#automl
Explore tagged Tumblr posts
Text
youtube
#sub media#gaza#palestine#israel#operation iron sword#hamas#habsora#ai#israel defense forces#idf#google#amazon#youtube#dahiya doctrine#nizoz#storm clouds#iai harpy#project nimbus#automl#elbit systems#israeli occupation#genocide#war crimes
7 notes
·
View notes
Text
Inteligencia Artificial: El Motor de la Nueva Era del Análisis de Datos
Introducción: De Datos Crudos a Decisiones Inteligentes Gracias a la IA Vivimos en una era de información sin precedentes. Las organizaciones generan y recopilan volúmenes masivos de datos cada segundo. Sin embargo, estos datos son solo potencial en bruto. El verdadero valor reside en la capacidad de analizarlos para extraer conocimientos, identificar patrones, predecir tendencias y, en última…
#Alteryx#Análisis de Datos#Analítica Aumentada#Analítica Predictiva#AutoML#Azure ML#BI#Big Data#Business Intelligence#Ciencia de Datos#Data Mining#Domo#Google#IA#IBM#IBM Watson#inteligencia artificial#Looker#machine learning#Microsoft#MicroStrategy#Oracle#Oracle Analytics#Palantir Technologies#Power BI#Qlik#RapidMiner#SAP#SAP Analytics Cloud#SAS Institute
0 notes
Text
How Vertical AI Agents Are Transforming Industry Intelligence in 2025
New Post has been published on https://thedigitalinsider.com/how-vertical-ai-agents-are-transforming-industry-intelligence-in-2025/
How Vertical AI Agents Are Transforming Industry Intelligence in 2025
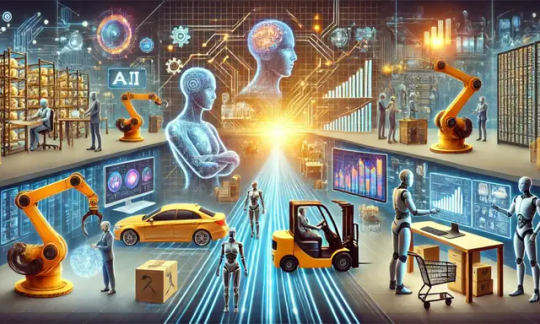
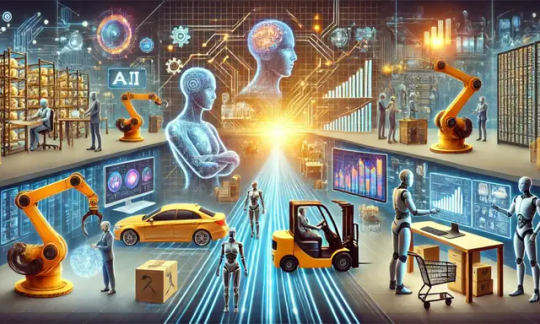
If 2024 was the year of significant advancements in general AI, 2025 is shaping up to be the year of specialized AI systems. Known as vertical AI agents, these purpose-built solutions combine advanced AI capabilities with deep domain expertise to tackle industry-specific challenges. McKinsey estimates that over 70% of AI’s total value potential will come from these vertical AI applications. Gartner predicts that more than 80% of enterprises will have used vertical AI by 2026. This article explores how vertical AI agents are reshaping industry intelligence and paving the way for a new era of business innovation.
From General-Purpose to Specialized AI
If you take a step back and look at the bigger picture of technological evolution, the shift from general-purpose AI to industry-specific AI is nothing new. It reflects a similar trend we have seen before. For instance, in the early days of enterprise software, platforms like SAP and Oracle offered broad capabilities that required extensive customization to meet unique business needs. Over time, vendors introduced tailored solutions like Salesforce Health Cloud for healthcare or Microsoft Dynamics 365 for retail, offering pre-built functionalities designed for specific industries.
Similarly, AI initially focused on general-purpose capabilities like pre-trained models and development platforms, which provided a foundation for building advanced solutions but required significant customization to develop industry-specific applications.
Vertical AI agents are bridging this gap. Solutions like PathAI in healthcare, Vue.ai in retail, and Feedzai in finance empower businesses with highly accurate and efficient tools specifically designed to meet their requirements. Gartner predicts that organizations using vertical AI see a 25% return on investment (ROI) compared to those relying on general-purpose AI. This figure highlights the effectiveness of vertical AI in addressing unique industry challenges.
Vertical AI: Next Level in AI Democratization
The rise of vertical AI agents is essentially the next big step in making AI more accessible to industry. In the early days, developing AI was expensive and limited to large corporations and research institutions due to the high costs and expertise required. Cloud platforms like AWS, Microsoft Azure, and Google Cloud have since made scalable infrastructure more affordable. Pre-trained models like OpenAI’s GPT and Google’s Gemini have allowed businesses to fine-tune AI for specific needs without requiring deep technical expertise or massive datasets. Low-code and no-code tools like Google AutoML and Microsoft Power Platform have taken it a step further, making AI accessible even to non-technical users. Vertical AI takes this accessibility to the next level by providing tools that are pre-configured for specific industry needs, reducing customization efforts and delivering better, more efficient results.
Why Vertical AI is a Billion Dollar Market
Vertical AI has the potential to redefine industries much like software-as-a-service (SaaS) did in the past. While SaaS made software scalable and accessible, vertical AI can take this one step further by automating entire workflows. For instance, while SaaS platforms like Salesforce improved customer relationship management, vertical AI agents can go a step further to autonomously identify sales opportunities and recommend personalized interactions.
By taking over repetitive tasks, vertical AI allows businesses to use their resources more effectively. In manufacturing, for example, vertical AI agents can predict equipment failures, optimize production schedules, and enhance supply chain management. These solutions not only improve efficiency but also reduce labor costs. Additionally, vertical AI agents integrate seamlessly with proprietary tools and workflows, significantly reducing the effort needed for integration. For example, in retail, vertical AI like Vue.ai integrates directly with e-commerce platforms and CRMs to analyze customer behavior and recommend personalized products, minimizing integration effort while improving efficiency. Moreover, vertical AI agents are designed to work within specific regulatory frameworks, such as Basel III in finance or HIPAA in healthcare, ensuring businesses can utilize AI without compromising on industry standards or ethical AI requirements.
Hence, it’s no surprise that the vertical AI market, valued at $5.1 billion in 2024, is projected to reach $47.1 billion by 2030 and could surpass $100 billion by 2032.
Vertical AI Agents in Action: Automotive AI Agents
Google Cloud has recently launched its vertical AI agents specifically designed for the automotive industry. Known as automotive AI agents, these tools are designed to help automakers create intelligent, customizable in-car assistants. Automakers can customize the agents by defining unique wake words, integrating third-party applications, and adding proprietary features. Integrated with vehicle systems and Android Automotive OS, these agents offer features like voice-controlled navigation, hands-free media playback, and predictive insights.
Mercedes-Benz has adopted Google Cloud’s Automotive AI Agent for its MBUX Virtual Assistant, debuting in the new CLA model. This enhanced assistant offers conversational interaction, personalized recommendations, proactive assistance, and precise navigation. By enabling hands-free operations, these agents enhance safety and cater to diverse user needs, showcasing the potential of vertical AI to revolutionize industries.
The Road Ahead: Challenges and Opportunities
While vertical AI agents have immense potential, they are not without challenges. Integrating these systems into businesses can be a challenging task due to legacy systems, data silos, and resistance to change. Also, building and deploying vertical AI agents isn’t easy as it requires a rare combination of AI expertise and industry-specific skills. Companies need teams that understand both the technology side and the specific needs of their industry.
As these systems play a bigger role in critical processes, ethical use and human oversight become crucial. Industries will need to develop ethical guidelines and governance frameworks to keep up with the technology.
That said, vertical AI offers enormous opportunities. With their combination of advanced AI and specialized expertise, these agents are set to become the cornerstone of business innovation in 2025 and beyond.
The Road Ahead
The rise of vertical AI agents is a vital moment in the evolution of industry intelligence. By addressing industry-specific challenges with ease and perfection, these systems have potential to redefine how businesses operate. However, their successful adoption will depend on overcoming integration challenges, building cross-disciplinary expertise, and ensuring ethical deployment.
As vertical AI continues to gain traction in 2025, it will likely reshape industries and redefine business operations. Companies that adopt these solutions early will position themselves to lead in an increasingly competitive market.
#2024#2025#Accessibility#adoption#agent#agents#ai#ai agent#AI AGENTS#AI systems#android#applications#Article#Artificial Intelligence#autoML#automotive#Automotive AI Agent#automotive industry#AWS#azure#Behavior#billion#Building#Business#change#Cloud#code#Commerce#Companies#customer relationship management
0 notes
Text
Integration of AutoML to Qlik Sense to enable Next Level Data Analytics
Data analytics have experienced an era of change with the advent of new technologies like machine learning (ML) and artificial intelligence (AI). Companies now are able to access more advanced tools that provide more accurate insights, faster operations, and better decision-making. One such effective combination can be found in the integration of Automated Machine Learning (AutoML) into Qlik Sense, a leading data visualization platform. This synergy enables businesses to increase their capabilities in data analytics and elevate your analytics-based (BI) initiatives to a higher level. The article below we'll discuss the benefits of connecting AutoML with Qlik Sense and how it will transform your data analytics processes.
What is AutoML?
AutoML refers to the automated of the complete process of applying machine-learning to real-world issues. This technology enables users regardless of their level of technical proficiency to build and implement models using machine learning without the need to write complicated software. AutoML platforms automatize tasks like data processing and model selection, as well as tuning of hyperparameters, and the evaluation of models. Through abstraction of a lot of the complexity that is involved with ML, AutoML tools make it simpler and quicker to incorporate advanced analytics into workflows for business.
Understanding Qlik Sense
Qlik Sense is a self-service data analytics and BI platform that lets users analyse data, design visualizations and share data in an easy-to-use interface. The platform is known for its associative database modeling, Qlik Sense enables users to look for patterns in data they would not otherwise be able to discover. The focus of the platform is on empowering users in the business sector means that no need for coding skills is required to develop sophisticated visualizations or dashboards.
Although Qlik Sense is already a effective tool to report and visualize data but the addition of AutoML capabilities offers a new level of capabilities. By connecting Qlik Sense's management capabilities and visualization abilities along with the predictive and analytical capabilities of AutoML companies can gain more insights, streamline the process of making data-driven decisions, and remain ahead of other businesses.
Benefits of integrating AutoML in Qlik Sense
1. Democratization of Advanced Analytics
Integrating AutoML in Qlik Sense lowers the barriers to the entry point for advanced analytics. In the past, companies relied on data scientists or machine-learning experts to develop prescriptive models or analyze huge data sets. With AutoML software, any person, whether an analyst in business or decision-maker can quickly create precise models and gain insight into predictive capabilities directly from Qlik Sense. Qlik Sense system.
The democratization of data science enables organizations to make the most of their data, without the need for advanced technical skills. In the end, many teams within the company can take data-driven decisions, enhancing overall performance of the business.
2. Improved Predictive Analytics
Predictive analytics is an important area in which AutoML can improve its capabilities in QlikSense. With AutoML software, users can apply algorithms that use machine learning on their data sets to predict the future behavior, trends or outcomes from previous data. These insights can be utilized to enhance the business processes, predict the demand, enhance customer experience and reduce risk.
For instance for example, integrating AutoML with Qlik Sense can enable a retail company to forecast the future trends in sales, or manufacturing firms to predict maintenance requirements for equipment. Through these predictions companies can take proactive action instead of reacting to events when they happen, leading to improved results.
3. Faster Decision-Making Using Automation
One of the major benefits of AutoML is its capacity to automate the lengthy process of developing models. Instead of manually creating data, choosing the right model, tuning hyperparameters and testing different approaches, AutoML platforms handle these processes automatically. This greatly reduces the time required to create meaningful conclusions from the data.
Through the use of AutoML embedded with Qlik Sense, business users can concentrate on interpreting data and making their own decisions while the platform takes care of the complex machine learning issues. The streamlining of workflows improves efficiency overall and allows businesses to make quick decision-making.
4. Scalability and Flexibility
Integrating AutoML in Qlik Sense enables organizations to increase their efforts in data analytics without burdening data science or IT teams. As data volumes increase, AutoML models can be quickly updated and retrained to keep up with the latest data. The scalability of AutoML ensures that businesses are able to continue to provide accurate data, even when the complexity and volume of their data grows.
Additionally, Qlik Sense's flexible structure lets businesses integrate AutoML models effortlessly into existing workflows. For the purpose of forecasting or anomaly identification or for classification, AutoML can be tailored to meet the particular requirements of each business.
Key Use Cases of AutoML in Qlik Sense
1. Customer Segmentation
Through the use of AutoML strategies, Qlik Sense can be employed to perform sophisticated customer segmentation using purchasing habits, demographics, and other important factors. This lets businesses determine distinct groups of customers and customize marketing strategies and improve customer satisfaction and increasing sales.
2. Churn Prediction
Companies can utilize AutoML models in Qlik Sense to predict customer turnover. By studying historical customer information, AutoML can identify patterns that show the time when customers are most likely to quit. Armed with this data businesses can devise targeted retention strategies to decrease the number of customers who leave and boost loyalty to their customers.
3. Anomaly Detection
AutoML could be utilized to spot odd patterns or outliers in data. For instance an institution of finance could utilize Qlik Sense with AutoML to identify fraudulent transactions, while an online retailer could be able to monitor supply chain irregularities. The early detection of these issues could help reduce risk and reduce loss.
How to Begin Using AutoML within Qlik Sense
To add AutoML in Qlik Sense, businesses should take a few steps:
Select the right AutoML Tool Choose the AutoML tool that works to Qlik Sense. The most well-known options are Google Cloud AutoML, H2O.ai, data science and DataRobot.
Preparing the data Check you have data that is in good condition and ready to be analyzed. This includes data preparation tasks like loss of value computation, normalization as well as feature engineering.
Train and deploy models Make use of the AutoML tool to build models using machine learning using your data. After the models have been created, you can deploy them in Qlik Sense to generate predictions and incorporate them into visualizations.
Monitor and refine Continuously check your models' performance. As data comes in make sure you retrain the models in order to ensure accuracy and relevance.
Conclusion
Integrating AutoML in Qlik Sense unlocks the full potential of data analytics, offering businesses with predictive insights advanced analytics capabilities and automated. Through reducing the requirement for specialist skills and speeding decision-making processes, AutoML empowers organizations to achieve a competitive advantage in today's highly data-driven world. Since businesses are continuing to focus on the use of data-driven strategies, pairing AutoML along with Qlik Sense training is an essential step toward achieving advanced analytics and accelerating expansion.
0 notes
Text
Evolusi Framework AI: Alat Terbaru untuk Pengembangan Model AI di 2025
Kecerdasan buatan (AI) telah menjadi salah satu bidang yang paling berkembang pesat dalam beberapa tahun terakhir. Pada tahun 2025, teknologi AI diperkirakan akan semakin maju, terutama dengan adanya berbagai alat dan framework baru yang memungkinkan pengembang untuk menciptakan model AI yang lebih canggih dan efisien. Framework AI adalah sekumpulan pustaka perangkat lunak dan alat yang digunakan…
#AI applications#AI automation#AI development tools#AI ethics#AI for business#AI framework#AI in 2025#AI in edge devices#AI technology trends#AI transparency#AutoML#deep learning#edge computing#future of AI#machine learning#machine learning automation#model optimization#PyTorch#quantum computing#TensorFlow
0 notes
Text
KNIME Analytics Platform
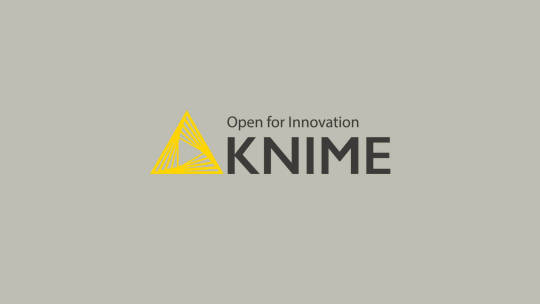
KNIME Analytics Platform: Open-Source Data Science and Machine Learning for All In the world of data science and machine learning, KNIME Analytics Platform stands out as a powerful and versatile solution that is accessible to both technical and non-technical users alike. Known for its open-source foundation, KNIME provides a flexible, visual workflow interface that enables users to create, deploy, and manage data science projects with ease. Whether used by individual data scientists or entire enterprise teams, KNIME supports the full data science lifecycle—from data integration and transformation to machine learning and deployment. Empowering Data Science with a Visual Workflow Interface At the heart of KNIME’s appeal is its drag-and-drop interface, which allows users to design workflows without needing to code. This visual approach democratizes data science, allowing business analysts, data scientists, and engineers to collaborate seamlessly and create powerful analytics workflows. KNIME’s modular architecture also enables users to expand its functionality through a vast library of nodes, extensions, and community-contributed components, making it one of the most flexible platforms for data science and machine learning. Key Features of KNIME Analytics Platform KNIME’s comprehensive feature set addresses a wide range of data science needs: - Data Preparation and ETL: KNIME provides robust tools for data integration, cleansing, and transformation, supporting everything from structured to unstructured data sources. The platform’s ETL (Extract, Transform, Load) capabilities are highly customizable, making it easy to prepare data for analysis. - Machine Learning and AutoML: KNIME comes with a suite of built-in machine learning algorithms, allowing users to build models directly within the platform. It also offers Automated Machine Learning (AutoML) capabilities, simplifying tasks like model selection and hyperparameter tuning, so users can rapidly develop effective machine learning models. - Explainable AI (XAI): With the growing importance of model transparency, KNIME provides tools for explainability and interpretability, such as feature impact analysis and interactive visualizations. These tools enable users to understand how models make predictions, fostering trust and facilitating decision-making in regulated industries. - Integration with External Tools and Libraries: KNIME supports integration with popular machine learning libraries and tools, including TensorFlow, H2O.ai, Scikit-learn, and Python and R scripts. This compatibility allows advanced users to leverage KNIME’s workflow environment alongside powerful external libraries, expanding the platform’s modeling and analytical capabilities. - Big Data and Cloud Extensions: KNIME offers extensions for big data processing, supporting frameworks like Apache Spark and Hadoop. Additionally, KNIME integrates with cloud providers, including AWS, Google Cloud, and Microsoft Azure, making it suitable for organizations with cloud-based data architectures. - Model Deployment and Management with KNIME Server: For enterprise users, KNIME Server provides enhanced capabilities for model deployment, automation, and monitoring. KNIME Server enables teams to deploy models to production environments with ease and facilitates collaboration by allowing multiple users to work on projects concurrently. Diverse Applications Across Industries KNIME Analytics Platform is utilized across various industries for a wide range of applications: - Customer Analytics and Marketing: KNIME enables businesses to perform customer segmentation, sentiment analysis, and predictive marketing, helping companies deliver personalized experiences and optimize marketing strategies. - Financial Services: In finance, KNIME is used for fraud detection, credit scoring, and risk assessment, where accurate predictions and data integrity are essential. - Healthcare and Life Sciences: KNIME supports healthcare providers and researchers with applications such as outcome prediction, resource optimization, and patient data analytics. - Manufacturing and IoT: The platform’s capabilities in anomaly detection and predictive maintenance make it ideal for manufacturing and IoT applications, where data-driven insights are key to operational efficiency. Deployment Flexibility and Integration Capabilities KNIME’s flexibility extends to its deployment options. KNIME Analytics Platform is available as a free, open-source desktop application, while KNIME Server provides enterprise-level features for deployment, collaboration, and automation. The platform’s support for Docker containers also enables organizations to deploy models in various environments, including hybrid and cloud setups. Additionally, KNIME integrates seamlessly with databases, data lakes, business intelligence tools, and external libraries, allowing it to function as a core component of a company’s data architecture. Pricing and Community Support KNIME offers both free and commercial licensing options. The open-source KNIME Analytics Platform is free to use, making it an attractive option for data science teams looking to minimize costs while maximizing capabilities. For organizations that require advanced deployment, monitoring, and collaboration, KNIME Server is available through a subscription-based model. The KNIME community is an integral part of the platform’s success. With an active forum, numerous tutorials, and a repository of workflows on KNIME Hub, users can find solutions to common challenges, share their work, and build on contributions from other users. Additionally, KNIME offers dedicated support and learning resources through KNIME Learning Hub and KNIME Academy, ensuring users have access to continuous training. Conclusion KNIME Analytics Platform is a robust, flexible, and accessible data science tool that empowers users to design, deploy, and manage data workflows without the need for extensive coding. From data preparation and machine learning to deployment and interpretability, KNIME’s extensive capabilities make it a valuable asset for organizations across industries. With its open-source foundation, active community, and enterprise-ready features, KNIME provides a scalable solution for data-driven decision-making and a compelling option for any organization looking to integrate data science into their operations. Read the full article
#AutomatedMachineLearning#AutoML#dataintegration#datapreparation#datascienceplatform#datatransformation#datawrangling#ETL#KNIME#KNIMEAnalyticsPlatform#machinelearning#open-sourceAI
0 notes
Text
Mastering Abacus.ai: Tips and Tricks for Effective AI Model Building
Abacus.ai is a powerful tool for creating AI models, but to get the best results, it’s important to know how to leverage its features effectively. Here are some tips and tricks to help you maximize your success with Abacus.ai.
Tip 1: Start with AutoML for Quick Results
Explanation: Use Abacus.ai’s AutoML feature to quickly build and deploy models without worrying about the complexities of manual coding. It’s a great way to get started and see initial results fast.
Tip 2: Utilize Custom Pipelines for Specific Needs
Explanation: If you have unique requirements, take advantage of Abacus.ai’s customizable pipelines. This feature allows you to modify data ingestion, feature engineering, and model training processes to suit specific business needs.
Tip 3: Monitor Real-Time Data for Dynamic Models
Explanation: Use real-time data processing to keep your models up to date. Regularly monitor incoming data streams to ensure that your AI models adapt to changes and stay relevant.
Tip 4: Experiment with Deep Learning for Complex Use Cases
Explanation: Abacus.ai supports deep learning models, which are ideal for complex use cases like image classification or natural language processing. Experiment with these features to unlock advanced capabilities.
Tip 5: Test Different Hyperparameters for Optimization
Explanation: When using AutoML, you can manually adjust hyperparameters to see how they affect model performance. Testing different settings can lead to better accuracy and improved model outcomes.
Leverage these tips to get the most out of Abacus.ai and create effective AI models for your business. Visit Abacus.ai to learn more and start building today!
#AITips#AbacusAI#MachineLearning#AutoML#DeepLearning#AIModelBuilding#DataScience#BusinessIntelligence#CustomPipelines#RealTimeAI
0 notes
Text
AutoML is revolutionizing the way AI is built and deployed. By automating the time-consuming tasks of model selection, hyperparameter tuning, and feature engineering, AutoML empowers businesses to implement AI solutions faster and more efficiently. Whether you're a small startup or a large enterprise, you can now leverage the power of machine learning without needing a team of data scientists. This means faster innovation, reduced costs, and better scalability for your AI projects.
Unlock the future of AI with AutoML and take your business to the next level.
Read more
0 notes
Text
Optimierung der KI-Modellentwicklung durch AutoML-Technologien
In der heutigen datengetriebenen Welt spielt die Entwicklung von Künstlicher Intelligenz (KI) eine zentrale Rolle in der Verbesserung von Geschäftsprozessen und Entscheidungen. AutoML-Technologien (Automated Machine Learning) bieten innovative Ansätze zur Optimierung der KI-Modellentwicklung, indem sie den manuellen Aufwand reduzieren und die Effizienz steigern. In diesem Artikel werden wir…
#Automatisierung#AutoML#Effizienzsteigerung#Feature Engineering#Geschäftsprozesse#Innovation#Innovationen#KI-Modelle#Machine Learning#Random Forests#RPA
0 notes
Text

Are you into data science? Because our conversation just hit all the right trends—insights, algorithms, and maybe even a bit of statistical romance!
.
#explainableai#coding#ai#programming#development#bigdata#python#datascience#automl#quantum computing#responsibleai
1 note
·
View note
Text
Understanding AutoML: An Overview | USAII®
AutoML has helped the development and deployment of machine learning models easier, and effortless. Learn its advantages, uses, popular AutoML tools, challenges, and more.
Read more: https://shorturl.at/CpscF
Automated machine learning, AutoML systems, AutoML models, AutoML platforms, automated AutoML, AutoML Tools, AI professionals, AI Certification Programs, Machine Learning Certifications. ML tools

0 notes
Text
0 notes
Text
#DataScience#AI#MachineLearning#HealthcareInnovation#FinanceTech#MarketingAnalytics#BigData#PredictiveAnalytics#FutureTech#QuantumComputing#EthicalAI#AutoML#TechTrends
0 notes
Text
#sub media#gaza#palestine#israel#operation iron sword#hamas#habsora#ai#israel defense forces#idf#google#amazon#youtube#dahiya doctrine#nizoz#storm clouds#iai harpy#project nimbus#automl#elbit systems#israeli occupation#genocide#war crimes
1 note
·
View note
Text
Semplificazione dei processi di machine learning con AutoML
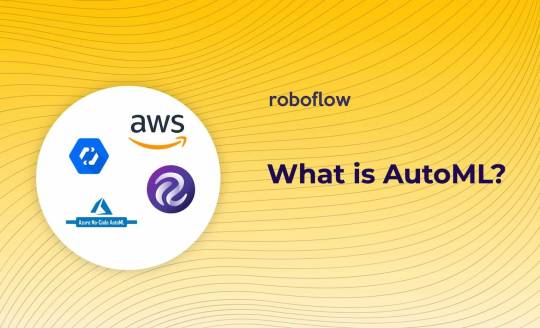
Negli ultimi anni, l’apprendimento automatico è diventato un potente strumento per estrarre informazioni e fare previsioni da set di dati complessi. Tuttavia, lo sviluppo e l’implementazione di modelli di machine learning può essere un processo dispendioso in termini di tempo e risorse. È qui che entra in gioco AutoML, o Automated Machine Learning. AutoML è un insieme di strumenti e processi che mirano ad automatizzare il processo di creazione e distribuzione di modelli di machine learning, rendendolo più veloce e più accessibile per le organizzazioni di tutte le dimensioni.
Cos'è AutoML?
AutoML comprende una gamma di tecnologie e tecniche che automatizzano varie fasi del processo di machine learning, tra cui la preelaborazione dei dati, l'ingegneria delle funzionalità, la selezione del modello, l'ottimizzazione degli iperparametri e la valutazione del modello. Automatizzando queste attività, AutoML mira a ridurre la necessità di intervento manuale e competenze, consentendo ai non esperti di creare e distribuire modelli di machine learning in modo più efficace.
Vantaggi di AutoML
Esistono diversi vantaggi chiave derivanti dall'utilizzo di AutoML per semplificare i processi di machine learning: - Risparmio di tempo e risorse: l'automazione della pipeline di machine learning può ridurre significativamente il tempo e le risorse necessarie per sviluppare e distribuire modelli, consentendo ai data scientist e agli ingegneri di concentrarsi su attività più complesse. - Accesso alle competenze: gli strumenti AutoML possono democratizzare l'accesso al machine learning fornendo ai non esperti la possibilità di creare e distribuire modelli senza una conoscenza tecnica approfondita. - Prestazioni del modello migliorate: automatizzando il processo di ottimizzazione degli iperparametri e di selezione del modello, AutoML può contribuire a migliorare le prestazioni dei modelli di machine learning, portando a previsioni e approfondimenti più accurati. - Scalabilità: gli strumenti AutoML sono progettati per essere scalabili, consentendo alle organizzazioni di creare e distribuire un gran numero di modelli di machine learning in diversi casi d'uso e domini.
Come funziona AutoML
AutoML funziona sfruttando una varietà di tecniche e algoritmi per automatizzare le diverse fasi della pipeline di machine learning: - Preelaborazione dei dati: Gli strumenti AutoML possono gestire automaticamente valori mancanti, rilevamento di valori anomali e ridimensionamento delle funzionalità, oltre ad altre attività di preelaborazione dei dati. - Ingegneria delle funzionalità: AutoML può generare e selezionare automaticamente le funzionalità rilevanti dai dati, riducendo la necessità di progettare manualmente le funzionalità. - Selezione del modello: Gli strumenti AutoML possono esplorare una gamma di modelli di machine learning e selezionare il modello con le migliori prestazioni per una determinata attività. - Ottimizzazione degli iperparametri: AutoML può ottimizzare automaticamente gli iperparametri dei modelli di machine learning per migliorarne le prestazioni. - Valutazione del modello: Gli strumenti AutoML possono automatizzare il processo di valutazione e confronto di diversi modelli, aiutando gli utenti a selezionare il modello con le migliori prestazioni.
Sfide di AutoML
Sebbene AutoML offra numerosi vantaggi, ci sono anche alcune sfide e limitazioni da considerare: - Modelli scatola nera: Alcuni strumenti AutoML possono generare modelli complessi di machine learning difficili da interpretare e spiegare, il che può rappresentare una sfida per settori come la sanità e la finanza in cui la spiegabilità del modello è fondamentale. - Competenza nel settore: Gli strumenti AutoML richiedono ancora competenze nel settore per definire il problema, selezionare funzionalità appropriate e interpretare i risultati, quindi non sostituiscono la conoscenza e l'esperienza nel settore. - Qualità dei dati: Gli strumenti AutoML dipendono fortemente dalla qualità e dalla quantità dei dati di input, quindi garantire set di dati diversificati e di alta qualità è ancora un aspetto cruciale del machine learning.
Casi d'uso di AutoML
AutoML offre un'ampia gamma di casi d'uso in diversi settori, tra cui: - Servizi finanziari: AutoML può essere utilizzato per creare e distribuire modelli predittivi per il credit scoring, il rilevamento delle frodi e la valutazione del rischio. - Assistenza sanitaria: AutoML può aiutare le organizzazioni sanitarie a creare e distribuire modelli per la previsione delle malattie, la previsione degli esiti dei pazienti e l'analisi delle immagini mediche. - Vedere al dettaglio: AutoML può essere utilizzato per creare sistemi di consigli, modelli di previsione della domanda e modelli di segmentazione della clientela. - Produzione: AutoML può aiutare i produttori a creare modelli di manutenzione predittiva, modelli di controllo qualità e modelli di ottimizzazione della catena di fornitura.
Conclusione
AutoML è un potente insieme di strumenti e tecniche che possono aiutare le organizzazioni a semplificare il processo di machine learning, rendendolo più accessibile, efficiente e scalabile. Automatizzando le diverse fasi della pipeline di machine learning, AutoML può aiutare le organizzazioni a creare e distribuire modelli in modo più efficace, consentendo loro di estrarre informazioni preziose e fare previsioni migliori dai propri dati. Read the full article
0 notes