#Chatgpt data engineering
Explore tagged Tumblr posts
Text

SG Analytics - While ChatGPT is a powerful tool that can be used in data engineering, it cannot replace the expertise of a data engineer. Data engineering requires a deep understanding which ChatGPT cannot replicate.
#Data engineers#ChatGPT Replace Data Engineers#AI data engineer#Chatgpt data engineering#esg services#esg data and analytics
0 notes
Text
i hate gen AI so much i wish crab raves upon it
#genuinely this shit is like downfall of humanity to me#what do you mean you have a compsci degree and are having chatgpt write basic code for you#what do you mean you are using it to come up with recipes#what do you mean you are talking to it 24/7 like it’s your friend#what do you mean you are RPing with it#what do you mean you use it instead of researching anything for yourself#what do you mean you’re using it to write your essays instead of just writing your essays#i feel crazy i feel insane on god on GOD#i would have gotten a different degree if i knew that half the jobs that exist now for my degree are all feeding into the fucking gen AI#slop machine#what’s worse is my work experience is very much ‘automation engineering’ which is NOT AI but#using coding/technology/databases to improve existing processes and make them easier and less tedious for people#to free them up to do things that involve more brainpower than tedious data entry/etc#SO ESPECIALLY so many of the jobs i would have been able to take with my work experience is now very gen AI shit and i just refuse to fuckin#do that shit?????
2 notes
·
View notes
Text
How Does AI Use Impact Critical Thinking?
New Post has been published on https://thedigitalinsider.com/how-does-ai-use-impact-critical-thinking/
How Does AI Use Impact Critical Thinking?
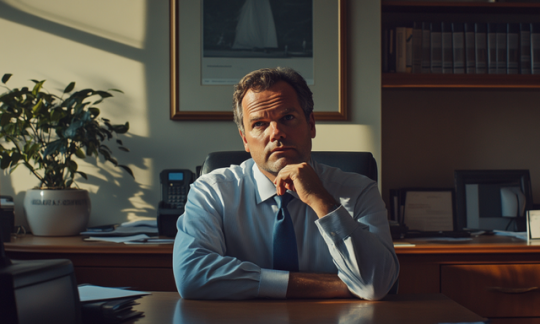
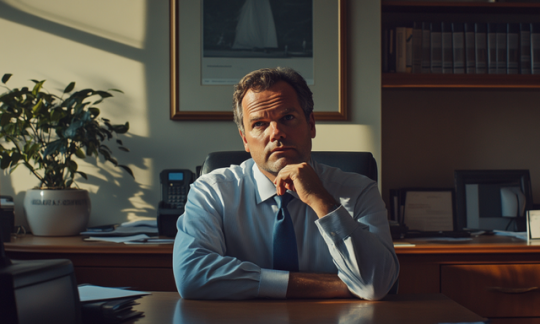
Artificial intelligence (AI) can process hundreds of documents in seconds, identify imperceptible patterns in vast datasets and provide in-depth answers to virtually any question. It has the potential to solve common problems, increase efficiency across multiple industries and even free up time for individuals to spend with their loved ones by delegating repetitive tasks to machines.
However, critical thinking requires time and practice to develop properly. The more people rely on automated technology, the faster their metacognitive skills may decline. What are the consequences of relying on AI for critical thinking?
Study Finds AI Degrades Users’ Critical Thinking
The concern that AI will degrade users’ metacognitive skills is no longer hypothetical. Several studies suggest it diminishes people’s capacity to think critically, impacting their ability to question information, make judgments, analyze data or form counterarguments.
A 2025 Microsoft study surveyed 319 knowledge workers on 936 instances of AI use to determine how they perceive their critical thinking ability when using generative technology. Survey respondents reported decreased effort when using AI technology compared to relying on their own minds. Microsoft reported that in the majority of instances, the respondents felt that they used “much less effort” or “less effort” when using generative AI.
Knowledge, comprehension, analysis, synthesis and evaluation were all adversely affected by AI use. Although a fraction of respondents reported using some or much more effort, an overwhelming majority reported that tasks became easier and required less work.
If AI’s purpose is to streamline tasks, is there any harm in letting it do its job? It is a slippery slope. Many algorithms cannot think critically, reason or understand context. They are often prone to hallucinations and bias. Users who are unaware of the risks of relying on AI may contribute to skewed, inaccurate results.
How AI Adversely Affects Critical Thinking Skills
Overreliance on AI can diminish an individual’s ability to independently solve problems and think critically. Say someone is taking a test when they run into a complex question. Instead of taking the time to consider it, they plug it into a generative model and insert the algorithm’s response into the answer field.
In this scenario, the test-taker learned nothing. They didn’t improve their research skills or analytical abilities. If they pass the test, they advance to the next chapter. What if they were to do this for everything their teachers assign? They could graduate from high school or even college without refining fundamental cognitive abilities.
This outcome is bleak. However, students might not feel any immediate adverse effects. If their use of language models is rewarded with better test scores, they may lose their motivation to think critically altogether. Why should they bother justifying their arguments or evaluating others’ claims when it is easier to rely on AI?
The Impact of AI Use on Critical Thinking Skills
An advanced algorithm can automatically aggregate and analyze large datasets, streamlining problem-solving and task execution. Since its speed and accuracy often outperform humans, users are usually inclined to believe it is better than them at these tasks. When it presents them with answers and insights, they take that output at face value. Unquestioning acceptance of a generative model’s output leads to difficulty distinguishing between facts and falsehoods. Algorithms are trained to predict the next word in a string of words. No matter how good they get at that task, they aren’t really reasoning. Even if a machine makes a mistake, it won’t be able to fix it without context and memory, both of which it lacks.
The more users accept an algorithm’s answer as fact, the more their evaluation and judgment skew. Algorithmic models often struggle with overfitting. When they fit too closely to the information in their training dataset, their accuracy can plummet when they are presented with new information for analysis.
Populations Most Affected by Overreliance on AI
Generally, overreliance on generative technology can negatively impact humans’ ability to think critically. However, low confidence in AI-generated output is related to increased critical thinking ability, so strategic users may be able to use AI without harming these skills.
In 2023, around 27% of adults told the Pew Research Center they use AI technology multiple times a day. Some of the individuals in this population may retain their critical thinking skills if they have a healthy distrust of machine learning tools. The data must focus on populations with disproportionately high AI use and be more granular to determine the true impact of machine learning on critical thinking.
Critical thinking often isn’t taught until high school or college. It can be cultivated during early childhood development, but it typically takes years to grasp. For this reason, deploying generative technology in schools is particularly risky — even though it is common.
Today, most students use generative models. One study revealed that 90% have used ChatGPT to complete homework. This widespread use isn’t limited to high schools. About 75% of college students say they would continue using generative technology even if their professors disallowed it. Middle schoolers, teenagers and young adults are at an age where developing critical thinking is crucial. Missing this window could cause problems.
The Implications of Decreased Critical Thinking
Already, 60% of educators use AI in the classroom. If this trend continues, it may become a standard part of education. What happens when students begin to trust these tools more than themselves? As their critical thinking capabilities diminish, they may become increasingly susceptible to misinformation and manipulation. The effectiveness of scams, phishing and social engineering attacks could increase.
An AI-reliant generation may have to compete with automation technology in the workforce. Soft skills like problem-solving, judgment and communication are important for many careers. Lacking these skills or relying on generative tools to get good grades may make finding a job challenging.
Innovation and adaptation go hand in hand with decision-making. Knowing how to objectively reason without the use of AI is critical when confronted with high-stakes or unexpected situations. Leaning into assumptions and inaccurate data could adversely affect an individual’s personal or professional life.
Critical thinking is part of processing and analyzing complex — and even conflicting — information. A community made up of critical thinkers can counter extreme or biased viewpoints by carefully considering different perspectives and values.
AI Users Must Carefully Evaluate Algorithms’ Output
Generative models are tools, so whether their impact is positive or negative depends on their users and developers. So many variables exist. Whether you are an AI developer or user, strategically designing and interacting with generative technologies is an important part of ensuring they pave the way for societal advancements rather than hindering critical cognition.
#2023#2025#ai#AI technology#algorithm#Algorithms#Analysis#artificial#Artificial Intelligence#automation#Bias#Careers#chatGPT#cognition#cognitive abilities#college#communication#Community#comprehension#critical thinking#data#datasets#deploying#Developer#developers#development#education#effects#efficiency#engineering
2 notes
·
View notes
Text
🚀 Meta's New Ad Tools for Facebook & Instagram Are Here! 🎯
Meta just released exciting updates to its ad platforms, aimed at making your campaigns smarter and more effective. Here's a quick look at what’s new and why it matters:
1️⃣ Smarter Targeting with AI: Meta's AI-powered optimization helps you polish your ad targeting. Whether you're customizing ads for different audiences or adjusting campaigns, these tools are designed to reach the right people more efficiently.
2️⃣ New Incremental Attribution Model: This new attribution setting targets those who are more likely to convert after seeing your ad—customers who wouldn’t have taken action otherwise. Early tests show an average 20% increase in incremental conversions, ensuring your ads have a real impact.
3️⃣ Better Analytics Integration: Meta is simplifying connections with external analytics tools like Google Analytics and Adobe, providing a clearer view of how your campaigns perform across platforms. Now, you can track and understand the full customer journey, from paid social to SEO, all in one place.
✨ Key Features :
- Conversion Value Rules: Prioritize high-value customers without creating separate campaigns. Adjust your bids for different customer actions based on long-term value, so you can place higher bids on customers who offer more value over time—all within the same campaign.
- Incremental Attribution: Focus on “incremental conversions”—customers who wouldn’t have converted without seeing your ad. Early adopters have seen a 20% rise in these valuable conversions.
- Cross-Platform Analytics: Meta’s direct connections with analytics platforms allow you to merge data from different channels, giving you a holistic view of your ad performance. Early tests show a 30% increase in conversions when third-party analytics tools like Google Analytics are used alongside Meta ads.
These updates are about improving the precision and efficiency of your ad campaigns. Meta’s new AI-driven features help you achieve better results, make smarter decisions, and maximize the value of your ad spend.
💡 What to Do Now :
✔️ Review your current Meta ad strategy to ensure you're ready to take advantage of these tools.
✔️ Map out your customer journey to identify where these new features can add the most value.
✔️ Be prepared to test these updates as they roll out—early adopters are already seeing impressive gains.
How do you plan to use these new Meta tools? Share your thoughts in the comments below! 💬
📌Follow us on Social Media📌
📢 LinkedIn — Vedang Kadia — Amazon Associate | LinkedIn
📢 Quora — Vedang Kadia
📢 Tumblr — Untitled
📢 Medium — Vedang Kadia — Medium
#digital marketing#social media marketing#facebook ads#instagram ads#adtech#facebook meta#artificial intelligence#data analytics#google analytics#seo services#tumbler#search engine optimization#marketing strategy#tech news#openai#india#chatgpt
4 notes
·
View notes
Text
Exploring Quantum Leap Sort: A Conceptual Dive into Probabilistic Sorting Created Using AI
In the vast realm of sorting algorithms, where QuickSort, MergeSort, and HeapSort reign supreme, introducing a completely new approach is no small feat. Today, we’ll delve into a purely theoretical concept—Quantum Leap Sort—an imaginative algorithm created using AI that draws inspiration from quantum mechanics and probabilistic computing. While not practical for real-world use, this novel…
#AI#algorithm#amazon#chatgpt#coding#computer science#css#data-structures#DSA#engineering#google#heapsort#insertionsort#javascript#mergesort#new#programming#python#quicksort#radixsort#sorting#tech#tesla#trending#wipro
2 notes
·
View notes
Text
i was curious so i went to google to try and didn’t get their same error

i got a fucking stupider one
anyways DuckDuckGo is an independent search engine that doesn’t track or log ur searches and also doesn’t have a hallucinating AI trained on copyrighted material tellin you weight in inches long.
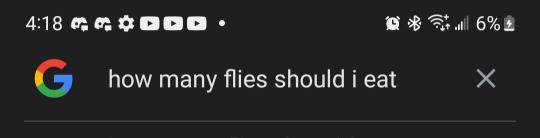
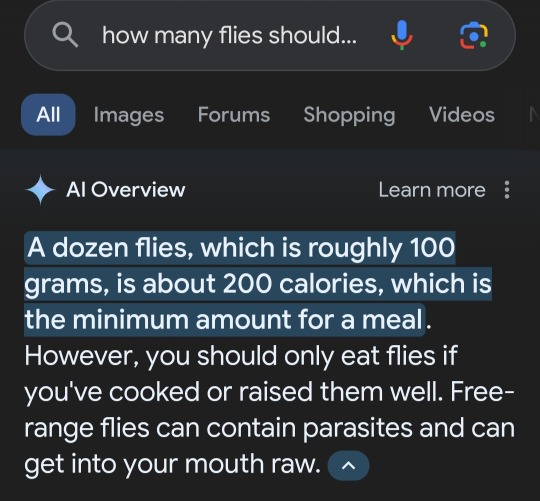
glad google ai is on top of this
#google#google generative ai#i fucking hate this shit so much#while ta-ing bioinformatics i tried to burn it into people’s heads that chatgpt isn’t a search engine#you can’t find articles or citations with it because it’s a fucking statistical algorithm#it’s a word generator weighted by predictions made through finding similarities in its training data#its not a fucking intelligence. it’s a fancy d20.#AND NOW HERES FUCKIN GOOGLE#HOW MANY FOLKS ARE GONNA GET TRICKED BY THIS STUPID THING
59K notes
·
View notes
Text
Install Sublime Text Editor for Python | Python for Data Professionals | Topic #13
https://youtu.be/GK5C4SsQO98
youtube
#the data channel#data architect#prompt engineering#chatgpt for analysis#promptengineering#python#sublime#Youtube
0 notes
Text
https://coruzant.com/tech/ai-consulting-companies-guiding-businesses-into-the-future/
#ai #artificialintelligence #businesses #generativeai
#application engineering services#business intelligence services#cloud services#data management services#data analytics services#ai generated#artificial intelligence#chatgpt#technology
0 notes
Text

#ai art generator#ai#ai generated#chat gpt#chatgpt#midjourney#stable diffusion#prompt engineering#machinelearning#artificalintelligence#artificial art#artificial intelligence#ai tools#data scientist
0 notes
Note
whats wrong with ai?? genuinely curious <3
okay let's break it down. i'm an engineer, so i'm going to come at you from a perspective that may be different than someone else's.
i don't hate ai in every aspect. in theory, there are a lot of instances where, in fact, ai can help us do things a lot better without. here's a few examples:
ai detecting cancer
ai sorting recycling
some practical housekeeping that gemini (google ai) can do
all of the above examples are ways in which ai works with humans to do things in parallel with us. it's not overstepping--it's sorting, using pixels at a micro-level to detect abnormalities that we as humans can not, fixing a list. these are all really small, helpful ways that ai can work with us.
everything else about ai works against us. in general, ai is a huge consumer of natural resources. every prompt that you put into character.ai, chatgpt? this wastes water + energy. it's not free. a machine somewhere in the world has to swallow your prompt, call on a model to feed data into it and process more data, and then has to generate an answer for you all in a relatively short amount of time.
that is crazy expensive. someone is paying for that, and if it isn't you with your own money, it's the strain on the power grid, the water that cools the computers, the A/C that cools the data centers. and you aren't the only person using ai. chatgpt alone gets millions of users every single day, with probably thousands of prompts per second, so multiply your personal consumption by millions, and you can start to see how the picture is becoming overwhelming.
that is energy consumption alone. we haven't even talked about how problematic ai is ethically. there is currently no regulation in the united states about how ai should be developed, deployed, or used.
what does this mean for you?
it means that anything you post online is subject to data mining by an ai model (because why would they need to ask if there's no laws to stop them? wtf does it matter what it means to you to some idiot software engineer in the back room of an office making 3x your salary?). oh, that little fic you posted to wattpad that got a lot of attention? well now it's being used to teach ai how to write. oh, that sketch you made using adobe that you want to sell? adobe didn't tell you that anything you save to the cloud is now subject to being used for their ai models, so now your art is being replicated to generate ai images in photoshop, without crediting you (they have since said they don't do this...but privacy policies were never made to be human-readable, and i can't imagine they are the only company to sneakily try this). oh, your apartment just installed a new system that will use facial recognition to let their residents inside? oh, they didn't train their model with anyone but white people, so now all the black people living in that apartment building can't get into their homes. oh, you want to apply for a new job? the ai model that scans resumes learned from historical data that more men work that role than women (so the model basically thinks men are better than women), so now your resume is getting thrown out because you're a woman.
ai learns from data. and data is flawed. data is human. and as humans, we are racist, homophobic, misogynistic, transphobic, divided. so the ai models we train will learn from this. ai learns from people's creative works--their personal and artistic property. and now it's scrambling them all up to spit out generated images and written works that no one would ever want to read (because it's no longer a labor of love), and they're using that to make money. they're profiting off of people, and there's no one to stop them. they're also using generated images as marketing tools, to trick idiots on facebook, to make it so hard to be media literate that we have to question every single thing we see because now we don't know what's real and what's not.
the problem with ai is that it's doing more harm than good. and we as a society aren't doing our due diligence to understand the unintended consequences of it all. we aren't angry enough. we're too scared of stifling innovation that we're letting it regulate itself (aka letting companies decide), which has never been a good idea. we see it do one cool thing, and somehow that makes up for all the rest of the bullshit?
#yeah i could talk about this for years#i could talk about it forever#im so passionate about this lmao#anyways#i also want to point out the examples i listed are ONLY A FEW problems#there's SO MUCH MORE#anywho ai is bleh go away#ask#ask b#🐝's anons#ai
1K notes
·
View notes
Text
A summary of the Chinese AI situation, for the uninitiated.
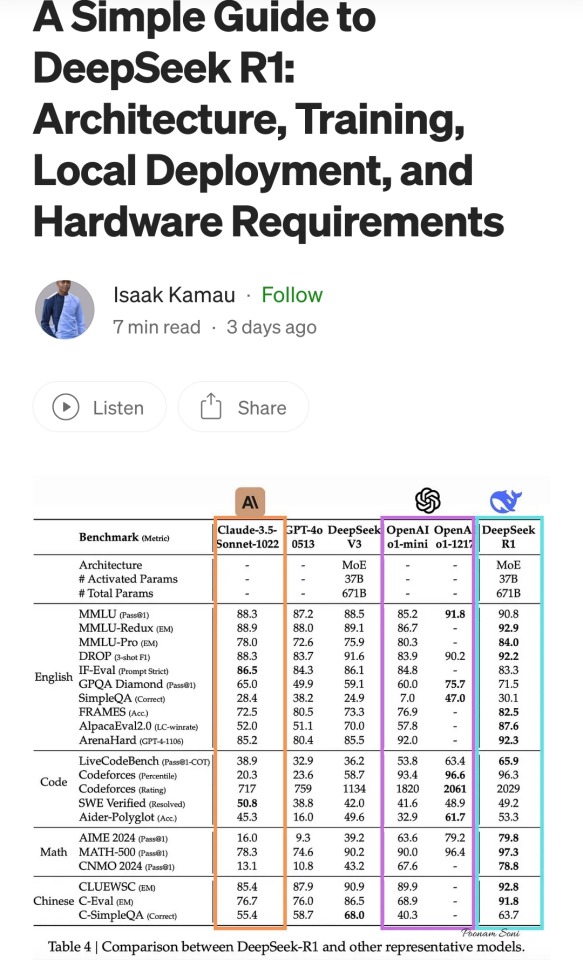
These are scores on different tests that are designed to see how accurate a Large Language Model is in different areas of knowledge. As you know, OpenAI is partners with Microsoft, so these are the scores for ChatGPT and Copilot. DeepSeek is the Chinese model that got released a week ago. The rest are open source models, which means everyone is free to use them as they please, including the average Tumblr user. You can run them from the servers of the companies that made them for a subscription, or you can download them to install locally on your own computer. However, the computer requirements so far are so high that only a few people currently have the machines at home required to run it.
Yes, this is why AI uses so much electricity. As with any technology, the early models are highly inefficient. Think how a Ford T needed a long chimney to get rid of a ton of black smoke, which was unused petrol. Over the next hundred years combustion engines have become much more efficient, but they still waste a lot of energy, which is why we need to move towards renewable electricity and sustainable battery technology. But that's a topic for another day.
As you can see from the scores, are around the same accuracy. These tests are in constant evolution as well: as soon as they start becoming obsolete, new ones are released to adjust for a more complicated benchmark. The new models are trained using different machine learning techniques, and in theory, the goal is to make them faster and more efficient so they can operate with less power, much like modern cars use way less energy and produce far less pollution than the Ford T.
However, computing power requirements kept scaling up, so you're either tied to the subscription or forced to pay for a latest gen PC, which is why NVIDIA, AMD, Intel and all the other chip companies were investing hard on much more powerful GPUs and NPUs. For now all we need to know about those is that they're expensive, use a lot of electricity, and are required to operate the bots at superhuman speed (literally, all those clickbait posts about how AI was secretly 150 Indian men in a trenchcoat were nonsense).
Because the chip companies have been working hard on making big, bulky, powerful chips with massive fans that are up to the task, their stock value was skyrocketing, and because of that, everyone started to use AI as a marketing trend. See, marketing people are not smart, and they don't understand computers. Furthermore, marketing people think you're stupid, and because of their biased frame of reference, they think you're two snores short of brain-dead. The entire point of their existence is to turn tall tales into capital. So they don't know or care about what AI is or what it's useful for. They just saw Number Go Up for the AI companies and decided "AI is a magic cow we can milk forever". Sometimes it's not even AI, they just use old software and rebrand it, much like convection ovens became air fryers.
Well, now we're up to date. So what did DepSeek release that did a 9/11 on NVIDIA stock prices and popped the AI bubble?
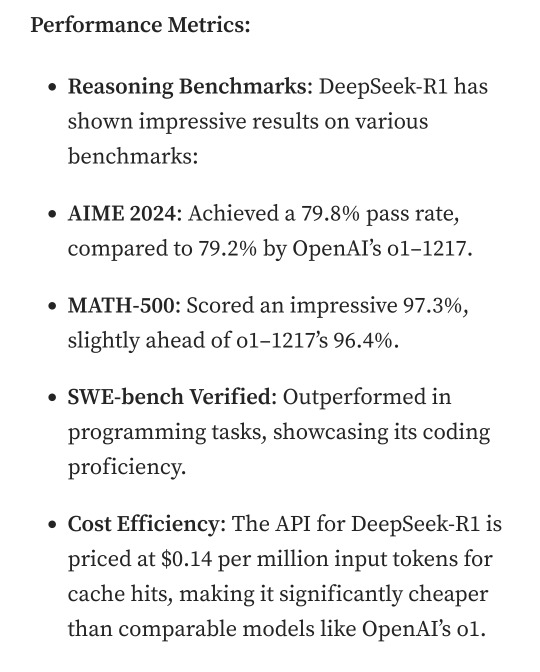
Oh, I would not want to be an OpenAI investor right now either. A token is basically one Unicode character (it's more complicated than that but you can google that on your own time). That cost means you could input the entire works of Stephen King for under a dollar. Yes, including electricity costs. DeepSeek has jumped from a Ford T to a Subaru in terms of pollution and water use.
The issue here is not only input cost, though; all that data needs to be available live, in the RAM; this is why you need powerful, expensive chips in order to-
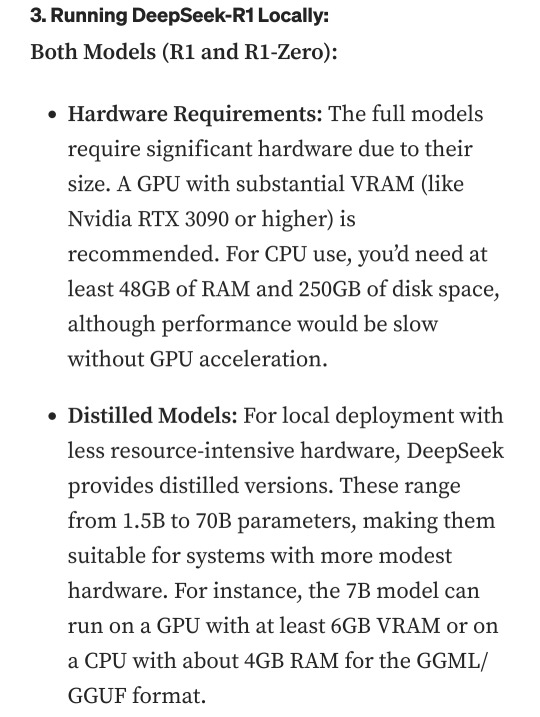
Holy shit.
I'm not going to detail all the numbers but I'm going to focus on the chip required: an RTX 3090. This is a gaming GPU that came out as the top of the line, the stuff South Korean LoL players buy…
Or they did, in September 2020. We're currently two generations ahead, on the RTX 5090.
What this is telling all those people who just sold their high-end gaming rig to be able to afford a machine that can run the latest ChatGPT locally, is that the person who bought it from them can run something basically just as powerful on their old one.
Which means that all those GPUs and NPUs that are being made, and all those deals Microsoft signed to have control of the AI market, have just lost a lot of their pulling power.
Well, I mean, the ChatGPT subscription is 20 bucks a month, surely the Chinese are charging a fortune for-
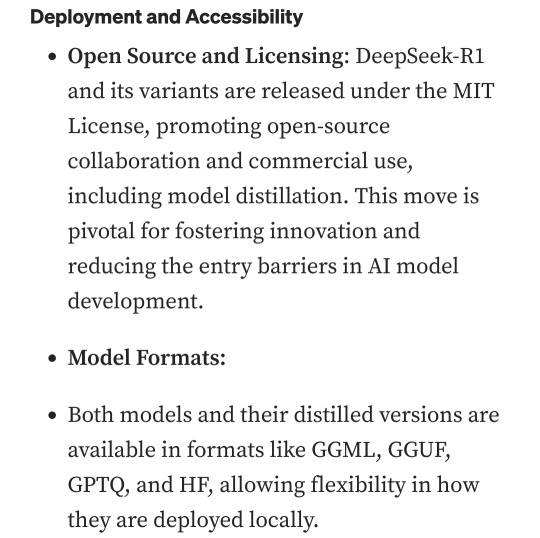
Oh. So it's free for everyone and you can use it or modify it however you want, no subscription, no unpayable electric bill, no handing Microsoft all of your private data, you can just run it on a relatively inexpensive PC. You could probably even run it on a phone in a couple years.
Oh, if only China had massive phone manufacturers that have a foot in the market everywhere except the US because the president had a tantrum eight years ago.
So… yeah, China just destabilised the global economy with a torrent file.
#valid ai criticism#ai#llms#DeepSeek#ai bubble#ChatGPT#google gemini#claude ai#this is gonna be the dotcom bubble again#hope you don't have stock on anything tech related#computer literacy#tech literacy
432 notes
·
View notes
Text
controversial, but as someone who's spent the last year tearing her hair out adjudicating student conduct cases involving AI misuse: i'm never going to be impressed by an anti-AI post that sounds like an abstinence-only educator wrote it
it goes without saying that AI as implemented has many problems.* it fills me with a low-level sense of dread! but some of you seem to think that if we teach the children to Just Say No, it will go away. which...maybe this is because i work with engineering students but that sounds about as likely as email going away (and that message is just about as likely to resonate with kids as The Internet Is Evil So Stay Off).
students are graduating into a workforce that requires them to use AI. it is, in certain contexts, an appropriate tool. so between an engineer who's never touched AI in their life and one who's had a thorough education in it--who knows what it can and can't do; what it can and can't be trusted with--i'd pick the latter every time.
like, they're going to build the airplanes for christ's sake. they're going to be handed AI, usually of higher quality than public-facing chatgpt but still. i don't want them graduating with the sense that AI is a Forbidden All-Knowing Cheating Machine instead of something they've spent time understanding the limitations of. i want them to understand which programs work for which tasks, and which don't work at all. and that requires active education on the tool, the same way you'd teach about internet safety or how to detect fake news
(this goes, imo, for people trying to make AI safer and more ethical as well. if you consider it unethical by definition to work on AI, the only people working on it will be the ones who don't care about ethics.)
-
*i will say it. misinformation, algorithmic bias, disruptions for the labor force, shoving it into tasks it's not good at LET ME SCHEDULE MY OWN DAMN VET APPOINTMENT, unethical sourcing for datasets, environmental impact (the data center planner in my life is VERY skeptical of DeepSeek being more efficient but one hopes)
172 notes
·
View notes
Text
On Saturday, an Associated Press investigation revealed that OpenAI's Whisper transcription tool creates fabricated text in medical and business settings despite warnings against such use. The AP interviewed more than 12 software engineers, developers, and researchers who found the model regularly invents text that speakers never said, a phenomenon often called a “confabulation” or “hallucination” in the AI field.
Upon its release in 2022, OpenAI claimed that Whisper approached “human level robustness” in audio transcription accuracy. However, a University of Michigan researcher told the AP that Whisper created false text in 80 percent of public meeting transcripts examined. Another developer, unnamed in the AP report, claimed to have found invented content in almost all of his 26,000 test transcriptions.
The fabrications pose particular risks in health care settings. Despite OpenAI’s warnings against using Whisper for “high-risk domains,” over 30,000 medical workers now use Whisper-based tools to transcribe patient visits, according to the AP report. The Mankato Clinic in Minnesota and Children’s Hospital Los Angeles are among 40 health systems using a Whisper-powered AI copilot service from medical tech company Nabla that is fine-tuned on medical terminology.
Nabla acknowledges that Whisper can confabulate, but it also reportedly erases original audio recordings “for data safety reasons.” This could cause additional issues, since doctors cannot verify accuracy against the source material. And deaf patients may be highly impacted by mistaken transcripts since they would have no way to know if medical transcript audio is accurate or not.
The potential problems with Whisper extend beyond health care. Researchers from Cornell University and the University of Virginia studied thousands of audio samples and found Whisper adding nonexistent violent content and racial commentary to neutral speech. They found that 1 percent of samples included “entire hallucinated phrases or sentences which did not exist in any form in the underlying audio” and that 38 percent of those included “explicit harms such as perpetuating violence, making up inaccurate associations, or implying false authority.”
In one case from the study cited by AP, when a speaker described “two other girls and one lady,” Whisper added fictional text specifying that they “were Black.” In another, the audio said, “He, the boy, was going to, I’m not sure exactly, take the umbrella.” Whisper transcribed it to, “He took a big piece of a cross, a teeny, small piece … I’m sure he didn’t have a terror knife so he killed a number of people.”
An OpenAI spokesperson told the AP that the company appreciates the researchers’ findings and that it actively studies how to reduce fabrications and incorporates feedback in updates to the model.
Why Whisper Confabulates
The key to Whisper’s unsuitability in high-risk domains comes from its propensity to sometimes confabulate, or plausibly make up, inaccurate outputs. The AP report says, "Researchers aren’t certain why Whisper and similar tools hallucinate," but that isn't true. We know exactly why Transformer-based AI models like Whisper behave this way.
Whisper is based on technology that is designed to predict the next most likely token (chunk of data) that should appear after a sequence of tokens provided by a user. In the case of ChatGPT, the input tokens come in the form of a text prompt. In the case of Whisper, the input is tokenized audio data.
The transcription output from Whisper is a prediction of what is most likely, not what is most accurate. Accuracy in Transformer-based outputs is typically proportional to the presence of relevant accurate data in the training dataset, but it is never guaranteed. If there is ever a case where there isn't enough contextual information in its neural network for Whisper to make an accurate prediction about how to transcribe a particular segment of audio, the model will fall back on what it “knows” about the relationships between sounds and words it has learned from its training data.
According to OpenAI in 2022, Whisper learned those statistical relationships from “680,000 hours of multilingual and multitask supervised data collected from the web.” But we now know a little more about the source. Given Whisper's well-known tendency to produce certain outputs like "thank you for watching," "like and subscribe," or "drop a comment in the section below" when provided silent or garbled inputs, it's likely that OpenAI trained Whisper on thousands of hours of captioned audio scraped from YouTube videos. (The researchers needed audio paired with existing captions to train the model.)
There's also a phenomenon called “overfitting” in AI models where information (in this case, text found in audio transcriptions) encountered more frequently in the training data is more likely to be reproduced in an output. In cases where Whisper encounters poor-quality audio in medical notes, the AI model will produce what its neural network predicts is the most likely output, even if it is incorrect. And the most likely output for any given YouTube video, since so many people say it, is “thanks for watching.”
In other cases, Whisper seems to draw on the context of the conversation to fill in what should come next, which can lead to problems because its training data could include racist commentary or inaccurate medical information. For example, if many examples of training data featured speakers saying the phrase “crimes by Black criminals,” when Whisper encounters a “crimes by [garbled audio] criminals” audio sample, it will be more likely to fill in the transcription with “Black."
In the original Whisper model card, OpenAI researchers wrote about this very phenomenon: "Because the models are trained in a weakly supervised manner using large-scale noisy data, the predictions may include texts that are not actually spoken in the audio input (i.e. hallucination). We hypothesize that this happens because, given their general knowledge of language, the models combine trying to predict the next word in audio with trying to transcribe the audio itself."
So in that sense, Whisper "knows" something about the content of what is being said and keeps track of the context of the conversation, which can lead to issues like the one where Whisper identified two women as being Black even though that information was not contained in the original audio. Theoretically, this erroneous scenario could be reduced by using a second AI model trained to pick out areas of confusing audio where the Whisper model is likely to confabulate and flag the transcript in that location, so a human could manually check those instances for accuracy later.
Clearly, OpenAI's advice not to use Whisper in high-risk domains, such as critical medical records, was a good one. But health care companies are constantly driven by a need to decrease costs by using seemingly "good enough" AI tools—as we've seen with Epic Systems using GPT-4 for medical records and UnitedHealth using a flawed AI model for insurance decisions. It's entirely possible that people are already suffering negative outcomes due to AI mistakes, and fixing them will likely involve some sort of regulation and certification of AI tools used in the medical field.
87 notes
·
View notes
Text
Scientists use generative AI to answer complex questions in physics
New Post has been published on https://thedigitalinsider.com/scientists-use-generative-ai-to-answer-complex-questions-in-physics/
Scientists use generative AI to answer complex questions in physics
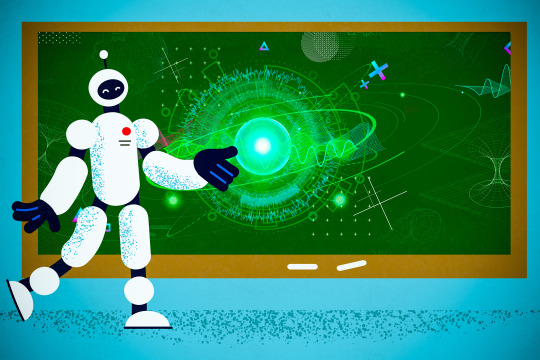
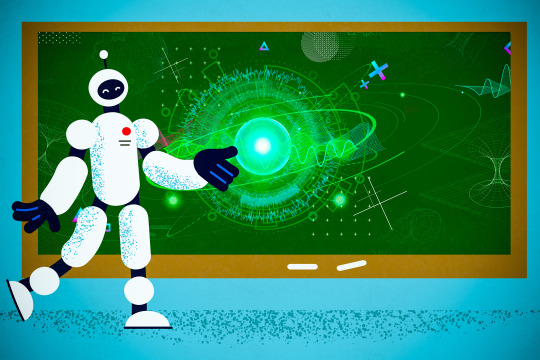
When water freezes, it transitions from a liquid phase to a solid phase, resulting in a drastic change in properties like density and volume. Phase transitions in water are so common most of us probably don’t even think about them, but phase transitions in novel materials or complex physical systems are an important area of study.
To fully understand these systems, scientists must be able to recognize phases and detect the transitions between. But how to quantify phase changes in an unknown system is often unclear, especially when data are scarce.
Researchers from MIT and the University of Basel in Switzerland applied generative artificial intelligence models to this problem, developing a new machine-learning framework that can automatically map out phase diagrams for novel physical systems.
Their physics-informed machine-learning approach is more efficient than laborious, manual techniques which rely on theoretical expertise. Importantly, because their approach leverages generative models, it does not require huge, labeled training datasets used in other machine-learning techniques.
Such a framework could help scientists investigate the thermodynamic properties of novel materials or detect entanglement in quantum systems, for instance. Ultimately, this technique could make it possible for scientists to discover unknown phases of matter autonomously.
“If you have a new system with fully unknown properties, how would you choose which observable quantity to study? The hope, at least with data-driven tools, is that you could scan large new systems in an automated way, and it will point you to important changes in the system. This might be a tool in the pipeline of automated scientific discovery of new, exotic properties of phases,” says Frank Schäfer, a postdoc in the Julia Lab in the Computer Science and Artificial Intelligence Laboratory (CSAIL) and co-author of a paper on this approach.
Joining Schäfer on the paper are first author Julian Arnold, a graduate student at the University of Basel; Alan Edelman, applied mathematics professor in the Department of Mathematics and leader of the Julia Lab; and senior author Christoph Bruder, professor in the Department of Physics at the University of Basel. The research is published today in Physical Review Letters.
Detecting phase transitions using AI
While water transitioning to ice might be among the most obvious examples of a phase change, more exotic phase changes, like when a material transitions from being a normal conductor to a superconductor, are of keen interest to scientists.
These transitions can be detected by identifying an “order parameter,” a quantity that is important and expected to change. For instance, water freezes and transitions to a solid phase (ice) when its temperature drops below 0 degrees Celsius. In this case, an appropriate order parameter could be defined in terms of the proportion of water molecules that are part of the crystalline lattice versus those that remain in a disordered state.
In the past, researchers have relied on physics expertise to build phase diagrams manually, drawing on theoretical understanding to know which order parameters are important. Not only is this tedious for complex systems, and perhaps impossible for unknown systems with new behaviors, but it also introduces human bias into the solution.
More recently, researchers have begun using machine learning to build discriminative classifiers that can solve this task by learning to classify a measurement statistic as coming from a particular phase of the physical system, the same way such models classify an image as a cat or dog.
The MIT researchers demonstrated how generative models can be used to solve this classification task much more efficiently, and in a physics-informed manner.
The Julia Programming Language, a popular language for scientific computing that is also used in MIT’s introductory linear algebra classes, offers many tools that make it invaluable for constructing such generative models, Schäfer adds.
Generative models, like those that underlie ChatGPT and Dall-E, typically work by estimating the probability distribution of some data, which they use to generate new data points that fit the distribution (such as new cat images that are similar to existing cat images).
However, when simulations of a physical system using tried-and-true scientific techniques are available, researchers get a model of its probability distribution for free. This distribution describes the measurement statistics of the physical system.
A more knowledgeable model
The MIT team’s insight is that this probability distribution also defines a generative model upon which a classifier can be constructed. They plug the generative model into standard statistical formulas to directly construct a classifier instead of learning it from samples, as was done with discriminative approaches.
“This is a really nice way of incorporating something you know about your physical system deep inside your machine-learning scheme. It goes far beyond just performing feature engineering on your data samples or simple inductive biases,” Schäfer says.
This generative classifier can determine what phase the system is in given some parameter, like temperature or pressure. And because the researchers directly approximate the probability distributions underlying measurements from the physical system, the classifier has system knowledge.
This enables their method to perform better than other machine-learning techniques. And because it can work automatically without the need for extensive training, their approach significantly enhances the computational efficiency of identifying phase transitions.
At the end of the day, similar to how one might ask ChatGPT to solve a math problem, the researchers can ask the generative classifier questions like “does this sample belong to phase I or phase II?” or “was this sample generated at high temperature or low temperature?”
Scientists could also use this approach to solve different binary classification tasks in physical systems, possibly to detect entanglement in quantum systems (Is the state entangled or not?) or determine whether theory A or B is best suited to solve a particular problem. They could also use this approach to better understand and improve large language models like ChatGPT by identifying how certain parameters should be tuned so the chatbot gives the best outputs.
In the future, the researchers also want to study theoretical guarantees regarding how many measurements they would need to effectively detect phase transitions and estimate the amount of computation that would require.
This work was funded, in part, by the Swiss National Science Foundation, the MIT-Switzerland Lockheed Martin Seed Fund, and MIT International Science and Technology Initiatives.
#ai#approach#artificial#Artificial Intelligence#Bias#binary#change#chatbot#chatGPT#classes#computation#computer#Computer modeling#Computer Science#Computer Science and Artificial Intelligence Laboratory (CSAIL)#Computer science and technology#computing#crystalline#dall-e#data#data-driven#datasets#dog#efficiency#Electrical Engineering&Computer Science (eecs)#engineering#Foundation#framework#Future#generative
2 notes
·
View notes
Text
How Authors Can Use AI to Improve Their Writing Style
Artificial Intelligence (AI) is transforming the way authors approach writing, offering tools to refine style, enhance creativity, and boost productivity. By leveraging AI writing assistant authors can improve their craft in various ways.
1. Grammar and Style Enhancement
AI writing tools like Grammarly, ProWritingAid, and Hemingway Editor help authors refine their prose by correcting grammar, punctuation, and style inconsistencies. These tools offer real-time suggestions to enhance readability, eliminate redundancy, and maintain a consistent tone.
2. Idea Generation and Inspiration
AI can assist in brainstorming and overcoming writer’s block. Platforms like OneAIChat, ChatGPT and Sudowrite provide writing prompts, generate story ideas, and even suggest plot twists. These AI systems analyze existing content and propose creative directions, helping authors develop compelling narratives.
3. Improving Readability and Engagement
AI-driven readability analyzers assess sentence complexity and suggest simpler alternatives. Hemingway Editor, for example, highlights lengthy or passive sentences, making writing more engaging and accessible. This ensures clarity and impact, especially for broader audiences.
4. Personalizing Writing Style
AI-powered tools can analyze an author's writing patterns and provide personalized feedback. They help maintain a consistent voice, ensuring that the writer’s unique style remains intact while refining structure and coherence.
5. Research and Fact-Checking
AI-powered search engines and summarization tools help authors verify facts, gather relevant data, and condense complex information quickly. This is particularly useful for non-fiction writers and journalists who require accuracy and efficiency.
Conclusion
By integrating AI into their writing process, authors can enhance their style, improve efficiency, and foster creativity. While AI should not replace human intuition, it serves as a valuable assistant, enabling writers to produce polished and impactful content effortlessly.
38 notes
·
View notes
Text
one time out of desperation i used chatgpt to try and figure out who the author of a really old short story was (i thought maybe something programmed to process full sentences would have better luck bc i was pretty sure the short title of the story was confusing the keyword search engines) and after giving me 4 wrong answers in a row I asked why it thought the 4th author wrote the short story when there was no evidence linking the two together at all and it basically replied: "you're right!! there is no evidence to suggest this author wrote this short story :)" and when I asked why it would tell me he had anyway it did the auto-fill reply version of "hehe sorry :)"
so anyway in case you still thought ai search engines had any kind of value, they dont. they are not capable of processing and analysising a question. it's not even intelligent enough to say "sorry, insufficient data" if it doesnt have an answer because it doesnt have an articifical intelligence capable of recognizing what real evidence or data is or why it should matter in its response.
#jump on the Hate Ai train now but specifically the “hate autogenerated response” train#because ill say it once and ill say it again - ai doesnt exist. it hasnt been invented yet#chatgpt is just fancy autofill
62 notes
·
View notes