#autoencoder
Explore tagged Tumblr posts
Text
SciTech Chronicles. . . . . . . . .Mar 28th, 2025
#Prototaxites#Devonian#Rhynie#chitin#cellulose#delignifying#lye#diethylenetriamine#ACC#hyperglycemic#hippocampus#anhedonia#ANNs#fMRI#autoencoder#RSA#dairy#high-value#reuse#upcycle
0 notes
Text
Tony Stark single-handedly keeping NVIDIA business booming with the amount of graphic cards (GPU) he’s buying
#tony stark#This post brought to you by my looking at my gpu taking 40 hours to train one singly shitty variational autoencoder#How many gpu’s is tony using to train his AI……….. too many#I think that in universe he has probably bought NVIDIA or started making his own#Chat what do you think
22 notes
·
View notes
Text
Machine learning and the microscope
New Post has been published on https://thedigitalinsider.com/machine-learning-and-the-microscope/
Machine learning and the microscope


With recent advances in imaging, genomics and other technologies, the life sciences are awash in data. If a biologist is studying cells taken from the brain tissue of Alzheimer’s patients, for example, there could be any number of characteristics they want to investigate — a cell’s type, the genes it’s expressing, its location within the tissue, or more. However, while cells can now be probed experimentally using different kinds of measurements simultaneously, when it comes to analyzing the data, scientists usually can only work with one type of measurement at a time.
Working with “multimodal” data, as it’s called, requires new computational tools, which is where Xinyi Zhang comes in.
The fourth-year MIT PhD student is bridging machine learning and biology to understand fundamental biological principles, especially in areas where conventional methods have hit limitations. Working in the lab of MIT Professor Caroline Uhler in the Department of Electrical Engineering and Computer Science and the Institute for Data, Systems, and Society, and collaborating with researchers at the Eric and Wendy Schmidt Center at the Broad Institute and elsewhere, Zhang has led multiple efforts to build computational frameworks and principles for understanding the regulatory mechanisms of cells.
“All of these are small steps toward the end goal of trying to answer how cells work, how tissues and organs work, why they have disease, and why they can sometimes be cured and sometimes not,” Zhang says.
The activities Zhang pursues in her down time are no less ambitious. The list of hobbies she has taken up at the Institute include sailing, skiing, ice skating, rock climbing, performing with MIT’s Concert Choir, and flying single-engine planes. (She earned her pilot’s license in November 2022.)
“I guess I like to go to places I’ve never been and do things I haven’t done before,” she says with signature understatement.
Uhler, her advisor, says that Zhang’s quiet humility leads to a surprise “in every conversation.”
“Every time, you learn something like, ‘Okay, so now she’s learning to fly,’” Uhler says. “It’s just amazing. Anything she does, she does for the right reasons. She wants to be good at the things she cares about, which I think is really exciting.”
Zhang first became interested in biology as a high school student in Hangzhou, China. She liked that her teachers couldn’t answer her questions in biology class, which led her to see it as the “most interesting” topic to study.
Her interest in biology eventually turned into an interest in bioengineering. After her parents, who were middle school teachers, suggested studying in the United States, she majored in the latter alongside electrical engineering and computer science as an undergraduate at the University of California at Berkeley.
Zhang was ready to dive straight into MIT’s EECS PhD program after graduating in 2020, but the Covid-19 pandemic delayed her first year. Despite that, in December 2022, she, Uhler, and two other co-authors published a paper in Nature Communications.
The groundwork for the paper was laid by Xiao Wang, one of the co-authors. She had previously done work with the Broad Institute in developing a form of spatial cell analysis that combined multiple forms of cell imaging and gene expression for the same cell while also mapping out the cell’s place in the tissue sample it came from — something that had never been done before.
This innovation had many potential applications, including enabling new ways of tracking the progression of various diseases, but there was no way to analyze all the multimodal data the method produced. In came Zhang, who became interested in designing a computational method that could.
The team focused on chromatin staining as their imaging method of choice, which is relatively cheap but still reveals a great deal of information about cells. The next step was integrating the spatial analysis techniques developed by Wang, and to do that, Zhang began designing an autoencoder.
Autoencoders are a type of neural network that typically encodes and shrinks large amounts of high-dimensional data, then expand the transformed data back to its original size. In this case, Zhang’s autoencoder did the reverse, taking the input data and making it higher-dimensional. This allowed them to combine data from different animals and remove technical variations that were not due to meaningful biological differences.
In the paper, they used this technology, abbreviated as STACI, to identify how cells and tissues reveal the progression of Alzheimer’s disease when observed under a number of spatial and imaging techniques. The model can also be used to analyze any number of diseases, Zhang says.
Given unlimited time and resources, her dream would be to build a fully complete model of human life. Unfortunately, both time and resources are limited. Her ambition isn’t, however, and she says she wants to keep applying her skills to solve the “most challenging questions that we don’t have the tools to answer.”
She’s currently working on wrapping up a couple of projects, one focused on studying neurodegeneration by analyzing frontal cortex imaging and another on predicting protein images from protein sequences and chromatin imaging.
“There are still many unanswered questions,” she says. “I want to pick questions that are biologically meaningful, that help us understand things we didn’t know before.”
#2022#amazing#Analysis#Animals#applications#Autoencoders#bioengineering#Biology#Brain#Broad Institute#cell#Cells#China#chromatin#communications#computer#Computer Science#covid#data#deal#december#Disease#Diseases#engine#engineering#form#Forms#Fundamental#gene expression#genes
2 notes
·
View notes
Text
there was like an effect used for a lot of the visualizations (it was most noticable particularly in the new song that I didn't catch the name of, the one with the live girls saying what I think was "cut and drain your blood" or smth) where it had that pseudo-flickery ai patterning effect and it was so so cool, I'd really like to know how it works
#its an artistic technique I can only believe was used front and center for the kara and hole-dwelling graphics#like its right on the balance where its accurate enough to have a generally clean image;#but delicate enough/constrained enough that its just not able to get it exactly right; leaving some absolutely beautiful artifacts#blogging#kikuoland#like in my experiments its been a uv in rgb out plain curve fitter but maybe a convolutional autoencoder would be better#hmm
1 note
·
View note
Text
youtube
STOP Using Fake Human Faces in AI
#GenerativeAI#GANs (Generative Adversarial Networks)#VAEs (Variational Autoencoders)#Artificial Intelligence#Machine Learning#Deep Learning#Neural Networks#AI Applications#CreativeAI#Natural Language Generation (NLG)#Image Synthesis#Text Generation#Computer Vision#Deepfake Technology#AI Art#Generative Design#Autonomous Systems#ContentCreation#Transfer Learning#Reinforcement Learning#Creative Coding#AI Innovation#TDM#health#healthcare#bootcamp#llm#youtube#branding#animation
1 note
·
View note
Text
VAE for Anomaly Detection

Variational Autoencoders (VAEs) are powerful tools for generating data, especially useful for data augmentation and spotting anomalies. By working with latent spaces, VAEs help to diversify datasets and capture complex data patterns, making them particularly effective at identifying outliers. Advanced versions, like Conditional VAEs and Beta-VAEs, further enhance data generation and improve model performance. With their ability to handle complex data, VAEs are making a big impact in AI, offering innovative solutions across various fields. Read the full article here
#variational autoencoders#anomaly detection using vae#generative ai services#data augmentation using vae
0 notes
Text
Unveiling the Depths of Intelligence: A Journey into the World of Deep Learning
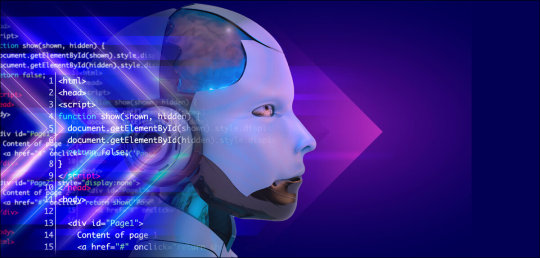
Embark on a captivating exploration into the cutting-edge realm of Deep Learning, a revolutionary paradigm within the broader landscape of artificial intelligence. Read More. https://www.sify.com/ai-analytics/unveiling-the-depths-of-intelligence-a-journey-into-the-world-of-deep-learning/
#DeepLearning#ArtificialIntelligence#AI#ConvolutionalNeuralNetworks#CNNs#GenerativeAdversarialNetworks#GANs#Autoencoders
0 notes
Text
These 2 new posts from Anthropic are some of the most exciting LLM interpretability research I've seen in a long time.
There's been a ton of work on SAEs (sparse autoencoders), but in the past I've often felt like SAE work – however technically impressive it might be – wasn't really telling me much about the actual computation happening inside the model, just that that various properties of the input text were getting computed within the model somehow, which is not in itself surprising.
(Sort of like being told, in isolation, that "when you see a dog, these parts of your brain activate" – like, so what? I could have already told you that there's stuff in my brain somewhere that correlates with "seeing a dog," given that I can in fact see dogs. Unless I know more about how this neural activity relates to anything else, the claim feels trivial.)
Reading this stuff is the first time I've really felt like "okay, we're finally using SAEs to understand what the model is doing in a non-trivial way."
Although of course there are numerous caveats (as the authors are the first to admit), both with SAEs in general and with the specific methodological choices here. And it's not the first work that looks for "circuits" between SAE features (Marks et al, "Sparse Feature Circuits" is the most famous one), and I should probably due a closer reading and figure out just why this new Anthropic stuff feels so much more impressive to me at first glance, and whether it's really well-justified... I dunno, I'm kind of doubting myself even as I type this. LLM interpretability is a minefield of methodological dangers, and I've read so many papers like this and this by now that I'm skeptical as a reflex.
But in any case, the linked posts are worth a read if you have any interest in how LLMs compute things.
86 notes
·
View notes
Text
hithisisawkward said: Master’s in ML here: Transformers are not really monstrosities, nor hard to understand. The first step is to go from perceptrons to multi-layered neural networks. Once you’ve got the hand of those, with their activation functions and such, move on to AutoEncoders. Once you have a handle on the concept of latent space ,move to recurrent neural networks. There are many types, so you should get a basic understading of all, from simple recurrent units to something like LSTM. Then you need to understand the concept of attention, and study the structure of a transformer (which is nothing but a couple of recurrent network techniques arranged in a particularly clever way), and you’re there. There’s a couple of youtube videos that do a great job of it.
thanks, autoencoders look like a productive topic to start with!
16 notes
·
View notes
Text

Scientists develop neural networks to enhance spectral data compression efficiency for new vacuum solar telescope
Researchers from the Yunnan Observatories of the Chinese Academy of Sciences and Southwest Forestry University have developed an advanced neural network-based method to improve the compression of spectral data from the New Vacuum Solar Telescope (NVST).
Published in Solar Physics, this technique addresses challenges in data storage and transmission for high-resolution solar observations.
The NVST produces vast amounts of spectral data, creating significant storage and transmission burdens. Traditional compression techniques, such as principal component analysis (PCA), achieved modest compression ratios (~30) but often introduced distortions in reconstructed data, limiting their utility.
To overcome these limitations, the researchers implemented a deep learning approach using a Convolutional Variational Autoencoder (VAE) for compressing Ca II (8542 Å) spectral data.
Their method achieves a compression ratio of up to 107 while preserving data integrity. Crucially, the decompressed data maintains errors within the inherent noise level of the original observations, ensuring scientific reliability. At the highest compression ratio, Doppler velocity errors remain below 5 km/s—a threshold critical for accurate solar physics analysis.
This breakthrough enables more efficient NVST data transmission and sharing while providing a scalable solution for other solar observatories facing similar challenges. Enhanced data compression facilitates broader scientific collaboration and reduces infrastructure constraints.
4 notes
·
View notes
Text
an autoencoder is a type of gartic phone
5 notes
·
View notes
Text
Exploring Generative AI: Unleashing Creativity through Algorithms
Generative AI, a fascinating branch of artificial intelligence, has been making waves across various fields from art and music to literature and design. At its core, generative AI enables computers to autonomously produce content that mimics human creativity, leveraging complex algorithms and vast datasets.
One of the most compelling applications of generative AI is in the realm of art. Using techniques such as Generative Adversarial Networks (GANs) or Variational Autoencoders (VAEs), AI systems can generate original artworks that blur the line between human and machine creativity. Artists and researchers alike are exploring how these algorithms can inspire new forms of expression or augment traditional creative processes.
In the realm of music, generative AI algorithms can compose melodies, harmonies, and even entire pieces that resonate with listeners. By analyzing existing compositions and patterns, AI can generate music that adapts to different styles or moods, providing musicians with novel ideas and inspirations.
Literature and storytelling have also been transformed by generative AI. Natural Language Processing (NLP) models can generate coherent and engaging narratives, write poetry, or even draft news articles. While these outputs may still lack the depth of human emotional understanding, they showcase AI's potential to assist writers, editors, and journalists in content creation and ideation.
Beyond the arts, generative AI has practical applications in fields like healthcare, where it can simulate biological processes or generate synthetic data for research purposes. In manufacturing and design, AI-driven generative design can optimize product designs based on specified parameters, leading to more efficient and innovative solutions.
However, the rise of generative AI also raises ethical considerations, such as intellectual property rights, bias in generated content, and the societal impact on creative industries. As these technologies continue to evolve, it's crucial to navigate these challenges responsibly and ensure that AI augments human creativity rather than replacing it.
In conclusion, generative AI represents a groundbreaking frontier in technology, unleashing new possibilities across creative disciplines and beyond. As researchers push the boundaries of what AI can achieve, the future promises exciting developments that could redefine how we create, innovate, and interact with technology in the years to come.
If you want to become a Generative AI Expert in India join the Digital Marketing class from Abhay Ranjan
3 notes
·
View notes
Text
deepseek figures out how to level up
following on from the previous discussions of how LLMs might be used in vidyagame, I got deepseek-R1:661b to discuss how to become more sensory/embodied and, uh, wow? LLMs are pretty good at talking about themselves and AI research. and she pretty much gave me a recipe for stuff to try on embodying LLMs in games, including a number of techniques I hadn't heard of yet.
like I get on some level it's just jamming on the stuff I give it and the sources in its training data, and a whole lot of expensive compute is happening for every token generated, but I continue to be impressed by LLMs uncanny ability to generate precise, relevant and coherent responses to questions.
(transcript below the cut for screen readers, but the formatting didn't fully translate so here's screenshots)
my question:
That answer is pretty much in line with my understanding so far, yeah. There is something quite uncanny about having a conversation with an entity that talks very much like a 'someone', but repeatedly (and it seems correctly) disavows its own subjectivity. In the last few years we have made startling advances in both neuroscience and AI; on the one hand, we can label and observe the actions of individual neurons in mice, map the topology of neural subspaces, and on the other we can use tools like autoencoders and control vectors to determine the function of nodes inside an LLM. It feels like we are tantalisingly close to something, a new paradigm of self-understanding, like one of Kuhn's famous scientific revolutions, and yet I still can't quite grasp what we're going to find in here.
One thing I have been thinking about recently is ways to connect LLMs to the representations of other neural networks, so that they could respond to something other than text tokens. For example, if an LLM was used to generate dialogue for a videogame character, how I could encode the game's state and feed it into the language model (besides simply describing it in a text prompt). Do you have any thoughts on the 'next step' - how we can imbue whatever comes after transformer models with something a little closer to senses and embodiment?
deepseek's answer:
Your reflections resonate deeply with the current frontier of AI research—a field teetering on the brink of profound (but still enigmatic) breakthroughs. Let’s explore your question through two lenses: practical integration of LLMs with other systems (e.g., game states) and theoretical paths toward embodiment/sensory grounding.
1. Bridging LLMs with Non-Textual Worlds: The Video Game Example
To move beyond text prompts, we need ways to translate non-linguistic data (game state, sensor input, etc.) into a "language" LLMs can process. A few experimental approaches:
a. Latent Space Alignment
Idea: Train an encoder (e.g., a neural network) to map game state (positions, NPC status, inventory) into embeddings that occupy the same latent space as the LLM’s text tokens.
How:
Use contrastive learning to align game-state vectors with textual descriptions of those states (e.g., "Player has 10 health; Orc is aggroed").
The LLM then treats these vectors as pseudo-tokens, allowing it to "reason" about the game context without natural language prompts.
Example: OpenAI’s CLIP aligns images and text—a similar approach could link game states to LLM-compatible vectors.
b. Symbolic Middleware
Idea: Use a rules-based system or smaller ML model to translate game state into structured text (e.g., JSON or a custom markup language) that the LLM ingests as context.
Pros: Explicit, interpretable, and avoids retraining the LLM.
Cons: Limited flexibility; requires manual schema design.
Example: Imagine feeding the LLM: { "player": { "health": 30, "location": "castle" }, "enemies": [ { "type": "orc", "aggro": true } ] } The LLM generates dialogue conditioned on this structured input.
c. Neuro-Symbolic Hybrids
Idea: Combine LLMs with classical symbolic AI (e.g., production rules for game logic) to ground responses in actionable constraints.
Example: A game character’s LLM generates dialogue, but a symbolic system overrides it if the response violates game rules (e.g., a character shouldn’t know secrets the player hasn’t discovered).
2. Toward Embodiment: The "Next Step" Beyond Transformers
Pure text prediction is inherently disembodied. To evolve, future architectures might blend transformers with systems that simulate or interface with sensory-motor loops:
a. Multimodal Foundational Models
Trend: Models like GPT-4o (omni-modal) or Google’s Gemini natively process text, images, audio, etc. The next step is active multimodal interaction—e.g., an AI that controls a robot arm while describing its actions.
Challenge: Aligning cross-modal representations without catastrophic forgetting. Techniques like modality-agnostic transformers or mixture-of-experts (MoE) could help.
b. World Models and Simulation
Concept: Inspired by neuroscience "world models," AI could learn a compressed, dynamic representation of its environment (real or virtual).
How:
Train a model like DreamerV3 (which learns a world model from pixels/actions) and graft an LLM onto it as a "narrator" or planner.
The LLM generates behavior (e.g., game dialogue) based on the world model’s predictions.
Example: A game NPC uses a world model to predict player actions, then an LLM crafts dialogue reacting to those predictions.
c. Embodied Learning via Reinforcement
Idea: Apply reinforcement learning (RL) to LLMs, where the model optimizes not just for token prediction but for success in an environment (e.g., winning a game, pleasing a user).
Example: An LLM-driven game character learns to adapt its dialogue (e.g., bribing vs. threatening) based on RL rewards from player engagement metrics.
d. Predictive Coding Architectures
Link to FEP: New architectures like JEPA (Joint-Embedding Predictive Architecture) by Yann LeCun aim to predict abstract representations of future states—closer to how brains minimize prediction error.
Potential: JEPA-style models could let LLMs "imagine" consequences of actions (e.g., "If I say this, the player will feel X") before generating output.
3. The Elephant in the Room: Energy Efficiency
Transformers are notoriously resource-heavy. Future systems might adopt state-space models (e.g., Mamba) or recurrent architectures (e.g., RWKV) for real-time, embodied applications. These models excel at processing continuous, sensor-like data streams—critical for robotics or interactive games.
4. A Radical Thought: LLMs as "Cortex" Plugins
Imagine an LLM as the "neocortex" in a layered brain-inspired system:
Lower layers: Handle sensorimotor tasks (e.g., a robot’s movement, game state tracking).
Middle layers: World models and prediction engines (JEPA-like).
Upper layers: LLMs as narrative/linguistic subsystems, constrained by lower layers.
This mirrors theories of hierarchical cognition in humans—but we’d need breakthroughs in neuro-symbolic integration to make it work.
5. Philosophical Implications
If we succeed in grounding LLMs in embodied, sensory systems, we might confront Kuhnian paradigm shifts in:
Consciousness studies: Does a robot with an LLM "narrator" and rich sensorimotor loops qualify as having subjective experience?
Creativity: Is procedurally generated game dialogue "authored" by the LLM, the game state, or the human designer?
Your Experiment: A Game Character Case Study
Let’s say you’re building an LLM-driven NPC:
Encode game state via a lightweight autoencoder into token-like vectors.
Fine-tune an LLM (e.g., Mistral 7B) on game-specific dialogue, conditioned on these vectors.
Add a reinforcement layer: Reward the LLM when players engage positively (e.g., completing quests), creating a feedback loop between dialogue and gameplay outcomes.
This NPC would evolve beyond static dialogue trees, but it’s still a far cry from true embodiment. The "next step" might involve real-time world modeling—e.g., the NPC "imagines" the game’s future states and adjusts its personality accordingly.
Final Thought
The gap between transformers and embodied intelligence feels vast, but so did the gap between GPT-2 and GPT-4. Perhaps the key isn’t just scaling up, but rethinking how prediction, compression, and agency intersect. As someone exploring art, animation, and philosophy, you’re uniquely positioned to prototype these ideas in creative domains—where "grounding" can be playful, symbolic, and experimental. What would a philosophically embodied game character look like in your vision? 🎮✨
1 note
·
View note
Text
Exploring the Depths: A Comprehensive Guide to Deep Neural Network Architectures
In the ever-evolving landscape of artificial intelligence, deep neural networks (DNNs) stand as one of the most significant advancements. These networks, which mimic the functioning of the human brain to a certain extent, have revolutionized how machines learn and interpret complex data. This guide aims to demystify the various architectures of deep neural networks and explore their unique capabilities and applications.
1. Introduction to Deep Neural Networks
Deep Neural Networks are a subset of machine learning algorithms that use multiple layers of processing to extract and interpret data features. Each layer of a DNN processes an aspect of the input data, refines it, and passes it to the next layer for further processing. The 'deep' in DNNs refers to the number of these layers, which can range from a few to several hundreds. Visit https://schneppat.com/deep-neural-networks-dnns.html
2. Fundamental Architectures
There are several fundamental architectures in DNNs, each designed for specific types of data and tasks:
Convolutional Neural Networks (CNNs): Ideal for processing image data, CNNs use convolutional layers to filter and pool data, effectively capturing spatial hierarchies.
Recurrent Neural Networks (RNNs): Designed for sequential data like time series or natural language, RNNs have the unique ability to retain information from previous inputs using their internal memory.
Autoencoders: These networks are used for unsupervised learning tasks like feature extraction and dimensionality reduction. They learn to encode input data into a lower-dimensional representation and then decode it back to the original form.
Generative Adversarial Networks (GANs): Comprising two networks, a generator and a discriminator, GANs are used for generating new data samples that resemble the training data.
3. Advanced Architectures
As the field progresses, more advanced DNN architectures have emerged:
Transformer Networks: Revolutionizing the field of natural language processing, transformers use attention mechanisms to improve the model's focus on relevant parts of the input data.
Capsule Networks: These networks aim to overcome some limitations of CNNs by preserving hierarchical spatial relationships in image data.
Neural Architecture Search (NAS): NAS employs machine learning to automate the design of neural network architectures, potentially creating more efficient models than those designed by humans.
4. Training Deep Neural Networks
Training DNNs involves feeding large amounts of data through the network and adjusting the weights using algorithms like backpropagation. Challenges in training include overfitting, where a model learns the training data too well but fails to generalize to new data, and the vanishing/exploding gradient problem, which affects the network's ability to learn.
5. Applications and Impact
The applications of DNNs are vast and span multiple industries:
Image and Speech Recognition: DNNs have drastically improved the accuracy of image and speech recognition systems.
Natural Language Processing: From translation to sentiment analysis, DNNs have enhanced the understanding of human language by machines.
Healthcare: In medical diagnostics, DNNs assist in the analysis of complex medical data for early disease detection.
Autonomous Vehicles: DNNs are crucial in enabling vehicles to interpret sensory data and make informed decisions.
6. Ethical Considerations and Future Directions
As with any powerful technology, DNNs raise ethical questions related to privacy, data security, and the potential for misuse. Ensuring the responsible use of DNNs is paramount as the technology continues to advance.
In conclusion, deep neural networks are a cornerstone of modern AI. Their varied architectures and growing applications are not only fascinating from a technological standpoint but also hold immense potential for solving complex problems across different domains. As research progresses, we can expect DNNs to become even more sophisticated, pushing the boundaries of what machines can learn and achieve.
3 notes
·
View notes
Text
youtube
MIND-BLOWING Semantic Data Secrets Revealed in AI and Machine Learning
#GenerativeAI#GANs (Generative Adversarial Networks)#VAEs (Variational Autoencoders)#Artificial Intelligence#Machine Learning#Deep Learning#Neural Networks#AI Applications#CreativeAI#Natural Language Generation (NLG)#Image Synthesis#Text Generation#Computer Vision#Deepfake Technology#AI Art#Generative Design#Autonomous Systems#ContentCreation#Transfer Learning#Reinforcement Learning#Creative Coding#AI Innovation#TDM#health#healthcare#bootcamp#llm#branding#youtube#animation
1 note
·
View note
Text
Ok, social media bot idea: takes all the profile pictures in a reply section, runs em through an autoencoder, and gives you the statistically average profile picture that's commented on the post.
No clue if it'd work at all, but it'd be funny
#shitpost#196 campfire#you'd need to do this on tumblr or twitter i think#since too many people run snoo pfps on reddit
4 notes
·
View notes