technology#agentic ai#artificial intelligence#machine learning#ai-driven business solutions#ai#machine learning for workflow#cloud computing#cloudmigration#cloudcomputing#dataanalytics#datadrivendecisions#data analytics#the next generation#digital technology#digital trends#digitaltools#aisolutionsfordatadrivendecisionmaking#ai solutions for data driven decision making#ai tools#ai technology#ai training
0 notes
Text
Data Analytics Training In Marathahalli
Techyse Education in Marathahalli, Bangalore, offers specialized Data Analytics Training in Marathahalli for individuals looking to build expertise in Python, Power BI, and data analysis techniques. Their industry-aligned courses focus on practical learning through real-world projects, ensuring students gain hands-on experience in data manipulation, visualization, and dashboard creation. Whether you are a beginner or an experienced professional, Techyse’s programs are designed to enhance your skill set, making you job-ready for roles in data analytics.
Comprehensive Data Analytics Training in Marathahalli Techyse Education takes pride in delivering high-quality Data Analytics Training in Marathahalli, backed by experienced instructors with deep industry knowledge. The curriculum covers essential tools and techniques, from data wrangling with Python to creating interactive dashboards using Power BI, ensuring students are prepared to meet industry demands. With personalized mentorship, career support, and placement assistance, Techyse provides a well-rounded learning experience. Whether aiming for career growth or a fresh start in data analytics, Techyse Education equips learners with the skills to excel in a competitive job market.
Techyse Education | Data Analyst, Python, Power BI Training in Marathahalli, Bangalore
18, Krishna Summit, 307, 3rd Floor, Aswath Nagar, Next to Canara Bank, Marathahalli, Bangalore, Karnataka 560037
Phone: 098445 14333 Website : https://techyse.in/
Our Google Map Location is : https://maps.app.goo.gl/dLsBM669nKHTutxu9
Follow us: Facebook : https://www.facebook.com/techyse.education/ Twitter: https://x.com/techyse_edu/ Instagram : https://www.instagram.com/techyeseducation/ LinkedIn : https://www.linkedin.com/company/techyse-education/ Youtube: https://www.youtube.com/@TechyseEducation
#Data Analytics Marathahalli#Analytics Training#Data Science Marathahalli#Big Data Training#Data Analytics Course#Learn Data Analytics#Marathahalli Training#Data Science Bangalore#Analytics Experts#Data Analyst Training#Data Skills#Marathahalli Courses#Data Analytics India#Bangalore Training#Data Visualization#SQL Training#Python for Data Analytics#Machine Learning Training#Data Science Bootcamp#Business Intelligence#AI and Analytics#Learn Data Science#Career in Analytics#Analytics Certification#Marathahalli Academy#Data Driven#Advanced Analytics#Real World Analytics#Data Analytics Hub#Data Science Courses Bangalore
0 notes
Text
What Is the Future of Digital Marketing in the Age of AI?
As artificial intelligence (AI) continues to evolve, it is dramatically altering the landscape of digital marketing. No longer just a futuristic concept, AI has become an essential tool that companies of all sizes are leveraging to streamline processes, improve customer experiences, and stay competitive. But what is the future of digital marketing in the age of AI, and how will these changes…
#AI advancements#AI algorithms#AI and privacy#AI and SEO#AI automation#AI chatbots#AI content#AI content creation#AI data#AI ethics#AI in advertising#AI in business#AI in customer service#AI in marketing#AI marketing#AI optimization#AI personalization#AI technology#AI tools#AI training#AI trends#AI-driven#AI-Powered#automation#chatbots#content creation#content strategy#customer engagement#customer experience#data privacy
0 notes
Text
Mastering Sales Force Management: Strategies for Efficient Team Performance
This blog explains the advanced strategies for managing a sales force, emphasizing data-driven insights, sophisticated management techniques, and technology integration to elevate performance.
#Sales Force Management#Team Performance#Data-Driven Decision Making#Sales Strategies#Leadership#Technology Integration#Performance Monitoring#Continuous Improvement#Customer Relationship Management (CRM)#Sales Enablement Tools#Sales Analytics#AI in Sales#Sales Training#Sales Motivation#Team Collaboration Tools
0 notes
Text
One assessment suggests that ChatGPT, the chatbot created by OpenAI in San Francisco, California, is already consuming the energy of 33,000 homes. It’s estimated that a search driven by generative AI uses four to five times the energy of a conventional web search. Within years, large AI systems are likely to need as much energy as entire nations. And it’s not just energy. Generative AI systems need enormous amounts of fresh water to cool their processors and generate electricity. In West Des Moines, Iowa, a giant data-centre cluster serves OpenAI’s most advanced model, GPT-4. A lawsuit by local residents revealed that in July 2022, the month before OpenAI finished training the model, the cluster used about 6% of the district’s water. As Google and Microsoft prepared their Bard and Bing large language models, both had major spikes in water use — increases of 20% and 34%, respectively, in one year, according to the companies’ environmental reports. One preprint suggests that, globally, the demand for water for AI could be half that of the United Kingdom by 2027. In another, Facebook AI researchers called the environmental effects of the industry’s pursuit of scale the “elephant in the room”. Rather than pipe-dream technologies, we need pragmatic actions to limit AI’s ecological impacts now.
1K notes
·
View notes
Text
the scale of AI's ecological footprint
standalone version of my response to the following:
"you need soulless art? [...] why should you get to use all that computing power and electricity to produce some shitty AI art? i don’t actually think you’re entitled to consume those resources." "i think we all deserve nice things. [...] AI art is not a nice thing. it doesn’t meaningfully contribute to us thriving and the cost in terms of energy use [...] is too fucking much. none of us can afford to foot the bill." "go watch some tv show or consume some art that already exists. […] you know what’s more environmentally and economically sustainable […]? museums. galleries. being in nature."
you can run free and open source AI art programs on your personal computer, with no internet connection. this doesn't require much more electricity than running a resource-intensive video game on that same computer. i think it's important to consume less. but if you make these arguments about AI, do you apply them to video games too? do you tell Fortnite players to play board games and go to museums instead?
speaking of museums: if you drive 3 miles total to a museum and back home, you have consumed more energy and created more pollution than generating AI images for 24 hours straight (this comes out to roughly 1400 AI images). "being in nature" also involves at least this much driving, usually. i don't think these are more environmentally-conscious alternatives.
obviously, an AI image model costs energy to train in the first place, but take Stable Diffusion v2 as an example: it took 40,000 to 60,000 kWh to train. let's go with the upper bound. if you assume ~125g of CO2 per kWh, that's ~7.5 tons of CO2. to put this into perspective, a single person driving a single car for 12 months emits 4.6 tons of CO2. meanwhile, for example, the creation of a high-budget movie emits 2840 tons of CO2.
is the carbon cost of a single car being driven for 20 months, or 1/378th of a Marvel movie, worth letting anyone with a mid-end computer, anywhere, run free offline software that consumes a gaming session's worth of electricity to produce hundreds of images? i would say yes. in a heartbeat.
even if you see creating AI images as "less soulful" than consuming Marvel/Fortnite content, it's undeniably "more useful" to humanity as a tool. not to mention this usefulness includes reducing the footprint of creating media. AI is more environment-friendly than human labor on digital creative tasks, since it can get a task done with much less computer usage, doesn't commute to work, and doesn't eat.
and speaking of eating, another comparison: if you made an AI image program generate images non-stop for every second of every day for an entire year, you could offset your carbon footprint by… eating 30% less beef and lamb. not pork. not even meat in general. just beef and lamb.
the tech industry is guilty of plenty of horrendous stuff. but when it comes to the individual impact of AI, saying "i don’t actually think you’re entitled to consume those resources. do you need this? is this making you thrive?" to an individual running an AI program for 45 minutes a day per month is equivalent to questioning whether that person is entitled to a single 3 mile car drive once per month or a single meatball's worth of beef once per month. because all of these have the same CO2 footprint.
so yeah. i agree, i think we should drive less, eat less beef, stream less video, consume less. but i don't think we should tell people "stop using AI programs, just watch a TV show, go to a museum, go hiking, etc", for the same reason i wouldn't tell someone "stop playing video games and play board games instead". i don't think this is a productive angle.
(sources and number-crunching under the cut.)
good general resource: GiovanH's article "Is AI eating all the energy?", which highlights the negligible costs of running an AI program, the moderate costs of creating an AI model, and the actual indefensible energy waste coming from specific companies deploying AI irresponsibly.
CO2 emissions from running AI art programs: a) one AI image takes 3 Wh of electricity. b) one AI image takes 1mn in, for example, Midjourney. c) so if you create 1 AI image per minute for 24 hours straight, or for 45 minutes per day for a month, you've consumed 4.3 kWh. d) using the UK electric grid through 2024 as an example, the production of 1 kWh releases 124g of CO2. therefore the production of 4.3 kWh releases 533g (~0.5 kg) of CO2.
CO2 emissions from driving your car: cars in the EU emit 106.4g of CO2 per km. that's 171.19g for 1 mile, or 513g (~0.5 kg) for 3 miles.
costs of training the Stable Diffusion v2 model: quoting GiovanH's article linked in 1. "Generative models go through the same process of training. The Stable Diffusion v2 model was trained on A100 PCIe 40 GB cards running for a combined 200,000 hours, which is a specialized AI GPU that can pull a maximum of 300 W. 300 W for 200,000 hours gives a total energy consumption of 60,000 kWh. This is a high bound that assumes full usage of every chip for the entire period; SD2’s own carbon emission report indicates it likely used significantly less power than this, and other research has shown it can be done for less." at 124g of CO2 per kWh, this comes out to 7440 kg.
CO2 emissions from red meat: a) carbon footprint of eating plenty of red meat, some red meat, only white meat, no meat, and no animal products the difference between a beef/lamb diet and a no-beef-or-lamb diet comes down to 600 kg of CO2 per year. b) Americans consume 42g of beef per day. this doesn't really account for lamb (egads! my math is ruined!) but that's about 1.2 kg per month or 15 kg per year. that single piece of 42g has a 1.65kg CO2 footprint. so our 3 mile drive/4.3 kWh of AI usage have the same carbon footprint as a 12g piece of beef. roughly the size of a meatball [citation needed].
311 notes
·
View notes
Text
Still thinking about "Dot and Bubble."
Specifically, I'm thinking about how the racists of FineTime aren't just written to be cruel and entitled, but downright childish too. Lindy - in a move that dovetails nicely into the episode's commentary on social media - has the attention span of a toddler, going on and on about how boring work is even though, from what we're shown, she doesn't have to do anything other than sit there and socialize, which is presumably what she'd be doing if she didn't have to work, right? But since this is something she has to do per orders of the gross old people, she complains. "You're no fun!" she yells at Gothic Paul, the only one in her group taking a mature stance on this issue (and, notably, the only one with a very small number of subscribers).
Lindy lacks the maturity and critical thinking skills we would expect from someone her age. Again, this is definitely a layer of the social media side of the episode's thesis, but she nevertheless demonstrates a kind of emotional dysregulation that's usually only seen in younger, developing children. Lindy does not think for herself and cannot adapt to changes in routine/the way things are "supposed" to be. When told a fact - the police are unavailable - Lindy repeats, "but I really need the police" over and over as if her need is going to magic up a change in reality. She parrots rules and rejects them in equal measure, driven solely by her current desires: "We don't do that [lower the bubble]."/"I can do whatever I want!" She moves from disgusted to infatuated to angry in the blink of an eye, with her anger characterized by childish outbursts and language: "Now shut up I hate you, I hate you, I hate you!" When faced with something life-threatening, Lindy's response is to a) distract herself (by watching Ricky) and b) find a hiding place. Even taking her terror into account, she responds to these situations like someone far younger would. If I cover my eyes the bad thing disappears. If I hide under the bed, I'm safe.
And of course, Lindy's body is monitored in the way you would a child's. She's constantly watched by others, both her peers and, presumably, by the Homeworld. She's told when she needs to use the restroom which for me was VERY evocative of a parent speaking to their potty training child, trying to get them to articulate when they need to go by informing them of when it's most likely. Hell, Lindy literally can't walk without the assistance of this AI parent.
Yes, there are plenty of moments that evoke the very stereotypical, entitled teenager - talk of "partying," bragging about clothes, being obsessed with the guy online - but even more, I think, evoke the child. When Lindy plays the recording of "Mummy," smiling shyly at the praise before throwing out the kind of insults you'd expect to hear on an elementary school playground - "You're stupid" - she reads like she's a kid. Which is a hell of a commentary on her racism. The episode doesn't say that Lindy is literally a child (she's not, she even snaps as much). The episode also doesn't try to claim that being childlike equals harmless (quite the opposite). But equating racism with a childish, dangerously inept, can't-even-walk-or-use-the-bathroom-by-herself white woman... damn if that's not a statement.
483 notes
·
View notes
Note
I think your V and J designs are some of my favorite fan designs I've seen so far. Good work!
As far as the AU goes, what's the basic concept of it with revealing any spoilers?
So I don’t have a full plot or anything but I can give some background info!
Before the disassembly drones were sent to Copper 9, Cyn had decided to erase their memories and personalities, at least attempt to. Turns out completely erasing the personalities of Tessa’s personal drones wasn’t as easy as just deleting them. Cyn infected them with a virus that would slowly eat away at the complicated code of their personality AI with a little bit of psychological torment to hurry the process along (but mostly for fun giggle)
They were reverted to a primitive state, basically like untrained nueral networks that was only given a basic drive to hunt and kill to survive. It wasn’t permanent though, their AIs were still able to grow and relearn what was lost. after a few years, the disassemblers started to regain their sentience.
J was the first to regain her faculties, while N and V were still feral. They're at the phase where they can understand language but just aren’t able to speak yet and are still driven by their hunting instincts. To cope with the whole sudden sentience after years of mindlessly killing and eating worker drones, and her lost traumatic memories slowly coming back. She tries to bring more structure to this killing, remembering Tessa’s business lessons and taking inspiration to create a new management (ha ha)
only problem is she pretty much has to train dogs (or a dog and a cat) to run a business.
She doesn’t take orders from Cyn and is basically just making the best of her situation with her subordinates.
There’s a whole other thing going on with the workers, Nori being alive, Kahn dying before he could become the Doorman. I’ll have more info on Uzi, Nori and the others when I design them, cuz explaining them would kinda spoil the designs
Hope this is something, I’m more of an artist then a writer and didn’t expect this to get much attention but feel free to ask more questions or give suggestions :)
#murder drones#murder drones au#new management au#j murder drones#v murder drones#n murder drones#ask
220 notes
·
View notes
Text
EXPOSED: DARPA’s Role in Creating AI Censorship Tools Now Used Against Americans
Mike Benz revealed to Joe Rogan how DARPA developed the AI-driven censorship technology now weaponized against free speech in America. Originally designed to monitor terrorist groups like ISIS, these tools evolved into "weapons of mass deletion," capable of eliminating entire political movements and controlling narratives with just a few lines of code. Benz compared this censorship technology to an "atom bomb" for speech, permanently altering political warfare by automating suppression without the need for a vast network of human censors. His research since 2016 uncovered how machine learning models trained on millions of tweets were used to map and censor politically sensitive topics, proving that these powerful tools, once intended for national security, have been turned inward against the American citizens. 🤔
#pay attention#educate yourselves#educate yourself#reeducate yourselves#knowledge is power#reeducate yourself#think about it#think for yourselves#think for yourself#do your homework#do your own research#do some research#do your research#ask yourself questions#question everything#government corruption#government secrets#government lies#truth be told#lies exposed#darpa#evil lives here#joe rogan#censorship#news#you decide#crimes against humanity#free speech#1st amendment
96 notes
·
View notes
Text
youtube
#Oncology education#artificial intelligence#AI in healthcare#cancer diagnostics#machine learning#precision medicine#personalized medicine#radiomics#predictive analytics#cancer treatment#genomics#immunotherapy#medical education#AI-driven tools#data-driven decision-making#healthcare technology#cancer research#real-time data analysis#oncology training#future of oncology.#Youtube
0 notes
Text
Gartner Data & Analytics Summit São Paulo: Mercado Livre’s AI and Data Democratization in Brazil
New Post has been published on https://thedigitalinsider.com/gartner-data-analytics-summit-sao-paulo-mercado-livres-ai-and-data-democratization-in-brazil/
Gartner Data & Analytics Summit São Paulo: Mercado Livre’s AI and Data Democratization in Brazil
I had the opportunity to attend the Gartner Data & Analytics Summit in São Paulo, Brazil, from March 25-27. The conference brought together industry leaders, experts, and practitioners to discuss the latest trends, strategies, and best practices in data and analytics. Brazil’s growing importance in the AI landscape was evident throughout the event, with many insightful presentations and discussions focusing on AI adoption and innovation.
One of the interesting talks I attended was delivered by Eduardo Cantero Gonçalves, a senior Data Analytics manager at Mercado Livre (MercadoLibre). Mercado Livre is a leading e-commerce and fintech company that has established itself as a dominant player in the Latin American market. With operations spanning 18 countries, including major economies such as Brazil, Argentina, Mexico, and Colombia, Mercado Livre has built a vast online commerce and payments ecosystem. The company’s strong market presence and extensive user base have positioned it as a leader in the region.
During his presentation, Gonçalves shared Mercado Livre’s remarkable journey in democratizing data and AI across the organization while fostering a strong data-driven culture. As AI continues to transform industries worldwide, Mercado Livre’s experience offers valuable lessons for organizations looking to harness the power of AI and build a data-driven culture.
In this article, we will explore the key takeaways from Gonçalves’s presentation, focusing on the company’s approach to data democratization, empowering non-technical users with low-code AI tools, and cultivating a data-driven mindset throughout the organization.
Mercado Livre’s Data Democratization Journey
Mercado Livre’s journey towards data democratization has been a transformative process that has reshaped the company’s approach to data and AI. Gonçalves emphasized the importance of transitioning from a centralized data environment to a decentralized one, enabling teams across the organization to access and leverage data for decision-making and innovation.
A key aspect of this transition was the development of in-house data tools. By creating their own tools, Mercado Livre was able to tailor solutions to their specific needs and ensure seamless integration with their existing systems. This approach not only provided greater flexibility but also fostered a sense of ownership and collaboration among teams.
One of the most significant milestones in Mercado Livre’s data democratization journey was the introduction of machine learning tools designed for both data scientists and business users. Gonçalves highlighted the importance of empowering non-technical users to harness the power of AI and ML without relying heavily on data science teams. By providing low-code tools and intuitive interfaces, Mercado Livre has enabled business users to experiment with AI and ML, driving innovation and efficiency across various departments.
The democratization of data and AI has had a profound impact on Mercado Livre’s operations and culture. It has fostered a more collaborative and data-driven environment, where teams can easily access and analyze data to inform their strategies and decision-making processes. This shift has not only improved operational efficiency but has also opened up new opportunities for growth and innovation.
Empowering Non-Technical Users with Low-Code AI Tools
One of the key highlights of Mercado Livre’s data democratization journey is their focus on empowering non-technical users with low-code AI tools. During his presentation, Gonçalves emphasized the importance of enabling business users to experiment with AI and machine learning without relying heavily on data science teams.
To achieve this, Mercado Livre developed an in-house tool called “Data Switch,” which serves as a single web portal for users to access all data-related tools, including query builders, dashboards, and machine learning tools. This centralized platform makes it easier for non-technical users to leverage AI and ML capabilities without needing extensive programming knowledge.
Gonçalves specifically mentioned that Mercado Livre introduced low-code machine learning tools to allow business users to run experiments independently. By providing intuitive interfaces and pre-built models, these tools enable domain experts to apply their knowledge and insights to AI-powered solutions. This approach not only democratizes AI but also accelerates innovation by allowing more people within the organization to contribute to AI initiatives.
The impact of empowering non-technical users with low-code AI tools has been significant for Mercado Livre. Gonçalves noted that the company saw a substantial increase in the number of active users, data storage, ETL jobs, and dashboards following the introduction of these tools.
Mercado Livre’s success in this area serves as a valuable case study for other organizations looking to democratize AI and empower their workforce. By investing in low-code AI tools and providing the necessary training and support, companies can unlock the potential of their non-technical users and foster a culture of innovation.
Fostering a Data-Driven Culture
In addition to democratizing data and AI tools, Mercado Livre recognized the importance of fostering a data-driven culture throughout the organization. Gonçalves highlighted several key initiatives that the company undertook to cultivate a mindset that embraces data and AI-driven decision-making.
One notable step was the creation of a dedicated Data Culture area within Mercado Livre. This team was tasked with promoting data literacy, providing training, and supporting data-driven initiatives across the organization.
To measure the success of their data culture efforts, Mercado Livre developed a “Data Driven Index” that tracks user engagement with data tools. This index provides a quantitative measure of how well employees are adopting and leveraging data in their daily work. By regularly monitoring this index, the company can identify areas for improvement and adjust their strategies accordingly.
Another key initiative was the “Data Champions” program, which aimed to train advanced users who could then help multiply the data-driven culture throughout the organization. These champions serve as advocates and mentors, promoting best practices and assisting their colleagues in leveraging data and AI tools effectively. By empowering a network of champions, Mercado Livre was able to scale its data culture efforts and drive adoption across the company.
Lessons Learned from Mercado Livre’s Experience
Mercado Livre’s journey in democratizing data and AI offers valuable lessons for other organizations looking to embark on a similar path. One of the key takeaways from Gonçalves’s presentation was the importance of executive sponsorship in promoting a data-driven culture. Having strong support and advocacy from leadership is critical in driving organizational change and ensuring that data and AI initiatives are given the necessary resources and priority.
Another important lesson is the value of collaborating with HR to integrate data-driven culture into employee onboarding and development programs. By making data literacy and AI skills a core part of employee training, organizations can ensure that their workforce is well-equipped to leverage these tools effectively. Mercado Livre’s partnership with HR helped them to scale their data culture efforts and make it a fundamental part of their employees’ growth and development.
Finally, Gonçalves emphasized the importance of continuously measuring and iterating on data-driven initiatives. By tracking key metrics such as the Data Driven Index and regularly seeking feedback from employees, organizations can identify areas for improvement and make data-informed decisions to optimize their strategies. This iterative approach ensures that data and AI initiatives remain aligned with business objectives and drive meaningful impact.
As organizations navigate the challenges and opportunities of the AI era, Mercado Livre’s experience serves as a valuable case study in democratizing data and AI while fostering a data-driven culture. By empowering employees at all levels to leverage these tools and cultivating a mindset that embraces data-driven decision-making, companies can position themselves for success in our AI-driven world.
#ai#ai tools#AI-powered#amp#Analytics#approach#Article#Artificial Intelligence#Brazil#Business#Case Study#change#code#Collaboration#collaborative#Commerce#Companies#conference#data#data analytics#data democratization#data literacy#data science#data storage#data-driven#democratization#development#E-Commerce#efficiency#Employee Training
0 notes
Text
Techyse Education in Marathahalli, Bangalore, offers specialized Data Analytics Training in Marathahalli for individuals looking to build expertise in Python, Power BI, and data analysis techniques. Their industry-aligned courses focus on practical learning through real-world projects, ensuring students gain hands-on experience in data manipulation, visualization, and dashboard creation. Whether you are a beginner or an experienced professional, Techyse’s programs are designed to enhance your skill set, making you job-ready for roles in data analytics.
Comprehensive Data Analytics Training in Marathahalli Techyse Education takes pride in delivering high-quality Data Analytics Training in Marathahalli, backed by experienced instructors with deep industry knowledge. The curriculum covers essential tools and techniques, from data wrangling with Python to creating interactive dashboards using Power BI, ensuring students are prepared to meet industry demands. With personalized mentorship, career support, and placement assistance, Techyse provides a well-rounded learning experience. Whether aiming for career growth or a fresh start in data analytics, Techyse Education equips learners with the skills to excel in a competitive job market.
Techyse Education | Data Analyst, Python, Power BI Training in Marathahalli, Bangalore
18, Krishna Summit, 307, 3rd Floor, Aswath Nagar, Next to Canara Bank, Marathahalli, Bangalore, Karnataka 560037
Phone: 098445 14333 Website : https://techyse.in/
Our Google Map Location is : https://maps.app.goo.gl/dLsBM669nKHTutxu9
Follow us: Facebook : https://www.facebook.com/techyse.education/ Twitter: https://x.com/techyse_edu/ Instagram : https://www.instagram.com/techyeseducation/ LinkedIn : https://www.linkedin.com/company/techyse-education/ Youtube: https://www.youtube.com/@TechyseEducation
#Data Analytics Marathahalli#Analytics Training#Data Science Marathahalli#Big Data Training#Data Analytics Course#Learn Data Analytics#Marathahalli Training#Data Science Bangalore#Analytics Experts#Data Analyst Training#Data Skills#Marathahalli Courses#Data Analytics India#Bangalore Training#Data Visualization#SQL Training#Python for Data Analytics#Machine Learning Training#Data Science Bootcamp#Business Intelligence#AI and Analytics#Learn Data Science#Career in Analytics#Analytics Certification#Marathahalli Academy#Data Driven#Advanced Analytics#Real World Analytics#Data Analytics Hub#Data Science Courses Bangalore
0 notes