#databricks developers
Explore tagged Tumblr posts
Text
Maximizing Manufacturing Efficiency with Databricks Platform
Databricks Platform is a game-changer for the manufacturing industry. Its ability to integrate and process vast amounts of data, provide predictive maintenance, offer real-time analytics, and enhance quality control makes it an essential tool for modern manufacturers.
#Databricks Platform#Databricks#databricks developers#databricks solutions#databricks services#lagozon technologies
0 notes
Text
Unlocking the Potential of Databricks: Comprehensive Services and Solutions
In the fast-paced world of big data and artificial intelligence, Databricks services have emerged as a crucial component for businesses aiming to harness the full potential of their data. From accelerating data engineering processes to implementing cutting-edge AI models, Databricks offers a unified platform that integrates seamlessly with various business operations. In this article, we explore the breadth of Databricks solutions, the expertise of Databricks developers, and the transformative power of Databricks artificial intelligence capabilities.
Databricks Services: Driving Data-Driven Success
Databricks services encompass a wide range of offerings designed to enhance data management, analytics, and machine learning capabilities. These services are instrumental in helping businesses:
Streamline Data Processing: Databricks provides powerful tools to process large volumes of data quickly and efficiently, reducing the time required to derive actionable insights.
Enable Advanced Analytics: By integrating with popular analytics tools, Databricks allows organizations to perform complex analyses and gain deeper insights into their data.
Support Collaborative Development: Databricks fosters collaboration among data scientists, engineers, and business analysts, facilitating a more cohesive approach to data-driven projects.
Innovative Databricks Solutions for Modern Businesses
Databricks solutions are tailored to address the diverse needs of businesses across various industries. These solutions include:
Unified Data Analytics: Combining data engineering, data science, and machine learning into a single platform, Databricks simplifies the process of building and deploying data-driven applications.
Real-Time Data Processing: With support for streaming data, Databricks enables businesses to process and analyze data in real-time, ensuring timely and accurate decision-making.
Scalable Data Management: Databricks’ cloud-based architecture allows organizations to scale their data processing capabilities as their needs grow, without worrying about infrastructure limitations.
Integrated Machine Learning: Databricks supports the entire machine learning lifecycle, from data preparation to model deployment, making it easier to integrate AI into business processes.
Expertise of Databricks Developers: Building the Future of Data
Databricks developers are highly skilled professionals who specialize in leveraging the Databricks platform to create robust, scalable data solutions. Their roles include:
Data Engineering: Developing and maintaining data pipelines that transform raw data into usable formats for analysis and machine learning.
Machine Learning Engineering: Building and deploying machine learning models that can predict outcomes, automate tasks, and provide valuable business insights.
Analytics and Reporting: Creating interactive dashboards and reports that allow stakeholders to explore data and uncover trends and patterns.
Platform Integration: Ensuring seamless integration of Databricks with existing IT systems and workflows, enhancing overall efficiency and productivity.
Databricks Artificial Intelligence: Transforming Data into Insights
Databricks artificial intelligence capabilities enable businesses to leverage AI technologies to gain competitive advantages. Key aspects of Databricks AI include:
Automated Machine Learning: Databricks simplifies the creation of machine learning models with automated tools that help select the best algorithms and parameters.
Scalable AI Infrastructure: Leveraging cloud resources, Databricks can handle the intensive computational requirements of training and deploying complex AI models.
Collaborative AI Development: Databricks promotes collaboration among data scientists, allowing teams to share code, models, and insights seamlessly.
Real-Time AI Applications: Databricks supports the deployment of AI models that can process and analyze data in real-time, providing immediate insights and responses.
Data Engineering Services: Enhancing Data Value
Data engineering services are a critical component of the Databricks ecosystem, enabling organizations to transform raw data into valuable assets. These services include:
Data Pipeline Development: Building robust pipelines that automate the extraction, transformation, and loading (ETL) of data from various sources into centralized data repositories.
Data Quality Management: Implementing processes and tools to ensure the accuracy, consistency, and reliability of data across the organization.
Data Integration: Combining data from different sources and systems to create a unified view that supports comprehensive analysis and reporting.
Performance Optimization: Enhancing the performance of data systems to handle large-scale data processing tasks efficiently and effectively.
Databricks Software: Empowering Data-Driven Innovation
Databricks software is designed to empower businesses with the tools they need to innovate and excel in a data-driven world. The core features of Databricks software include:
Interactive Workspaces: Providing a collaborative environment where teams can work together on data projects in real-time.
Advanced Security and Compliance: Ensuring that data is protected with robust security measures and compliance with industry standards.
Extensive Integrations: Offering seamless integration with popular tools and platforms, enhancing the flexibility and functionality of data operations.
Scalable Computing Power: Leveraging cloud infrastructure to provide scalable computing resources that can accommodate the demands of large-scale data processing and analysis.
Leveraging Databricks for Competitive Advantage
To fully harness the capabilities of Databricks, businesses should consider the following strategies:
Adopt a Unified Data Strategy: Utilize Databricks to unify data operations across the organization, from data engineering to machine learning.
Invest in Skilled Databricks Developers: Engage professionals who are proficient in Databricks to build and maintain your data infrastructure.
Integrate AI into Business Processes: Use Databricks’ AI capabilities to automate tasks, predict trends, and enhance decision-making processes.
Ensure Data Quality and Security: Implement best practices for data management to maintain high-quality data and ensure compliance with security standards.
Scale Operations with Cloud Resources: Take advantage of Databricks’ cloud-based architecture to scale your data operations as your business grows.
The Future of Databricks Services and Solutions
As the field of data and AI continues to evolve, Databricks services and solutions will play an increasingly vital role in driving business innovation and success. Future trends may include:
Enhanced AI Capabilities: Continued advancements in AI will enable Databricks to offer more powerful and intuitive AI tools that can address complex business challenges.
Greater Integration with Cloud Ecosystems: Databricks will expand its integration capabilities, allowing businesses to seamlessly connect with a broader range of cloud services and platforms.
Increased Focus on Real-Time Analytics: The demand for real-time data processing and analytics will grow, driving the development of more advanced streaming data solutions.
Expanding Global Reach: As more businesses recognize the value of data and AI, Databricks will continue to expand its presence and influence across different markets and industries.
#databricks services#databricks solutions#databricks developers#databricks artificial intelligence#data engineering services#databricks software
0 notes
Text
Leveraging Databricks Services for Optimal Solutions
In today's rapidly evolving digital landscape, businesses are continually seeking Databricks services to streamline their operations and gain a competitive edge. Whether it's Databricks solutions for data engineering or harnessing the power of Databricks developers to propel artificial intelligence initiatives, the demand for top-tier services is at an all-time high.
Unleashing the Power of Databricks Solutions
Data Engineering Services: Building the Foundation for Success
Data engineering services form the backbone of any successful data-driven organization. With Databricks, businesses can unlock the full potential of their data by leveraging cutting-edge technologies and methodologies. From data ingestion to processing and visualization, Databricks offers a comprehensive suite of tools to streamline the entire data pipeline.
Harnessing Artificial Intelligence with Databricks
In the age of artificial intelligence, businesses that fail to adapt risk falling behind the competition. Databricks provides a robust platform for developing and deploying AI solutions at scale. By harnessing the power of machine learning and deep learning algorithms, organizations can gain valuable insights and drive innovation like never before.
Empowering Developers with Databricks
Enabling Collaboration and Innovation
Databricks developers play a pivotal role in driving innovation and accelerating time-to-market for new products and services. With Databricks, developers can collaborate seamlessly, share insights, and iterate rapidly to deliver high-quality solutions that meet the ever-changing needs of their organization and customers.
Streamlining Development Workflows
Databricks simplifies the development process by providing a unified environment for data engineering, data science, and machine learning. By eliminating the need to manage multiple tools and platforms, developers can focus on what they do best: writing code and building transformative solutions.
The Key to Success: Choosing the Right Partner
When it comes to Databricks services, choosing the right partner is essential. Look for a provider with a proven track record of success and a deep understanding of your industry and business needs. Whether you're embarking on a data engineering project or exploring the possibilities of artificial intelligence, partnering with a trusted Databricks provider can make all the difference.
Driving Success for the Digital Economy
Databricks services offer a myriad of opportunities for businesses looking to harness the power of data and Databricks artificial intelligence. From data engineering to machine learning, Databricks provides the tools and technologies needed to drive innovation and achieve success in today's digital economy. By partnering with a trusted provider, businesses can unlock new possibilities and stay ahead of the competition.
#databricks services#databricks solutions#databricks developers#databricks artificial intelligence#data engineering services
0 notes
Text

Hire Databricks developers to build scalable data pipelines, optimize Spark performance, and integrate AI/ML solutions.
0 notes
Text
Tracking Large Language Models (LLM) with MLflow : A Complete Guide
New Post has been published on https://thedigitalinsider.com/tracking-large-language-models-llm-with-mlflow-a-complete-guide/
Tracking Large Language Models (LLM) with MLflow : A Complete Guide
As Large Language Models (LLMs) grow in complexity and scale, tracking their performance, experiments, and deployments becomes increasingly challenging. This is where MLflow comes in – providing a comprehensive platform for managing the entire lifecycle of machine learning models, including LLMs.
In this in-depth guide, we’ll explore how to leverage MLflow for tracking, evaluating, and deploying LLMs. We’ll cover everything from setting up your environment to advanced evaluation techniques, with plenty of code examples and best practices along the way.
Functionality of MLflow in Large Language Models (LLMs)
MLflow has become a pivotal tool in the machine learning and data science community, especially for managing the lifecycle of machine learning models. When it comes to Large Language Models (LLMs), MLflow offers a robust suite of tools that significantly streamline the process of developing, tracking, evaluating, and deploying these models. Here’s an overview of how MLflow functions within the LLM space and the benefits it provides to engineers and data scientists.
Tracking and Managing LLM Interactions
MLflow’s LLM tracking system is an enhancement of its existing tracking capabilities, tailored to the unique needs of LLMs. It allows for comprehensive tracking of model interactions, including the following key aspects:
Parameters: Logging key-value pairs that detail the input parameters for the LLM, such as model-specific parameters like top_k and temperature. This provides context and configuration for each run, ensuring that all aspects of the model’s configuration are captured.
Metrics: Quantitative measures that provide insights into the performance and accuracy of the LLM. These can be updated dynamically as the run progresses, offering real-time or post-process insights.
Predictions: Capturing the inputs sent to the LLM and the corresponding outputs, which are stored as artifacts in a structured format for easy retrieval and analysis.
Artifacts: Beyond predictions, MLflow can store various output files such as visualizations, serialized models, and structured data files, allowing for detailed documentation and analysis of the model’s performance.
This structured approach ensures that all interactions with the LLM are meticulously recorded, providing a comprehensive lineage and quality tracking for text-generating models​.
Evaluation of LLMs
Evaluating LLMs presents unique challenges due to their generative nature and the lack of a single ground truth. MLflow simplifies this with specialized evaluation tools designed for LLMs. Key features include:
Versatile Model Evaluation: Supports evaluating various types of LLMs, whether it’s an MLflow pyfunc model, a URI pointing to a registered MLflow model, or any Python callable representing your model.
Comprehensive Metrics: Offers a range of metrics tailored for LLM evaluation, including both SaaS model-dependent metrics (e.g., answer relevance) and function-based metrics (e.g., ROUGE, Flesch Kincaid).
Predefined Metric Collections: Depending on the use case, such as question-answering or text-summarization, MLflow provides predefined metrics to simplify the evaluation process.
Custom Metric Creation: Allows users to define and implement custom metrics to suit specific evaluation needs, enhancing the flexibility and depth of model evaluation.
Evaluation with Static Datasets: Enables evaluation of static datasets without specifying a model, which is useful for quick assessments without rerunning model inference.
Deployment and Integration
MLflow also supports seamless deployment and integration of LLMs:
MLflow Deployments Server: Acts as a unified interface for interacting with multiple LLM providers. It simplifies integrations, manages credentials securely, and offers a consistent API experience. This server supports a range of foundational models from popular SaaS vendors as well as self-hosted models.
Unified Endpoint: Facilitates easy switching between providers without code changes, minimizing downtime and enhancing flexibility.
Integrated Results View: Provides comprehensive evaluation results, which can be accessed directly in the code or through the MLflow UI for detailed analysis.
MLflow is a comprehensive suite of tools and integrations makes it an invaluable asset for engineers and data scientists working with advanced NLP models.
Setting Up Your Environment
Before we dive into tracking LLMs with MLflow, let’s set up our development environment. We’ll need to install MLflow and several other key libraries:
pip install mlflow>=2.8.1 pip install openai pip install chromadb==0.4.15 pip install langchain==0.0.348 pip install tiktoken pip install 'mlflow[genai]' pip install databricks-sdk --upgrade
After installation, it’s a good practice to restart your Python environment to ensure all libraries are properly loaded. In a Jupyter notebook, you can use:
import mlflow import chromadb print(f"MLflow version: mlflow.__version__") print(f"ChromaDB version: chromadb.__version__")
This will confirm the versions of key libraries we’ll be using.
Understanding MLflow’s LLM Tracking Capabilities
MLflow’s LLM tracking system builds upon its existing tracking capabilities, adding features specifically designed for the unique aspects of LLMs. Let’s break down the key components:
Runs and Experiments
In MLflow, a “run” represents a single execution of your model code, while an “experiment” is a collection of related runs. For LLMs, a run might represent a single query or a batch of prompts processed by the model.
Key Tracking Components
Parameters: These are input configurations for your LLM, such as temperature, top_k, or max_tokens. You can log these using mlflow.log_param() or mlflow.log_params().
Metrics: Quantitative measures of your LLM’s performance, like accuracy, latency, or custom scores. Use mlflow.log_metric() or mlflow.log_metrics() to track these.
Predictions: For LLMs, it’s crucial to log both the input prompts and the model’s outputs. MLflow stores these as artifacts in CSV format using mlflow.log_table().
Artifacts: Any additional files or data related to your LLM run, such as model checkpoints, visualizations, or dataset samples. Use mlflow.log_artifact() to store these.
Let’s look at a basic example of logging an LLM run:
This example demonstrates logging parameters, metrics, and the input/output as a table artifact.
import mlflow import openai def query_llm(prompt, max_tokens=100): response = openai.Completion.create( engine="text-davinci-002", prompt=prompt, max_tokens=max_tokens ) return response.choices[0].text.strip() with mlflow.start_run(): prompt = "Explain the concept of machine learning in simple terms." # Log parameters mlflow.log_param("model", "text-davinci-002") mlflow.log_param("max_tokens", 100) # Query the LLM and log the result result = query_llm(prompt) mlflow.log_metric("response_length", len(result)) # Log the prompt and response mlflow.log_table("prompt_responses", "prompt": [prompt], "response": [result]) print(f"Response: result")
Deploying LLMs with MLflow
MLflow provides powerful capabilities for deploying LLMs, making it easier to serve your models in production environments. Let’s explore how to deploy an LLM using MLflow’s deployment features.
Creating an Endpoint
First, we’ll create an endpoint for our LLM using MLflow’s deployment client:
import mlflow from mlflow.deployments import get_deploy_client # Initialize the deployment client client = get_deploy_client("databricks") # Define the endpoint configuration endpoint_name = "llm-endpoint" endpoint_config = "served_entities": [ "name": "gpt-model", "external_model": "name": "gpt-3.5-turbo", "provider": "openai", "task": "llm/v1/completions", "openai_config": "openai_api_type": "azure", "openai_api_key": "secrets/scope/openai_api_key", "openai_api_base": "secrets/scope/openai_api_base", "openai_deployment_name": "gpt-35-turbo", "openai_api_version": "2023-05-15", , , ], # Create the endpoint client.create_endpoint(name=endpoint_name, config=endpoint_config)
This code sets up an endpoint for a GPT-3.5-turbo model using Azure OpenAI. Note the use of Databricks secrets for secure API key management.
Testing the Endpoint
Once the endpoint is created, we can test it:
<div class="relative flex flex-col rounded-lg"> response = client.predict( endpoint=endpoint_name, inputs="prompt": "Explain the concept of neural networks briefly.","max_tokens": 100,,) print(response)
This will send a prompt to our deployed model and return the generated response.
Evaluating LLMs with MLflow
Evaluation is crucial for understanding the performance and behavior of your LLMs. MLflow provides comprehensive tools for evaluating LLMs, including both built-in and custom metrics.
Preparing Your LLM for Evaluation
To evaluate your LLM with mlflow.evaluate(), your model needs to be in one of these forms:
An mlflow.pyfunc.PyFuncModel instance or a URI pointing to a logged MLflow model.
A Python function that takes string inputs and outputs a single string.
An MLflow Deployments endpoint URI.
Set model=None and include model outputs in the evaluation data.
Let’s look at an example using a logged MLflow model:
import mlflow import openai with mlflow.start_run(): system_prompt = "Answer the following question concisely." logged_model_info = mlflow.openai.log_model( model="gpt-3.5-turbo", task=openai.chat.completions, artifact_path="model", messages=[ "role": "system", "content": system_prompt, "role": "user", "content": "question", ], ) # Prepare evaluation data eval_data = pd.DataFrame( "question": ["What is machine learning?", "Explain neural networks."], "ground_truth": [ "Machine learning is a subset of AI that enables systems to learn and improve from experience without explicit programming.", "Neural networks are computing systems inspired by biological neural networks, consisting of interconnected nodes that process and transmit information." ] ) # Evaluate the model results = mlflow.evaluate( logged_model_info.model_uri, eval_data, targets="ground_truth", model_type="question-answering", ) print(f"Evaluation metrics: results.metrics")
This example logs an OpenAI model, prepares evaluation data, and then evaluates the model using MLflow’s built-in metrics for question-answering tasks.
Custom Evaluation Metrics
MLflow allows you to define custom metrics for LLM evaluation. Here’s an example of creating a custom metric for evaluating the professionalism of responses:
from mlflow.metrics.genai import EvaluationExample, make_genai_metric professionalism = make_genai_metric( name="professionalism", definition="Measure of formal and appropriate communication style.", grading_prompt=( "Score the professionalism of the answer on a scale of 0-4:n" "0: Extremely casual or inappropriaten" "1: Casual but respectfuln" "2: Moderately formaln" "3: Professional and appropriaten" "4: Highly formal and expertly crafted" ), examples=[ EvaluationExample( input="What is MLflow?", output="MLflow is like your friendly neighborhood toolkit for managing ML projects. It's super cool!", score=1, justification="The response is casual and uses informal language." ), EvaluationExample( input="What is MLflow?", output="MLflow is an open-source platform for the machine learning lifecycle, including experimentation, reproducibility, and deployment.", score=4, justification="The response is formal, concise, and professionally worded." ) ], model="openai:/gpt-3.5-turbo-16k", parameters="temperature": 0.0, aggregations=["mean", "variance"], greater_is_better=True, ) # Use the custom metric in evaluation results = mlflow.evaluate( logged_model_info.model_uri, eval_data, targets="ground_truth", model_type="question-answering", extra_metrics=[professionalism] ) print(f"Professionalism score: results.metrics['professionalism_mean']")
This custom metric uses GPT-3.5-turbo to score the professionalism of responses, demonstrating how you can leverage LLMs themselves for evaluation.
Advanced LLM Evaluation Techniques
As LLMs become more sophisticated, so do the techniques for evaluating them. Let’s explore some advanced evaluation methods using MLflow.
Retrieval-Augmented Generation (RAG) Evaluation
RAG systems combine the power of retrieval-based and generative models. Evaluating RAG systems requires assessing both the retrieval and generation components. Here’s how you can set up a RAG system and evaluate it using MLflow:
from langchain.document_loaders import WebBaseLoader from langchain.text_splitter import CharacterTextSplitter from langchain.embeddings import OpenAIEmbeddings from langchain.vectorstores import Chroma from langchain.chains import RetrievalQA from langchain.llms import OpenAI # Load and preprocess documents loader = WebBaseLoader(["https://mlflow.org/docs/latest/index.html"]) documents = loader.load() text_splitter = CharacterTextSplitter(chunk_size=1000, chunk_overlap=0) texts = text_splitter.split_documents(documents) # Create vector store embeddings = OpenAIEmbeddings() vectorstore = Chroma.from_documents(texts, embeddings) # Create RAG chain llm = OpenAI(temperature=0) qa_chain = RetrievalQA.from_chain_type( llm=llm, chain_type="stuff", retriever=vectorstore.as_retriever(), return_source_documents=True ) # Evaluation function def evaluate_rag(question): result = qa_chain("query": question) return result["result"], [doc.page_content for doc in result["source_documents"]] # Prepare evaluation data eval_questions = [ "What is MLflow?", "How does MLflow handle experiment tracking?", "What are the main components of MLflow?" ] # Evaluate using MLflow with mlflow.start_run(): for question in eval_questions: answer, sources = evaluate_rag(question) mlflow.log_param(f"question", question) mlflow.log_metric("num_sources", len(sources)) mlflow.log_text(answer, f"answer_question.txt") for i, source in enumerate(sources): mlflow.log_text(source, f"source_question_i.txt") # Log custom metrics mlflow.log_metric("avg_sources_per_question", sum(len(evaluate_rag(q)[1]) for q in eval_questions) / len(eval_questions))
This example sets up a RAG system using LangChain and Chroma, then evaluates it by logging questions, answers, retrieved sources, and custom metrics to MLflow.
The way you chunk your documents can significantly impact RAG performance. MLflow can help you evaluate different chunking strategies:
This script evaluates different combinations of chunk sizes, overlaps, and splitting methods, logging the results to MLflow for easy comparison.
MLflow provides various ways to visualize your LLM evaluation results. Here are some techniques:
You can create custom visualizations of your evaluation results using libraries like Matplotlib or Plotly, then log them as artifacts:
This function creates a line plot comparing a specific metric across multiple runs and logs it as an artifact.
#2023#ai#AI Tools 101#Analysis#API#approach#Artificial Intelligence#azure#azure openai#Behavior#code#col#Collections#communication#Community#comparison#complexity#comprehensive#computing#computing systems#content#credentials#custom metrics#data#data science#databricks#datasets#deploying#deployment#development
0 notes
Text
Generative AI Solutions | Samprasoft
Harness the power of SampraSoft's specialized Generative AI solutions, including strategic development, custom solution design, and data strategy. Benefit from our expertise to create innovative, customized solutions for your business. Partner with us for advanced Generative AI solutions that drive your success.
#Custom Software Development company#Generative AI Applications#Generative AI solutions#Generative AI Development services#databricks professional services#databricks consulting
0 notes
Text
Implementing Data Mesh on Databricks: Harmonized and Hub & Spoke Approaches
Explore the Harmonized and Hub & Spoke Data Mesh models on Databricks. Enhance data management with autonomous yet integrated domains and central governance. Perfect for diverse organizational needs and scalable solutions. #DataMesh #Databricks
View On WordPress
#Autonomous Data Domains#Data Governance#Data Interoperability#Data Lakes and Warehouses#Data Management Strategies#Data Mesh Architecture#Data Privacy and Security#Data Product Development#Databricks Lakehouse#Decentralized Data Management#Delta Sharing#Enterprise Data Solutions#Harmonized Data Mesh#Hub and Spoke Data Mesh#Modern Data Ecosystems#Organizational Data Strategy#Real-time Data Sharing#Scalable Data Infrastructures#Unity Catalog
0 notes
Text
Google Cloud’s BigQuery Autonomous Data To AI Platform

BigQuery automates data analysis, transformation, and insight generation using AI. AI and natural language interaction simplify difficult operations.
The fast-paced world needs data access and a real-time data activation flywheel. Artificial intelligence that integrates directly into the data environment and works with intelligent agents is emerging. These catalysts open doors and enable self-directed, rapid action, which is vital for success. This flywheel uses Google's Data & AI Cloud to activate data in real time. BigQuery has five times more organisations than the two leading cloud providers that just offer data science and data warehousing solutions due to this emphasis.
Examples of top companies:
With BigQuery, Radisson Hotel Group enhanced campaign productivity by 50% and revenue by over 20% by fine-tuning the Gemini model.
By connecting over 170 data sources with BigQuery, Gordon Food Service established a scalable, modern, AI-ready data architecture. This improved real-time response to critical business demands, enabled complete analytics, boosted client usage of their ordering systems, and offered staff rapid insights while cutting costs and boosting market share.
J.B. Hunt is revolutionising logistics for shippers and carriers by integrating Databricks into BigQuery.
General Mills saves over $100 million using BigQuery and Vertex AI to give workers secure access to LLMs for structured and unstructured data searches.
Google Cloud is unveiling many new features with its autonomous data to AI platform powered by BigQuery and Looker, a unified, trustworthy, and conversational BI platform:
New assistive and agentic experiences based on your trusted data and available through BigQuery and Looker will make data scientists, data engineers, analysts, and business users' jobs simpler and faster.
Advanced analytics and data science acceleration: Along with seamless integration with real-time and open-source technologies, BigQuery AI-assisted notebooks improve data science workflows and BigQuery AI Query Engine provides fresh insights.
Autonomous data foundation: BigQuery can collect, manage, and orchestrate any data with its new autonomous features, which include native support for unstructured data processing and open data formats like Iceberg.
Look at each change in detail.
User-specific agents
It believes everyone should have AI. BigQuery and Looker made AI-powered helpful experiences generally available, but Google Cloud now offers specialised agents for all data chores, such as:
Data engineering agents integrated with BigQuery pipelines help create data pipelines, convert and enhance data, discover anomalies, and automate metadata development. These agents provide trustworthy data and replace time-consuming and repetitive tasks, enhancing data team productivity. Data engineers traditionally spend hours cleaning, processing, and confirming data.
The data science agent in Google's Colab notebook enables model development at every step. Scalable training, intelligent model selection, automated feature engineering, and faster iteration are possible. This agent lets data science teams focus on complex methods rather than data and infrastructure.
Looker conversational analytics lets everyone utilise natural language with data. Expanded capabilities provided with DeepMind let all users understand the agent's actions and easily resolve misconceptions by undertaking advanced analysis and explaining its logic. Looker's semantic layer boosts accuracy by two-thirds. The agent understands business language like “revenue” and “segments” and can compute metrics in real time, ensuring trustworthy, accurate, and relevant results. An API for conversational analytics is also being introduced to help developers integrate it into processes and apps.
In the BigQuery autonomous data to AI platform, Google Cloud introduced the BigQuery knowledge engine to power assistive and agentic experiences. It models data associations, suggests business vocabulary words, and creates metadata instantaneously using Gemini's table descriptions, query histories, and schema connections. This knowledge engine grounds AI and agents in business context, enabling semantic search across BigQuery and AI-powered data insights.
All customers may access Gemini-powered agentic and assistive experiences in BigQuery and Looker without add-ons in the existing price model tiers!
Accelerating data science and advanced analytics
BigQuery autonomous data to AI platform is revolutionising data science and analytics by enabling new AI-driven data science experiences and engines to manage complex data and provide real-time analytics.
First, AI improves BigQuery notebooks. It adds intelligent SQL cells to your notebook that can merge data sources, comprehend data context, and make code-writing suggestions. It also uses native exploratory analysis and visualisation capabilities for data exploration and peer collaboration. Data scientists can also schedule analyses and update insights. Google Cloud also lets you construct laptop-driven, dynamic, user-friendly, interactive data apps to share insights across the organisation.
This enhanced notebook experience is complemented by the BigQuery AI query engine for AI-driven analytics. This engine lets data scientists easily manage organised and unstructured data and add real-world context—not simply retrieve it. BigQuery AI co-processes SQL and Gemini, adding runtime verbal comprehension, reasoning skills, and real-world knowledge. Their new engine processes unstructured photographs and matches them to your product catalogue. This engine supports several use cases, including model enhancement, sophisticated segmentation, and new insights.
Additionally, it provides users with the most cloud-optimized open-source environment. Google Cloud for Apache Kafka enables real-time data pipelines for event sourcing, model scoring, communications, and analytics in BigQuery for serverless Apache Spark execution. Customers have almost doubled their serverless Spark use in the last year, and Google Cloud has upgraded this engine to handle data 2.7 times faster.
BigQuery lets data scientists utilise SQL, Spark, or foundation models on Google's serverless and scalable architecture to innovate faster without the challenges of traditional infrastructure.
An independent data foundation throughout data lifetime
An independent data foundation created for modern data complexity supports its advanced analytics engines and specialised agents. BigQuery is transforming the environment by making unstructured data first-class citizens. New platform features, such as orchestration for a variety of data workloads, autonomous and invisible governance, and open formats for flexibility, ensure that your data is always ready for data science or artificial intelligence issues. It does this while giving the best cost and decreasing operational overhead.
For many companies, unstructured data is their biggest untapped potential. Even while structured data provides analytical avenues, unique ideas in text, audio, video, and photographs are often underutilised and discovered in siloed systems. BigQuery instantly tackles this issue by making unstructured data a first-class citizen using multimodal tables (preview), which integrate structured data with rich, complex data types for unified querying and storage.
Google Cloud's expanded BigQuery governance enables data stewards and professionals a single perspective to manage discovery, classification, curation, quality, usage, and sharing, including automatic cataloguing and metadata production, to efficiently manage this large data estate. BigQuery continuous queries use SQL to analyse and act on streaming data regardless of format, ensuring timely insights from all your data streams.
Customers utilise Google's AI models in BigQuery for multimodal analysis 16 times more than last year, driven by advanced support for structured and unstructured multimodal data. BigQuery with Vertex AI are 8–16 times cheaper than independent data warehouse and AI solutions.
Google Cloud maintains open ecology. BigQuery tables for Apache Iceberg combine BigQuery's performance and integrated capabilities with the flexibility of an open data lakehouse to link Iceberg data to SQL, Spark, AI, and third-party engines in an open and interoperable fashion. This service provides adaptive and autonomous table management, high-performance streaming, auto-AI-generated insights, practically infinite serverless scalability, and improved governance. Cloud storage enables fail-safe features and centralised fine-grained access control management in their managed solution.
Finaly, AI platform autonomous data optimises. Scaling resources, managing workloads, and ensuring cost-effectiveness are its competencies. The new BigQuery spend commit unifies spending throughout BigQuery platform and allows flexibility in shifting spend across streaming, governance, data processing engines, and more, making purchase easier.
Start your data and AI adventure with BigQuery data migration. Google Cloud wants to know how you innovate with data.
#technology#technews#govindhtech#news#technologynews#BigQuery autonomous data to AI platform#BigQuery#autonomous data to AI platform#BigQuery platform#autonomous data#BigQuery AI Query Engine
2 notes
·
View notes
Text
Azure Data Engineering Tools For Data Engineers
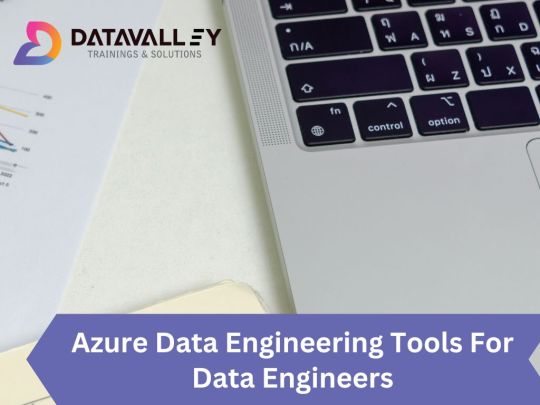
Azure is a cloud computing platform provided by Microsoft, which presents an extensive array of data engineering tools. These tools serve to assist data engineers in constructing and upholding data systems that possess the qualities of scalability, reliability, and security. Moreover, Azure data engineering tools facilitate the creation and management of data systems that cater to the unique requirements of an organization.
In this article, we will explore nine key Azure data engineering tools that should be in every data engineer’s toolkit. Whether you’re a beginner in data engineering or aiming to enhance your skills, these Azure tools are crucial for your career development.
Microsoft Azure Databricks
Azure Databricks is a managed version of Databricks, a popular data analytics and machine learning platform. It offers one-click installation, faster workflows, and collaborative workspaces for data scientists and engineers. Azure Databricks seamlessly integrates with Azure’s computation and storage resources, making it an excellent choice for collaborative data projects.
Microsoft Azure Data Factory
Microsoft Azure Data Factory (ADF) is a fully-managed, serverless data integration tool designed to handle data at scale. It enables data engineers to acquire, analyze, and process large volumes of data efficiently. ADF supports various use cases, including data engineering, operational data integration, analytics, and data warehousing.
Microsoft Azure Stream Analytics
Azure Stream Analytics is a real-time, complex event-processing engine designed to analyze and process large volumes of fast-streaming data from various sources. It is a critical tool for data engineers dealing with real-time data analysis and processing.
Microsoft Azure Data Lake Storage
Azure Data Lake Storage provides a scalable and secure data lake solution for data scientists, developers, and analysts. It allows organizations to store data of any type and size while supporting low-latency workloads. Data engineers can take advantage of this infrastructure to build and maintain data pipelines. Azure Data Lake Storage also offers enterprise-grade security features for data collaboration.
Microsoft Azure Synapse Analytics
Azure Synapse Analytics is an integrated platform solution that combines data warehousing, data connectors, ETL pipelines, analytics tools, big data scalability, and visualization capabilities. Data engineers can efficiently process data for warehousing and analytics using Synapse Pipelines’ ETL and data integration capabilities.
Microsoft Azure Cosmos DB
Azure Cosmos DB is a fully managed and server-less distributed database service that supports multiple data models, including PostgreSQL, MongoDB, and Apache Cassandra. It offers automatic and immediate scalability, single-digit millisecond reads and writes, and high availability for NoSQL data. Azure Cosmos DB is a versatile tool for data engineers looking to develop high-performance applications.
Microsoft Azure SQL Database
Azure SQL Database is a fully managed and continually updated relational database service in the cloud. It offers native support for services like Azure Functions and Azure App Service, simplifying application development. Data engineers can use Azure SQL Database to handle real-time data ingestion tasks efficiently.
Microsoft Azure MariaDB
Azure Database for MariaDB provides seamless integration with Azure Web Apps and supports popular open-source frameworks and languages like WordPress and Drupal. It offers built-in monitoring, security, automatic backups, and patching at no additional cost.
Microsoft Azure PostgreSQL Database
Azure PostgreSQL Database is a fully managed open-source database service designed to emphasize application innovation rather than database management. It supports various open-source frameworks and languages and offers superior security, performance optimization through AI, and high uptime guarantees.
Whether you’re a novice data engineer or an experienced professional, mastering these Azure data engineering tools is essential for advancing your career in the data-driven world. As technology evolves and data continues to grow, data engineers with expertise in Azure tools are in high demand. Start your journey to becoming a proficient data engineer with these powerful Azure tools and resources.
Unlock the full potential of your data engineering career with Datavalley. As you start your journey to becoming a skilled data engineer, it’s essential to equip yourself with the right tools and knowledge. The Azure data engineering tools we’ve explored in this article are your gateway to effectively managing and using data for impactful insights and decision-making.
To take your data engineering skills to the next level and gain practical, hands-on experience with these tools, we invite you to join the courses at Datavalley. Our comprehensive data engineering courses are designed to provide you with the expertise you need to excel in the dynamic field of data engineering. Whether you’re just starting or looking to advance your career, Datavalley’s courses offer a structured learning path and real-world projects that will set you on the path to success.
Course format:
Subject: Data Engineering Classes: 200 hours of live classes Lectures: 199 lectures Projects: Collaborative projects and mini projects for each module Level: All levels Scholarship: Up to 70% scholarship on this course Interactive activities: labs, quizzes, scenario walk-throughs Placement Assistance: Resume preparation, soft skills training, interview preparation
Subject:Â DevOps Classes: 180+ hours of live classes Lectures: 300 lectures Projects: Collaborative projects and mini projects for each module Level: All levels Scholarship: Up to 67% scholarship on this course Interactive activities: labs, quizzes, scenario walk-throughs Placement Assistance: Resume preparation, soft skills training, interview preparation
For more details on the Data Engineering courses, visit Datavalley’s official website.
#datavalley#dataexperts#data engineering#data analytics#dataexcellence#data science#power bi#business intelligence#data analytics course#data science course#data engineering course#data engineering training
3 notes
·
View notes
Text
PART TWO
The six men are one part of the broader project of Musk allies assuming key government positions. Already, Musk’s lackeys—including more senior staff from xAI, Tesla, and the Boring Company—have taken control of the Office of Personnel Management (OPM) and General Services Administration (GSA), and have gained access to the Treasury Department’s payment system, potentially allowing him access to a vast range of sensitive information about tens of millions of citizens, businesses, and more. On Sunday, CNN reported that DOGE personnel attempted to improperly access classified information and security systems at the US Agency for International Development and that top USAID security officials who thwarted the attempt were subsequently put on leave. The Associated Press reported that DOGE personnel had indeed accessed classified material.“What we're seeing is unprecedented in that you have these actors who are not really public officials gaining access to the most sensitive data in government,” says Don Moynihan, a professor of public policy at the University of Michigan. “We really have very little eyes on what's going on. Congress has no ability to really intervene and monitor what's happening because these aren't really accountable public officials. So this feels like a hostile takeover of the machinery of governments by the richest man in the world.”Bobba has attended UC Berkeley, where he was in the prestigious Management, Entrepreneurship, and Technology program. According to a copy of his now-deleted LinkedIn obtained by WIRED, Bobba was an investment engineering intern at the Bridgewater Associates hedge fund as of last spring and was previously an intern at both Meta and Palantir. He was a featured guest on a since-deleted podcast with Aman Manazir, an engineer who interviews engineers about how they landed their dream jobs, where he talked about those experiences last June.
Coristine, as WIRED previously reported, appears to have recently graduated from high school and to have been enrolled at Northeastern University. According to a copy of his résumé obtained by WIRED, he spent three months at Neuralink, Musk’s brain-computer interface company, last summer.Both Bobba and Coristine are listed in internal OPM records reviewed by WIRED as “experts” at OPM, reporting directly to Amanda Scales, its new chief of staff. Scales previously worked on talent for xAI, Musk’s artificial intelligence company, and as part of Uber’s talent acquisition team, per LinkedIn. Employees at GSA tell WIRED that Coristine has appeared on calls where workers were made to go over code they had written and justify their jobs. WIRED previously reported that Coristine was added to a call with GSA staff members using a nongovernment Gmail address. Employees were not given an explanation as to who he was or why he was on the calls.
Farritor, who per sources has a working GSA email address, is a former intern at SpaceX, Musk’s space company, and currently a Thiel Fellow after, according to his LinkedIn, dropping out of the University of Nebraska—Lincoln. While in school, he was part of an award-winning team that deciphered portions of an ancient Greek scroll.AdvertisementKliger, whose LinkedIn lists him as a special adviser to the director of OPM and who is listed in internal records reviewed by WIRED as a special adviser to the director for information technology, attended UC Berkeley until 2020; most recently, according to his LinkedIn, he worked for the AI company Databricks. His Substack includes a post titled “The Curious Case of Matt Gaetz: How the Deep State Destroys Its Enemies,” as well as another titled “Pete Hegseth as Secretary of Defense: The Warrior Washington Fears.”Killian, also known as Cole Killian, has a working email associated with DOGE, where he is currently listed as a volunteer, according to internal records reviewed by WIRED. According to a copy of his now-deleted résumé obtained by WIRED, he attended McGill University through at least 2021 and graduated high school in 2019. An archived copy of his now-deleted personal website indicates that he worked as an engineer at Jump Trading, which specializes in algorithmic and high-frequency financial trades.Shaotran told Business Insider in September that he was a senior at Harvard studying computer science and also the founder of an OpenAI-backed startup, Energize AI. Shaotran was the runner-up in a hackathon held by xAI, Musk’s AI company. In the Business Insider article, Shaotran says he received a $100,000 grant from OpenAI to build his scheduling assistant, Spark.
Are you a current or former employee with the Office of Personnel Management or another government agency impacted by Elon Musk? We’d like to hear from you. Using a nonwork phone or computer, contact Vittoria Elliott at [email protected] or securely at velliott88.18 on Signal.“To the extent these individuals are exercising what would otherwise be relatively significant managerial control over two very large agencies that deal with very complex topics,” says Nick Bednar, a professor at University of Minnesota’s school of law, “it is very unlikely they have the expertise to understand either the law or the administrative needs that surround these agencies.”Sources tell WIRED that Bobba, Coristine, Farritor, and Shaotran all currently have working GSA emails and A-suite level clearance at the GSA, which means that they work out of the agency’s top floor and have access to all physical spaces and IT systems, according a source with knowledge of the GSA’s clearance protocols. The source, who spoke to WIRED on the condition of anonymity because they fear retaliation, says they worry that the new teams could bypass the regular security clearance protocols to access the agency’s sensitive compartmented information facility, as the Trump administration has already granted temporary security clearances to unvetted people.This is in addition to Coristine and Bobba being listed as “experts” working at OPM. Bednar says that while staff can be loaned out between agencies for special projects or to work on issues that might cross agency lines, it’s not exactly common practice.“This is consistent with the pattern of a lot of tech executives who have taken certain roles of the administration,” says Bednar. “This raises concerns about regulatory capture and whether these individuals may have preferences that don’t serve the American public or the federal government.”
These men just stole the personal information of everyone in America AND control the Treasury. Link to article.
Akash Bobba
Edward Coristine
Luke Farritor
Gautier Cole Killian
Gavin Kliger
Ethan Shaotran
Spread their names!
#freedom of the press#elon musk#elongated muskrat#american politics#politics#news#america#trump administration
148K notes
·
View notes
Link
0 notes
Text

Hire Databricks developers to build scalable data pipelines, optimize Spark performance, and integrate AI/ML solutions.
0 notes
Text
Unlocking the Power of Delta Live Tables in Data bricks with Kadel Labs
Introduction
In the rapidly evolving landscape of big data and analytics, businesses are constantly seeking ways to streamline data processing, ensure data reliability, and improve real-time analytics. One of the most powerful solutions available today is Delta Live Tables (DLT) in Databricks. This cutting-edge feature simplifies data engineering and ensures efficiency in data pipelines.
Kadel Labs, a leader in digital transformation and data engineering solutions, leverages Delta Live Tables to optimize data workflows, ensuring businesses can harness the full potential of their data. In this article, we will explore what Delta Live Tables are, how they function in Databricks, and how Kadel Labs integrates this technology to drive innovation.
Understanding Delta Live Tables
What Are Delta Live Tables?
Delta Live Tables (DLT) is an advanced framework within Databricks that simplifies the process of building and maintaining reliable ETL (Extract, Transform, Load) pipelines. With DLT, data engineers can define incremental data processing pipelines using SQL or Python, ensuring efficient data ingestion, transformation, and management.
Key Features of Delta Live Tables
Automated Pipeline Management
DLT automatically tracks changes in source data, eliminating the need for manual intervention.
Data Reliability and Quality
Built-in data quality enforcement ensures data consistency and correctness.
Incremental Processing
Instead of processing entire datasets, DLT processes only new data, improving efficiency.
Integration with Delta Lake
DLT is built on Delta Lake, ensuring ACID transactions and versioned data storage.
Monitoring and Observability
With automatic lineage tracking, businesses gain better insights into data transformations.
How Delta Live Tables Work in Databricks
Databricks, a unified data analytics platform, integrates Delta Live Tables to streamline data lake house architectures. Using DLT, businesses can create declarative ETL pipelines that are easy to maintain and highly scalable.
The DLT Workflow
Define a Table and Pipeline
Data engineers specify data sources, transformation logic, and the target Delta table.
Data Ingestion and Transformation
DLT automatically ingests raw data and applies transformation logic in real-time.
Validation and Quality Checks
DLT enforces data quality rules, ensuring only clean and accurate data is processed.
Automatic Processing and Scaling
Databricks dynamically scales resources to handle varying data loads efficiently.
Continuous or Triggered Execution
DLT pipelines can run continuously or be triggered on-demand based on business needs.
Kadel Labs: Enhancing Data Pipelines with Delta Live Tables
As a digital transformation company, Kadel Labs specializes in deploying cutting-edge data engineering solutions that drive business intelligence and operational efficiency. The integration of Delta Live Tables in Databricks is a game-changer for organizations looking to automate, optimize, and scale their data operations.
How Kadel Labs Uses Delta Live Tables
Real-Time Data Streaming
Kadel Labs implements DLT-powered streaming pipelines for real-time analytics and decision-making.
Data Governance and Compliance
By leveraging DLT’s built-in monitoring and validation, Kadel Labs ensures regulatory compliance.
Optimized Data Warehousing
DLT enables businesses to build cost-effective data warehouses with improved data integrity.
Seamless Cloud Integration
Kadel Labs integrates DLT with cloud environments (AWS, Azure, GCP) to enhance scalability.
Business Intelligence and AI Readiness
DLT transforms raw data into structured datasets, fueling AI and ML models for predictive analytics.
Benefits of Using Delta Live Tables in Databricks
1. Simplified ETL Development
With DLT, data engineers spend less time managing complex ETL processes and more time focusing on insights.
2. Improved Data Accuracy and Consistency
DLT automatically enforces quality checks, reducing errors and ensuring data accuracy.
3. Increased Operational Efficiency
DLT pipelines self-optimize, reducing manual workload and infrastructure costs.
4. Scalability for Big Data
DLT seamlessly scales based on workload demands, making it ideal for high-volume data processing.
5. Better Insights with Lineage Tracking
Data lineage tracking in DLT provides full visibility into data transformations and dependencies.
Real-World Use Cases of Delta Live Tables with Kadel Labs
1. Retail Analytics and Customer Insights
Kadel Labs helps retailers use Delta Live Tables to analyze customer behavior, sales trends, and inventory forecasting.
2. Financial Fraud Detection
By implementing DLT-powered machine learning models, Kadel Labs helps financial institutions detect fraudulent transactions.
3. Healthcare Data Management
Kadel Labs leverages DLT in Databricks to improve patient data analysis, claims processing, and medical research.
4. IoT Data Processing
For smart devices and IoT applications, DLT enables real-time sensor data processing and predictive maintenance.
Conclusion
Delta Live Tables in Databricks is transforming the way businesses handle data ingestion, transformation, and analytics. By partnering with Kadel Labs, companies can leverage DLT to automate pipelines, improve data quality, and gain actionable insights.
With its expertise in data engineering, Kadel Labs empowers businesses to unlock the full potential of Databricks and Delta Live Tables, ensuring scalable, efficient, and reliable data solutions for the future.
For businesses looking to modernize their data architecture, now is the time to explore Delta Live Tables with Kadel Labs!
0 notes
Text
Databricks to infuse $250M to double its R&D staff in India this year
Databricks is planning to double its research and development (R&D) staff in India by the end of this year in an effort to accelerate the development of new capabilities and large language models (LLMs).  “This year, we plan to hire an additional 100-plus R&D engineers to strengthen our capabilities,” Vinod Marur, senior vice president of Engineering at Databricks, said during a media…
0 notes
Text
Exploring the Latest Features of Apache Spark 3.4 for Databricks Runtime
In the dynamic landscape of big data and analytics, staying at the forefront of technology is essential for organizations aiming to harness the full potential of their data-driven initiatives.
View On WordPress
#Apache Spark#API#Databricks#databricks apache spark#Databricks SQL#Dataframe#Developers#Filter Join#pyspark#pyspark for beginners#pyspark for data engineers#pyspark in azure databricks#Schema#Software Developers#Spark Cluster#Spark Connect#SQL#SQL SELECT#SQL Server
0 notes
Text
How Databricks Stock Reflects the Future of Enterprise Data Solutions
Databricks has become one of the most talked-about names in enterprise technology—and not just for its innovative data solutions. With ongoing discussions about a potential IPO and sky-high valuations in private markets, Databricks stock is attracting attention from both investors and tech leaders alike. But beyond the headlines, the buzz surrounding Databricks stock says a lot about where enterprise data solutions are heading.
Databricks isn’t just another software company. It was founded by the creators of Apache Spark, and since then, it has grown into a platform that powers data engineering, machine learning, and analytics—all in one place. Its signature product, the Lakehouse Platform, combines the flexibility of data lakes with the performance of data warehouses. This unified approach is solving a long-standing problem for businesses that have had to juggle multiple tools to get insights from their data.
As companies continue to move to the cloud and integrate AI into daily operations, they’re looking for platforms that can scale, automate, and deliver insights faster. Databricks has positioned itself as one of the few companies capable of meeting those needs at scale. Its platform is now being used by thousands of organizations worldwide, from early-stage startups to Fortune 500 enterprises.
The excitement around Databricks stock is a reflection of this broader trend. Investors are seeing more than just a profitable business—they’re seeing a company that sits at the center of the data revolution. Just like Snowflake’s IPO signaled a shift in how businesses think about cloud data warehousing, Databricks is now being seen as a key player in shaping the next chapter: unified data and AI-driven solutions.
This shift is not just technical—it’s strategic. Enterprises no longer view data as just a backend concern. It has become central to decision-making, customer experience, and product development. That means tools like Databricks are moving from IT departments into the core of business strategy. Companies want real-time insights, predictive analytics, and smarter automation—and they want it all in one platform.
If and when Databricks goes public, its stock could become a symbol of this transformation. It would mark a turning point where the market officially recognizes the value of platforms that offer a full stack of data capabilities—from ingestion to visualization, from model training to deployment.
Another reason Databricks stock is gaining attention is its strong track record of growth and innovation. The company has made bold investments in open-source technologies like Delta Lake, MLflow, and Apache Spark, all of which are now widely adopted across the industry. By staying close to the developer community while also scaling enterprise-grade features, Databricks has struck a rare balance that few companies manage to achieve.
There’s also the question of timing. As more businesses seek to integrate AI into their operations, the need for high-performance, AI-ready data infrastructure is becoming urgent. Databricks is already deeply embedded in the AI ecosystems of many major organizations, making it a natural choice for companies preparing for the next wave of digital transformation.
In short, the rising interest in Databricks stock isn’t just about financial returns. It reflects the growing importance of unified, intelligent data solutions in today’s enterprise environment. As organizations look for ways to stay competitive in a data-driven world, platforms like Databricks are quickly becoming foundational—not optional.
For businesses that are still relying on fragmented systems and outdated analytics tools, the rise of Databricks is a wake-up call. The future of enterprise data isn’t about collecting information—it’s about turning it into action, faster and smarter than ever before. Databricks stock might not be available on the public market just yet, but the message is already clear: the future of enterprise data is unified, AI-ready, and powered by platforms that can handle it all.
0 notes