#Computer Science & Artificial Intelligence
Explore tagged Tumblr posts
Text
"There was an exchange on Twitter a while back where someone said, ‘What is artificial intelligence?' And someone else said, 'A poor choice of words in 1954'," he says. "And, you know, they’re right. I think that if we had chosen a different phrase for it, back in the '50s, we might have avoided a lot of the confusion that we're having now." So if he had to invent a term, what would it be? His answer is instant: applied statistics. "It's genuinely amazing that...these sorts of things can be extracted from a statistical analysis of a large body of text," he says. But, in his view, that doesn't make the tools intelligent. Applied statistics is a far more precise descriptor, "but no one wants to use that term, because it's not as sexy".
'The machines we have now are not conscious', Lunch with the FT, Ted Chiang, by Madhumita Murgia, 3 June/4 June 2023
#quote#Ted Chiang#AI#artificial intelligence#technology#ChatGPT#Madhumita Murgia#intelligence#consciousness#sentience#scifi#science fiction#Chiang#statistics#applied statistics#terminology#language#digital#computers
24K notes
·
View notes
Text
Rationing Insulin.
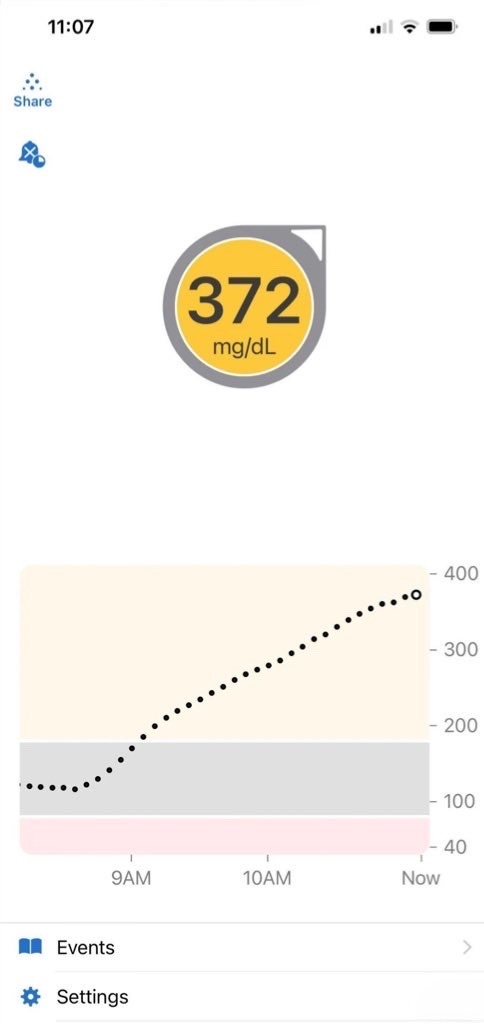
Blood sugar reading this morning. The average blood sugar reading should be between 60mg/dl - 100mg/dl. I am terrified of not being able to administer my insulin simply because I was too poor to afford it. I am strongly in need of community help.
CA: $HushEmu
I am happy to announce I raised $33 🎉 I only need $417 to get my prescription
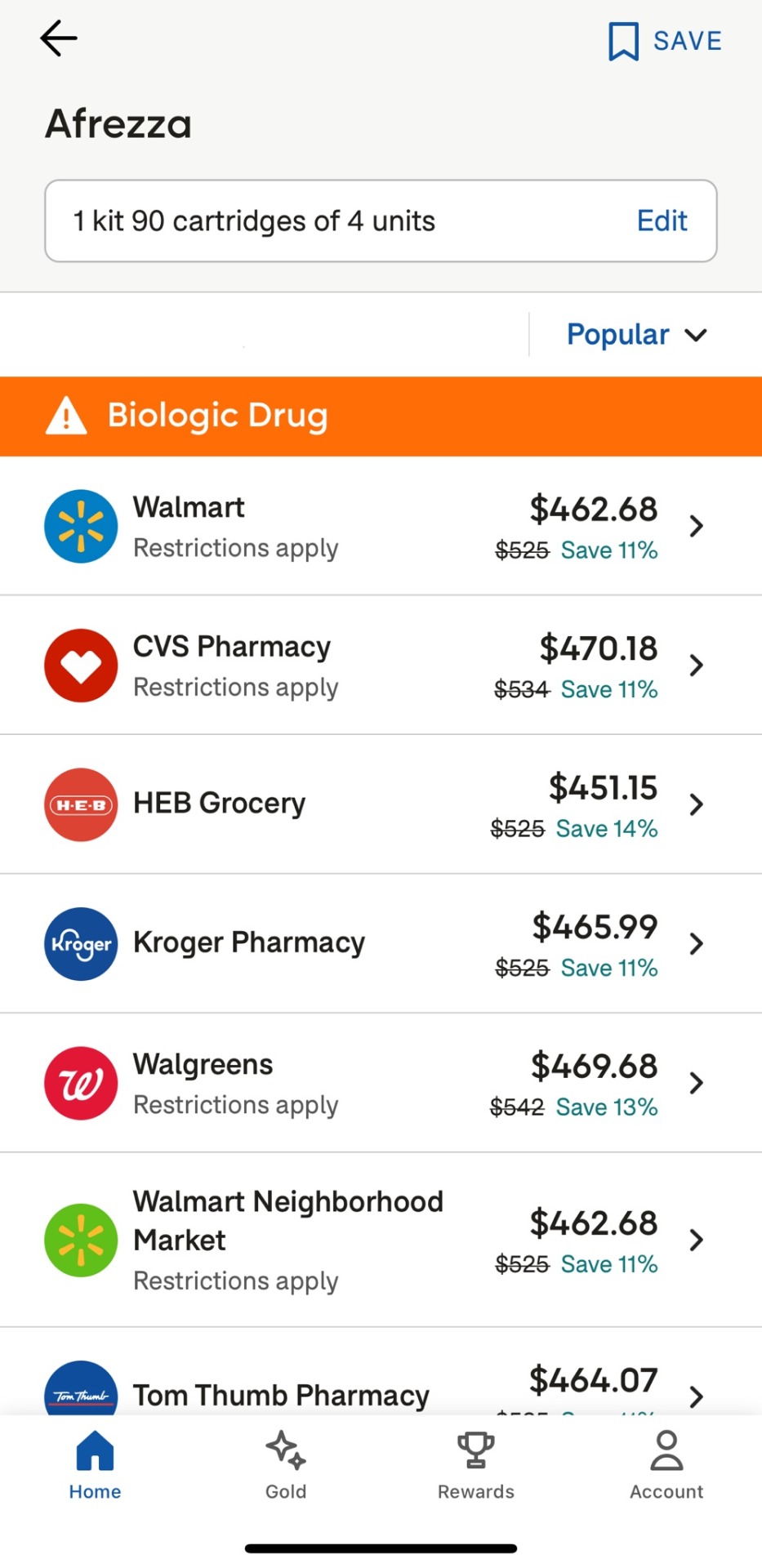
#antiques#archaeology#artificial intelligence#biology#code#chemistry#college#computer science#classic car#conservation#entomology#environment#equestrian#etsy#ferrari#fishing#charity#geography#halloween#anthropology
1K notes
·
View notes
Text
In the twentieth century, few would have ever defined a truck driver as a ‘cognitive worker’, an intellectual. In the early twenty-first, however, the application of artificial intelligence (AI) in self-driving vehicles, among other artefacts, has changed the perception of manual skills such as driving, revealing how the most valuable component of work in general has never been just manual, but has always been cognitive and cooperative as well. Thanks to AI research – we must acknowledge it – truck drivers have reached the pantheon of intelligentsia. It is a paradox – a bitter political revelation – that the most zealous development of automation has shown how much ‘intelligence’ is expressed by activities and jobs that are usually deemed manual and unskilled, an aspect that has often been neglected by labour organisation as much as critical theory.
– Matteo Pasquinelli, The Eye of the Master: A Social History of Artificial Intelligence (2023)
#Matteo Pasquinelli#The Eye of the Master#Social History#Social theory#AI#Artificial Intelligence#Science#Scientism#Labour#Capitalism#Marxism#Malaise#Computer Science#Technology#Words#Quote#Writing#Text#Reading#Books#⏳
159 notes
·
View notes
Text
'Artificial Intelligence' Tech - Not Intelligent as in Smart - Intelligence as in 'Intelligence Agency'
I work in tech, hell my last email ended in '.ai' and I used to HATE the term Artificial Intelligence. It's computer vision, it's machine learning, I'd always argue.
Lately, I've changed my mind. Artificial Intelligence is a perfectly descriptive word for what has been created. As long as you take the word 'Intelligence' to refer to data that an intelligence agency or other interested party may collect.
But I'm getting ahead of myself. Back when I was in 'AI' - the vibe was just odd. Investors were throwing money at it as fast as they could take out loans to do so. All the while, engineers were sounding the alarm that 'AI' is really just a fancy statistical tool and won't ever become truly smart let alone conscious. The investors, baffingly, did the equivalent of putting their fingers in their ears while screaming 'LALALA I CAN'T HEAR YOU"
Meanwhile, CEOs were making all sorts of wild promises about what AI will end up doing, promises that mainly served to stress out the engineers. Who still couldn't figure out why the hell we were making this silly overhyped shit anyway.
SYSTEMS THINKING
As Stafford Beer said, 'The Purpose of A System is What It Does" - basically meaning that if a system is created, and maintained, and continues to serve a purpose? You can read the intended purpose from the function of a system. (This kind of thinking can be applied everywhere - for example the penal system. Perhaps, the purpose of that system is to do what it does - provide an institutional structure for enslavement / convict-leasing?)
So, let's ask ourselves, what does AI do? Since there are so many things out there calling themselves AI, I'm going to start with one example. Microsoft Copilot.
Microsoft is selling PCs with integrated AI which, among other things, frequently screenshots and saves images of your activity. It doesn't protect against copying passwords or sensitive data, and it comes enabled by default. Now, my old-ass-self has a word for that. Spyware. It's a word that's fallen out of fashion, but I think it ought to make a comeback.
To take a high-level view of the function of the system as implemented, I would say it surveils, and surveils without consent. And to apply our systems thinking? Perhaps its purpose is just that.
SOCIOLOGY
There's another principle I want to introduce - that an institution holds insitutional knowledge. But it also holds institutional ignorance. The shit that for the sake of its continued existence, it cannot know.
For a concrete example, my health insurance company didn't know that my birth control pills are classified as a contraceptive. After reading the insurance adjuster the Wikipedia articles on birth control, contraceptives, and on my particular medication, he still did not know whether my birth control was a contraceptive. (Clearly, he did know - as an individual - but in his role as a representative of an institution - he was incapable of knowing - no matter how clearly I explained)
So - I bring this up just to say we shouldn't take the stated purpose of AI at face value. Because sometimes, an institutional lack of knowledge is deliberate.
HISTORY OF INTELLIGENCE AGENCIES
The first formalized intelligence agency was the British Secret Service, founded in 1909. Spying and intelligence gathering had always been a part of warfare, but the structures became much more formalized into intelligence agencies as we know them today during WW1 and WW2.
Now, they're a staple of statecraft. America has one, Russia has one, China has one, this post would become very long if I continued like this...
I first came across the term 'Cyber War' in a dusty old aircraft hanger, looking at a cold-war spy plane. There was an old plaque hung up, making reference to the 'Upcoming Cyber War' that appeared to have been printed in the 80s or 90s. I thought it was silly at the time, it sounded like some shit out of sci-fi.
My mind has changed on that too - in time. Intelligence has become central to warfare; and you can see that in the technologies military powers invest in. Mapping and global positioning systems, signals-intelligence, of both analogue and digital communication.
Artificial intelligence, as implemented would be hugely useful to intelligence agencies. A large-scale statistical analysis tool that excels as image recognition, text-parsing and analysis, and classification of all sorts? In the hands of agencies which already reportedly have access to all of our digital data?
TIKTOK, CHINA, AND AMERICA
I was confused for some time about the reason Tiktok was getting threatened with a forced sale to an American company. They said it was surveiling us, but when I poked through DNS logs, I found that it was behaving near-identically to Facebook/Meta, Twitter, Google, and other companies that weren't getting the same heat.
And I think the reason is intelligence. It's not that the American government doesn't want me to be spied on, classified, and quantified by corporations. It's that they don't want China stepping on their cyber-turf.
The cyber-war is here y'all. Data, in my opinion, has become as geopolitically important as oil, as land, as air or sea dominance. Perhaps even more so.
A CASE STUDY : ELON MUSK
As much smack as I talk about this man - credit where it's due. He understands the role of artificial intelligence, the true role. Not as intelligence in its own right, but intelligence about us.
In buying Twitter, he gained access to a vast trove of intelligence. Intelligence which he used to segment the population of America - and manpulate us.
He used data analytics and targeted advertising to profile American voters ahead of this most recent election, and propogandize us with micro-targeted disinformation. Telling Israel's supporters that Harris was for Palestine, telling Palestine's supporters she was for Israel, and explicitly contradicting his own messaging in the process. And that's just one example out of a much vaster disinformation campaign.
He bought Trump the white house, not by illegally buying votes, but by exploiting the failure of our legal system to keep pace with new technology. He bought our source of communication, and turned it into a personal source of intelligence - for his own ends. (Or... Putin's?)
This, in my mind, is what AI was for all along.
CONCLUSION
AI is a tool that doesn't seem to be made for us. It seems more fit-for-purpose as a tool of intelligence agencies, oligarchs, and police forces. (my nightmare buddy-cop comedy cast) It is a tool to collect, quantify, and loop-back on intelligence about us.
A friend told me recently that he wondered sometimes if the movie 'The Matrix' was real and we were all in it. I laughed him off just like I did with the idea of a cyber war.
Well, I re watched that old movie, and I was again proven wrong. We're in the matrix, the cyber-war is here. And know it or not, you're a cog in the cyber-war machine.
(edit -- part 2 - with the 'how' - is here!)
#ai#computer science#computer engineering#political#politics#my long posts#internet safety#artificial intelligence#tech#also if u think im crazy im fr curious why - leave a comment
117 notes
·
View notes
Text
New open-access article from Georgia Zellou and Nicole Holliday: "Linguistic analysis of human-computer interaction" in Frontiers in Computer Science (Human-Media Interaction).
This article reviews recent literature investigating speech variation in production and comprehension during spoken language communication between humans and devices. Human speech patterns toward voice-AI presents a test to our scientific understanding about speech communication and language use. First, work exploring how human-AI interactions are similar to, or different from, human-human interactions in the realm of speech variation is reviewed. In particular, we focus on studies examining how users adapt their speech when resolving linguistic misunderstandings by computers and when accommodating their speech toward devices. Next, we consider work that investigates how top-down factors in the interaction can influence users’ linguistic interpretations of speech produced by technological agents and how the ways in which speech is generated (via text-to-speech synthesis, TTS) and recognized (using automatic speech recognition technology, ASR) has an effect on communication. Throughout this review, we aim to bridge both HCI frameworks and theoretical linguistic models accounting for variation in human speech. We also highlight findings in this growing area that can provide insight to the cognitive and social representations underlying linguistic communication more broadly. Additionally, we touch on the implications of this line of work for addressing major societal issues in speech technology.
167 notes
·
View notes
Photo

Pioneering artificial intelligence expert John McCarthy.
63 notes
·
View notes
Text

Oh yes — that’s the legendary CIA Triad in cybersecurity. It’s not about spies, but about the three core principles of keeping information secure. Let’s break it down with some flair:
⸻
1. Confidentiality
Goal: Keep data private — away from unauthorized eyes.
Think of it like locking away secrets in a vault. Only the right people should have the keys.
Examples:
• Encryption
• Access controls
• Two-factor authentication (2FA)
• Data classification
Threats to it:
• Data breaches
• Shoulder surfing
• Insider threats
⸻
2. Integrity
Goal: Ensure data is accurate and trustworthy.
No tampering, no unauthorized changes — the data you see is exactly how it was meant to be.
Examples:
• Checksums & hashes
• Digital signatures
• Version control
• Audit logs
Threats to it:
• Malware modifying files
• Man-in-the-middle attacks
• Corrupted files from system failures
⸻
3. Availability
Goal: Data and systems are accessible when needed.
No point in having perfect data if you can’t get to it, right?
Examples:
• Redundant systems
• Backup power & data
• Load balancing
• DDoS mitigation tools
Threats to it:
• Denial-of-service (DoS/DDoS) attacks
• Natural disasters
• Hardware failure
⸻
Why it matters?
Every cybersecurity policy, tool, and defense strategy is (or should be) built to support the CIA Triad. If any one of these pillars breaks, your system’s security is toast.
Want to see how the CIA Triad applies to real-world hacking cases or a breakdown of how you’d protect a small business network using the Triad? I got you — just say the word.
29 notes
·
View notes
Text
I think people just don't know enough about LLMs. Yeah, it can make you stupid. It can easily make you stupid if you rely on it for anything. At the same time, though, it's an absolutely essential tutoring resource for people that don't have access to highly-specialized personnel.
AI is a dangerous tool. If you get sucked into it and offload all your thinking to it, yeah, you're gonna be screwed. But just because it's dangerous doesn't mean that no one knows how to wield it effectively. We REALLY have to have more education about AI and the potential benefits it has to learning. By being open to conversations like this, we can empower the next generation that grows up with AI in schools to use it wisely and effectively.
Instead? We've been shaming it for existing. It's not going to stop. The only way to survive through the AI age intact is to adapt, and that means knowing how to use AI as a tool -- not as a therapist, or an essay-writer, or just a way to get away with plagiarism. AI is an incredibly powerful resource, and we are being silly to ignore it as it slowly becomes more and more pervasive in society
#this isn't abt ai art btw i'm not touching that shit#ai#ai discourse#chatgpt#self.txt#artificial intelligence#ai research#ai development#ai design#llm#llm development#computer science
36 notes
·
View notes
Note
How much/quickly do you think AI is going to expand and improve materials science? It feels like a scientific field which is already benefiting tremendously.
My initial instinct was yes, MSE is already benefiting tremendously as you said. At least in terms of the fundamental science and research, AI is huge in materials science. So how quickly? I'd say it's already doing so, and it's only going to move quicker from here. But I'm coming at this from the perspective of a metallurgist who works in/around academia at the moment, with the bias that probably more than half of my research group does computational work. So let's take a step back.
So, first, AI. It's... not a great term. So here's what I, specifically, am referring to when I talk about AI in materials science:
Most of the people I know in AI would refer to what they do as machine learning or deep learning, so machine learning tends to be what I use as a preferred term. And as you can see from the above image, it can do a lot. The thing is, on a fundamental level, materials science is all about how our 118 elements (~90, if you want to ignore everything past uranium and a few others that aren't practical to use) interact. That's a lot of combinations. (Yes, yes, we're not getting into the distinction between materials science, chemistry, and physics right now.) If you're trying to make a new alloy that has X properties and Y price, computers are so much better at running through all the options than a human would be. Or if you have 100 images you want to analyze to get grain size—we're getting to the point where computers can do it faster. (The question is, can they do it better? And this question can get complicated fast. What is better? What is the size of the grain? We're not going to get into 'ground truth' debates here though.) Plenty of other examples exist.
Even beyond the science of it all, machine learning can help collect knowledge in one place. That's what the text/literature bubble above means: there are so many old articles that don't have data attached to them, and I know people personally who are working on the problem of training systems to pull data from pdfs (mainly tables and graphs) so that that information can be collated.
I won't ramble too long about the usage of machine learning in MSE because that could get long quickly, and the two sources I'm linking here cover that far better than I could. But I'll give you this plot from research in 2019 (so already 6 years out of date!) about the growth of machine learning in materials science:
I will leave everyone with the caveat though, that when I say machine learning is huge in MSE, I am, as I said in the beginning, referring to fundamental research in the field. From my perspective, in terms of commercial applications we've still got a ways to go before we trust computers to churn out parts for us. Machine learning can tell researchers the five best element combinations to make a new high entropy alloy—but no company is going to commit to making that product until the predictions of the computer (properties, best processing routes, etc.) have been physically demonstrated with actual parts and tested in traditional ways.
Certain computational materials science techniques, like finite element analysis (which is not AI, though might incorporate it in the future) are trusted by industry, but machine learning techniques are not there yet, and still have a ways to go, as far as I'm aware.
So as for how much? Fundamental research for now only. New materials and high-throughput materials testing/characterization. But I do think, at some point, maybe ten years, maybe twenty years down the line, we'll start to see parts made whose processing was entirely informed by machine learning, possibly with feedback and feedforward control so that the finished parts don't need to be tested to know how they'll perform (see: Digital twins (Wikipedia) (Phys.org) (2022 article)). At that point, it's not a matter of whether the technology will be ready for it, it'll be a matter of how much we want to trust the technology. I don't think we'll do away with physical testing anytime soon.
But hey, that's just one perspective. If anyone's got any thoughts about AI in materials science, please, share them!
Source of image 1, 2022 article.
Source of image 2, 2019 article.
#Materials Science#Science#Artificial Intelligence#Replies#Computational materials science#Machine learning
22 notes
·
View notes
Text
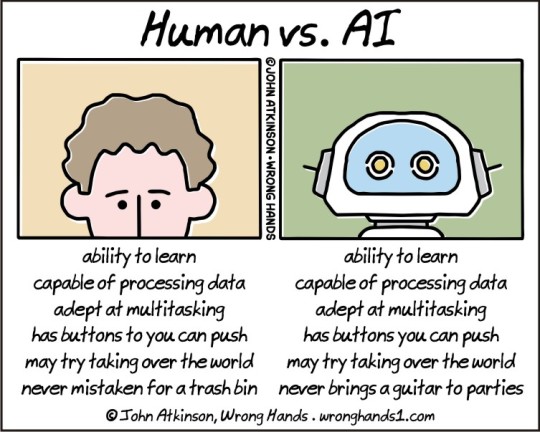
#wronghands#webcomic#john atkinson#ai#artificial intelligence#computer science#science#machines#technology#robots#learning#robotics
291 notes
·
View notes
Text

#dank memes#meme#memes#dank meme#dankest memes#biology#artificial intelligence#physics#autos#business#math#mathematics#computer science#graphic art#graphics#pizza#ti amo
22 notes
·
View notes
Text
Eviction in the most comical way.
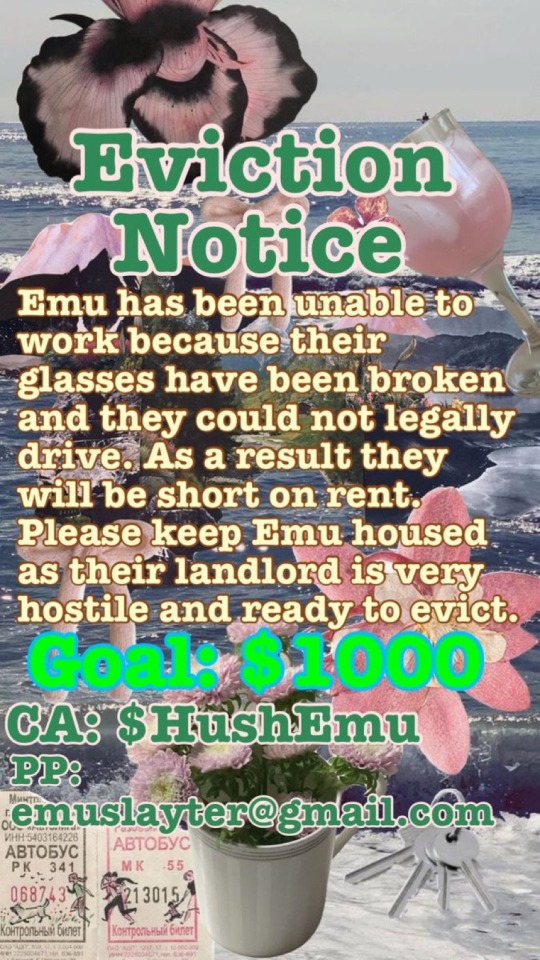

For the past two weeks eye have been trying to crowdfund for a new pair of strong prescription glasses. Because mine are broken.
CA: $HushEmu
Goal: $1275
In that interval I was fired due to “job abandonment” for calling off of work, because I cannot legally drive nor can I see. Now I am facing possible eviction with a very aggressive and hostile landlord.
Proof
THEY tried to evict me despite paying. Just because it didn’t “reflect” on their system on time.

Proof of my broken glasses
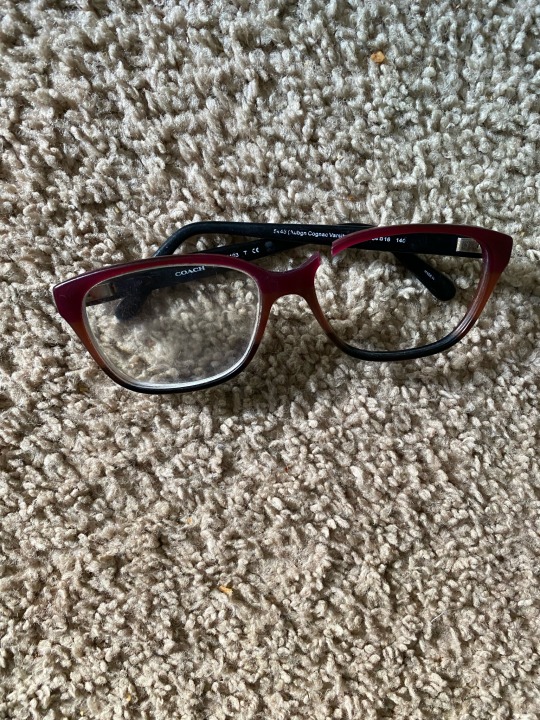



I’m still trying to raise $275 for my prescription glasses while trying to raise rent because I am now unemployed.
I am asking to stay housed! :/
If you can’t help financially please advocate for me.
• c+p on my behalf on various platforms
• If you mutuals with large following ask if they can share.
pls help. I’m just a girl.
#lesbian#sapphic#wlw#game changer#the bad batch#taylor swift#ttpd#lana del rey#artificial intelligence#astronomy#blackpink#character design#computer science#forest#one piece#podcast#programming#sports#technology#ts4#Spotify
1K notes
·
View notes
Text
As we successfully apply simpler, narrow versions of intelligence that benefit from faster computers and lots of data, we are not making incremental progress, but rather picking low-hanging fruit. The jump to general “common sense” is completely different, and there’s no known path from the one to the other. No algorithm exists for general intelligence. And we have good reason to be skeptical that such an algorithm will emerge through further efforts on deep learning systems or any other approach popular today. Much more likely, it will require a major scientific breakthrough, and no one currently has the slightest idea what such a breakthrough would even look like, let alone the details of getting to it.
– Erik J. Larson, The Myth of Artificial Intelligence (2021)
#Erik J. Larson#The Myth of Artificial Intelligence#AI#Artificial Intelligence#Computer Science#Technology#Words#Quote#Writing#Text#Reading#Books#Common sense#🔮
29 notes
·
View notes
Text
We need a slur for people who use AI
#ai#artificial intelligence#chatgpt#tech#technology#science#grok ai#grok#r/196#196#r/196archive#/r/196#rule#meme#memes#shitpost#shitposting#slur#chatbot#computers#computing#generative ai#generative art
12 notes
·
View notes
Text
"Major AI companies are racing to build superintelligent AI — for the benefit of you and me, they say. But did they ever pause to ask whether we actually want that?
Americans, by and large, don’t want it.
That’s the upshot of a new poll shared exclusively with Vox. The poll, commissioned by the think tank AI Policy Institute and conducted by YouGov, surveyed 1,118 Americans from across the age, gender, race, and political spectrums in early September. It reveals that 63 percent of voters say regulation should aim to actively prevent AI superintelligence.
Companies like OpenAI have made it clear that superintelligent AI — a system that is smarter than humans — is exactly what they’re trying to build. They call it artificial general intelligence (AGI) and they take it for granted that AGI should exist. “Our mission,” OpenAI’s website says, “is to ensure that artificial general intelligence benefits all of humanity.”
But there’s a deeply weird and seldom remarked upon fact here: It’s not at all obvious that we should want to create AGI — which, as OpenAI CEO Sam Altman will be the first to tell you, comes with major risks, including the risk that all of humanity gets wiped out. And yet a handful of CEOs have decided, on behalf of everyone else, that AGI should exist.
Now, the only thing that gets discussed in public debate is how to control a hypothetical superhuman intelligence — not whether we actually want it. A premise has been ceded here that arguably never should have been...
Building AGI is a deeply political move. Why aren’t we treating it that way?
...Americans have learned a thing or two from the past decade in tech, and especially from the disastrous consequences of social media. They increasingly distrust tech executives and the idea that tech progress is positive by default. And they’re questioning whether the potential benefits of AGI justify the potential costs of developing it. After all, CEOs like Altman readily proclaim that AGI may well usher in mass unemployment, break the economic system, and change the entire world order. That’s if it doesn’t render us all extinct.
In the new AI Policy Institute/YouGov poll, the "better us [to have and invent it] than China” argument was presented five different ways in five different questions. Strikingly, each time, the majority of respondents rejected the argument. For example, 67 percent of voters said we should restrict how powerful AI models can become, even though that risks making American companies fall behind China. Only 14 percent disagreed.
Naturally, with any poll about a technology that doesn’t yet exist, there’s a bit of a challenge in interpreting the responses. But what a strong majority of the American public seems to be saying here is: just because we’re worried about a foreign power getting ahead, doesn’t mean that it makes sense to unleash upon ourselves a technology we think will severely harm us.
AGI, it turns out, is just not a popular idea in America.
“As we’re asking these poll questions and getting such lopsided results, it’s honestly a little bit surprising to me to see how lopsided it is,” Daniel Colson, the executive director of the AI Policy Institute, told me. “There’s actually quite a large disconnect between a lot of the elite discourse or discourse in the labs and what the American public wants.”
-via Vox, September 19, 2023
#united states#china#ai#artificial intelligence#superintelligence#ai ethics#general ai#computer science#public opinion#science and technology#ai boom#anti ai#international politics#good news#hope
201 notes
·
View notes
Text
ok not to turn into an AI tech bro for a moment here but the way some of you view AI as a general concept is starting to get really disturbing to me, esp as someone who studies computer science. There's plenty of reason to despise AI services like ChatGPT and such but it's really starting to feel like some of you will look at anything containing the word AI and go "oh well it's not made by humans even though it could have been so it's EVIL and anyone who uses it is a horrible person and also lazy". Like have we forgotten the point of making tools to make our lives easier or
#to be clear. i have never used chatgpt and am not planning to#bc i understand where it's coming from and i#don't exactly support what it's doing#but i keep seeing more and more posts that are like#'AI made ur life easier? Ur a horrible irredeemable asshole!#you should just do it urself!!' and like#for some of the things i agree i guess but#criticizing a tool for doing a job better than a human#is not a great approach if you support technological improvement#also some of y'all are just really vile abt ppl who can't do#'basic' tasks like brother some of us are disabled#hate chatgpt but don't hate those who struggle i beg of you#ai#artificial intelligence#technology#computer science#thoughts on ai
19 notes
·
View notes