#Data analytics in business
Explore tagged Tumblr posts
Text
The Evolution of PGDM Curriculums: Adapting to Industry Trends and Demands
#Post Graduate Diploma in Management#Business management education#Bangalore business ecosystem#PGDM Specialization#PGDM Courses#Data analytics in business#Soft skills development#Future of PGDM education#Global perspectives in management
1 note
·
View note
Text
New Business Marketing Tips And Tricks for Success
Starting a new business can be an exciting endeavor, but it also comes with its fair share of challenges, especially in the competitive landscape of today's market. Effective marketing is crucial for the success of any new venture. Here are four essential marketing tips and tricks to help your new business thrive.
Define Your Target Audience: Before diving into marketing efforts, it's essential to identify and understand your target audience. Define your ideal customer persona by considering demographics, interests, pain points, and buying behaviors. Conduct market research to gather valuable insights that will guide your marketing strategies. Tailoring your messages and campaigns to resonate with your target audience will significantly increase your chances of success.
Once you have a clear picture of your audience, choose the most suitable marketing channels to reach them effectively. Social media, email marketing, content marketing, and pay-per-click advertising are just a few options to consider. Your choice of channels should align with where your audience spends their time online.
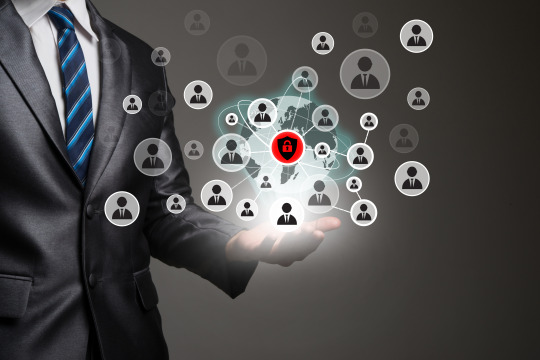
Create Compelling Content: Content marketing is a powerful tool for new businesses to establish their brand and build credibility. Develop high-quality, informative, and engaging content that addresses the needs and interests of your target audience. This content can take various forms, including blog posts, videos, infographics, and podcasts.
Consistency is key when it comes to content creation. Develop a content calendar to plan and schedule regular updates. Providing valuable content not only helps you connect with your audience but also boosts your search engine rankings, making it easier for potential customers to find you.
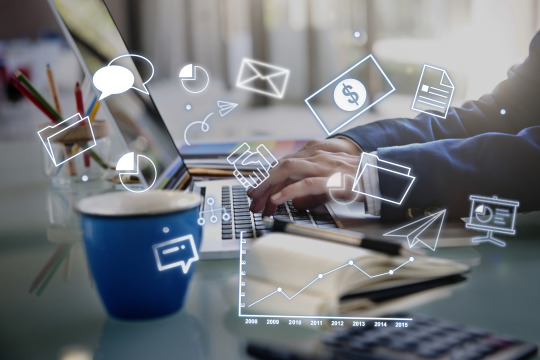
Leverage Social Media: Social media platforms have become indispensable for marketing in today's digital age. Create profiles on relevant social media platforms and engage with your audience regularly. Share your content, interact with followers, and participate in industry-related discussions.
Paid advertising on social media can also be a cost-effective way to reach a broader audience. Platforms like Facebook, Instagram, and LinkedIn offer targeting options that allow you to reach users who match your ideal customer profile.
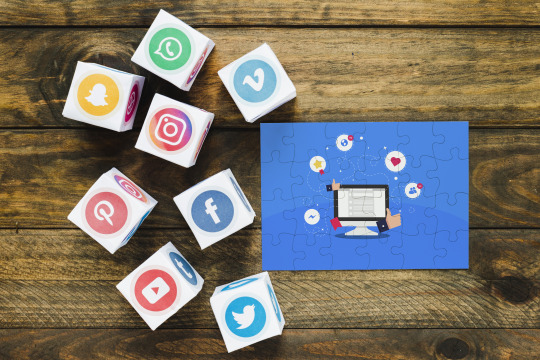
Monitor and Adapt: Marketing is an ever-evolving field, and what works today may not work tomorrow. To stay ahead of the curve, regularly monitor the performance of your marketing efforts. Analyze key metrics such as website traffic, conversion rates, and return on investment (ROI). Use tools like Google Analytics and social media insights to gather data and insights.
Based on your findings, be prepared to adapt your strategies and tactics. If a particular marketing channel isn't delivering the expected results, reallocate your resources to more promising avenues. Stay up-to-date with industry trends and keep an eye on your competitors to ensure your marketing efforts remain relevant and competitive.
In conclusion, effective marketing is essential for the success of any new business. By defining your target audience, creating compelling content, leveraging social media, and continuously monitoring and adapting your strategies, you can position your new business for growth and long-term success in a competitive market. Remember that success may not come overnight, but with persistence and the right marketing approach, your new business can thrive.
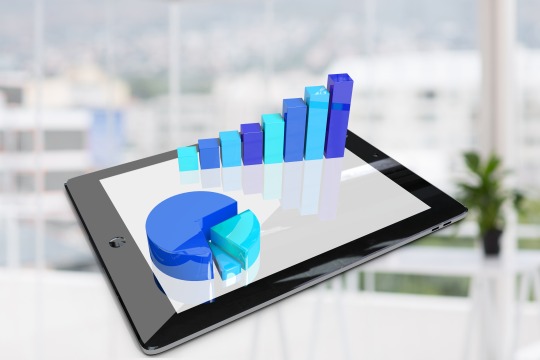
#business#digitalbusiness#digitalmarketing#seo#market analysis#data analytics#marketing#management#sales#learn digital marketing#Digital marketing course#seo expert#business success#businessgrowth
88 notes
·
View notes
Text
AI’s Role in Business Process Automation
Automation has come a long way from simply replacing manual tasks with machines. With AI stepping into the scene, business process automation is no longer just about cutting costs or speeding up workflows—it’s about making smarter, more adaptive decisions that continuously evolve. AI isn't just doing what we tell it; it’s learning, predicting, and innovating in ways that redefine how businesses operate.
From hyperautomation to AI-powered chatbots and intelligent document processing, the world of automation is rapidly expanding. But what does the future hold?
What is Business Process Automation?
Business Process Automation (BPA) refers to the use of technology to streamline and automate repetitive, rule-based tasks within an organization. The goal is to improve efficiency, reduce errors, cut costs, and free up human workers for higher-value activities. BPA covers a wide range of functions, from automating simple data entry tasks to orchestrating complex workflows across multiple departments.
Traditional BPA solutions rely on predefined rules and scripts to automate tasks such as invoicing, payroll processing, customer service inquiries, and supply chain management. However, as businesses deal with increasing amounts of data and more complex decision-making requirements, AI is playing an increasingly critical role in enhancing BPA capabilities.
AI’s Role in Business Process Automation
AI is revolutionizing business process automation by introducing cognitive capabilities that allow systems to learn, adapt, and make intelligent decisions. Unlike traditional automation, which follows a strict set of rules, AI-driven BPA leverages machine learning, natural language processing (NLP), and computer vision to understand patterns, process unstructured data, and provide predictive insights.
Here are some of the key ways AI is enhancing BPA:
Self-Learning Systems: AI-powered BPA can analyze past workflows and optimize them dynamically without human intervention.
Advanced Data Processing: AI-driven tools can extract information from documents, emails, and customer interactions, enabling businesses to process data faster and more accurately.
Predictive Analytics: AI helps businesses forecast trends, detect anomalies, and make proactive decisions based on real-time insights.
Enhanced Customer Interactions: AI-powered chatbots and virtual assistants provide 24/7 support, improving customer service efficiency and satisfaction.
Automation of Complex Workflows: AI enables the automation of multi-step, decision-heavy processes, such as fraud detection, regulatory compliance, and personalized marketing campaigns.
As organizations seek more efficient ways to handle increasing data volumes and complex processes, AI-driven BPA is becoming a strategic priority. The ability of AI to analyze patterns, predict outcomes, and make intelligent decisions is transforming industries such as finance, healthcare, retail, and manufacturing.
“At the leading edge of automation, AI transforms routine workflows into smart, adaptive systems that think ahead. It’s not about merely accelerating tasks—it’s about creating an evolving framework that continuously optimizes operations for future challenges.”
— Emma Reynolds, CTO of QuantumOps
Trends in AI-Driven Business Process Automation
1. Hyperautomation
Hyperautomation, a term coined by Gartner, refers to the combination of AI, robotic process automation (RPA), and other advanced technologies to automate as many business processes as possible. By leveraging AI-powered bots and predictive analytics, companies can automate end-to-end processes, reducing operational costs and improving decision-making.
Hyperautomation enables organizations to move beyond simple task automation to more complex workflows, incorporating AI-driven insights to optimize efficiency continuously. This trend is expected to accelerate as businesses adopt AI-first strategies to stay competitive.
2. AI-Powered Chatbots and Virtual Assistants
Chatbots and virtual assistants are becoming increasingly sophisticated, enabling seamless interactions with customers and employees. AI-driven conversational interfaces are revolutionizing customer service, HR operations, and IT support by providing real-time assistance, answering queries, and resolving issues without human intervention.
The integration of AI with natural language processing (NLP) and sentiment analysis allows chatbots to understand context, emotions, and intent, providing more personalized responses. Future advancements in AI will enhance their capabilities, making them more intuitive and capable of handling complex tasks.
3. Process Mining and AI-Driven Insights
Process mining leverages AI to analyze business workflows, identify bottlenecks, and suggest improvements. By collecting data from enterprise systems, AI can provide actionable insights into process inefficiencies, allowing companies to optimize operations dynamically.
AI-powered process mining tools help businesses understand workflow deviations, uncover hidden inefficiencies, and implement data-driven solutions. This trend is expected to grow as organizations seek more visibility and control over their automated processes.
4. AI and Predictive Analytics for Decision-Making
AI-driven predictive analytics plays a crucial role in business process automation by forecasting trends, detecting anomalies, and making data-backed decisions. Companies are increasingly using AI to analyze customer behaviour, market trends, and operational risks, enabling them to make proactive decisions.
For example, in supply chain management, AI can predict demand fluctuations, optimize inventory levels, and prevent disruptions. In finance, AI-powered fraud detection systems analyze transaction patterns in real-time to prevent fraudulent activities. The future of BPA will heavily rely on AI-driven predictive capabilities to drive smarter business decisions.
5. AI-Enabled Document Processing and Intelligent OCR
Document-heavy industries such as legal, healthcare, and banking are benefiting from AI-powered Optical Character Recognition (OCR) and document processing solutions. AI can extract, classify, and process unstructured data from invoices, contracts, and forms, reducing manual effort and improving accuracy.
Intelligent document processing (IDP) combines AI, machine learning, and NLP to understand the context of documents, automate data entry, and integrate with existing enterprise systems. As AI models continue to improve, document processing automation will become more accurate and efficient.
Going Beyond Automation
The future of AI-driven BPA will go beyond automation—it will redefine how businesses function at their core. Here are some key predictions for the next decade:
Autonomous Decision-Making: AI systems will move beyond assisting human decisions to making autonomous decisions in areas such as finance, supply chain logistics, and healthcare management.
AI-Driven Creativity: AI will not just automate processes but also assist in creative and strategic business decisions, helping companies design products, create marketing strategies, and personalize customer experiences.
Human-AI Collaboration: AI will become an integral part of the workforce, working alongside employees as an intelligent assistant, boosting productivity and innovation.
Decentralized AI Systems: AI will become more distributed, with businesses using edge AI and blockchain-based automation to improve security, efficiency, and transparency in operations.
Industry-Specific AI Solutions: We will see more tailored AI automation solutions designed for specific industries, such as AI-driven legal research tools, medical diagnostics automation, and AI-powered financial advisory services.
AI is no longer a futuristic concept—it’s here, and it’s already transforming the way businesses operate. What’s exciting is that we’re still just scratching the surface. As AI continues to evolve, businesses will find new ways to automate, innovate, and create efficiencies that we can’t yet fully imagine.
But while AI is streamlining processes and making work more efficient, it’s also reshaping what it means to be human in the workplace. As automation takes over repetitive tasks, employees will have more opportunities to focus on creativity, strategy, and problem-solving. The future of AI in business process automation isn’t just about doing things faster—it’s about rethinking how we work all together.
Learn more about DataPeak:
#datapeak#factr#technology#agentic ai#saas#artificial intelligence#machine learning#ai#ai-driven business solutions#machine learning for workflow#ai solutions for data driven decision making#ai business tools#aiinnovation#digitaltools#digital technology#digital trends#dataanalytics#data driven decision making#data analytics#cloudmigration#cloudcomputing#cybersecurity#cloud computing#smbs#chatbots
2 notes
·
View notes
Text
Data-Driven Decision Making improves strategies, boosts efficiency and drives business success with accurate insights and informed choices.
3 notes
·
View notes
Text
Acadecraft Partners with Wadhwani Foundation's Government Digital Transformation Initiative to Develop eLearning Courses
#digitaltransformation#technology#innovation#business#digitalmarketing#ai#digital#artificialintelligence#software#machinelearning#automation#businessgrowth#tech#iot#techinnovation#bigdata#cybersecurity#cloud#data#cloudcomputing#smallbusiness#customerexperience#marketing#sap#webdevelopment#erp#blockchain#analytics#ecommerce#datascience
2 notes
·
View notes
Text
Top 5 DeepSeek AI Features Powering Industry Innovation
Table of Contents1. The Problem: Why Legacy Tools Can’t Keep Up2. What Makes DeepSeek AI Unique?3. 5 Game-Changing DeepSeek AI Features (with Real Stories)3.1 Adaptive Learning Engine3.2 Real-Time Anomaly Detection3.3 Natural Language Reports3.4 Multi-Cloud Sync3.5 Ethical AI Auditor4. How These Features Solve Everyday Challenges5. Step-by-Step: Getting Started with DeepSeek AI6. FAQs: Your…
#affordable AI solutions#AI automation#AI for educators#AI for entrepreneurs#AI for non-techies#AI for small business#AI in manufacturing#AI innovation 2024#AI time management#business growth tools#data-driven decisions#DeepSeek AI Features#ethical AI solutions#healthcare AI tools#no-code AI tools#Predictive Analytics#real-time analytics#remote work AI#retail AI features#startup AI tech
2 notes
·
View notes
Text
French initiative for responsible AI leaders - AI News
New Post has been published on https://thedigitalinsider.com/french-initiative-for-responsible-ai-leaders-ai-news/
French initiative for responsible AI leaders - AI News
ESSEC Business School and Accenture have announced the launch of a new initiative, ‘AI for Responsible Leadership,’ which marks the 10th anniversary of the establishment of the role of Chair at ESSEC, titled the ESSEC Accenture Strategic Business Analytics Chair.
The initiative aims to encourage the use of artificial intelligence by leaders in ways that are responsible and ethical, and that lead to high levels of professional performance. It aims to provide current and future leaders with the skills they require when faced with challenges in the future; economic, environmental, or social.
Several organisations support the initiative, including institutions, businesses, and specialised groups, including ESSEC Metalab for Data, Technology & Society, and Accenture Research.
Executive Director of the ESSEC Metalab, Abdelmounaim Derraz, spoke of the collaboration, saying, “Technical subjects are continuing to shake up business schools, and AI has opened up opportunities for collaboration between partner companies, researchers, and other members of the ecosystem (students, think tanks, associations, [and] public service).”
ESSEC and Accenture aim to integrate perspectives from multiple fields of expertise, an approach that is a result of experimentation in the decade the Chair has existed.
The elements of the initiative include workshops and talks designed to promote the exchange of knowledge and methods. It will also include a ‘barometer’ to help track AI’s implementation and overall impact on responsible leadership.
The initiative will engage with a network of institutions and academic publications, and an annual Grand Prix will recognise projects that focus on and explore the subject of AI and leadership.
Fabrice Marque, founder of the initiative and the current ESSEC Accenture Strategics Business Analytics Chair, said, “For years, we have explored the potential of using data and artificial intelligence in organisations. The synergies we have developed with our partners (Accenture, Accor, Dataiku, Engie, Eurofins, MSD, Orange) allowed us to evaluate and test innovative solutions before deploying them.
“With this initiative, we’re taking a major step: bringing together an engaged ecosystem to sustainably transform how leaders think, decide, and act in the face of tomorrow’s challenges. Our ambition is clear: to make AI a lever for performance, innovation and responsibility for […] leaders.”
Managing Director at Accenture and sponsor of the ESSEC/Accenture Chair and initiative, Aurélien Bouriot, said, “The ecosystem will benefit from the resources that Accenture puts at its disposal, and will also benefit our employees who participate.”
Laetitia Cailleteau, Managing Director at Accenture and leader of Responsible AI & Generative AI for Europe, highlighted the importance of future leaders understanding all aspects of AI.
“AI is a pillar of the ongoing industrial transformation. Tomorrow’s leaders must understand the technical, ethical, and human aspects and risks – and know how to manage them. In this way, they will be able to maximise value creation and generate a positive impact for the organisation, its stakeholders and society as a whole.”
Image credit: Wikimedia Commons
See also: Microsoft and OpenAI probe alleged data theft by DeepSeek
Want to learn more about AI and big data from industry leaders? Check out AI & Big Data Expo taking place in Amsterdam, California, and London. The comprehensive event is co-located with other leading events including Intelligent Automation Conference, BlockX, Digital Transformation Week, and Cyber Security & Cloud Expo.
Explore other upcoming enterprise technology events and webinars powered by TechForge here.
#accenture#ai#ai & big data expo#ai news#amp#Analytics#anniversary#approach#artificial#Artificial Intelligence#automation#Big Data#Business#business analytics#california#Cloud#Collaboration#Companies#comprehensive#conference#cyber#cyber security#data#data theft#deepseek#deploying#Digital Transformation#economic#education#employees
3 notes
·
View notes
Video
youtube
How to use Microsoft Forms
2 notes
·
View notes
Text
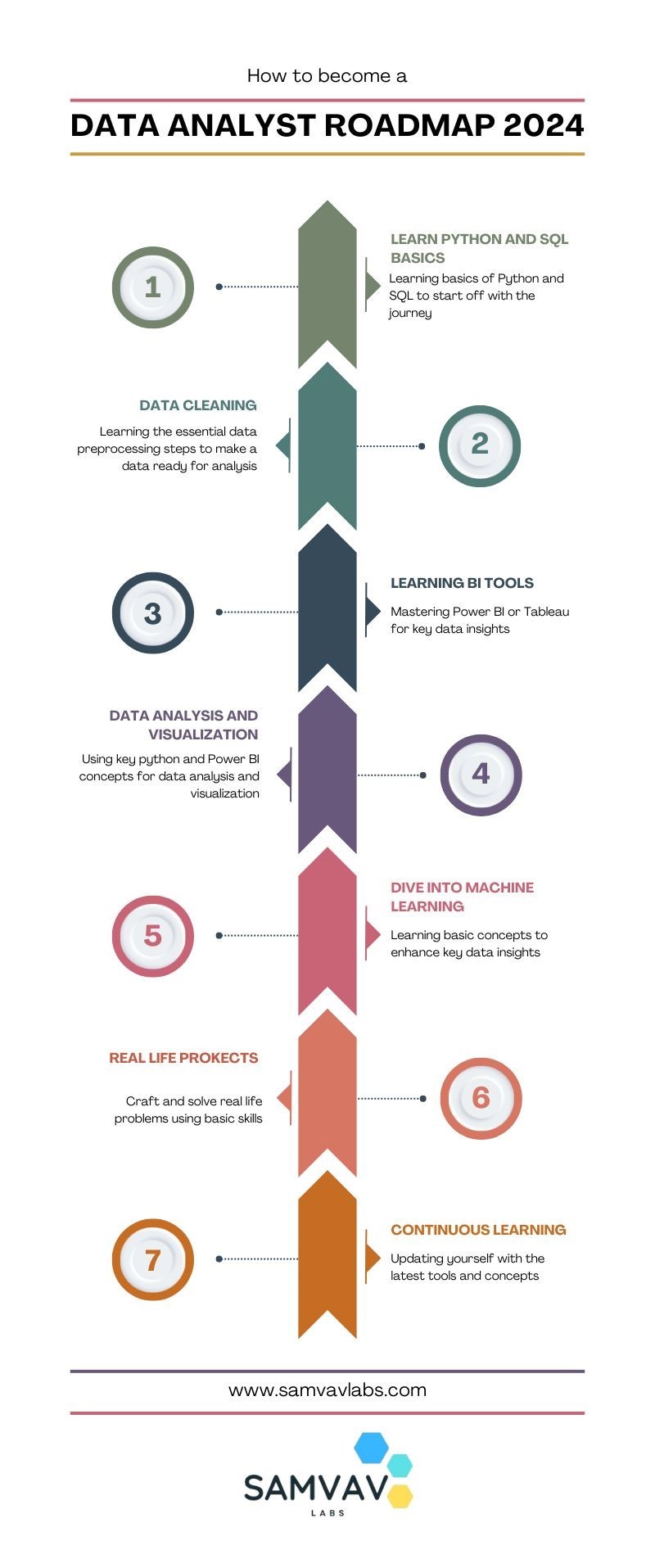
Data Analyst Roadmap for 2024!
Cracking the Data Analyst Roadmap for 2024! Kick off your journey by mastering and delving into Python for data manipulation magic, and dazzle stakeholders with insights using PowerBi or Tableau. Don't forget, that SQL proficiency and hands-on projects refine your skillset, but never overlook the importance of effective communication and problem-solving. Are you checking off these milestones on your path to success? 📌 For more details, visit our website: https://www.samvavlabs.com . . . #DataAnalyst2024 #CareerGrowth #roadmap #DataAnalyst #samvavlabs #roadmap2024 #dataanalystroadmap #datavisualization
#business analytics#data analytics#data analyst#machinelearning#data visualization#datascience#deep learning#data analyst training#dataanalystcourseinKolkata#data analyst certification#data analyst course#data science course#business analyst
9 notes
·
View notes
Text
what u think, to much colour, or less?
https://sdesignt.threadless.com/
#tshirt#animals#design#rainbow#computer#Innovation#AI#Blockchain#Crypto#Tech#Digital#Data#BigData#Automation#Cloud#Cybersecurity#Startup#Entrepreneur#Leadership#Marketing#Business#Ecommerce#Content#Performance#Development#Research#Analytics#Growth#Productivity#Trend
4 notes
·
View notes
Text
Big Data and AI: The Perfect Partnership for Future Innovations
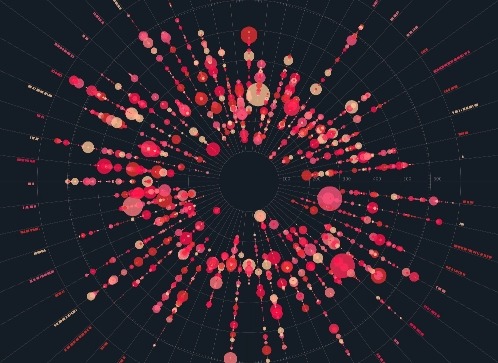
Innovation allows organizations to excel at differentiation, boosting competitive advantages. Amid the growth of industry-disrupting technologies, big data analytics and artificial intelligence (AI) professionals want to support brands seeking bold design, delivery, and functionality ideas. This post discusses the importance of big data and AI, explaining why they matter to future innovations and business development.
Understanding Big Data and AI
Big data is a vast data volume, and you will find mixed data structures because of continuous data collection involving multimedia data objects. A data object or asset can be a document, an audio track, a video clip, a photo, or identical objects with special file formats. Since big data services focus on sorting and exploring data objects’ attributes at an unprecedented scale, integrating AI tools is essential.
Artificial intelligence helps computers simulate human-like thinking and idea synthesis capabilities. Most AI ecosystems leverage advanced statistical methods and machine learning models. Their developers train the AI tools to develop and document high-quality insights by processing unstructured and semi-structured data objects.
As a result, the scope of big data broadens if you add AI integrations that can determine data context. Businesses can generate new ideas instead of recombining recorded data or automatically filter data via AI-assisted quality assurances.
Why Are Big Data and AI Perfect for Future Innovations?
1| They Accelerate Scientific Studies
Material sciences, green technology projects, and rare disorder research projects have provided humans with exceptional lifestyle improvements. However, as markets mature, commoditization becomes inevitable.
At the same time, new, untested ideas can fail, attracting regulators’ dismay, disrespecting consumers’ beliefs, or hurting the environment. Additionally, bold ideas must not alienate consumers due to inherent complexity. Therefore, private sector stakeholders must employ scientific methods to identify feasible, sustainable, and consumer-friendly product ideas for brand differentiation.
AI-powered platforms and business analytics solutions help global corporations immediately acquire, filter, and document data assets for independent research projects. For instance, a pharmaceutical firm can use them during clinical drug formulations and trials, while a car manufacturer might discover efficient production tactics using AI and big data.
2| Brands Can Objectively Evaluate Forward-Thinking Business Ideas
Some business ideas that a few people thought were laughable or unrealistic a few decades ago have forced many brands and professionals to abandon conventional strategies. Consider how streaming platforms’ founders affected theatrical film releases. They have reduced the importance of box office revenues while increasing independent artists’ discoverability.
Likewise, exploring real estate investment opportunities on a tiny mobile or ordering clothes online were bizarre practices, according to many non-believers. They also predicted socializing through virtual reality (VR) avatars inside a computer-generated three-dimensional space would attract only the tech-savvy young adults.
Today, customers and investors who underestimated those innovations prefer religiously studying how disrupting startups perform. Brands care less about losing money than missing an opportunity to be a first mover for a niche consumer base. Similarly, rejecting an idea without testing it at least a few times has become a taboo.
Nobody can be 100% sure which innovation will gain global momentum, but AI and big data might provide relevant hints. These technologies are best for conducting unlimited scenario analyses and testing ideas likely to satisfy tomorrow’s customer expectations.
3| AI-Assisted Insight Explorations Gamifies Idea Synthesis
Combining a few ideas is easy but finding meaningful and profitable ideas by sorting the best ones is daunting. Innovative individuals must embrace AI recommendations to reduce time spent on brainstorming, product repurposing, and multidisciplinary collaborations. Furthermore, they can challenge themselves to find ideas better than an AI tool.
Gamification of brainstorming will facilitate a healthy pursuit of novel product features, marketing strategies, and customer journey personalization. Additionally, incentivizing employees to leverage AI and big data to experiment with designing methods provides unique insights for future innovations.
4| You Can Optimize Supply Chain Components with Big Data and AI Programs
AI can capture extensive data on supply chains and offer suggestions on alternative supplier relations. Therefore, businesses will revise supply and delivery planning to overcome the flaws in current practices.
For instance, Gartner awarded Beijing’s JD.com the Technology Innovation Award in 2024 because they combined statistical forecasting. The awardee has developed an explainable artificial intelligence to enhance its supply chain. Other finalists in this award category were Google, Cisco, MTN Group, and Allina Health.
5| Academia Can Embrace Adaptive Learning and Psychological Well-Being
Communication barriers and trying to force all learners to follow the standard course material based on a fixed schedule have undermined educational institutions’ goals worldwide. Understandably, expecting teachers to customize courses and multimedia assets for each student is impractical and humanly infeasible.
As a result, investors, policymakers, parents, and student bodies seek outcome-oriented educational innovations powered by AI and big data for a learner-friendly, inclusive future. For instance, some edtech providers use AI computer-aided learning and teaching ecosystems leveraging videoconferencing, curriculum personalization, and psycho-cognitive support.
Adaptive learning applications build student profiles and segments like marketers’ consumer categorizations. Their AI integrations can determine the ideal pace for teaching, whether a student exhibits learning disabilities, and whether a college or school has adequate resources.
Challenges in Promoting Innovations Based on Big Data and AI Use Cases
Encouraging stakeholders to acknowledge the need for big data and AI might be challenging. After all, uninformed stakeholders are likely to distrust tech-enabled lifestyle changes. Therefore, increasing AI awareness and educating everyone on data ethics are essential.
In some regions, the IT or network infrastructure necessary for big data is unavailable or prone to stability flaws. This issue requires more investments and talented data specialists to leverage AI tools or conduct predictive analyses.
Today’s legal frameworks lack provisions for regulating AI, big data, and scenario analytics. So, brands are unsure whether expanding data scope will get public administrators’ approvals. Lawmakers must find a balanced approach to enable AI-powered big data innovations without neglecting consumer rights or “privacy by design” principles.
Conclusion
The future of enterprise, institutional, and policy innovations lies in responsible technology implementations. Despite the obstacles, AI enthusiasts are optimistic that more stakeholders will admire the potential of new, disruptive technologies.
Remember, gamifying how your team finds new ideas or predicting the actual potential of a business model necessitates AI’s predictive insights. At the same time, big data will offer broader perspectives on global supply chains and how to optimize a company’s policies.
Lastly, academic improvements and scientific research are integral to developing sustainable products, accomplishing educational objectives, and responding to global crises. As a result, the informed stakeholders agree that AI and big data are perfect for shaping future innovations.
2 notes
·
View notes
Text
#artificial intelligence#business#data analytics#datascience#power bi#sql#it services#information technology
4 notes
·
View notes
Text
youtube
It's Over - Gino vs Harvard Fake Data Scandal
#youtube#gino#fake#fake data#harvard#scandal#pete judo#francesca gino#professor#data science#rebel talent#lost#manipulation#data#data analytics#university#paper#harvard business school#crime#guilty#harvardbusinessschool#hbs#data colada#plagiarism
2 notes
·
View notes
Text
How Large Language Models (LLMs) are Transforming Data Cleaning in 2024
Data is the new oil, and just like crude oil, it needs refining before it can be utilized effectively. Data cleaning, a crucial part of data preprocessing, is one of the most time-consuming and tedious tasks in data analytics. With the advent of Artificial Intelligence, particularly Large Language Models (LLMs), the landscape of data cleaning has started to shift dramatically. This blog delves into how LLMs are revolutionizing data cleaning in 2024 and what this means for businesses and data scientists.
The Growing Importance of Data Cleaning
Data cleaning involves identifying and rectifying errors, missing values, outliers, duplicates, and inconsistencies within datasets to ensure that data is accurate and usable. This step can take up to 80% of a data scientist's time. Inaccurate data can lead to flawed analysis, costing businesses both time and money. Hence, automating the data cleaning process without compromising data quality is essential. This is where LLMs come into play.
What are Large Language Models (LLMs)?
LLMs, like OpenAI's GPT-4 and Google's BERT, are deep learning models that have been trained on vast amounts of text data. These models are capable of understanding and generating human-like text, answering complex queries, and even writing code. With millions (sometimes billions) of parameters, LLMs can capture context, semantics, and nuances from data, making them ideal candidates for tasks beyond text generation—such as data cleaning.
To see how LLMs are also transforming other domains, like Business Intelligence (BI) and Analytics, check out our blog How LLMs are Transforming Business Intelligence (BI) and Analytics.

Traditional Data Cleaning Methods vs. LLM-Driven Approaches
Traditionally, data cleaning has relied heavily on rule-based systems and manual intervention. Common methods include:
Handling missing values: Methods like mean imputation or simply removing rows with missing data are used.
Detecting outliers: Outliers are identified using statistical methods, such as standard deviation or the Interquartile Range (IQR).
Deduplication: Exact or fuzzy matching algorithms identify and remove duplicates in datasets.
However, these traditional approaches come with significant limitations. For instance, rule-based systems often fail when dealing with unstructured data or context-specific errors. They also require constant updates to account for new data patterns.
LLM-driven approaches offer a more dynamic, context-aware solution to these problems.

How LLMs are Transforming Data Cleaning
1. Understanding Contextual Data Anomalies
LLMs excel in natural language understanding, which allows them to detect context-specific anomalies that rule-based systems might overlook. For example, an LLM can be trained to recognize that “N/A” in a field might mean "Not Available" in some contexts and "Not Applicable" in others. This contextual awareness ensures that data anomalies are corrected more accurately.
2. Data Imputation Using Natural Language Understanding
Missing data is one of the most common issues in data cleaning. LLMs, thanks to their vast training on text data, can fill in missing data points intelligently. For example, if a dataset contains customer reviews with missing ratings, an LLM could predict the likely rating based on the review's sentiment and content.
A recent study conducted by researchers at MIT (2023) demonstrated that LLMs could improve imputation accuracy by up to 30% compared to traditional statistical methods. These models were trained to understand patterns in missing data and generate contextually accurate predictions, which proved to be especially useful in cases where human oversight was traditionally required.
3. Automating Deduplication and Data Normalization
LLMs can handle text-based duplication much more effectively than traditional fuzzy matching algorithms. Since these models understand the nuances of language, they can identify duplicate entries even when the text is not an exact match. For example, consider two entries: "Apple Inc." and "Apple Incorporated." Traditional algorithms might not catch this as a duplicate, but an LLM can easily detect that both refer to the same entity.
Similarly, data normalization—ensuring that data is formatted uniformly across a dataset—can be automated with LLMs. These models can normalize everything from addresses to company names based on their understanding of common patterns and formats.
4. Handling Unstructured Data
One of the greatest strengths of LLMs is their ability to work with unstructured data, which is often neglected in traditional data cleaning processes. While rule-based systems struggle to clean unstructured text, such as customer feedback or social media comments, LLMs excel in this domain. For instance, they can classify, summarize, and extract insights from large volumes of unstructured text, converting it into a more analyzable format.
For businesses dealing with social media data, LLMs can be used to clean and organize comments by detecting sentiment, identifying spam or irrelevant information, and removing outliers from the dataset. This is an area where LLMs offer significant advantages over traditional data cleaning methods.
For those interested in leveraging both LLMs and DevOps for data cleaning, see our blog Leveraging LLMs and DevOps for Effective Data Cleaning: A Modern Approach.

Real-World Applications
1. Healthcare Sector
Data quality in healthcare is critical for effective treatment, patient safety, and research. LLMs have proven useful in cleaning messy medical data such as patient records, diagnostic reports, and treatment plans. For example, the use of LLMs has enabled hospitals to automate the cleaning of Electronic Health Records (EHRs) by understanding the medical context of missing or inconsistent information.
2. Financial Services
Financial institutions deal with massive datasets, ranging from customer transactions to market data. In the past, cleaning this data required extensive manual work and rule-based algorithms that often missed nuances. LLMs can assist in identifying fraudulent transactions, cleaning duplicate financial records, and even predicting market movements by analyzing unstructured market reports or news articles.
3. E-commerce
In e-commerce, product listings often contain inconsistent data due to manual entry or differing data formats across platforms. LLMs are helping e-commerce giants like Amazon clean and standardize product data more efficiently by detecting duplicates and filling in missing information based on customer reviews or product descriptions.

Challenges and Limitations
While LLMs have shown significant potential in data cleaning, they are not without challenges.
Training Data Quality: The effectiveness of an LLM depends on the quality of the data it was trained on. Poorly trained models might perpetuate errors in data cleaning.
Resource-Intensive: LLMs require substantial computational resources to function, which can be a limitation for small to medium-sized enterprises.
Data Privacy: Since LLMs are often cloud-based, using them to clean sensitive datasets, such as financial or healthcare data, raises concerns about data privacy and security.

The Future of Data Cleaning with LLMs
The advancements in LLMs represent a paradigm shift in how data cleaning will be conducted moving forward. As these models become more efficient and accessible, businesses will increasingly rely on them to automate data preprocessing tasks. We can expect further improvements in imputation techniques, anomaly detection, and the handling of unstructured data, all driven by the power of LLMs.
By integrating LLMs into data pipelines, organizations can not only save time but also improve the accuracy and reliability of their data, resulting in more informed decision-making and enhanced business outcomes. As we move further into 2024, the role of LLMs in data cleaning is set to expand, making this an exciting space to watch.
Large Language Models are poised to revolutionize the field of data cleaning by automating and enhancing key processes. Their ability to understand context, handle unstructured data, and perform intelligent imputation offers a glimpse into the future of data preprocessing. While challenges remain, the potential benefits of LLMs in transforming data cleaning processes are undeniable, and businesses that harness this technology are likely to gain a competitive edge in the era of big data.
#Artificial Intelligence#Machine Learning#Data Preprocessing#Data Quality#Natural Language Processing#Business Intelligence#Data Analytics#automation#datascience#datacleaning#large language model#ai
2 notes
·
View notes
Text
#Finance#Business#Work Meme#Work Humor#Excel#Hilarious#funny meme#funny#accounting#office humor#consulting#big data#data analysis#data visualization#data analytics#data#dashboard commentary#tableau#power bi
13 notes
·
View notes
Text
Qatar Partners With Scale AI for AI-Powered Digital Transformation of Government Services
#digitaltransformation#technology#innovation#business#digitalmarketing#ai#digital#artificialintelligence#software#machinelearning#automation#businessgrowth#tech#iot#techinnovation#bigdata#cybersecurity#cloud#data#cloudcomputing#smallbusiness#customerexperience#marketing#sap#webdevelopment#erp#blockchain#analytics#ecommerce#datascience
2 notes
·
View notes